- School of Maritime Economics and Management, Dalian Maritime University, Dalian, China
As urban amenity welfare, exotic food is related to consumers’ equal access to diversified food and a healthy diet. This study uses big data provided by an online catering platform to explore Japanese cuisine in China. The field intensity model and spatial econometric model are used to analyze the place effect and its relationship with local socioeconomic factors. The results illustrate that 1) the spatial distribution of Japanese cuisine shows the characteristics of an unbalanced agglomeration distribution, with the coastal economically developed cities as the key layout area and gradually extending to inland cities. 2) Price characteristics indicate that the service target of Japanese cuisine is mainly the middle class. In addition, the spatial inequality of field intensity value indicates that wealthy Eastern cities have more opportunities to enjoy more kinds of and higher quality exotic food. 3) In the local socioeconomic environment, urbanization level, population size, and economic scale are significantly related to inequal access to Japanese cuisine. The essential mechanism of these circumstances is the internal needs of pricing characteristics and the negative externalities caused by unequal urban infrastructure.
1 Introduction
Love for variety is a common assumption of consumers in Modern Economics (Glaeser et al., 2001; Nathan, 2015). Rich and diverse diet not only can improve the supporting services of urbanization construction but also is a strong force to shape urban amenity welfare. With the increasing demand for food diversity and the advancement of globalization, more and more dietary choices are made for cross-local production (Willmott, 2007).
As a typical representative of space production, exotic food is a process of production of space, which constantly exceeds the limits of geographical space (Zeng and Liu, 2013). In different socio-economic environments, exotic food is providing differentiated services to meet people’s diverse food preferences and needs (Armington, 1969; Clark et al., 2017). Its spatial distribution is related to whether consumers can have equal access to food and nutrients (Çanakçı and Birdir, 2020), and it affects the comfort and welfare level of cities (Glaeser et al., 2001). However, affected by spatial and non-spatial mediating factors (including geographical, economic, information, and cultural aspects), the distribution of food is often unbalanced, which leads to inequality of access to food and barriers to choice for consumers (Chen and Yang, 2014).
Most of the existing studies describe the spatial inequality of food by analyzing the differences of food environments in different places (An and Sturm, 2012; Caspi et al., 2012; Swinburn et al., 2015; An et al., 2020). Researchers usually describe food environment in terms of families, streets, and communities, such as
• Family food environment usually includes physical environment (e.g., family food supply) and socio-cultural environment (e.g., parenting styles, practices, and rules) (Adams et al., 2020; Jang et al., 2020);
• The street food environment was quantified as healthy food sources (chain stores and independent grocery stores, fruit and vegetable suppliers, and supermarkets) and unhealthy food sources (chain and independent convenience stores, fast food, and alcohol stores) (Lytle and Sokol, 2017; Anderson et al., 2020);
• The community food environment “comprises the foods available to people in their surroundings as they go about their everyday lives and the nutritional quality, safety, price, convenience, labeling, and promotion of these foods” (Black et al., 2014; Cobb et al., 2015; FAO-Food and Agriculture Organization of the United Nations, 2016; Luciene et al., 2020).
The abovementioned studies have found that regional differences in food environment will lead to food access inequalities in food clusters, eating patterns, and access routes in different spatial places, which will have many adverse effects on resident welfare and health status (Leslie et al., 2012; Chen and Yang, 2014; Eckert and Vojnovic, 2017; Garcia et al., 2020). However, the abovementioned research also has some limitations: 1) researchers mainly focus on the supply system and access of local food and interpret its relationship with the food environment of the community and street, but few researchers pay attention to exotic food; 2) by means of interviews and questionnaires, the research conclusion does not seem to be able to describe the food environment of urban units well.
The abovementioned findings confirm that urban food environment should include the sum of the effects of environment, opportunity, or living conditions on the health and eating behavior of individuals or people (Kwate and Loh, 2016; Osei-Kwasi et al., 2020). With the need for multiscale and three-dimensional research, it is more practical to measure the diversity of food environment at the urban level and its impact on food inequality. Especially with the acceleration of globalization and population flow, food from all over the world is part of the lives and social activities of residents in different cities in a variety of forms. To some extent, these foreign foods have affected the quality of life of urban residents and have become an important part of the local unique social landscape of the city. Social economic factors such as residential population, travel behavior, and mobility related to food environment have attracted more and more attention (Eckert and Vojnovic, 2017; Li and Kim, 2020).
The introduction of exotic food will bring about the remould and reframe of local food supply channels and supply structure. However, the local food environment with strong rooting will reverse the emergence of exotic food and form new service features (for example, deep-fried dough sticks and soybean milk appear on the menus of McDonald’s and KFC in China) (Shen and Xiao, 2014; Rui et al., 2016). It is a city problem worthy of attention to analyze whether the influx and development of exotic food will improve the city’s food access and purchase behavior.
As an important market for exotic food, China has a large consumer group, diverse dietary needs, unique food culture, and dramatic social and economic evolution. With the rise of e-commerce and online catering platforms, exotic food has formed a unique service characteristic and distribution status in China. It is more representative and typical to analyze the distribution characteristics of exotic foods and its relationship with the urban food environment. As one of the important forms of exotic food, Japanese cuisine is an important cooking school in the world. Compared with other exotic foods in China, the number of Japanese restaurants in 2019 is close to 70,000 (ranking first in the world), which has become the “leader” of exotic foods in China (data from dianping.com). According to the survey report of China’s restaurant industry in 2019, China’s catering revenue in 2018 was 4,271.6 billion yuan, 780 times that of 1978, while foreign catering accounted for 26.24% of that revenue, which has become an important part of China’s catering industry. Taking Japanese restaurants as an example, our statistics show that the number of Japanese restaurants in China in 2022 increased by 13,678 compared with that in 2019.
Simultaneously, big data thinking has brought about a revolution in scientific research methods, and the data-intensive research paradigm has been widely recognized. An increasing number of scholars have obtained internet spatial–temporal data, such as the facility interest points of internet maps and social media check-in interest points, and analyzed and explored issues related to urban space through a new data-oriented research paradigm. In this study, Japanese cuisine in China is taken as a representative of exotic food, and the larger sample size of internet big data is used to describe the service characteristics and identify the internal relationship between the service characteristics and local food environment. The purpose of this study is to analyze whether the continuous influx of exotic food can alleviate the disadvantage of vulnerable group access to food diversity, meet the needs of theoretical development of dietary communication, and promote international dialogue on diet and explore the pricing strategy and distribution pattern of exotic food in consumption areas, which can also influence the diet structure, healthy diet concept, and nutrition intake of consumption areas. The approach provides evidence to promote the reproduction of traditional diet and healthy diet. The contribution of this study is as follows: It is the first time the data of online catering websites have been used to discuss the geographical pattern of Japanese restaurants in China and analyze the price differences of Japanese restaurants in 332 cities in China. In addition, we discuss the spatial distribution differences of Japanese restaurants and the relationship between urban, social, and economic factors.
2 Materials and Methods
2.1 Data
The traditional statistical data of the catering industry have some shortcomings, such as incomplete classification information and time lag. With the rapid development of internet technology, big data represented by mobile phone signaling data, GPS trajectory data, and POI (point of interest) data have been constantly enriched and improved. As a new spatial data source, POI data have the advantages of a large amount of data, wide coverage, and high recognition accuracy and is easy to obtain (Zhang et al., 2021b).
Figure 1 shows our data acquisition and processing method. We divide the target city into different regions to obtain the street locations of Japanese restaurants across different regions. Next, we aggregate these street data into the city data set and establish a database of Japanese restaurants in the city. We use dianping.com and map.baidu.com as our sources for Japanese restaurant data.
We use the key search term “Japanese cuisine” in dianping.com and map.baidu.com; meanwhile, our collection rule is “whether customers choose to eat Japanese cuisine” and the collection objects are from 332 cities in China. The data set was collected from 28 May to 30 May 2022. Then, the data set is checked and rechecked for data loss. Map matching and database establishment are performed via ArcGIS and Google Earth, respectively, (Qin et al., 2019; Tian et al., 2021).
In the process of data acquisition and cleaning, we obtain the text data of Japanese restaurants from dianping.com (including restaurants that provide sushi and other dishes). After eliminating duplicate data and data that did not meet the search requirements, a total of 82,687 restaurant text data were included as part of this study. We found that the obtained restaurant data could be queried through batch comparison with the restaurant location data provided by map.baidu.com. The data used met our subsequent research needs.
2.2 Methods
2.1.1 Field Intensity Model
The equations should be inserted in an editable format from the equation editor. The field intensity model is derived from a physical concept and is mostly used to determine the division of the radiation and development range of urban hinterlands (Wu et al., 2020; Wu et al., 2021). The field intensity model was selected to measure the service capacity of Japanese cuisine in different cities. The higher the field intensity value, the stronger the service capacity, and the wider the service range. City residents can also enjoy better Japanese cuisine and better services. The calculation formula is as follows:
where
2.1.2 Multiple Linear Regression Model
Multiple linear regression models are usually used to study the relationship between a dependent variable and multiple independent variables. The spatial inequality of Japanese cuisine in China is not only affected by economic and social factors in the place of origin but also closely related to the external environment of the consumption location. If x1, x2, … , xn socioeconomic factors are involved in the development of Japanese cuisine service characteristics in China, the regression model is as follows:
2.1.3 Spatial Econometric Model
For model
Spatial econometric models can avoid the deviation of classical econometrics when analyzing spatial effects. The spatial econometric models used in this study are SLM (spatial lag model) and SEM (spatial error model). The spatial lag model is as follows:
where Y is the dependent variable, X is the explanatory variable, W is the spatial weight matrix, Wy is the spatial lag variable, ρ is the spatial regression coefficient, reflecting the degree of diffusion or spillover between adjacent spatial units, β reflects the influence of X on Y, and ε is the random error term.
The spatial lag model mainly verifies the spatial spillover effect of dependent variables in a region and verifies that the influencing factors of dependent variables are applied to other regions through the spatial transmission mechanism. In contrast to the spatial lag model, the spatial error model verifies the spatial dependence existing in the disturbance error term and measures the impact of the error impact of the dependent variables in adjacent areas on the local dependent variables (Guo et al., 2020a). The spatial error model is as follows:
where Y is the dependent variable, X is the explanatory variable, W is the spatial weight matrix, ε is the random error term, λ is the spatial error coefficient, μ is the random error term of the normal distribution, and β reflects the influence of X on Y.
2.3 The Choice of Socioeconomic Factors
In this study, the influencing factors are divided into economic factors, spatial factors, information factors, and humanistic factors (Yang et al., 2019; Guo et al., 2020b; Tian et al., 2021).
Economic factors: For the service industry, the pursuit of profit maximization is the factor preferred by producers for determining production location; that is, maximizing the difference between income and cost. Sufficient market demand is the first consideration in the spatial choice of the catering industry. Cities with large population size and high population density are the first choice for restaurant locations. Under sufficient market demand, the purchasing power level of the region is also an important factor in its location choice. The higher the disposable income of people in the region, the stronger the purchasing power, and the greater the possibility of the location will be. As an important part of the expansion cost of service enterprises, land rent is also one of the necessary conditions for location.
Spatial factors: Traffic accessibility is an important factor affecting the layout of the catering industry (Yang et al., 2018; Zhang et al., 2021a; Zhou et al., 2021). Good traffic accessibility is an important channel to communicate with consumers and retail facilities, and only areas with good accessibility can ensure that consumers can easily reach the retail business center. Simultaneously, smooth logistics distribution can save transportation costs for retail businesses, and good traffic accessibility can ensure the distribution of raw materials (Zhang et al., 2021b).
Information factors: Consumers often consider the place of origin of images and popularity of exotic foods, personal consumption concepts, eating habits, food quality, and impressions of a restaurant’s decor and then reach a purchase intention and choice preferences. Of these criteria, consumer knowledge of exotic food mainly comes through public media such as Japanese variety shows, animation, film, and television (Thorogood, 2020).
Humanistic factors: War can expose people to exotic food and deepen the impression and association of exotic cultures (Wallin and Sandlin, 2020). To understand the influence of the War of Aggression against China on the spread and development of Japanese cuisine in China, the cities affected by the war were assigned a value of 1 and the cities not affected by the war were assigned a value of 0. Moreover, consumers can directly contact the origin of exotic food through travel, which also increases familiarity with specific exotic foods.
The socioeconomic factors screened in this study are shown in Table 1. Considering the integrity of urban, economic, and social data, we used data from the Statistical Yearbook of 2021 for analysis.
3 Results
3.1 Price Characteristics: Middle-Income Group as Service Object
According to economics, consumer demand is the quantity of goods or services that consumers are willing and able to buy at a certain price level. There must be an effective demand with both purchasing desire and purchasing power. The main factors include the price of goods, consumer income levels, consumer preferences, and consumer expectations of the price of the goods. Studies conducted in different environments have indicated that price is one of the main barriers to consumer access to dietary diversity benefits (Herforth and Ahmed, 2015; Vittersø and Tangeland, 2015).
By analyzing the average price of Japanese restaurants, we found that 17% of restaurants have an average price lower than 50¥, 63% have an average price of 50–211¥, and 20% have an average price higher than 211¥. Figure 2 shows that the pricing characteristic of Japanese cuisine is spindle-type, which signals that the main customers of Japanese cuisine are middle-income people, including the middle class and the “new middle class.” Therefore, wealthier areas may have more opportunities to enjoy more diverse and higher quality foods, while small and medium-sized cities and their residents are at a disadvantage in this sense. The eastern cities of China, which are economically dominant, have more potential consumers and greater consumer demand. Therefore, these cities are more likely to have a spatial agglomeration of restaurants and dishes. This finding is in line with general knowledge that big cities often have greater quality of life and wellbeing.
3.2 Spatial Inequality of Geographical Distribution
The distribution of restaurant locations and the field intensity of Japanese restaurants is basically bounded by the Hu line (The Hu line is a line that divides China’s population by density; it was proposed by Chinese geographer Hu Huanyong in 1935. According to the analysis of statistical data in 2000, the National Conditions group at the Chinese Academy of Sciences indicated that the southeast side of the Hu line accounts for 43.18% of China’s total land area, with 93.77% of the population and 95.7% of the GDP, an area with an overwhelmingly high-density economic and social function), but its distribution characteristics are more significant than population density.
In addition, in cities east of the Hu line, the total number of restaurants is 80,212, accounting for 97% of the national total, whereas the total number of restaurants in western cities is 2,475, accounting for only 3% of the national total (Table 2). If NR represents the number of Japanese restaurants, 168 cities have 0 ≤NR <100 restaurants, accounting for 51% of all cities (Table 2), and these cities are mainly distributed in central and western regions; 164 cities with 100 ≤NR <4,963 restaurants, accounting for 49% of all cities, are mainly distributed in eastern coastal areas (Figure 3A).
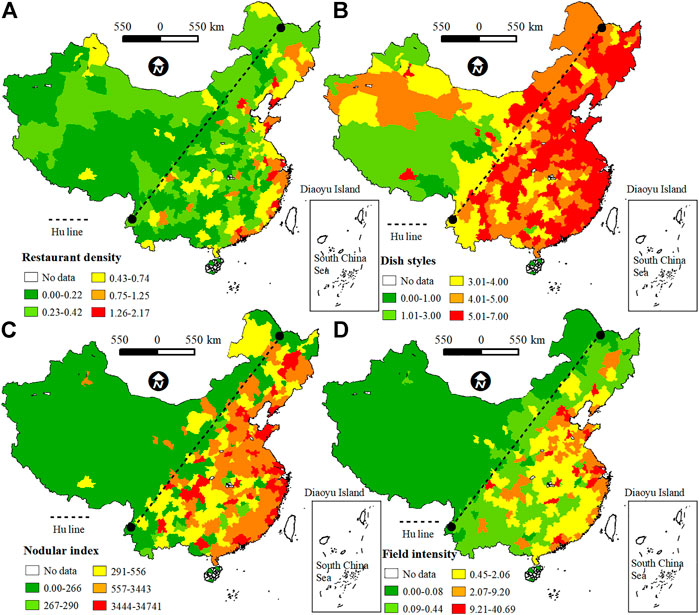
FIGURE 3. Spatial inequality of Japanese cuisine distribution in China. (A) Shows the spatial imbalance of the restaurant density of Japanese cuisine between cities. (B) Shows the spatial imbalance of the dish styles of Japanese cuisine between cities. (C) Shows the spatial imbalance of the nodular index of Japanese cuisine between cities. (D) shows the spatial imbalance of the field intensity of Japanese cuisine between cities.
Figure 3B shows that there are 309 cities with dish styles (DS) between 4 ≤DS ≤7, accounting for 93% of the total number of cities. There are only 23 cities with 0 ≤DS <4 styles, but 82 cities have seven kinds of dishes. In terms of dish styles, there is a significant two-level differentiation in the service characteristics of Japanese cuisine.
Figures 3C,D show the spatial imbalance of the service capacity of Japanese cuisine between cities. In cities east of the Hu line, the field intensity value is basically higher than 0.08, and the service ability is strong; in western cities, the field intensity (FI) value is basically lower than 0.08, and the service ability is weak. Overall, there are 61cities with 0 ≤FI ≤0.08, accounting for 18.4% of all cities, and these are concentrated in central and western regions; there are 271 cities with 0.09 <FI <41, accounting for 81.6% of all cities, and these are mainly distributed in the East.
3.3 The Relationship Between Spatial Imbalance and the Socioeconomic Factors
In the socioeconomic environment, the characteristics or identity of the media often determine the characteristics of catering services. The impact of non-equalization of the external environment, such as social economy, on producers and consumers became the main criteria for screening socio-economic environment factors in this study.
GeoDa is a free and open-source software tool that serves as an introduction to spatial data science; thus, we used GeoDa to solve the model. Before the establishment of the spatial econometric model, the spatial correlation of the sample data was tested. Table 3 shows that Moran’s I are 10.818, 5.768, and 5.989, and their significance levels are all lower than 0.001, indicating that the spatial distribution of restaurant locations, the formation of dish styles, and the consumption intensity of Japanese restaurants are not completely random, but have a certain spatial correlation. Therefore, the OLS regression results may be biased. It is necessary to use the spatial econometric model to for estimation.
From the perspective of variable coefficient and significance (Table 3), the results of the model calculation are consistently high, and the R2 value increases after considering the spatial correlation (SLM = 0.92, 0.72, and 0.89 and SEM = 0.94, 0.72, 0.89, higher than the R2 value of OLS). The SLM and SEM interpretation results are better than the OLS regression results. Combining the LM and R-LM values and their significance and comparing the fitting effects of SLM and SEM, the significance of SLM regression results and test results is better than SEM model results. Thus, this study selects SLM results for subsequent analysis.
Table 3 shows that urbanization level, population size, and economic scale are the key factors that lead to spatial distribution inequality and service imbalance of Japanese cuisine in China. Cities with a large economic scale can often gather more resources. This agglomeration enables economic activities to play a scale effect, which makes the price of products in big cities not only lower but also increases the variety available for selection (Handbury and Weinstein, 2015). Further research shows that in China, large cities have more products and lower prices (Feenstra et al., 2017). Therefore, larger economic-scale cities can gather more consumer demand and market of Japanese cuisine, and the scale of development of Japanese restaurants in these first-tier cities is significantly higher than in other cities.
Population size affects the quantity of food supply and the diversity of dishes. The main reason for this effect is that the size of a population increases the demand for food diversity at the consumption level, which leads to aggregation of various kinds of foods (including exotic foods) and finally results in greater diversity in a more densely populated city, giving the city stronger service ability and higher radiation field intensity. The larger the population, the more Japanese restaurants there are. For example, the top 25 Chinese cities for the number of restaurants accounts for 23% of the total population, but the number of restaurants accounts for 49% of the country (Figure 4). The number of restaurants in cities is basically consistent with the size of the urban population. In addition, the huge population size also entails a complex population structure and an ease in creating a complex heterogeneous demand set in a city, which produces high demand for food types in certain areas. The large coefficient also implies that there may be such a mechanism. The diversity of the population leads to integration of different cultures, making people more willing to try exotic foods (Glaeser et al., 2001).
Simultaneously, the degrees of effect of the elements in model 1 are the following: LNTV> LNTIP> LNUR> LNPOP> LNLR> LNPGDP> LNWAR. The degrees of the functions of the elements in model 2 are the following: LNUR> LNPOP> LNPGDP> LNFL. The degree of the functions of the elements in model 3 are the following: LNTV> LNUR> LNTIP> LNPOP> LNLR> LNPGDP> LNRND.
4 Discussion and Conclusion
4.1 Discussion
Consumer love for variety is a common assumption in modern economics (Glaeser et al., 2001). Due to the heterogeneity of cities and the non-equalization of service facilities, the spatial distribution of food and drink is often unbalanced and complex. Considering the necessity to promote food equality in different socioeconomic areas and the offsetting of the negative impact on the health of vulnerable groups, it is necessary to interpret the spatial equality of dietary distribution (Turner et al., 2018, Turner et al., 2020).
The global commodity network brings a systematic and interconnected consumption landscape. Considering that the socioeconomic environment of consumers is not consistent under different urbanization levels, the place effect should be considered when describing the characteristics of urban catering services; that is, different spatial locations of food, different degrees of realization of the same food, and different benefits. It is more comprehensive and objective to identify the service differences of Japanese cuisine in different regions and spaces with the help of a field intensity model.
This study also encourages and advocates the use of multiple geographic data, the use of data provided by other GeoWeb websites, and other Web2.0 online websites, actively exploring the connection and interaction between geosciences and socio-economic phenomena. In the era of mobile internet, the internet catering service platform has become an important channel for the majority of catering business operators to obtain business data and consumer evaluation information. This would largely change the impact of the competitive rent theory on the location choice of restaurants. These online catering websites can provide more positive and beneficial opportunities for researchers to pay attention to the urban food environment. Simultaneously, it is necessary to explore the application of big data in the field of geographical sustainability. The rise of big data provides new methods, new ideas, and new solutions to pressing urban issues (Xue et al., 2020; Liu et al., 2021; Yang et al., 2021). In contrast to questionnaire and interview approaches, we collected third-party big data provided by an online catering platform to analyze the spatial differences in the number of restaurants and dish styles of exotic food. The multifusion data and big data in this study also provided references for solutions to other urban problems (Shelestov et al., 2017; Haworth et al., 2018; Shirazi et al., 2021; Zhang et al., 2020).
Our results show that there are more Japanese restaurants and dish styles in Eastern China. This conclusion is consistent with the main findings of Tian et al. (2021) on the spatial distribution pattern of Chinese seafood restaurants. They found that seafood restaurants are concentrated in coastal cities. Our results also support the significant correlation between population size, economic development, and the distribution of different types of restaurants. However, this study does not confirm that the geographical distance proposed by Tian et al. (2022) has a significant impact on the distribution of restaurants. We believe that this may be related to consumers’ eating habits and the pricing of Japanese restaurants. In addition to the socioeconomic factors in the socioeconomic environment, food similarity and cultural similarity may also have a potential impact on the spatial inequality of dietary distribution (Figure 5). Rice is a staple of Japanese cuisine and so is very easily accepted by Chinese consumers. In addition, Japanese cuisine consists mainly of seafood, and the acceptability of seafood in China’s coastal regions is very high. It is not difficult to understand that Eastern coastal cities become hot spots and regions where Japanese restaurants are found in higher concentrations. In inland cities, local consumers are not accustomed to eating seafood, and so, the acceptability of this cuisine is relatively low, making these cities cold spots of distribution. This also demonstrates the objectivity of our findings.
A limitation of this study is that the topographic division of urban space, such as by landscape patterns, hinders the spread and diffusion of Japanese cuisine in China, and the research results may contain some errors as a result. In addition, the distance friction coefficient in the field intensity model is usually between 1.0 and 3.0 (the distance attenuation curve varies with the coefficient). The difference in parameter values may lead to deviation from the actual situation, which, in turn, may lead to less accurate calculation.
4.2 Conclusion
By describing the place effect of Japanese cuisine on different spaces in China, we found that the number of restaurants, dish styles, and field intensity shows a gradual decline from eastern cities to western cities. Compared to the Hu line, which reflects the spatial difference in population, the number of restaurants in eastern cities is 32 times that in western cities.
The influx of Japanese cuisine has not alleviated inequality in the urban food environment. The pricing strategies of different grades of dishes show that Japanese cuisine mainly serves the middle class, which means that rich cities often enjoy higher quality food. For cities in a weak position, this undoubtedly damages the wellbeing and the diversity of comfort that food can provide.
Based on the analysis of the local socioeconomic factors, urbanization level, population size, and economic scale are significantly correlated with the maturity of Japanese cuisine. However, the degree of responsiveness of service ability to the socioeconomic environment changes with the location. This also implies that the positioning demand of Japanese cuisine and urban socioeconomic environment is intrinsically related to its price characteristics. The choice of restaurant location and the strength of service ability are the result of the interaction of external socioeconomic environment and internal price characteristics.
We also find that sushi (in 328 cities) and other culturally distinctive dishes have become the most accessible and most likely to be sampled. For certain dishes, the spatial imbalance may not be significant. In the future, it will be necessary to analyze consumer personal values and the relationship between their purchasing lines and specific food choices.
Data Availability Statement
The original contributions presented in the study are included in the article/Supplementary Material; further inquiries can be directed to the corresponding author.
Author Contributions
Methodology, WL; software and validation, CT and HW.; formal analysis, CT; writing—original draft preparation, CT; writing—review and editing, WL; visualization, HW; supervision, HW; funding acquisition, WL. All authors have read and agreed to the published version of the manuscript.
Funding
This research was funded by the Key Program of National Natural Science Foundation of China, Grant Number 42030409.
Conflict of Interest
The authors declare that the research was conducted in the absence of any commercial or financial relationships that could be construed as a potential conflict of interest.
Publisher’s Note
All claims expressed in this article are solely those of the authors and do not necessarily represent those of their affiliated organizations, or those of the publisher, the editors, and the reviewers. Any product that may be evaluated in this article, or claim that may be made by its manufacturer, is not guaranteed or endorsed by the publisher.
References
Adams, E. L., Caccavale, L. J., Smith, D., and Bean, M. K. (2020). Food Insecurity, the Home Food Environment, and Parent Feeding Practices in the Era of COVID‐19. Obesity 28 (11), 2056–2063. doi:10.1002/oby.22996
An, R., and Sturm, R. (2012). School and Residential Neighborhood Food Environment and Diet Among California Youth. Am. J. Prev. Med. 42 (2), 129–135. doi:10.1016/j.amepre.2011.10.012
An, R., He, L., and Shen, M. J. (2020). Impact of Neighbourhood Food Environment on Diet and Obesity in China: a Systematic Review. Public Health Nutr. 23 (3), 457–473. doi:10.1017/S1368980019002167
Anderson, C. E., Crespi, C. M., Wang, M. C., Whaley, S. E., and Chaparro, M. P. (2020). The Neighborhood Food Environment Modifies the Effect of the 2009 WIC Food Package Change on Childhood Obesity in Los Angeles County, California. BMC Public Health 20 (1), 678. doi:10.1186/s12889-020-08779-2
Armington, P. S. (1969). A Theory of Demand for Products Distinguished by Place of Production. Staff Pap. - Int. Monet. Fund. 16, 159–176. doi:10.2307/3866403
Black, C., Moon, G., and Baird, J. (2014). Dietary Inequalities: what Is the Evidence for the Effect of the Neighbourhood Food Environment? Health Place 27, 229–242. doi:10.1016/j.healthplace.2013.09.015
Çanakçı, S. D., and Birdir, K. (2020). The Relation Among Food Involvement, Food Variety Seeking and Food Neophobia: A Study on Foreign Tourists Visiting Turkey. Curr. Issues Tour. 23 (8), 917–928. doi:10.1080/13683500.2019.1602114
Caspi, C. E., Sorensen, G., Subramanian, S. V., and Kawachi, I. (2012). The Local Food Environment and Diet: a Systematic Review. Health Place 18 (5), 1172–1187. doi:10.1016/j.healthplace.2012.05.006
Chen, X., and Yang, X. (2014). Does Food Environment Influence Food Choices? A Geographical Analysis through “Tweets”. Appl. Geogr. 51, 82–89. doi:10.1016/j.apgeog.2014.04.003
Clark, B., Stewart, G. B., Panzone, L. A., Kyriazakis, I., and Frewer, L. J. (2017). Citizens, Consumers and Farm Animal Welfare: a Meta-Analysis of Willingness-To-Pay Studies. Food Policy 68, 112–127. doi:10.1016/j.foodpol.2017.01.006
Cobb, L. K., Appel, L. J., Franco, M., Jones-Smith, J. C., Nur, A., and Anderson, C. A. M. (2015). The Relationship of the Local Food Environment with Obesity: a Systematic Review of Methods, Study Quality, and Results. Obesity 23 (7), 1331–1344. doi:10.1002/oby.21118
Eckert, J., and Vojnovic, I. (2017). Fast Food Landscapes: Exploring Restaurant Choice and Travel Behavior for Residents Living in Lower Eastside Detroit Neighborhoods. Appl. Geogr. 89, 41–51. doi:10.1016/j.apgeog.2017.09.011
FAO-Food and Agriculture Organization of the United Nations (2016). Influencing Food Environments for Healthy Diets through Food Safety. Rome: FAO.
Feenstra, R. C., Xu, M., and Antoniades, A. (2017). What Is the Price of Tea in China? towards the Relative Cost of Living in Chinese and U.S. Cities. SSRN J. (No. w23161). National Bureau of Economic Research. doi:10.2139/ssrn.2925530
Garcia, X., Garcia-Sierra, M., and Domene, E. (2020). Spatial Inequality and its Relationship with Local Food Environments: The Case of Barcelona. Appl. Geogr. 115, 102140. doi:10.1016/j.apgeog.2019.102140
Glaeser, E. L., Kolko, J., and Saiz, A. (2001). Consumer City. J. Econ. Geogr. 1 (1), 27–50. doi:10.1093/jeg/1.1.27
Guo, A., Yang, J., Sun, W., Xiao, X., Xia Cecilia, J., Jin, C., et al. (2020a). Impact of Urban Morphology and Landscape Characteristics on Spatiotemporal Heterogeneity of Land Surface Temperature. Sustain. Cities Soc. 63, 102443. doi:10.1016/j.scs.2020.102443
Guo, A., Yang, J., Xiao, X., Xia, J., Jin, C., and Li, X. (2020b). Influences of Urban Spatial Form on Urban Heat Island Effects at the Community Level in China. Sustain. Cities Soc. 53, 101972. doi:10.1016/j.scs.2019.101972
Handbury, J., and Weinstein, D. E. (2015). Goods Prices and Availability in Cities. Rev. Econ. Stud. 82 (1), 258–296. doi:10.1093/restud/rdu033
Haworth, B. T., Bruce, E., Whittaker, J., and Read, R. (2018). The Good, the Bad, and the Uncertain: Contributions of Volunteered Geographic Information to Community Disaster Resilience. Front. Earth Sci. 6, 183. doi:10.3389/feart.2018.00183
Herforth, A., and Ahmed, S. (2015). The Food Environment, its Effects on Dietary Consumption, and Potential for Measurement within Agriculture-Nutrition Interventions. Food Sec. 7 (3), 505–520. doi:10.1007/s12571-015-0455-8
Jang, M., Brown, R., and Vang, P. Y. (2020). The Relationships between Parental Stress, Home Food Environment, and Child Diet Patterns in Families of Preschool Children. Am. J. Health Promot 35, 131–139. doi:10.1177/0890117120929541
Kwate, N. O. A., and Loh, J. M. (2016). Fast Food and Liquor Store Density, Co-tenancy, and Turnover: Vice Store Operations in Chicago, 1995-2008. Appl. Geogr. 67, 1–13. doi:10.1016/j.apgeog.2015.11.021
Leslie, T. F., Frankenfeld, C. L., and Makara, M. A. (2012). The Spatial Food Environment of the DC Metropolitan Area: Clustering, Co-location, and Categorical Differentiation. Appl. Geogr. 35, 300–307. doi:10.1016/j.apgeog.2012.07.008
Li, J., and Kim, C. (2020). Exploring Relationships of Grocery Shopping Patterns and Healthy Food Accessibility in Residential Neighborhoods and Activity Space. Appl. Geogr. 116, 102169. doi:10.1016/j.apgeog.2020.102169
Liu, X., Fatoyinbo, T. E., Thomas, N. M., Guan, W. W., Zhan, Y., Mondal, P., et al. (2021). Large-Scale High-Resolution Coastal Mangrove Forests Mapping across West Africa with Machine Learning Ensemble and Satellite Big Data. Front. Earth Sci. 8. doi:10.3389/feart.2020.560933
Luciene, F. F. A., Taiane, G. N., Pessoa, M. C., Carmo, A. S. D., and Andréia, Q. R. (2020). Socioeconomic Disparities in the Community Food Environment of a Medium-Sized City of Brazil. J. Am. Coll. Nutr. 40 (2), 253–260. doi:10.1080/07315724.2020.1755911
Lytle, L. A., and Sokol, R. L. (2017). Measures of the Food Environment: A Systematic Review of the Field, 2007-2015. Health Place 44, 18–34. doi:10.1016/j.healthplace.2016.12.007
Osei-Kwasi, H., Mohindra, A., Booth, A., Laar, A., Wanjohi, M., Graham, F., et al. (2020). Factors Influencing Dietary Behaviours in Urban Food Environments in Africa: a Systematic Mapping Review. Public Health Nutr. 23, 2584–2601. doi:10.1017/S1368980019005305
Qin, X., Zhen, F., and Gong, Y. (2019). Combination of Big and Small Data: Empirical Study on the Distribution and Factors of Catering Space Popularity in Nanjing, China. J. Urban Plann. Dev. 145 (1), 05018022. doi:10.1061/(ASCE)UP.1943-5444.0000489
Rui, Y., Huang, H., Lu, M., Wang, B., and Wang, J. (2016). A Comparative Analysis of the Distributions of KFC and McDonald’s Outlets in China. ISPRS Int. J. Geo-Inf. 5 (3), 27. doi:10.3390/ijgi5030027
Schiff, N. (2015). Cities and Product Variety: Evidence from Restaurants. J. Econ. Geogr. 15 (6), 1085–1123. doi:10.1093/jeg/lbu040
Shelestov, A., Lavreniuk, M., Kussul, N., Novikov, A., and Skakun, S. (2017). Exploring Google Earth Engine Platform for Big Data Processing: Classification of Multi-Temporal Satellite Imagery for Crop Mapping. Front. Earth Sci. 5, 17. doi:10.3389/feart.2017.00017
Shen, Q., and Xiao, P. (2014). McDonald's and KFC in China: Competitors or Companions? Mark. Sci. 33 (2), 287–307. doi:10.1287/mksc.2013.0824
Shirazi, Z., Wang, L., and Bondur, V. G. (2021). Modeling Conditions Appropriate for Wildfire in South East China-A Machine Learning Approach. Front. Earth Sci. 9, 361. doi:10.3389/feart.2021.622307
Swinburn, B., Kraak, V., Rutter, H., Vandevijvere, S., Lobstein, T., Sacks, G., et al. (2015). Strengthening of Accountability Systems to Create Healthy Food Environments and Reduce Global Obesity. Lancet 385 (9986), 2534–2545. doi:10.1016/s0140-6736(14)61747-5
Thorogood, J. (2020). Cartoon Controversies and Geopolitics: Archer, Animators and Audiences. Soc. Cult. Geogr. 21 (3), 357–379. doi:10.1080/14649365.2018.1497190
Tian, C., Luan, W., Li, S., Xue, Y., and Jin, X. (2021). Spatial Imbalance of Chinese Seafood Restaurants and its Relationship with Socioeconomic Factors. Ocean Coast. Manag. 211, 105764. doi:10.1016/j.ocecoaman.2021.105764
Tian, C., Luan, W., You, D., Su, M., and Jin, X. (2022). Seafood Availability and Geographical Distance: Evidence from Chinese Seafood Restaurants. Ocean Coast. Manag. 225, 106219. doi:10.1016/j.ocecoaman.2022.106219
Turner, C., Aggarwal, A., Walls, H., Herforth, A., Drewnowski, A., Coates, J., et al. (2018). Concepts and Critical Perspectives for Food Environment Research: A Global Framework with Implications for Action in Low-And Middle-Income Countries. Glob. food Secur. 18, 93–101. doi:10.1016/j.gfs.2018.08.003
Turner, C., Kalamatianou, S., Drewnowski, A., Kulkarni, B., Kinra, S., and Kadiyala, S. (2020). Food Environment Research in Low-And Middle-Income Countries: a Systematic Scoping Review. Adv. Nutr. 11 (2), 387–397. doi:10.1093/advances/nmz031
Vittersø, G., and Tangeland, T. (2015). The Role of Consumers in Transitions towards Sustainable Food Consumption. The Case of Organic Food in Norway. J. Clean. Prod. 92, 91–99. doi:10.1016/j.jclepro.2014.12.055
Wallin, J. J., and Sandlin, J. A. (2020). Plastic Fantasies: Globalization and the Japanese Cultural Imaginary. Cult. Stud. ↔ Crit. Methodol. 20 (6), 524–534. doi:10.1177/1532708620911407
Willmott, H. (2007). “Political Cultural Economy and the Financialization of Brand Equity,” in ESRC Centre for Research on Socio-Cultural Change 3rd Annual Conference: Re-thinking Cultural Economy, Manchester, United Kingdom, September 5–7, 2007.
Wu, J., Feng, Z., Zhang, X., Xu, Y., and Peng, J. (2020). Delineating Urban Hinterland Boundaries in the Pearl River Delta: An Approach Integrating Toponym Co-occurrence with Field Strength Model. Cities 96, 102457. doi:10.1016/j.cities.2019.102457
Wu, M., Pei, T., Wang, W., Guo, S., Song, C., Chen, J., et al. (2021). Roles of Locational Factors in the Rise and Fall of Restaurants: A Case Study of Beijing with POI Data. Cities 113, 103185. doi:10.1016/j.cities.2021.103185
Xue, B., Xiao, X., and Li, J. (2020). Identification Method and Empirical Study of Urban Industrial Spatial Relationship Based on POI Big Data: a Case of Shenyang City, China. Geogr. Sustain. 1 (2), 152–162. doi:10.1016/j.geosus.2020.06.003
Yang, J., Guo, A., Li, X., and Huang, T. (2018). Study of the Impact of a High-Speed Railway Opening on China’s Accessibility Pattern and Spatial Equality. Sustainability 10 (8), 2943. doi:10.3390/su10082943
Yang, J., Guo, A., Li, Y., Zhang, Y., and Li, X. (2019). Simulation of Landscape Spatial Layout Evolution in Rural-Urban Fringe Areas: a Case Study of Ganjingzi District. GIScience remote Sens. 56 (3), 388–405. doi:10.1080/15481603.2018.1533680
Yang, J., Ren, J., Sun, D., Xiao, X., Xia, J., Jin, C., et al. (2021). Understanding Land Surface Temperature Impact Factors Based on Local Climate Zones. Sustain. Cities Soc. 69, 102818. doi:10.1016/j.scs.2021.102818
Zeng, G. J., and Liu, M. (2013). Diet Geography and Translocal Restaurants' Cultural Production. Tour. Trib. 3, 9–11. doi:10.13284/j.cnki.rddl.002816
Zhang, D., Zhou, C., and Xu, W. (2020). Spatial-temporal Characteristics of Primary and Secondary Educational Resources for Relocated Children of Migrant Workers: The Case of Liaoning Province. Complexity 2020, 1–13. doi:10.1155/2020/7457109
Zhang, Z., Luan, W., Tian, C., Su, M., and Li, Z. (2021). Spatial Distribution Equilibrium and Relationship between Construction Land Expansion and Basic Education Schools in Shanghai Based on POI Data. Land 10 (10), 1059. doi:10.3390/land10101059
Zhang, D., Zhang, G., and Zhou, C. (2021a). Differences in Accessibility of Public Health Facilities in Hierarchical Municipalities and the Spatial Pattern Characteristics of Their Services in Doumen District, China. Land 10 (11), 1249. doi:10.3390/land10111249
Zhang, D., Zhou, C., Sun, D., and Qian, Y. (2021b). The Influence of the Spatial Pattern of Urban Road Networks on the Quality of Business Environments: the Case of Dalian City. Environ. Dev. Sustain., 1–18. doi:10.1007/s10668-021-01832-z
Keywords: exotic food, spatial differentiation, local socioeconomic factors, spatial econometric model, POI (point of interest) data
Citation: Tian C, Luan W and Wang H (2022) Exotic Food, Food Environment, and Geographical Patterns: Big Data Analytics From Japanese Cuisine in China. Front. Earth Sci. 10:944927. doi: 10.3389/feart.2022.944927
Received: 16 May 2022; Accepted: 07 June 2022;
Published: 08 July 2022.
Edited by:
Jianhong Xia, Curtin University, AustraliaReviewed by:
Dahao Zhang, Sun Yat-sen University, ChinaChenxi Li, Xi’an University of Architecture and Technology, China
Ling-en Wang, Institute of Geographic Sciences and Natural Resources Research (CAS), China
Copyright © 2022 Tian, Luan and Wang. This is an open-access article distributed under the terms of the Creative Commons Attribution License (CC BY). The use, distribution or reproduction in other forums is permitted, provided the original author(s) and the copyright owner(s) are credited and that the original publication in this journal is cited, in accordance with accepted academic practice. No use, distribution or reproduction is permitted which does not comply with these terms.
*Correspondence: Weixin Luan, Z3VhbmxsMjAxOUB2aXAuMTYzLmNvbQ==