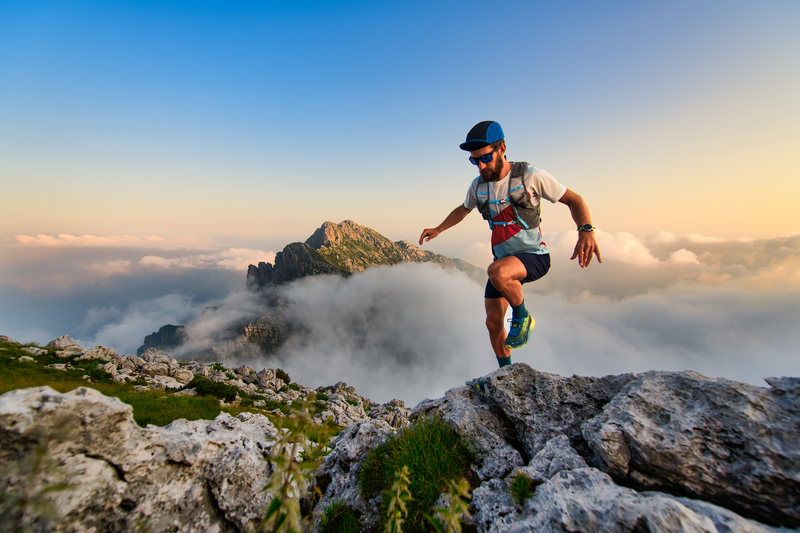
95% of researchers rate our articles as excellent or good
Learn more about the work of our research integrity team to safeguard the quality of each article we publish.
Find out more
ORIGINAL RESEARCH article
Front. Earth Sci. , 24 June 2022
Sec. Geohazards and Georisks
Volume 10 - 2022 | https://doi.org/10.3389/feart.2022.931505
This article is part of the Research Topic Monitoring, Early Warning and Mitigation of Natural and Engineered Slopes View all 15 articles
The Qinghai–Tibet Plateau is a region sensitive to climate change with significant changes in topography, and the increase in extreme precipitation in the region easily leads to landslides and debris flows. To mitigate the effects of climate change, the Chinese government has pledged to an emission reduction target that achieves a “carbon peak’” in 2030 and being “carbon neutral” in 2060 at a huge cost. In order to explore the quantitative flood risk at the county level in Qinghai province (the core province of the Qinghai–Tibet Plateau) and the contribution of emission reduction efforts to risk mitigation, this article constructs a loss rate curve based on the data of more than 1,000 single flood disasters from 2009 to 2019 through government cooperation and the data of observed cumulative precipitation. Combined with the latest CMIP6 climate model data, the temporal and spatial variation characteristics of the flood loss ratio in Qinghai province from 2020 to 2060 are quantitatively characterized. The results show the following: 1) the curve of the flood loss rate in Qinghai province from 2020 to 2060 may present a trend of “rising in the early stage, stable in the middle stage, and declining in the late stage” under low (SSP126) and medium (SSP245) emission scenarios and presents a trend of rising fluctuation under the high-emission (SSP585) scenario. The flood loss ratio under SSP585 is 10 times higher than that under SSP126; 2) the flood risk of 44 counties from 2020 to 2060 in Qinghai province may be concentrated in the southeast region, with Jiuzhi county, Banma county, Nangqian county, Gande county, and Dari county being the most prominent; (3) compared with SSP585, the Qinghai province in SSP126 may cumulatively avoid about 24 billion CNY in economic losses from 2020 to 2060 and avoid nearly 600 million CNY in losses per year, which is equivalent to 93% of Qinghai province’s special funds for ecological and environmental protection in 2019. The research aims to provide theoretical and data support for flood disaster risk prevention and management in China’s high-altitude areas and to promote the initiative of emission reduction in China and even the world.
The regional extreme precipitation intensity and global warming degree represent an approximate linear relationship (high confidence) (IPCC, 2021). According to the CMIP5 scenarios, the relevant meteorological indices of the Qinghai–Tibet Plateau have a greater range of variation than the global meteorological indices, and its response to climate change is more obvious (Supplementary Table S1) (Wu and You, 2019). Also, the Tibetan Plateau in China is a region sensitive to climate change due to its unique and complex high-altitude geographical conditions (Duan al., 2016). The risk of regional extreme precipitation may be further amplified in this region (Ma et al., 2021) and more vulnerable to induce mass movement disasters such as landslides and debris flow (Ma et al., 2021; Liu et al., 2022; Yang et al., 2022). This change will threaten the lives and property of people on the Qinghai–Tibet Plateau and even downstream regions (Zhou et al., 2022).
In order to further reduce the impact of climate change, the Chinese government has set the carbon emission reduction target of “carbon peak by 2030 and carbon neutral by 2060” and formulated strong emission reduction measures. Due to the Tibetan Plateau’s high sensitivity to climate change response and the positive relationship between regional extreme precipitation and climate change, the assessment of future flood risk changes in the region can reflect the effectiveness of emission reduction in China and even the world to a certain extent. The current risk assessment of extreme flood has experienced a process from qualitative methods to semi-qualitative and semi-quantitative methods and then to quantitative methods. The qualitative methods mainly used questionnaire survey, data analysis, and other methods combined with expert experience to determine the relative risk degree (Meihua et al., 2008; Zeleňáková, 2009; Wang et al., 2018). With the maturity of the disaster risk theory, the research on disaster risk assessment mostly adopts the method of multi-factor weighted syntheses of categories like hazards, exposure, and fragility (Guo et al., 2021; Lv et al., 2021). However, as all relevant indicators need to be uniform in dimension, the result is a “relative” risk level of “low/medium/high.” In order to meet the evaluation needs of the “absolute” degree of disaster risk, the loss function or expected loss function based on disaster statistics and the intensity of hazard to calculate the loss rate curve is built to evaluate the results measured by the monetary value of economic losses or the number of affected populations (Delalay et al., 2020; Su et al., 2021), but this method also puts high requirements for the reliability, timing, and comprehensiveness of basic data (Li et al., 2016).
Qinghai province is the core province of the Qinghai–Tibet Plateau in China, and it is urgent to carry out a risk assessment for flood disasters. Due to the relatively low social and economic development of Qinghai province and the backward construction of disaster management departments, there is a lack of sufficient public historical disaster data in this area. Therefore, the qualitative and semi-quantitative methods are mostly used for disaster risk assessment (Jinhu et al., 2007; Dou and Yan, 2013; Zou et al., 2013; Chen et al., 2021; Weidong et al., 2021). However, with the government’s increasing demand for the assessment of the “absolute” degree of flood risk in Qinghai province and also with the exploration of the contribution of emission reduction efforts to the risk reduction degree, the quantitative method for the Qinghai flood disaster becomes an urgent work. Some research studies use information diffusion methods such as the Monte Carlo method to supplement missing historical disaster data, but this brings large uncertainty compared to actual information (Kalyanapu et al., 2012; Peng et al., 2017; Koc and Işık, 2021).
In this article, the disaster data composed of more than 1,000 single flood disasters in the flood season (May–October) in Qinghai province from 2009 to 2019 were obtained by cooperating with the disaster management departments of Qinghai province, and the accumulated precipitation during a flood disaster process was obtained by combining the observed daily precipitation data. Based on the aforementioned data, the expected loss function is constructed by the regression prediction model and probability density analysis method. In addition, daily precipitation grid data of 22 climate models (CMIP6) and future GDP grid data (SSP database) were used to evaluate the flood disaster loss rate curves of 44 counties in Qinghai province from 2020 to 2060 under three scenarios. This study takes the low-emission scenario SSP126 as the “carbon neutral” target scenario, medium-emission scenario SSP245, and high-emission scenario SSP585, which were used as comparative reference scenarios to evaluate the temporal and spatial variation characteristics of flood risk at provincial and county scales in Qinghai province from 2020 to 2060 (O’Neill et al., 2016; Ridder et al., 2022). The purpose was to analyze the contribution of emission reduction to reducing flood disaster risk in Qinghai province and identify the potential “positive impact” brought by China’s emission reduction efforts, providing data and method support for formulating sustainable disaster prevention and mitigation policies and social-economic development policies in Qinghai province, and also promoting China and the global initiative to reduce emissions.
Qinghai, a province in western China located in the northeast of the Qinghai–Tibet Plateau, is 1200 km long from east to west and more than 800 km wide from north to south, accounting for 1/13th of China’s total area. The average altitude of the province is over 3000 m above sea level. Its topography shows downward elevation from west to east and a saddle shape from north to south (Figure 1A). The annual precipitation in Qinghai province is 15.4–732.5 mm with a general distribution landscape of gradual decrease from southeast to northwest. In most areas of the province, the annual precipitation is below 400 mm and features sharp seasonal fluctuations that the precipitation in the flood season accounts for more than 70% of its annual total. The topography makes it easy for heavy rain to develop into flash floods, and even geological disasters, such as debris flow and landslides.
FIGURE 1. Distribution landscape of natural and economic characteristics of Qinghai province. (A) Geographical location of Qinghai province and the Qinghai–Tibet Plateau, and the terrain of Qinghai province; (B) average precipitation distribution in Qinghai province from 2009 to 2019; (C) GDP distribution of Qinghai province in 2019.
The precipitation distribution in Qinghai province from 2009 to 2019 shows that the east is rainier than the west, while the south is rainier than the north (Figure 1B). Jiuzhi county in the southeast of Qinghai province has the highest annual average precipitation in the province. The precipitation of counties in Qinghai province is mainly in the flood season (June to September), accounting for more than 65% of the annual precipitation. The spatial and temporal distribution of landslide and debris flow disasters coincides with that of rainstorm and flood disasters caused by precipitation in the eastern part of the Qinghai–Tibet Plateau (Lin et al., 2020). As the risks of flood hazards are high in the east and low in the west, those in Chengduo county, Nangqian county, Zeku county, and Xunhua county possess high-risk curves. According to the GDP distribution in 2019 (Figure 1C), except Golmud county, we can see that the economically developed areas in Qinghai are mainly in Xining city and its surrounding regions. The high direct losses (DEL) all happened in the northeast of Qinghai province, and Xining city and its surrounding areas are the most severely affected, showing an ascending trend from southwest to northeast. A total of 11 counties in Qinghai province suffered direct economic losses of over 100 million CNY each from 2009 to 2019, with Xunhua county suffering the highest DEL of 593 million CNY.
From 2009 to 2019, Qinghai province counted 1,512 county-level flood and secondary disasters, such as landslides and debris flow data, including the direct economic losses, affected population, dead population, and damaged farmland. The meteorological data include daily precipitation and daily mean temperature data of 41 meteorological stations in Qinghai province from 2009 to 2019. The social statistical data are the annual GDP data of 44 counties in Qinghai province from 2009 to 2019. The specific information is shown in Table 1:
Future flood disaster risk assessment uses CMIP6 (https://esgf-node.llnl.gov/search/cmip6/) future climate scenarios data from 2020 to 2060, including daily scale precipitation data of 22 GCM models (Supplementary Table S2) under different scenarios with different spatial resolutions. Future emission scenarios include CMIP6’s latest SSPs (Shared Socioeconomic Pathways and the Representative Concentration Pathways): SSP126, SSP245, and SSP585. The SSPs are the updated RCP (Representative Concentration Pathway) scenarios SSP126, SSP245, and SSP585, representing the low social vulnerability and low-emission scenario, medium social vulnerability and medium-emission scenario, and high social vulnerability and high-emission scenario, respectively. The future economic forecast data adopt the future GDP grid data of three emission scenarios under the SSP database during the period 2020–2060. Detailed information is listed in Table 2:
This article mainly includes three parts: 1) construction of an expected loss model based on historical data; 2) future climate model data extraction; 3) future risk assessment of flood disaster in Qinghai province.
In this study, the single-process precipitation of 377 flood disasters in Qinghai province from 2009 to 2019 was fitted with the corresponding loss rate of flood by polynomial fitting, and the expected loss curve of rainstorm flood disasters in Qinghai province is determined as follows:
where x is the function fitting mode, and this formula selects the best fitting function through linear fit and nonlinear curve fit (e.g., convolution, exponential, growth/sigmoidal, logarithm, polynomial, power, and rational); i is the ith county; j is the jth flood precipitation process; d is the accumulated days of flood disaster; Accprhis is the single rainstorm flood disaster-accumulated precipitation; α = −0.08092 [−0.17124, 0.0094]; β = -0.12987 [−0.15164, −0.1081]; γ = 5.38928 [2.13541, 8.64315]; and QRloss is the loss rate of flood disaster:
where DLyear is the direct economic loss of a single flood disaster, GDPyear is the current GDP (Gross domestic product), and Deƒiyear=2015 is the GDP deflator set 2015 = 100.
Figure 2 shows the trend that the loss rate of flood disaster changes with the change of precipitation during the disaster process. It can be seen from the expected loss curve in Figure 2 that with the increase of the process precipitation, the loss rate increases rapidly in the range of 0–20 mm process precipitation and increases more slowly after 20 mm process precipitation, showing a “logarithmic” shape as a whole.
The precipitation data of the future CMIP climate model were interpolated by quadratic spline interpolation with a uniform accuracy of 0.0083° × 0.0083° (1 km). The formula for future precipitation data and GDP data is as follows:
where g is the number of grid points in a region, GDPfuture is the projected future GDP, Accprfuture is the projected future process precipitation.
DLifuture (direct economic losses of future flood disasters) can be obtained by Eqs. 2, 3, 4:
The ratio of direct economic loss (P) is calculated as the “absolute” value of the future flood disaster risk assessment in Qinghai province through the formula in Eq (6):
The quantiles of 90%, 98%, and 99% of precipitation in the historical flood disaster process are selected as the threshold values of flood disasters that occur once in 10 years, once in 50 years, and once in 100 years, respectively, and the threshold values are used to evaluate the risk of extreme flood disasters in the future. Taking the 10-year flood risk as an example:
Take 5% and 95% of the multi-model data set under each scenario as the error range of precipitation for future flood disasters. Under the high-emission scenario (SSP585), the GDP loss ratio in 2055 is 0.7%, and the uncertainty caused by the multi-model 95% and 5% data is in the range of (0.00003%, 70%), that is, it causes the loss of 29,400 CNY to 205.8 billion CNY. The reason for such a large error is that the multi-mode data of CMIP6 vary greatly in the high-altitude area of the Qinghai–Tibet Plateau. Therefore, only the mean value data of 22 models are used to calculate the future flood disaster loss ratio.
Figure 2 shows the changing trend of the loss rate of flood in the context of precipitation fluctuations during the disaster. As can be seen from the expected loss curve in the figure, with the increase of precipitation, the loss rate rises rapidly in the precipitation range of 0–20 mm and then more and more slowly after 20 mm, presenting a “logarithmic” change trend as a whole.
Due to the large vertical elevation difference in Qinghai province, small precipitation can quickly give rise to runoffs and confluences to form flash floods, but more precipitation is needed in plain areas to cause regional floods. Combined with the loss rate of Y-axis, “small and frequent” flood disasters are more likely to occur in Qinghai province, but the losses caused by the accumulation of those disasters cannot be ignored. According to the distribution of precipitation and loss rate of the flood, the disaster with the highest loss rate in history is not the one with the heaviest precipitation. Due to the underdeveloped economy in some areas in Qinghai province, the number of hazard-affected bodies is small. With the continuous growth of precipitation, most of those bodies exposed to the affected area of the disaster are damaged, so the losses reach the peak value. In addition, for relatively developed areas with insufficient disaster protection capacity, rainstorms and floods often lead to landslides, debris flows, and other geological disasters, resulting in a high loss rate with low precipitation.
1) Annual trend analysis of the loss ratio in Qinghai province from 2020 to 2060
Figure 3 shows the annual change curve of the loss ratio of flood in Qinghai province from 2020 to 2060 under the scenarios SSP126, SSP245, and SSP585, calculated based on the historically expected loss curve, the future precipitation, and the forecast data of GDP. The shaded part is the error range caused by the 95% confidence interval of the expected loss curve. On the whole, the scenarios SSP126 and SSP245 show a trend of “rising in the early stage, stable in the middle stage, and declining in the late stage,” while scenario SSP585 shows a rising trend of fluctuation, which is becoming wilder. From 2050 to 2060, the fluctuation degree will reach its peak. The average annual loss ratios under the three scenarios are 0.231%, 0.249%, and 0.429%, respectively. The higher the emission force is, the higher the overall loss rate will be.
FIGURE 3. Annual loss ratio curves under three scenarios in Qinghai province from 2020 to 2060. (A) Loss ratio curves under SSP126 scenario;. (B) loss ratio curves under the SSP245 scenario; and (C) loss ratio curves under the SSP585 scenario.
In scenario SSP126, the year with the highest loss ratio will be around 2040 and the highest loss ratio (0.499%) will be found in 2037 while the lowest loss ratio (0.075%) in 2058. In the figure, the most obvious increase and decrease changes will be found from 2037 to 2038 and from 2055 to 2058, with the loss ratio increasing by 0.3560% and decreasing by 0.290%, respectively. From 2020 to 2060, the total loss ratio will reach 9.4808%.
In scenario SSP245, the highest loss ratio (0.522%) will be found in 2048, while the lowest loss ratio (0.121%) in 2020. From 2047 to 2048, the most obvious loss ratio increase will be 0.3688%, while from 2048 to 2049, the most obvious loss ratio decrease will be 0.2193%. The total loss ratio through the period from 2020 to 2060 is expected to reach 10.1938%.
In scenario SSP585, the loss ratio will reach a peak (0.8063%) in 2058, while the lowest loss ratio (0.125%) was seen in 2020. The most obvious increase and decrease changes will be found during the periods 2055–2058 and 2058–2060, with the loss ratio increasing by 0.364% and decreasing by 0.371%, respectively. The cumulative loss ratio from 2020 to 2060 will be 17.6011%. With the time going on, the maximum loss ratio in the SSP585 scenario will be constantly refreshed, and its fluctuation range will become larger and larger.
Based on the aforementioned variation trends of the loss ratio and precipitation in the three scenarios, the lowest value (0.075%) of the flood risk loss ratio in Qinghai province is found in scenario SSP126 and the highest value (0.8063%) in scenario SSP585. The increasing trend and fluctuation range of the loss ratio of SSP585 are significantly larger than those of SSP126 and SSP245.
2) Comparative analysis of the difference in losses under three scenarios in Qinghai province from 2020 to 2060
Based on the assessment results of flood disaster loss ratios through the period of 2020–2060 under the scenario SSP126/245/585 in Qinghai province, Figure 4 further analyzes the disparity of future flood loss ratios among the three scenarios.
FIGURE 4. Comparison chart of loss ratios under three scenarios in Qinghai province from 2020 to 2060. (A) Change range of the flood disaster loss ratio under SSP245 scenario with medium carbon emission compared with SSP126 scenario with low-carbon emission; (B) change range of the flood disaster loss ratio of SSP585 scenario with high-carbon emission compared with SSP245 scenario with medium carbon emission; (C) change range of the flood disaster loss ratio under SSP585 scenario with high-carbon emissions compared with SSP126 scenario with low-carbon emission.
The ratio of actual loss to GDP in 2019 under the future scenarios SSP126/245/585 shows significant differences in maximum value, minimum value, and inter-annual fluctuation. The difference between SSP585 and SSP126 is greater than that between SSP585 and SSP245, and that between SSP245 and SSP126 is the smallest.
Compared with that under the scenario SSP126 with low emission, the change extent of annual flood disaster loss under scenario SSP245 with medium emission (Figure 4A) shows that the loss ratio under SSP245 during 2020–2060 is 0.017% high. In most of the years, the loss of SSP245 is higher than that of SSP126, and the widest discrepancy between them will be 186.745% in 2024. In the next 41 years, the cumulative loss ratio of SSP245 will be 0.713% higher than that of SSP126. Given the GDP, 294 billion CNY, of Qinghai province in 2019, the DEL of flooding in the province will increase by about 2.1 billion CNY. Figure 4B shows that under SSP585, the annual average loss ratio is 0.1807% higher than that of SSP245, and the loss caused by flood will increase by 436.566% to the peak (2057), with an average annual increase of 97.777%. From 2020 to 2060, the difference of cumulative disaster loss ratios will be 7.4073%. Given the GDP of Qinghai province in 2019, the loss of flood in the province under the SSP585 scenario will increase by about 21.777 billion CNY compared with that under the SSP245 scenario.
From 2020 to 2060, the annual average loss ratio under the SSP585 scenario is 0.198% higher than that under scenario SSP126. In 41 years, the loss ratio of flood disasters under the SSP585 scenario was less than that under the SSP126 scenario only by 4 years. At the same time, the difference in the GDP loss ratio between the two scenarios varies greatly from year to year, and the loss of scenario SSP585 is 126% higher than that of scenario SSP126 a year on average. The loss caused by the flood disaster will increase by nearly 10 times than that in 2058 (980.733%). This is mainly because under scenario SSP585, the loss ratio of flood will fluctuate greatly during 2050–2060, and its extreme value will also be seen in 2058.
The aforementioned results show that from 2020 to 2060, compared with scenario SSP585, the loss ratio of flood in Qinghai province will decrease by 8.12% under scenario SSP126, which is to achieve carbon emission reduction targets. With the emission reduction action of China’s “carbon neutrality and carbon peak” target, only the loss caused by the flood in Qinghai can drop by 23.874 billion CNY (based on the GDP of Qinghai province of 294 billion CNY in 2019) from 2020 to 2060, about saving 600 million CNY per year on average, equivalent to 93% of Qinghai’s 2019 provincial special fund for ecological and environmental protection (626 million CNY). From the perspective of disaster risk, the results can quantitatively reveal the hidden “benefits” behind the costs for China to achieve the “carbon neutrality and carbon peak” target.
3) Analysis of the frequency of flood return period in Qinghai province from 2020 to 2060
During 2020–2060 in Qinghai province, the occurrences of extreme floods within a 10-, 50-, and 100-year return period (Figure 5) show that the frequency of those extreme disasters occurring within the 10-year return period is greater than that occurring within the 50-year return period and 100-year return period under scenario SSP126/245/585, and all of them show a fluctuant-rising trend. In particular, around 2058, there has been a significant increasing trend.
FIGURE 5. Extreme flood frequency in Qinghai province from 2020 to 2060. (A) 10/50/100-year return period flood frequency under the SSP126 scenario; (B) 10/50/100-year return period flood frequency under the SSP245 scenario; and (C) 10/50/100-year return period flood frequency under the SSP585 scenario.
Under scenario SSP126, the average annual occurrences of 10-, 50-, and 100-year return period flooding in Qinghai province from 2020 to 2060 will amount to 53, 19, and 9, respectively. In 2057, the number of extreme floods occurring within the 10-year return period will increase sharply and will reach 176 in 2060. In 2060, the number of the 50-year return period flood will be 91 and the number of 100-year return period floods will rise to its peak of 25 in 2055.
Under the scenario SSP245, the average number of 10-, 50-, and 100-year return period floods in Qinghai province in the future will be 52, 19, and 10 per year, respectively. The frequency of occurrences of these three types of flood return periods shows a slow-rising trend and will increase significantly in 2056. In 2059, the number of extreme floods during the 10-year return period will increase to a peak of 143. Both that number of 50-year return period and 100-year return period flood will reach peaks of 56 and 40 in 2058, respectively.
Under scenario SSP585, the average number of 10-, 50-, and 100-year return period floods in Qinghai province in the future will be 58, 21, and 10 per year, respectively. In 2060, the number of these three types of extreme floods will reach their peaks of 265, 90, and 26, respectively.
By comparing the total number of extreme disasters of different return periods, the frequency of flood of diverse return periods under scenario SSP585 is significantly higher than that under SSP126 and SSP245. From 2020 to 2060, the smaller the emission reduction, the higher the frequencies of extreme rainstorms and flood disasters and their peaks.
In conclusion, under three scenarios in Qinghai province from 2020 to 2060, the occurrence frequencies of the extreme flood occurring within a 10-, 50-, and 100-year return period all show an increasing trend. However, compared with scenario SSP126, under scenario SSP585 with high emission, extreme flood disasters occurring within the 100-year return period may have 37 extra cases, those within the 50-year return period may have 94 extra cases, and those within the 10-year return period may have 167 extra cases.
According to casualties caused by diverse return periods of flood from historical disaster data, if the emission reduction target (SSP126 scenario) could be achieved, it might lower the casualties by the numbers of 58, 77, and 88, respectively, due to minimizing the occurrence of 10-year return period/50-year return period/100-year return period flood (compared with SSP585 scenario). Therefore, measures to reduce emissions may significantly cut the potential loss of life caused by floods.
1) Analysis of the characteristics of a county loss ratio of rainstorm and flood disasters in Qinghai province in typical years
Figure 6 shows the distribution of the loss ratio of rainstorm and flood disasters in each county of Qinghai province in 2030, 2050, and 2060 under three scenarios. The disaster loss ratio in Qinghai province will be in a descending pattern from southeast to northwest in three typical years. The southeast of Qinghai province suffers a bigger loss than other regions of the province, while the west and north suffer less. In Qinghai province, the counties of Banma, Dari, Gande, Jiuzhi, and Nangqian will all have high or mid-high loss ratios under scenarios SSP126, SSP245, and SSP585, and the eastern part of Qinghai will suffer mid-high loss ratios, the central and southwestern parts will have medium loss ratios, and the western part will be subjected to low or very low loss ratios.
FIGURE 6. Spatial distribution of the loss ratio in Qinghai province in 2030/2050/2060. (A–C) Countries’ flood loss ratios under three scenarios in 2030; (D–F) countries’ flood loss ratios under three scenarios in 2050; and (G–I) countries’ flood loss ratios under three scenarios in 2060.
According to the figure for 2030, the distribution pattern of the high and low values of the loss ratio in each county under the three emission scenarios is similar. Under scenario SSP126, the areas with the highest loss ratios are Jiuzhi, Banma, Gande, Dari, and Nangqian counties successively. The loss ratios of Jiuzhi, Banma, and Gande counties are all higher than 1%, and the sum of that is 3.17%, accounting for more than half of the total loss ratio of rainstorm and flood disasters in 2030. Under scenario SSP245, the loss ratios of Banma, Nangqian, and Gande counties are the highest, with a sum of 3.67%. Under scenario SSP585, the loss ratios of Jiuzhi, Banma, Dari, and Gande counties are significantly higher than those of other regions, and the sum of that is 4.56%, accounting for nearly 60% of the province. In 2050, the counties of Banma, Jiuzhi, Dari, Gande, and Nangqian will be the five counties with the highest loss ratios of rainstorm and flood disasters in Qinghai under the three scenarios, and the sum of their loss ratios will be 5.784%, 14.830%, and 27.611%, respectively, under the low-, medium- and high-emission scenarios, accounting for 48.5%, 72.0%, and 79.0% of the province, respectively. In 2060, the counties of Banma, Jiuzhi, Dari, Gande, and Nangqian still suffer higher loss ratios, but the sum of their loss ratios is 2.40%, 2.82%, and 3.10%, respectively, under the low-, medium-, and high-emission scenarios, accounting for 36.9%, 42.4%, 42.9% of the province, respectively.
Under the three scenarios of SSP126, SSP245, and SSP585, the loss ratios of each county in Qinghai province will reach the peak in 2050 and drop to a trough in 2060, which is consistent with the results. Before China achieves a carbon peak in 2030, fossil fuels will stay as its main energy. So, carbon dioxide emissions may reach a peak, which may increase the frequency and intensity of flood disasters to some extent, leading to the reduction of the disaster loss ratio later than carbon peaking. China plans to achieve carbon neutrality by 2060, and the reduction of the disaster loss ratio caused by social and economic transformation will be earlier than achieving that goal and reaching its lowest point in 2060. With the growth of carbon emissions, more regions will suffer high losses in the province, causing more extreme and concentrated flood disasters.
2) The distribution of extreme flood loss ratios in Qinghai province in 2050
In 2050, by adding and counting the loss ratios of the extreme flood of different return periods for each county in Qinghai province, the spatial loss distribution characteristics of extreme floods with different intensities in specific years in Qinghai province are shown in Figure 7.
FIGURE 7. Spatial distribution of the cumulative loss ratio of extreme rainstorms and flood disasters in Qinghai province in 2050. (A–C) countries’ cumulative flood loss ratios of the 10-year return period under three scenarios; (D–F) countries’ cumulative flood loss ratios of the 50-year return period under three scenarios; and (G–I) countries’ cumulative flood loss ratios of 100-year return period under three scenarios.
Under the same scenario, 10-, 50-, and 100-year return period flood disasters all have the greatest losses in the counties of Banma, Jiuzhi, Gande, Dari, and Nangqian. The spatial impact of the extreme flood is similar to the distribution of the high and low values of flood loss ratios in typical years in Qinghai. With the growing rate of emissions, the area affected by the extreme flood (especially the 10-year return period flood) decreases, but the cumulative loss ratio of the key disaster areas affected by the extreme flood will rise. Therefore, the higher the emission scenario is, the stronger the extreme of flood will be, and the disaster-prone areas such as Banma, Jiuzhi, Gande, Dari, and Nangqian counties will suffer higher risks.
Among the 44 counties in Qinghai province, the three counties with the highest frequencies (1–2 times) of 100-year return period flood in 2050 are Banma county, Jiuzhi county, and Yushu City. The losses caused by floods to these three areas account for 0.042%–1.187% of their GDP, respectively. The ranges of their total loss ratio are from 0.26% to 1.187%, 0.176%–0.625%, and 0.048%–0.185%, respectively. In addition, the spatial distribution of cumulative loss ratios of flood in 2050 also has significant unevenness in Qinghai province, and the counties with higher cumulative loss ratios are mainly concentrated in the southern part such as Banma county, Gande county, Dari county, Jiuzhi county, and Yushu City.
1) In the past, due to the improvement of China’s disaster statistics system (mostly after the Wenchuan earthquake in 2008) (Guo et al., 2016) and the strength of the actual disaster statistics department in Qinghai province, data before 2009 are difficult to obtain. However, in this article, there are still 1,512 disaster data from 2009 to 2019 and more than 100,000 daily precipitation data. In addition, climate change and economic development in Qinghai province start to increase during the period 2009–2019. Therefore, the historical disaster data from 2009 to 2019 can represent the future trend to a certain extent.
2) This study only analyzed the difference of future precipitation change dominated by the single precipitation factor, without integrating temperature and precipitation calculation to synthesize the loss rate. This may result in insufficient consideration of flood disasters caused by temperature changes in the plateau region. However, some studies (Ping et al., 2014; Ji et al., 2015) on the risk of flood disaster in plateau areas also show that the flood disaster is more related to extreme precipitation. In addition, the influence of pressure and temperature on precipitation is taken into account in future precipitation dates in the CMIP6 climate model. Still, a comprehensive temperature and precipitation assessment of floods in Qinghai province will be the focus of future research.
3) The future meteorological data used in this study are from the CMIP6 global climate model (GCM). Although this model is the most authoritative future climate prediction data at present, its simulation accuracy is still limited for county-level areas (Kim et al., 2020) and also for high-altitude areas (Zhu et al., 2020; Zhu and Yang, 2020; Lun et al., 2021), which may bring some uncertainties to the results. For example, there may be errors in the accuracy of the simulation effect for the relatively developed areas in the northeast of Qinghai province, such as Xining, the capital city of Qinghai province. If smaller scale meteorology model data are available, historical model data can be corrected from historical site data to obtain more accurate future model data. The ideal data may take a regional climate model (RCM) to simulate the precipitation for Qinghai province in the future, but the RCM parameter setting needs field experiment and experience estimation (Weigel et al., 2021), and this could also bring the subjective experience of error and uncertainty. In order to reduce the uncertainty, this study uses 22 models of collection and data processing methods.
4) This study demonstrates the contribution of emission reduction through the assessment of regional flood disaster risk. But the direct correlation between the risk reduction of flood disasters and the intensity of emission reduction is not shown. Although many studies have shown that floods are closely related to extreme precipitation and the positive relationship between regional extreme precipitation and climate change, the assessment of future flood risk changes in the region can reflect the effectiveness of emission reduction only to a certain extent. Therefore, the quantitative calculation of the relationship between disaster risk and emission reduction in the direction of future research and the further demand of government disaster reduction departments.
Based on the disaster data of more than 1,000 flood disasters from 2009 to 2019, observed precipitation data, and future precipitation and GDP data, this study constructed the loss rate curve of cumulative precipitation and disaster loss, evaluated the quantitative risk of flood disasters at the district- and county-scale in Qinghai province from 2020 to 2060, and compared the temporal and spatial distribution characteristics of loss rate differences under different scenarios and return periods. The main conclusions are as follows:
1) The flood disaster loss ratios of Qinghai province in 2020–2060 present a trend of “rising in the early stage, stable in the middle stage, and declining in the late stage,” under low- (SSP126) and medium- (SSP245) emission scenarios, and presenting a trend of rising fluctuation under the high-emission (SSP585) scenario, respectively, are (0.075%, 0.499%), (0.121%, 0.522%), and (0.125%, 0.806%). The results show that the future flood disaster risk of Qinghai province under the high-emission SSP585 scenario is significantly higher than that under the low-emission SSP126 scenario, and the maximum risk difference may reach up to10 times.
(2) Compared with the high-emission SSP585 scenario, the cumulative loss of Qinghai province in 2020–2060 under the emission reduction target scenario SSP126 will be reduced by 8.1203%. Based on Qinghai’s 2019 GDP of 294 billion CNY, the emission reduction target will avoid a potential economic loss of nearly 24 billion CNY in the future and an average of 582 million CNY per year, which is equivalent to 93% of Qinghai’s 2019 provincial special fund for ecological and environmental protection of 626 million CNY. In addition, the frequency of extreme flood disasters over a 100-year return period, 50-year return period, and 10-year return period may also be reduced by 37 times, 94 times, and 167 times, respectively, avoiding in total 223 casualties.
3) Without considering the impact of climate model accuracy and resolution on the assessment accuracy of northeastern Qinghai province, the high-value areas of the flood disaster loss ratio caused by 44 counties in Qinghai province in the future are mainly located in Banma county, Gande county, Dari county, Jiuzhi county, and Nangqian county in southeastern Qinghai province. Under SSP585, Jiuzhi county, Banma county, and Nangqian county may suffer the most severe losses from the flood disaster.
This study quantitatively reveals the spatial and temporal variation characteristics of flood risk and makes an application contribution to the improvement of flood risk assessment from “relative” risk degree to “absolute” risk degree in high-altitude regions. Therefore, this study provides data and method support for flood risk prevention and management in China’s high-altitude regions. More importantly, from the perspective of casualties and economic losses caused by flood disasters, this study evaluates the potential “positive impact” brought by multiple costs of emission reduction, providing theoretical and practical evidence for promoting the enthusiasm for emission reduction in China and even worldwide.
The raw data supporting the conclusion of this article will be made available by the authors, without undue reservation.
BW computed data, made diagrams, and wrote the manuscript; ZZ directed the thesis idea and revised the full manuscript; XG helped in data processing and diagrams; CT provided data for the study area; CH provided methods for processing data and writing; JT provided suggestions for revision of the manuscript.
This research was funded by the Second Tibetan Plateau Scientific Expedition and Research Program (No.2019QZKK0606), the National Natural Science Foundation of China (No. 41907395; No. 42171074), the China Postdoctoral Science Foundation (2021M690425), and the Fundamental Research Funds for the Central Universities (No. 2021NTST28). The authors declare that they have no known competing financial interests or personal relationships that could have appeared to influence the work reported in this article.
The authors declare that the research was conducted in the absence of any commercial or financial relationships that could be construed as a potential conflict of interest.
All claims expressed in this article are solely those of the authors and do not necessarily represent those of their affiliated organizations, or those of the publisher, the editors, and the reviewers. Any product that may be evaluated in this article, or claim that may be made by its manufacturer, is not guaranteed or endorsed by the publisher.
The Supplementary Material for this article can be found online at: https://www.frontiersin.org/articles/10.3389/feart.2022.931505/full#supplementary-material
Chen, J., Liu, L., Pei, J., and Deng, M. (2021). An Ensemble Risk Assessment Model for Urban Rainstorm Disasters Based on Random Forest and Deep Belief Nets: a Case Study of Nanjing, China. Nat. Hazards 107, 2671–2692. doi:10.1007/s11069-021-04630-y
Delalay, M., Ziegler, A. D., Shrestha, M. S., and Gopal, V. (2020). Methodology for Future Flood Assessment in Terms of Economic Damage: Development and Application for a Case Study in Nepal. J. Flood Risk Manag. 13, e12623. doi:10.1111/jfr3.12623
Dou, R., and Yan, J. (2013). Spatial-temporal Variations of Drought and Flood Intensities in East of Qinghai Province during Last 52 Years. J. Nat. Disasters 22, e12365. doi:10.1371/journal.pone.0148072
Duan, A., Xiao, Z., and Wu, G. (2016). Characteristics of Climate Change over the Tibetan Plateau under the Global Warming during 1979-2014. Clim. Change Res. 12, 374–381. doi:10.12006/j.issn.1673-1719.2016.039
Guo, G., Liu, L., Li, Y., Gao, J., Lin, S., and Wu, S. (2021). A Vulnerability Curve Method to Assess Risks of Climate-Related Hazards at County Level. Atmosphere 12, 1022. doi:10.3390/atmos12081022
Guo, X., Cui, P., Li, Y., Ma, L., Ge, Y., and Mahoney, W. B. (2016). Intensity-duration Threshold of Rainfall-Triggered Debris Flows in the Wenchuan Earthquake Affected Area, China. Geomorphology 253, 208–216. doi:10.1016/j.geomorph.2015.10.009
Ji, Y., Zhou, G., Wang, S., and Wang, L. (2015). Increase in Flood and Drought Disasters during 1500-2000 in Southwest China. Nat. Hazards 77, 1853–1861. doi:10.1007/s11069-015-1679-9
Jinhu, Y., Zhihong, J., Qiguo, Y., Xuying, S., and Yubi, Y. (2007). Anlysis on Extreme Precipitation Envent over the Northwest China in Flood Season. J. Desert Res. 27, 320–325.
Kalyanapu, A. J., Judi, D. R., McPherson, T. N., and Burian, S. J. (2012). Monte Carlo-Based Flood Modelling Framework for Estimating Probability Weighted Flood Risk. J. Flood Risk Manage 5, 37–48. doi:10.1111/j.1753-318X.2011.01123.x
Kim, Y.-H., Min, S.-K., Zhang, X., Sillmann, J., and Sandstad, M. (2020). Evaluation of the CMIP6 Multi-Model Ensemble for Climate Extreme Indices. Weather Clim. Extrem. 29, 100269. doi:10.1016/j.wace.2020.100269
Koc, K., and Işık, Z. (2021). Assessment of Urban Flood Risk Factors Using Monte Carlo Analytical Hierarchy Process. Nat. Hazards Rev. 22, 516. doi:10.1061/(asce)nh.1527-6996.0000516
Li, N., Wang, Y., and Zhang, Z. (2016). Transformation of Natural Disaster Risk Assessment Method Based on Number and Content of Scientific Papers. J. Catastrophology 31, 8–14. doi:10.3969/j.issn.1000-811X.2016.03.002
Lin, Q., Wang, Y., Glade, T., Zhang, J., and Zhang, Y. (2020). Assessing the Spatiotemporal Impact of Climate Change on Event Rainfall Characteristics Influencing Landslide Occurrences Based on Multiple GCM Projections in China. Clim. Change 162, 761–779. doi:10.1007/s10584-020-02750-1
Liu, Y., Qiu, H., Yang, D., Liu, Z., Ma, S., Pei, Y., et al. (2022). Deformation Responses of Landslides to Seasonal Rainfall Based on InSAR and Wavelet Analysis. Landslides 19, 199–210. doi:10.1007/s10346-021-01785-4
Lun, Y., Liu, L., Cheng, L., Li, X., Li, H., and Xu, Z. (2021). Assessment of GCMs Simulation Performance for Precipitation and Temperature from CMIP5 to CMIP6 over the Tibetan Plateau. Int. J. Climatol. 41, 3994–4018. doi:10.1002/joc.7055
Lv, Y., Liu, S., and Zhong, G. (2021). Flood Risk Assessment in Small Watershed Based on Catastrophe Theory. IOP Conf. Ser. Earth Environ. Sci. 691, 012016. doi:10.1088/1755-1315/691/1/012016
Ma, S., Qiu, H., Hu, S., Yang, D., and Liu, Z. (2021). Characteristics and Geomorphology Change Detection Analysis of the Jiangdingya Landslide on July 12, 2018, China. Landslides 18, 383–396. doi:10.1007/s10346-020-01530-3
Meihua, Z., Yun, S., and Jingnai, Z. (2008). Trust in Local Disaster Reduction Ability and its Influences on Public Flood Risk Perception: Based on the Investigation and Analysis. J. Catastrophol. 23(4), 70–75. doi:10.3969/j.issn.1000-811X.2008.04.015
O'Neill, B. C., Tebaldi, C., van Vuuren, D. P., Eyring, V., Friedlingstein, P., Hurtt, G., et al. (2016). The Scenario Model Intercomparison Project (ScenarioMIP) for CMIP6. Geosci. Model. Dev. 9, 3461–3482. doi:10.5194/gmd-9-3461-2016
Peng, Y., Chen, K., Yan, H., and Yu, X. (2017). Improving Flood-Risk Analysis for Confluence Flooding Control Downstream Using Copula Monte Carlo Method. J. Hydrologic Eng. 22, 04017018. doi:10.1061/(asce)he.1943-5584.0001526
Ping, H., Jinxiao, L., Guoqin, X., and Hongbo, L. (2014). Analysis of Precipitation Distribution and Drought and Flood Disasters inChuxiong City on the Yunnan Plateau. Earth Environ. 42, 162–167. doi:10.14050/j.cnki.1672-9250.2014.02.005
Ridder, N. N., Ukkola, A. M., Pitman, A. J., and Perkins-Kirkpatrick, S. E. (2022). Increased Occurrence of High Impact Compound Events Under Climate Change. npj Clim. Atmos. Sci. 5, 3. doi:10.1038/s41612-021-00224-4
Su, X., Shao, W., Liu, J., Jiang, Y., and Wang, K. (2021). Dynamic Assessment of the Impact of Flood Disaster on Economy and Population under Extreme Rainstorm Events. Remote Sens. 13, 3924. doi:10.3390/rs13193924
Wang, Z., Wang, H., Huang, J., Kang, J., and Han, D. (2018). Analysis of the Public Flood Risk Perception in a Flood-Prone City: The Case of Jingdezhen City in China. Water 10, 1577. doi:10.3390/w10111577
Weidong, M., Fenggui, L., Qiang, Z., Qiong, C., Cungui, Z., Fei, L., et al. (2021). Estimation of Critical Rainfall for Flood Disasters in the Qinghai-Tibet Plateau. J. Resour. Ecol. 12, 600–608. doi:10.5814/j.issn.1674-764x.2021.05.003
Weigel, K., Bock, L., Gier, B. K., Lauer, A., Righi, M., Schlund, M., et al. (2021). Earth System Model Evaluation Tool (ESMValTool) v2.0 - Diagnostics for Extreme Events, Regional and Impact Evaluation, and Analysis of Earth System Models in CMIP. Geosci. Model. Dev. 14, 3159–3184. doi:10.5194/gmd-14-3159-2021
Wu, F., and You, Q. (2019). Temperature Change on the Tibetan Plateau under the Global Warming of 1.5°C and 2°C. Clim. Change Res. 12, 130–139. doi:10.12006/j.issn.1673-1719.2018.175
Yang, D., Qiu, H., Ma, S., Liu, Z., Du, C., Zhu, Y., et al. (2022). Slow Surface Subsidence and its Impact on Shallow Loess Landslides in a Coal Mining Area. Catena 209, 105830. doi:10.1016/j.catena.2021.105830
Zeleňáková, M. (2009). Preliminary Flood Risk Assessment in the Hornád Watershed. WIT Trans. Ecol. Environ. 124, 15–24. doi:10.2495/RM090021
Zhou, W., Qiu, H., Wang, L., Pei, Y., Tang, B., Ma, S., et al. (2022). Combining Rainfall-Induced Shallow Landslides and Subsequent Debris Flows for Hazard Chain Prediction. Catena 213, 106199. doi:10.1016/j.catena.2022.106199
Zhu, H., Jiang, Z., Li, J., Li, W., Sun, C., and Li, L. (2020). Does CMIP6 Inspire More Confidence in Simulating Climate Extremes over China? Adv. Atmos. Sci. 37, 1119–1132. doi:10.1007/s00376-020-9289-1
Zhu, Y.-Y., and Yang, S. (2020). Evaluation of CMIP6 for Historical Temperature and Precipitation over the Tibetan Plateau and its Comparison with CMIP5. Adv. Clim. Change Res. 11, 239–251. doi:10.1016/j.accre.2020.08.001
Keywords: Qinghai–Tibet Plateau, risk assessment, flood, loss rate, carbon neutral
Citation: Wu B, Zhang Z, Guo X, Tan C, Huang C and Tao J (2022) Spatial and Temporal Analysis of Quantitative Risk of Flood due to Climate Change in a China’s Plateau Province. Front. Earth Sci. 10:931505. doi: 10.3389/feart.2022.931505
Received: 29 April 2022; Accepted: 16 May 2022;
Published: 24 June 2022.
Edited by:
Haijun Qiu, Northwest University, ChinaCopyright © 2022 Wu, Zhang, Guo, Tan, Huang and Tao. This is an open-access article distributed under the terms of the Creative Commons Attribution License (CC BY). The use, distribution or reproduction in other forums is permitted, provided the original author(s) and the copyright owner(s) are credited and that the original publication in this journal is cited, in accordance with accepted academic practice. No use, distribution or reproduction is permitted which does not comply with these terms.
*Correspondence: Zhengtao Zhang, emhhbmd6aGVuZ3Rhb0BibnUuZWR1LmNu
Disclaimer: All claims expressed in this article are solely those of the authors and do not necessarily represent those of their affiliated organizations, or those of the publisher, the editors and the reviewers. Any product that may be evaluated in this article or claim that may be made by its manufacturer is not guaranteed or endorsed by the publisher.
Research integrity at Frontiers
Learn more about the work of our research integrity team to safeguard the quality of each article we publish.