- 1School of Earth Science and Technology, Southwest Petroleum University, Chengdu, China
- 2State Key Laboratory of Oil and Gas Reservoir Geology and Exploitation, Southwest Petroleum University, Chengdu, China
- 3Exploration and Development Research Institute, PetroChina Southwest Oil and Gas Field Company, Chengdu, China
The evaluation of reservoirs is of great importance in exploration practices, and reservoir distribution is dependent on multiple geological factors. The carbonate platform of the fourth member (Z2dn4) of the Dengying Formation (Z2dn) in the Gaoshiti-Moxi area (GMA) was uplifted above sea level during episode II of the Tongwan movement and underwent prolonged karstification by meteoric freshwater in the terminal Sinian, leading to a strong heterogeneity in reservoirs. Paleogeomorphology reflects hydraulic gradients that can affect the intensities of surface karstification and may be approximated by terrain slope gradients. Karst products (especially solution vugs and caverns) exist prevailingly in the Z2dn4; their development mirrors the extents of internal dissolution. Based on paleogeomorphological reconstruction using the elevation method, the slope angles of single boreholes were calculated through the digital elevation model (DEM). In accordance with single-borewell Fullbore Formation MicroImager (FMI) logging data, four electrofacies (host rock, vug, fracture, and cave) were identified in boreholes possessing FMI data. The identified electrofacies were matched with corresponding conventional logging curves to obtain the training data. A model generalized for other boreholes without FMI data was established with the random forest algorithm and the thicknesses of the four electrofacies in each borehole were determined to characterize the extent of karstification. Slope gradients and electrofacies thicknesses, together with multiple physical parameters of reservoirs in boreholes, were utilized for factor analysis. Four factors were obtained and fused into one comprehensive score based on their ratios of variance proportions. The favorability of reservoirs was described by the comprehensive scores of each borehole. These analyses showed that the most advantageous reservoirs are distributed in the vicinity of the platform margin in the west of the GMA, where they enjoy several benefits. Deposition of porous mound-shoal complexes occurs predominantly in the platform margin where large-scale caves are more developed in the mixing karst zone of seawater and freshwater. Meanwhile, the transmeridional lateral migration of hydrocarbons from the regional hydrocarbon-generating center abutting the west of the GMA contributes to earlier entrapment and accumulation in the mound-shoal complexes in the platform margin.
1 Introduction
In the most important reservoirs of petroliferous basins around the world, carbonate rocks have consistently been a major research focus for petroleum exploration in both academia and industry (Calner et al., 2010). Carbonate reservoirs account for approximately 50% of total reserves and represent 60% of total hydrocarbon production in the world (Gomez-Rivas et al., 2014; Su et al., 2021; Chen et al., 2022), with large-scale marine carbonate reservoirs discovered in petroliferous basins worldwide (Dyman et al., 2002; Halbouty, 2003; Biteau et al., 2006). Increasing demand and continuous consumption of fossil fuels have prompted petroleum exploration to expand from medium-shallow to deep and even ultra-deep domains of carbonate reservoirs. Deep and ultra-deep carbonate strata with depths exceeding 4,500 m are hotspots for oil and gas exploration and exploitation (Yang et al., 2021) and represent reserves of around 35% of global hydrocarbons (Ma et al., 2020).
However, the compaction of deep carbonate reservoirs using burial results in low interconnectivity and porosity (Morad et al., 2018; Perrin et al., 2020). High-quality reservoirs with low permeability and porosity that produce commercial hydrocarbon flow may be subject to the development of secondary porosity and fractures. These may be caused by constructive diageneses such as karstification and recrystallization and tectonic movement (Abarca et al., 2019; Dai et al., 2020; Dong et al., 2020). Karstification has been shown to play a significant role in enhancing the integrated reservoir quality, e.g., porosity and permeability, throughout the duration of diagenesis (James and Choquette, 1988; Ronchi et al., 2010; Sayago et al., 2012; Chen et al., 2022). Meteoric freshwater percolates and diffuses downward into inner carbonate strata and dissolves the soluble calcium minerals, resulting in considerable porosity due to secondary dissolution, caverns, and dissolution fractures that augment extra storage spaces (Loucks and Anderson, 1985; Moore and Wade, 2013). Prolonged karstification following integral platform exposure can cause disconformity, i.e., general weathering crust in carbonate reservoirs. Thus, karstification is associated with disconformity, and paleokarst reservoirs are also related to disconformity (Fritz et al., 1993; Zhu et al., 2019; Chen et al., 2022). This is highly prevalent, as 20%–30% of global oil and gas resources are subject to unconformities (Fritz et al., 1993; Burchette, 1996). Relief of disconformity may be implicitly related to and reflect the karst intensity, which is influenced by karst hydraulic gradients and chemical denudation rate. Prediction of karst reservoirs through reconstruction of paleogeomorphology, therefore, should be feasible and valid (Tang et al., 2013). Additionally, different methods have been employed to reconstruct paleogeomorphology, and interpretations of the outcomes demonstrate that hydrocarbons are apt to accumulate in one or more paleogeomorphological units, e.g., monadnock and karst slope (Tang et al., 2013; Jin et al., 2017; Yu et al., 2021). This type of result demonstrates the utility and feasibility of paleogeomorphological reconstruction.
The evaluation and prediction of paleokarst carbonate reservoirs are an important but challenging aspect of hydrocarbon exploration due to the extreme complexity and heterogeneity of karst systems, which are controlled by subaerial karstification and burial alteration after tens to hundreds of millions of years (Li R. et al., 2021; Zhang et al., 2022). Previous studies on paleokarst reservoir evaluation and prediction based on conventional paleogeomorphological reconstruction methods are commonly qualitative or semi-quantitative, which can only indirectly reflect karst fluid hydrodynamics and cannot depict internal karst systems in line with the degree of karstification. Conventional well logging curves and Fullbore Formation MicroImager (FMI) are high-resolution means for identifying electrofacies such as host rocks, fractures, vugs, and paleocaves of paleokarst reservoirs (Tian et al., 2019; Zheng W. et al., 2021). These identified electrofacies also reflect karst system development. Porosity, permeability, and other parameters that characterize pore system geometry is only used to authenticate the validity of reconstructed paleogeomorphology, yet key parameters would be missed in reservoir evaluation and prediction.
For responsible paleokarst reservoir evaluation and prediction, it is essential to consider and integrate multiple features that affect reservoir development, i.e., lithofacies, diagenetic alteration and tectonics, and corresponding characteristic parameters. The core idea of multi-feature or multi-parameter analysis in reservoir evaluation and prediction is that a comprehensive dimensionless parameter can effectively substitute and represent other characteristic attributes and reserve most of the data (Li Y. et al., 2021). Factor analysis (FA), principal component analysis (PCA), and linear discrimination analysis (LDA) are commonly used in multi-feature dimensional reduction, where FA and PCA are unsupervised analysis approaches, but LDA is supervised (Wang and Lu, 2021). In addition, the integrated single parameter can be obtained also by other weighting analyses, e.g., analytical hierarchy process (AHP) (Kayastha et al., 2013), greyness related analysis (GRA) (Jia et al., 2016), ReliefF algorithm (RFA) (Reyes et al., 2015), entropy weight method (EWM) (Li Y. et al., 2021), and critic weight method (CWM), etc. Moreover, FA, PCA, and LDA are also weighting analytical methods.
The Sinian Z2dn of the Gaoshiti-Moxi area in the Central Sichuan Basin has been discovered in recent years to be a giant gas field, which was a major breakthrough (Liu et al., 2015; Luo et al., 2017; Xie et al., 2019; Zhou et al., 2020). The proven total reserves of natural gas were as high as 1 × 1012 m3, and the cumulative gas production had reached 4.19 × 1010 m3 by the end of 2018 (Li et al., 2020). The interface between the Z2dn4 and the overlying Maidiping Formation (Є1m) or Qiongzhusi Formation (Є1q) is a disconformity. It is widely understood that the disconformity is a consequence of meteoric freshwater leaching and eroding after the regional tectonic uplift of the Tongwan Movement episode II (Luo et al., 2015; Yang et al., 2016; Wei et al., 2016; Zhou et al., 2016; Luo et al., 2017; Hao et al., 2017), though a debate co-exists on which karstification type (eogenetic, hypogenetic, or epigenetic) dominates the formation of numerous karst products in the Z2dn4 (Tang et al., 2013; Luo et al., 2015; Zhou et al., 2016; Jin et al., 2017; Che et al., 2019; Li et al., 2019; Jin et al., 2020; Zhou et al., 2020). Karstification is regarded as the vital diagenetic alteration in the formation of Z2dn4 reservoirs in the Gaoshiti-Moxi area (Luo et al., 2017; Shan et al., 2017; Duan et al., 2019; Zhou et al., 2020). However, it is difficult to quantitatively and precisely evaluate and predict paleokarst reservoirs via a single approach such as through paleogeomorphological reconstruction, paleokarst development characterization, or some other petrophysical parameters.
This study is aimed at integrating targets of paleogeomorphological reconstruction, paleokarst development characterization, and reservoir physical properties to quantitatively evaluate and predict potentially favorable areas of reservoirs for Z2dn4 using machine learning. The elevation method of paleogeomorphological reconstruction was employed, and slope gradient data were obtained through slope analysis to approximate hydraulic gradients. The principles of random forest (RF) and FA were discussed. Constrained by high-resolution core analysis, conventional well logging data, and FMI, paleokarst electrofacies of boreholes were identified and their thicknesses were calculated to characterize paleokarst development. Combined with porosity and permeability, FA was used to reduce data dimensions and fuse multi-features into four factors that describe the development of paleokarst reservoirs. Weight coefficients were derived from the variance contribution rate of each factor multiplied by the factor value to obtain final reservoir evaluation values. The results can essentially quantify the favorability of each paleokarst reservoir and illustrate the principles of Z2dn4 reservoir development and regulation.
2 Geological Settings and Stratigraphy
The Sichuan Basin is a constituent part of the Upper Yangtze Block located in Southwest China with an area of about 26 × 104 km2. Since its formation, the basin has undergone multiple tectonic movements in geological history. Its current structural pattern is the consequence of the integrative action of all tectonic movements since the Precambrian. The whole Sichuan Basin can be subdivided into six structural domains based on structural characteristics: the western high-steep structural belt (①), the northern low and gentle structural belt (②), the eastern high-steep structural belt (③), the southern low-steep structural belt (④), the southwest low-steep structural belt (⑤), and the central gentle structural belt (⑥) (Figure 1A). The Gaoshiti-Moxi Area (GMA), situated in the gentle structural belt of the Central Sichuan Basin, is close to the low-steep structural belt to the south. Crossing the Mianyang-Changning intracratonic sag, the low-steep and high-steep structural belts lie in the west (Figure 1A).
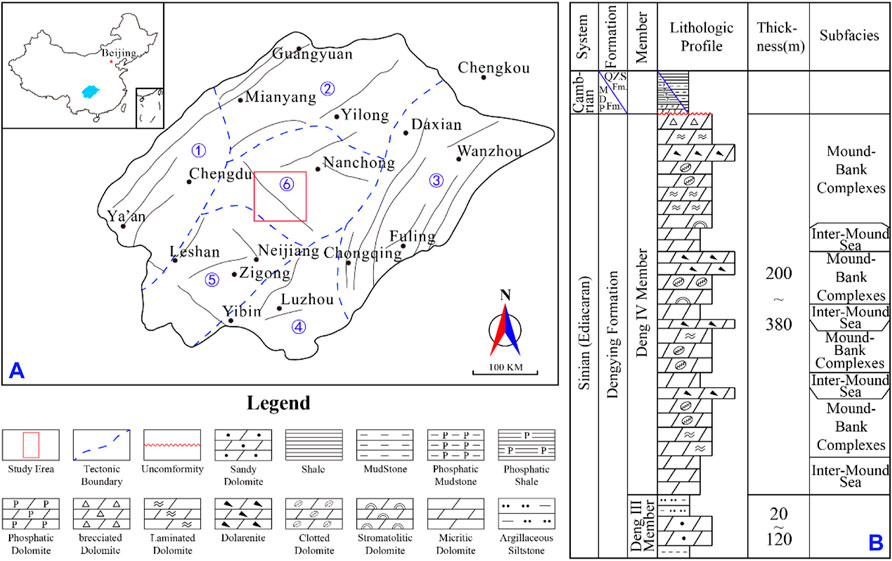
FIGURE 1. (A) structural map of the Sichuan Basin and the position of the study area. (B) comprehensive stratigraphic section of the Z2dn4 in the GMA.
The Z2dn4 of the GMA is the uppermost section of the Z2dn, mainly consisting of microbial and micritic dolomites of platform carbonates, the thickness of which ranges from 200 to 380 m. A disconformity on top of the Z2dn separates the overlying Є1m and Є1q and the underlying Z2dn4, and a subjacent third member of the Z2dn (i.e., Z2dn3) composed mainly of siltstone, silty mudstone, mudstone, and sandy dolomite vertically connects with the Z2dn4 (Figure 1B). Tectonic uplift in the late Sinian resulted in extensive exposure of the Sichuan Basin, and the warm paleoclimate caused abundant and frequent atmospheric precipitation (Zhang et al., 2014; Wang J. et al., 2020), producing prolonged leaching and infiltration of soluble carbonates that cultivated the complex karst system. Large quantities of organic matter from the basin floor in the Yangtze block oxidized due to the regional uplift, and the CO2 produced increased the partial pressure of CO2 in the atmosphere and elevated the acidity of meteoric precipitation. This further facilitated karstification, especially the chemical denudation in the platform part (Li et al., 2017). Karst phenomena and products are pervasive in Z2dn4, and reservoir characteristics are intrinsically related to karstification.
3 Methods
The distribution of reservoirs is generally considered a consequence of multiple factors and their interactions. It is, therefore, appropriate to apply mathematical tools to estimate reservoir distributions through multifactor analysis (Li Y. et al., 2021). Paleogeomorphological reconstruction using a quantitative digital elevation model (DEM) was performed to obtain the slope gradients of karst paleogeomorphology, which approximate the karst hydraulic gradients that characterize hydraulic potentials. The DEM is modeled from elevation values that are obtained from the reconstructed paleogeomorphology utilizing the elevation method, and the slope gradient data are acquired from slope calculation in the ArcGIS software. The RF algorithm was employed to identify the electrofacies of the host rock, fracture, vug, and cave in boreholes according to core analysis, thin sections, conventional logging data (i.e., GR, AC, DEN, CNL, RT, RXO, and RESD (resistivity difference between RT and RXO)), and FMI. The thicknesses of the electrofacies of each borehole served as features characterizing paleokarst development. A single estimating parameter (factor score) was calculated based on a quantitative evaluation approach (i.e., FA) combined with the integration of slope gradients, the thicknesses of the various electrofacies, and certain reservoir physical properties. This factor score was then used to plot a map of favorable reservoir distribution.
3.1 Elevation Method for Paleogeomorphological Reconstruction
Certain attempts of paleogeomorphological reconstruction performed in the Sinian/Cambrian boundary in the Gaoshiti-Moxi area have obtained some good achievements in recent years on the basis of the impression method and residual thickness-impression combination method (Wang et al., 2014; Liu et al., 2015; Jin et al., 2017). The most commonly used methods for paleogeomorphological reconstruction are the “residual thickness” method and the “impression” method (Yan et al., 2020). The residual thickness method is unsuitable for the Central Sichuan Basin for paleogeomorphological reconstruction, attributing to irrational onlaps that appeared on the reconstructed and interpreted karst highlands and depressions (Jin et al., 2017). Also, interpretations of reconstructed paleogeomorphology resulting from the impression method are opposite to the impression thickness. In other words, the thinner the impression thickness, the higher the geomorphology is. This might not be a big problem under normal circumstances; however, it could go badly wrong when applying the impression thickness to subsequently calculate the slope gradients. Therefore, we use the elevation method introduced by Yan et al. (2016) and Zhao et al. (2018) to reconstruct the paleogeomorphology of the Sinian/Cambrian interface. Compared to the impression method, the elevation method introduces a second base level (the lower base level) to convert the impression thickness into a quantitative elevation value. Also, the elevation value directly represents the reconstructed geomorphological height; thus, we can apply the elevation values to calculate the corresponding slope gradients. The overlying lower Cambrian sequences of Є1q- Є1c are a complete transgressive-regressive sedimentary cycle, which basically fills up the underlying Sinian/Cambrian karst geomorphology (Jin et al., 2017; Yan et al., 2020). The high-quality 2D and 3D seismic data are generally covered in the research area, and more than 40 boreholes have been drilled through the Z2dn4. Based on these data, it is feasible to perform paleogeomorphological reconstruction within the GMA.
3.1.1 Principles of Paleogeomorphological Reconstruction
The elevation method is similar to the impression method, especially in principle. The core idea for the two methods is to select an upper base level that corresponds to the transgressive surface or maximum flooding surface. The interval between the selected base level and the disconformity needs to fill up the disconformable interface, thus the morphological relief can be characterized by the thickness of the interval. The additional lower base level introduced by the elevation method is the nearest stratigraphic interface beneath the disconformity. The interface represents the underlying structural surface (in the context of leveling off the upper base level), and the relief of which affects the geomorphology of the overlying disconformity. The lowest point of this structural surface within the research area is regarded as the initial point of the elevation value which is equal to 0. A reference level horizontally extended from this point refers to a datum where the elevation value is 0. Also, the elevation values are calculated based on this horizontal reference level. Thus, the interval thickness between the disconformity and the upper base level is inversely converted to the interval thickness between the disconformity and the lower base level (i.e., the elevation value). The specific steps of the elevation method are as follows:
I) Selection of the upper base level that fills and levels the karst disconformity to characterize the ups and downs of the paleogeomorphology is the key to the paleogeomorphological reconstruction. The four criteria for selecting the upper base level are isochroneity, full covering within the scope of the research area, minimum distance away from the disconformity, and traceability in the seismic interface (Yan et al., 2016).
II) Once the upper base level is selected and flattened, the bottom boundary of one underlying stratum beneath the disconformity is requisite as the lower base level. The selection of the lower base level should meet the same requirements as those for selecting the upper base level. Then, the maximum thickness between the upper and lower base levels within the research area was identified and measured. From this point, a level surface was extended as the horizontal reference plane with an elevation of 0 m.
III) The elevations (HE) of other data points were equal to the maximum thickness (HTM) minus the impression thickness (HM). The equation can be given as follows:
where HE is the elevation, HTM is the maximum thickness between the upper base level and lower base level, and HM is the impression thickness.
The calculation model for the elevation is displayed in Figure 2A.
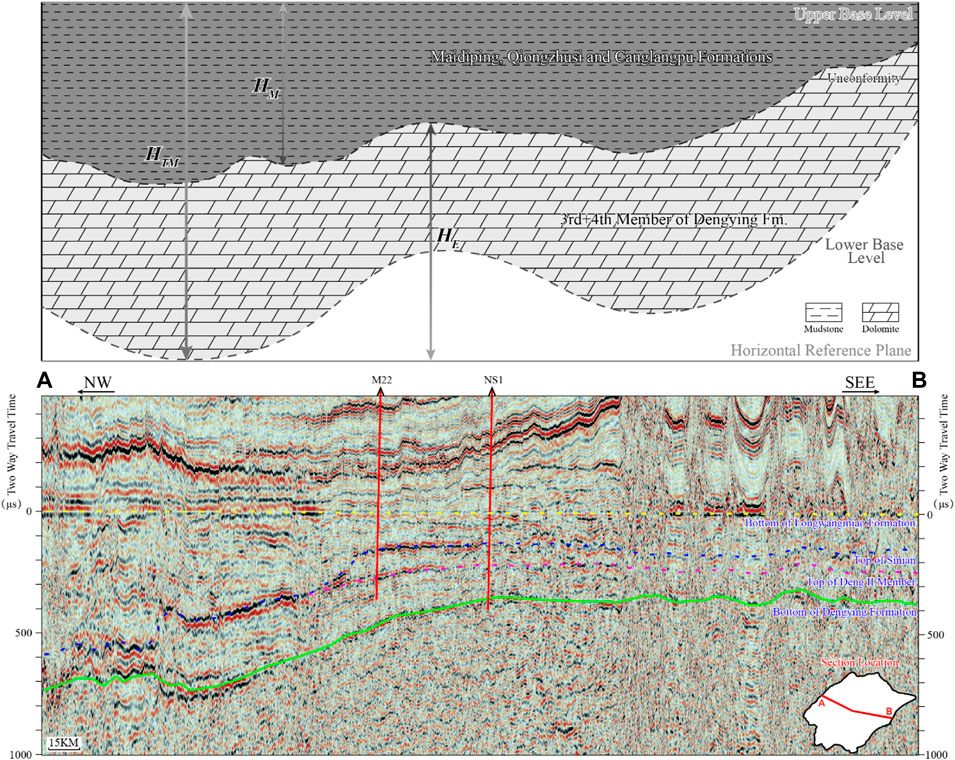
FIGURE 2. (A) principle of paleogeomorphological reconstruction via the elevation method (modified from Yan et al. (2016) and Zhao et al. (2018)); (B) seismic reflection profile of the upper and lower base levels. The bottom of the Є1l and the top of the Z2dn2 are optimal as the upper and lower base levels, respectively.
In addition, depth/thickness data points were obtained through time-depth conversion using drilling data and 2D and 3D seismic strata interpretations.
3.1.2 Selection of Base Levels
With regard to the reconstruction of the karst paleogeomorphology on the top of the Z2dn4 in the GMA, two base levels were selected: the upper base level—the bottom boundary of the Longwangmiao Formation (Є1l)—and the lower base level—the top of the Deng II member (Figure 2B).
The lower Cambrian successions consisted of the Є1m, Є1q, the Canglangpu Formation (Є1c), and Є1l, stacked upon the Z2dn. There were relatively small variations in the thickness of the Є1l in the GMA, implying that it was completely filled by deposits of the Є1c (Yang et al., 2016). Furthermore, the interface between the Є1l and Є1c is a transitional lithologic interface with preferable seismic traceability (Figure 2B), and an isochronous red bed is uniformly spread immediately close to the interface. Thus, the interface was optimal at the upper base level.
The interface between the Z2dn3 and the Z2dn2 is broadly distributed in the entire basin. This interface is a zonally isochronous disconformity. Moreover, the Z2dn3 is one of the marker layers in the region and exhibits an abrupt lithologic transition toward the Z2dn2. The interface between them is distinguishable and traceable (Figure 2B). For this reason, it was considered optimal at the lower base level.
3.2 Random Forest Algorithm
Random forest (RF) is a supervised ensemble algorithm containing multiple decision trees under a bagging framework (Breiman, 2001). The bagging framework performs random bootstrap resampling of the original data to generate input data for the RF that is equivalent to the original data in sample quantity. A number of decision trees are built through the selection of optimal features in differentiated random feature subspaces at splitting nodes, and the leaf nodes of each tree are established and labeled. The predictions of input sample categories are obtained via majority votes from all trees in the RF.
The decision tree is the foundation of the RF, the generation of which greatly affects the RF’s performance. The generation of a decision tree begins with a root node receiving input data. The root node then splits recursively in accordance with the optimal features in the random feature subspace until the total generated leaf nodes satisfy the stop-generating conditions. Each path from the root node to the leaf node represents a mode of regulation for classification, and the labels of the majority of samples in a leaf node would be taken as the output (Zhu et al., 2021). Multiple methods have been proposed for the feature-selecting criteria, including the ID3, C4.5, and the CART (Classification and Regression Tree) (Breimen et al., 1984; Olshen and Quinlan, 1986; Quinlan, 1992). The ID3 and C4.5 methods are both based on Shannon information entropy, except that the ID3 method seeks to maximize information gain while the C4.5 method focuses on maximizing information gain ratio. Meanwhile, the CART method aims to minimize the Gini index. The standard RF is built by decision trees based on CART splitting (Bai et al., 2022). The CART is the default option for the Python RF classifier and is the most commonly used method that works well in classification tasks. The CART method uses the Gini index minimization criterion, where the probability distributed Gini index is defined as follows:
For a given dataset S, its Gini index is calculated as follows:
If the dataset S is split into subsets S1 and S2 in accordance with one value of feature N, under the conditions of subsets S1 and S2 subdivided on the basis of feature N, the Gini index of dataset S is defined as
where K refers to categories of the data and pk is the probability of the category k,
A smaller Gini index indicates a lower uncertainty and higher accuracy of prediction. A CART constructed by the Gini index generates understandable rules, notwithstanding the possible rapid increase in classified error. The RF algorithm may resolve the problem reasonably effectively by constructing multiple decision trees. Each tree in the RF is generated by choosing optimal features from varied random feature subspaces at every non-leaf node; thus, all the trees are different from each other and characterize distinguished aspects of the feature space. As the majority votes determine the output category of the RF, uncertainty and classified error are greatly reduced, hence, enhancing the accuracy of the result compared to a single decision tree classifier.
3.3 Factor Analysis
Factor analysis (FA) is a statistical technique for extracting common factors from a multivariate. This technique can find hidden representative factors existing in multiple variables. By grouping variables that are intrinsically related into a single factor, the number of variables and the dimensions of the data are reduced, and the correlations among original variables are delineated. In general, the variables can be represented as a linear combination of common factors:
where vi (i = 1, 2, …, n) is the ith standardized variable, Fj (j = 1, 2, …, p) denotes the jth common factors, Ui (i = 1, 2, …, n) is the ith specific factor that only correlates to vi, and the coefficients aij and ci denote factor loading. The value of aij indicates the contribution of the ith original variable to the jth common factor.
The above equation can be expressed in the form of a matrix as
This is the factor analysis model, where v is the m-by-n data matrix, F is the m-by-p matrix of common factors, A is the n-by-p component matrix grouped by aij, T is the transpose symbol, and R is the m-by-n residual matrix.
The contributions of common factors to the variance of the ith original variable, i.e., the interpretation validity of factors, are denoted as follows:
where hi2 is the communality.
Estimation of the factor loading in FA is a basic problem. The most used approach is principal component analysis (PCA) based on a correlation matrix. The correlation matrix of original variables v can be obtained as follows:
where ψ is the diagonal matrix of specific variances of U and the factor loading matrix
Because the factors obtained are usually elusive and hard to interpret, the orthogonal transformation of factor loadings is applied so that the factors become easier to interpret (Lawley and Maxwell, 1962; Szabó, 2011). Generally, the transformation is achieved by factor rotation based on the varimax criterion (Kaiser, 1958). The rotated factor analysis model is calculated as follows:
where v* is the rotated data matrix,
The variance of the rotated factor loadings bij is calculated as follows:
Under the condition of
4 Results
4.1 Paleogeomorphology and Slope Gradients
The karst paleogeomorphology on top of the Sinian (Figure 3A) was plotted based on the difference between the maximum thickness and moulage thickness of the GMA. The paleogeomorphology of the study area is roughly composed of two parts: the low-lying part and the raised part, which are separated by a slope. The raised part is the main body of the Z2dn4 platform, and the low-lying part is a trough called the Mianyang-Changning intracratonic sag, which is the cumulative result of various geological processes, such as rifting, erosion, and dissolution (Li et al., 2015; Liu et al., 2016). Following the reconstruction of paleogeomorphology on top of the Z2dn4 in the GMA, a slope map was plotted according to the digital elevation model (DEM) (Figure 3B) to characterize its gradient distribution, which reflects the karst hydraulic gradient. Moreover, the slope gradient data of each borehole were obtained.
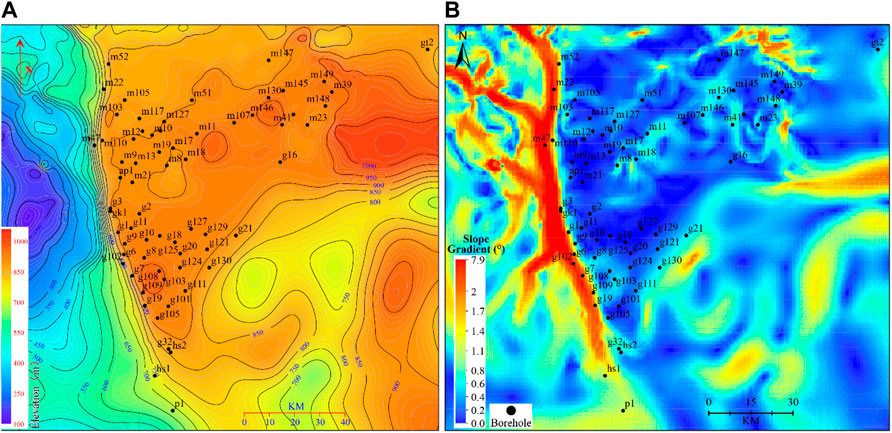
FIGURE 3. (A) reconstructed paleogeomorphology on top of the Z2dn4 in the GMA. (B) slope map corresponding to (A) based on DEM.
4.2 Electrofacies Identification in Boreholes
4.2.1 Selection of Conventional Logging Data
The gasfield in the GMA is a typical low-porosity and low-permeability reservoir. Its main storage spaces, vugs, and caves can be identified visually through high-resolution imaging. However, due to economic constraints, the technique was not applied to all boreholes (Zheng W. et al., 2021). Based on core-calibrated FMI images, the electrofacies of paleokarst reservoirs of Z2dn4 were divided into four types: host rock, fracture, vug, and cave.
The four types of electrofacies differ obviously in conventional logging data such as GR, AC, CNL, DEN, RT, and RXO (Figure 4). Thus, it is possible to identify the four electrofacies using basal conventional logging data. In addition to the aforementioned six types of logging data, the resistivity difference between RT and RXO (RESD) is also used in electrofacies identification. Therefore, the logging curves of GR, AC, CNL, DEN, RT, RXO, and RESD were selected as the seven dimensions of the input data for the RF.
4.2.2 Cleaning of Logging Data
Due to missing data in some characteristic dimensions, the data of the corresponding borehole interval were deleted. Furthermore, due to mismatches between conventional logging data and FMI images, non-matched data were also deleted. In addition, the popular boxplot technique was used to identify and remove irrational outliers in the logging data that can impair the fitting and prediction accuracy of the training model. Values were considered discrete outliers if they existed outside of the scope of normal values, defined by the range of the left and right boundaries, which relate to the lower and upper quartiles, respectively. The right boundary was determined by the upper quartile plus 1.5 times the interquartile range (IQR, the difference between the lower and upper quartiles), while the left boundary is determined by the lower quartile minus 1.5 times the IQR. Moreover, borehole interval data of inferior image quality were eliminated. Thus, the cleaned logging data were ready for the training classification model through the RF algorithm.
4.2.3 Accuracy of Classification by the Model
Prior to applying the supervised RF algorithm, the electrofacies in the conventional logging data of 16 boreholes were labeled via core-calibrated FMI. The 7-dimension logging data of the 16 boreholes served as input data and were split into 75% training dataset and 25% testing dataset for the hold-out cross-validation of the RF algorithm. The training dataset did not require further splitting due to the small size of both the training and validation datasets. This is because ∼36.8% of the out-of-bag data were not picked up by the single decision tree in the bootstrap process, that is, the out-of-bag data of the training dataset acted as the validation dataset. Due to the incompleteness of the training dataset, the whole input dataset was used as the training dataset to establish a more accurate model, and its accuracy was obtained by out-of-bag scoring. A 5-fold repeated stratified cross-validation was used to estimate the generalization capability of the trained RF model. Dummy classifiers using “stratified” and “most-frequent” strategies were applied as the benchmark classification model for comparison to the RF model. The results are shown in Table 1. The mean accuracies of the 5-split hold-out cross-validation were very close to the mean accuracy of the 5-fold repeated stratified cross-validation, all of which were slightly greater than 88% and much greater than 49.11% and 39.84% of dummy classifiers, respectively, showing a relatively good effect of classification. Based on the electrofacies prediction model constructed using the input data of 16 boreholes, the logging data of other boreholes without FMI images were used to predict the four types of electrofacies. The development thicknesses of the electrofacies in each borehole of the GMA were calculated.
4.3 Comprehensive Evaluation of Favorable Reservoirs
The calculated thickness of vuggy interval (TOVI), thickness of cave interval (TOCI), and slope gradients (SG) obtained earlier, together with the physical parameters of mean stratum porosity (MSPOR), mean stratum permeability (MSPER), mean reservoir porosity (MRPOR), mean reservoir permeability (MRPER), reservoir thicknesses (RT), reservoir/stratum ratio (RSR), reservoir quality index (RQI), and energy-storage coefficient (ESC) of the Z2dn4 of each borehole (Table 2) were applied to carry out factor analysis. As vugs and caves play a dominant role in reservoir spaces, their thicknesses describe reservoir space development. Therefore, the thicknesses of vuggy and cave intervals were taken into account for factor analysis. Also, the slope gradient implies the karst hydraulic gradient and was considered a characteristic parameter. The reservoir quality index, which indicates the hydraulic flow unit of the porous media, is also very useful. The two most important petrophysical properties, porosity and permeability, were integrated into one parameter (the RQI) (Al-Rbeawi and Kadhim, 2017), which reflects the microscopic pore structure and petrophysical properties of different reservoirs (Jiang et al., 2021). The RQI was calculated as
where k is permeability and φe denotes effective porosity.
The energy-storage coefficient indicates reservoir capacity and was calculated as follows:
where H denotes the effective thickness of the reservoirs.
The 11 characteristic parameters of each borehole were applied for factor analysis, producing four principal components that accounted for more than 85% of the variance obtained. Thus, the 11 parameters can be ascribed to four factors via factor rotation of the principal components. These four factors may be interpreted based on the rotated component matrix (Table 3). The first factor F1 primarily governed the mean stratum permeability, reservoir quality index, and mean reservoir permeability, reflecting the interconnectivity of the rock pore structure. The second factor F2 is mostly related to the reservoir/stratum ratio, reservoir thickness, and energy-storage coefficient, indicating reservoir development. The third factor F3 contributed mainly to slope gradient, thickness of cave intervals, and thickness of vuggy intervals, demonstrating karst impact. Finally, the last factor F4 primarily controlled the mean reservoir porosity, mean stratum porosity, and thickness of vuggy intervals, implying reservoir storage capacity.
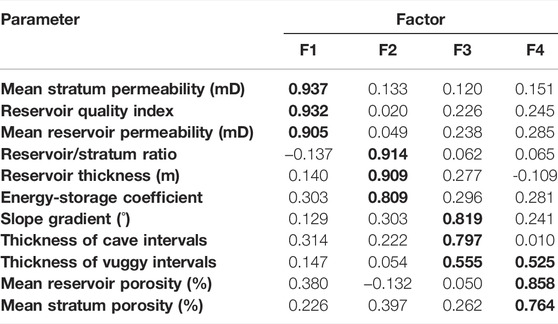
TABLE 3. Rotated component matrix. The bold values refer to large factor loadings, which indicates great impacts of different factors on various parameters.
After acquiring the rotated component matrix, the component score coefficient matrix was also obtained, in which the four factors were represented linearly by the 11 characteristic parameters/original variables as follows:
The comprehensive score of the factors of each borehole was calculated through the ratio of variance proportions of the four factors to the total cumulative variance proportion, i.e., the weight coefficient of each factor. The calculation formula is as follows:
The factor scores and comprehensive scores of each borehole are shown in Table 4. The comprehensive score is an integrated evaluation of the reservoirs using the aforementioned 11 original variables that characterize reservoir development. The distribution and evaluation of the reservoirs could be implemented by Kriging interpolation of single-well comprehensive scores, which is equivalent to map overlays of single-well factor scores considering corresponding weight coefficients (Figure 5).
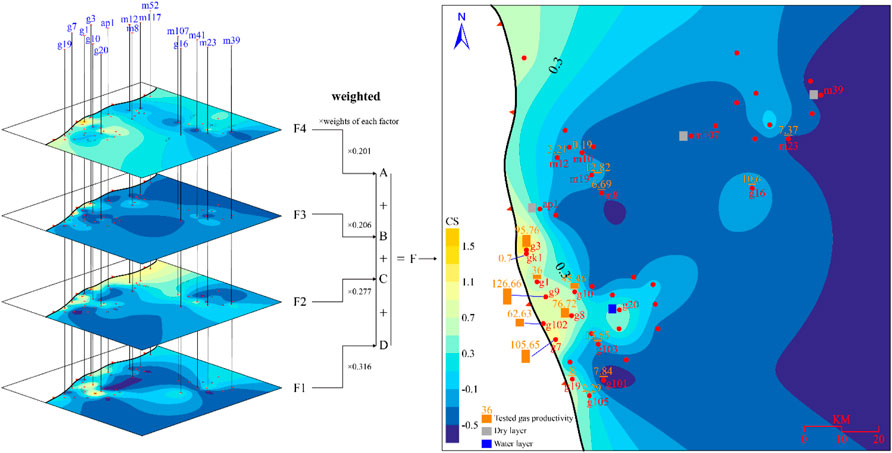
FIGURE 5. Comprehensive evaluation of reservoirs of Z2dn4 via factor analysis for the 11 characteristic parameters. Note the right panel, in which the tested gas productivities are superimposed on the comprehensive evaluation map and show a well-coupled matching with the comprehensive scores. CS-comprehensive score, dimensionless.
5 Discussion
Based on the comprehensive evaluation of favorable reservoirs, the tested gas productivity of each borehole was used to validate reservoir favorability (Figure 5, right panel). Gas testing data of boreholes corresponded well with their comprehensive scores. Reservoirs were more developed in the vicinity of the thin-out line, illustrating the heterogeneity in reservoir distribution. This heterogeneity may be related to several factors that affect reservoir development.
5.1 Distribution of Sedimentary Facies
The carbonate platform of Z2dn4 can be horizontally divided into the platform margin and platform interior based on their geometric anatomy. In the platform margin, the subtidal zone is normally dominated by thrombolites with intermittent laminae and fewer grains and transits upward to the high-wave energy lower intertidal zone. This region features combinations of granular rocks and corrugated stromatolites, which are characterized by their higher content of bonded grains. In the shallower upper part of the intertidal zone, the grain size reduces with decreasing wave energy, and the lithologies are primarily thin laminites with fine grains (Wen et al., 2017). Moreover, in the area only affected by storm waves, the supratidal zone, micrites containing dead microbial materials feature in mud cracks, bird-eye, and fenestral structures (Li A. P. et al., 2021). These imply evaporation- and degasification-controlled sedimentary environment that lies in the uppermost part of a shallowing-upward cycle.
Microbial frameworks are constructed and protrude around soft sediments. The microbial build-ups start with only a tiny convex structure, then accrete vertically and migrate laterally in response to sea-level eustacy. The frameworks may be broken by the agitation of the water column and shed redundant production sidewise after growing into a wave-acting zone. The broken clasts deposit in the flank of microbial mounds as grain shoals or aprons that are combined into mound-shoal complexes together with the encircled microbial mounds, and the complexes are separated by inter-mound depressions or lagoons. The longitudinal aggradation and lateral displacement of multi-stage complexes erect a protective platform margin in the west of the Z2dn4 in the GMA (Figure 6A).
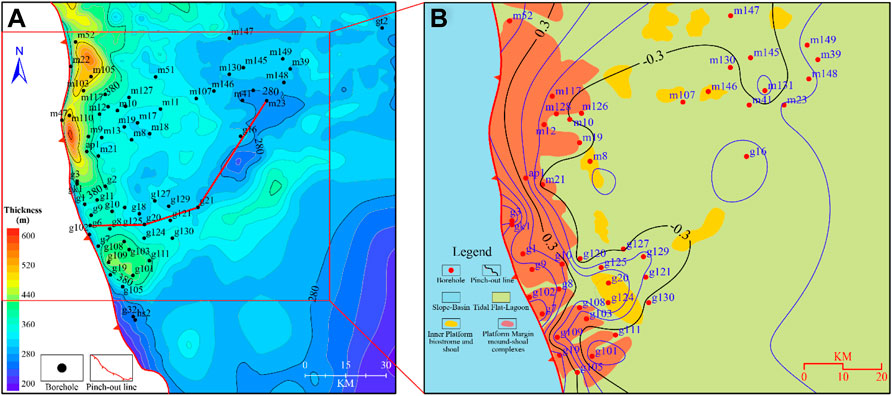
FIGURE 6. (A) converted residual thickness of Deng Ⅳ and Z2dn3s based on seismic data. Note the banded convex structure along the pinch-out line, which refers to the greater thickness in the platform margin. Due to the inferior traceability of the interface between the two members and the very thin thickness of the Z2dn3, the combined thickness is an acceptable approximation for the thickness distribution of the Z2dn4. (B) sedimentary facies of the Z2dn4 in the GMA superimposed with the reservoir evaluating line. The sedimentary base map is modified from Zhou et al. (2017) and Li A. P. et al. (2021).
The differences in primary porosities and pore structures, which closely correlate to microorganic activities, account for the differences among the porosities of the various sedimentary facies. The sedimentary framework with abundant microbial build-ups at the edge and scarce build-ups in the interior thus significantly affects the diageneses, particularly karstification. The primary pores of microbial mounds and grain shoals in the platform margin are bigger in size and, hence, are the preferential conduits for karst fluids. In addition, rigid microbial frameworks and coarse-grain sediments are more able to resist compaction, resulting in greater preservation of efficient reservoir spaces.
There is a possible “edge effect of dissolution” where dissolution is more intensive in the center than at the edge. This is because the lower flow velocity and longer dissolution time in a homogeneous model led to an elevated margin and a lower interior of the platform (Purdy and Winterer, 2001). The differences in sedimentary facies almost exist and the stratum of the Z2dn4 is actually heterogeneous. The thicker platform margin is more likely the result of differentiation in sedimentation, and the reconstructed paleogeomorphology on top of the Z2dn4 in the GMA (Figure 3A) also illustrates a flat terrain caused by prolonged deplanation. The platform margin is mainly occupied by shoal-water mound-shoal complexes, and the platform interior is a restricted platform that is a predominantly tidal flat and restricted lagoon with scattered patch mounds and shoals. Bioherms in the seismic sense are essentially complexes that are difficult to distinguish in seismic data due to limited horizontal resolution (Schlager, 2005). Therefore, the distribution of sedimentary facies plotted by chaotic seismic reflection configuration directing to mound-shoal complexes can indicate the distribution of complexes. By overlapping the favorable map with the planar distribution of sedimentary facies (Figure 6B), it was observed that the favorable reservoir area corresponds well with the mound-shoal area in the platform margin, indicating that high-quality reservoirs are distributed mainly in the mound-shoal complexes of the platform margin in the west of the GMA.
Statistically, the porosity distribution of the microbial mound, shoal, tidal flat, and lagoon of the Z2dn shows apparent variance. All samples from the tidal flat and lagoon were below 4% while considerable parts of those from the mound and shoal were above 4% (Hou et al., 2021) (Figure 7A). In addition, lithofacies demonstrated the highest porosity in dolarenite, followed by crystal-powder dolomite, stromatolite, thrombolite, and laminite, all of which may be underlying reservoirs (Figure 7B). In contrast, the micritic dolomite had the lowest porosity and has adverse effects on the reservoirs (Figure 7B).
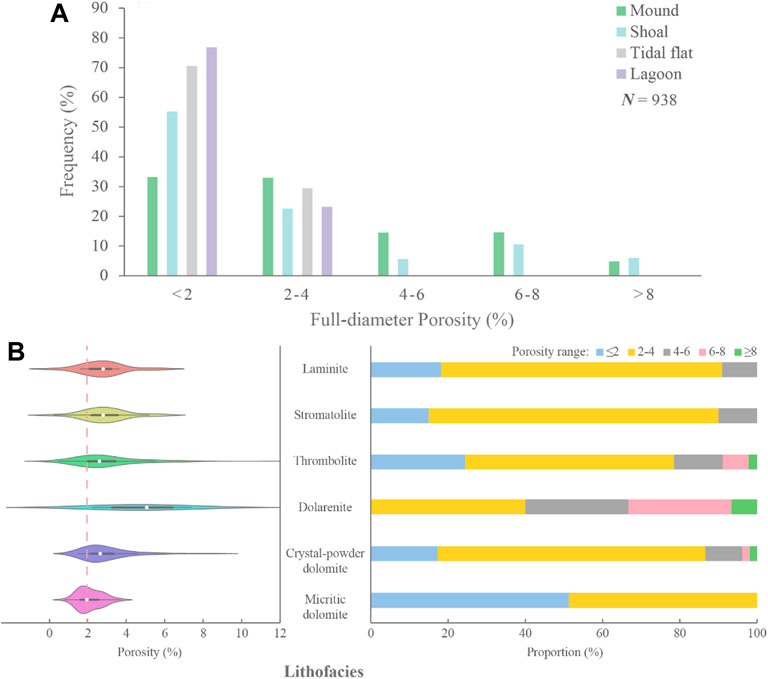
FIGURE 7. (A) porosity distribution histogram of various sedimentary facies (data derived from Hou et al. (2021)); (B) violin plot (left panel) of diverse lithofacies and corresponding proportion of different porosity ranges in the lithofacies (right panel).
To sum up, favorable sedimentary facies, i.e., mounds and shoals in the platform margin, are material foundations for high-quality reservoirs.
5.2 Paleogeomorphology and Karstification
The differences in material foundations and hydraulic gradients in various geomorphologic shapes result in diverse dissolution mechanisms and patterns. These result in differences in karst intensities, products, and donations for reservoir development.
There is a noticeable difference in the slope gradient (Figure 3B) of the top of Sinian in the GMA. The banded highlighted area referring to slope terrain exceeds the deep-blue part representing flat topography. Topographical relief of paleogeomorphology determines karst hydrodynamics to a great extent (Han et al., 2019). The recharge, runoff, and discharge of karst hydrodynamic systems also differ depending on the morphology and hydraulic gradient (White, 1988; White, 2002; Ford and Williams, 2007). In the areas with higher slope angles, including slopes and side slopes of convex and concave terrains, the hydraulic head, flow velocity, and potential energy are all higher and exert drastic mechanical erosion on the surface. Such rapid and frequent exchange of fluids caused by high hydraulic gradients guarantees less etching time for surface corrosion. Hence, there is greater internal dissolution resulting from calcium carbonate unsaturation in karst fluids that percolate through matrix pores and fractures. This produces honeycomb-like caves (Figure 8A), large grikes (Figure 8B), and caves developing from laminae in microbial dolomites (Figure 8C), ultimately causing solution collapse in dolomite breccias. The dissolution is so strong in slope terrains that the first-stage fibrous cement that precipitates from seawater is rarely well preserved. Moreover, the framework vugs of microbial rocks and other dissolution pores are cemented with bladed dolomites in the shallow burial stage and further filled by solid bitumen residue in the subsequent hydrocarbon charging process (Figure 8D, E) although there also exist “clean” dissolution voids without bitumen (Figure 8F).
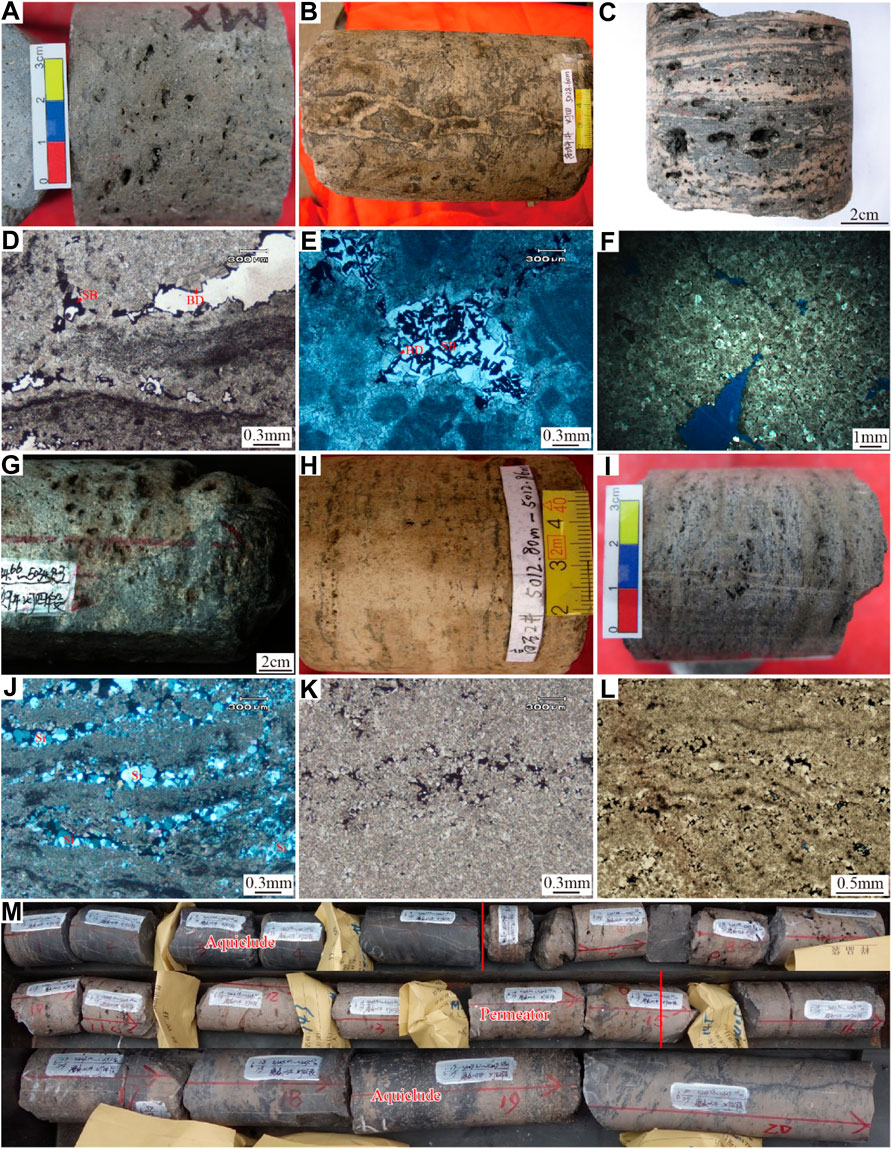
FIGURE 8. Macro and microscopic karst features of slope terrain and flat topography. (A) honeycomb-like vugs, borewell M109, 5,110.17 m; (B) vadose grike hatched by breccias, mud, and dolomites, borewell GK1, 5,028.35 m; (C) stromatolithic dolomite, horizontally elongated vugs distributed along laminae, borewell GK1, 5,150.58 m; (D) stromatolite, framework vugs half-filled by bladed dolomite and solid bitumen, borewell GK1, 5,149.58 m, plane-polarized light; (E) microbial micritic boundstone, solution pore half-hatched by bladed dolomite and solid bitumen, borewell GK1, 5,145.1 m, plane-polarized light; (F) granular dolostone, solution pore nearly unfilled, borewell M109, 5,107.43 m, plane-polarised light; (G) locally concentrated honeycomb-like vugs and pores, borewell M9, 5,034.66–5,034.83 m; (H) solution pinholes distributed along laminae, borewell G2, 5,012.8–5,012.86 m; (I) laminated dolomite, solution pinholes arranged along laminae, borewell G18, 5,134.2 m; (J) stromatolite, laminated framework vugs filled by silica, borewell AP1, 5062 m, cross-polarized light; (K) coarse-powder crystalline dolostone, horizontally distributed solution pinholes filled by fine crystalline dolomite and solid bitumen, borewell AP1, 5,053 m, plane-polarized light; (L) stromatolite, inter-laminae solution pores filled by fine-crystalline dolomite and solid bitumen, borewell G2, 5,013.99 m, plane-polarized light; (M) vertical alternation of aquiclude and permeator, manifesting control of sedimentary facies on karstification, borewell M105, 5,347.27–5,349.74 m (this photograph is cited from a Sinopec PPT).
The flat topography is the result of the terminal stage of the last erosional cycle. In the cycles, land raised above sea level would be eroded to the erosion base level (generally, sea level) and show less-undulated relief (Ford and Williams, 2007). On flat topography, runoff moves horizontally and laterally, and the relatively low average hydraulic gradients lead to a low hydraulic head difference, weak potential, slow velocity, and inadequate water incision. Hence, flat areas show inferior karstification compared to slope terrains. In the “inland” area, far from the west main discharge area (Figure 3A), part of the recharge that does not include runoff percolates and infiltrates downward via less-developed, smaller matrix pores and fractures as groundwater, forming locally concentrated vugs (Figure 8G) and pint-sized solution pores (Figure 8H). Laminae-arranged and bedding-parallel dissolution voids (Figure 8I) are rather common in microbial laminae of the platform interior due to modest downward erosion. The first-stage isopachous cement in prime intergranular pores are almost absent after prolonged karstification and other alterations, and voids that are sufficiently interconnected to hatch by silica (Figure 8J) and solid bitumen (Figures 8K,L).
The Z2dn4 in the GMA is mainly modified by eogenetic karstification prior to a complete deep burial stage. The Z2dn4 in the GMA had never been uplifted to subaerial conditions to undergo epigenetic karstification following the deep burial stage, and episode Ⅱ of the Tongwan Movement came up immediately followed by a shallow burial stage (Zhou et al., 2020). Large-scale exposure of the Z2dn4 occurred only in the eogenetic stage. Thus, the difference in materials affected the karst result greatly (Loucks, 1999; Vacher and Mylroie, 2002; Tan et al., 2015). Namely, microbial framestones and coarse-grain rocks like granular rocks possess stronger abilities to resist shallow burial compaction compared to fine-grain ones, resulting in lithofacies-controlled karstification (Jin et al., 2020). Therefore, the platform margin was occupied by a larger proportion of microbial build-ups and sand shoals that retained far more pore spaces than the platform interior. This provided preferential conduits for karst fluids and, hence, displayed more developed karst porosities, vugs, and cavities.
Furthermore, frequent sea-level eustacy brought about multi-stage exposures and synsedimentary karst from atmospheric freshwater occurred in local highs of the platform margin. During dramatic regression, i.e., episode Ⅱ of the Tongwan Movement, extensive exposure occurred and strata were eroded to even the upper Z2dn2 in the Mianyang-Changning intracratonic sag (Liu et al., 2016). Eogenetic karstification of the Z2dn4 in the GMA exhibits the peculiarities of coastal karstification (Jin et al., 2017; Jin et al., 2020). Due to the dissolution of seawater and meteoric freshwater, massive caverns tended to flank marginal caves and a mass of vugs appeared in the seawater-affected belt (Moore and Wade, 2013; Tan et al., 2015). The seawater-affected belt overlapping with favorable microbial and granular lithofacies led to the horizontal difference in reservoir development between the platform margin and interior. Additionally, due to the impact of constant sea-level eustacy, reservoirs derived from mixing karstification in the platform margin were distributed evenly in the whole member and were vertically separated by tight aquicludes (Figure 8M). This demonstrates peculiarities of sedimentary control (Figure 8M) that outmatch those in the platform interior in both quantity and quality, mostly scattered in the vadose and phreatic freshwater zones of the upper and middle Z2dn4 (Figure 9A). Statistical analysis of the vugs in the platform margin prominently surpassed those in the platform interior in both number and diameter (Tian et al., 2020) (Figure 9B).
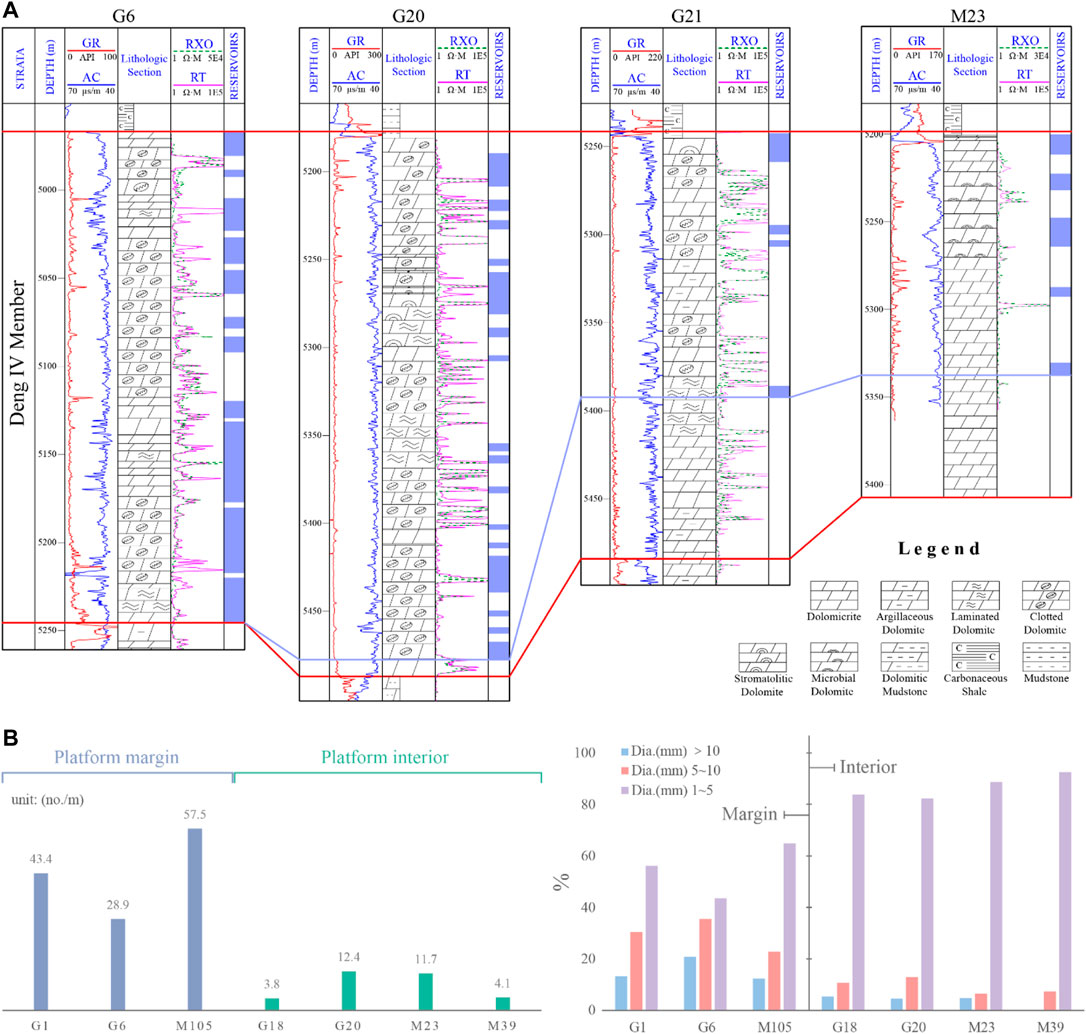
FIGURE 9. (A) multi-borewell correlation of reservoirs of Z2dn4. The reservoirs and non-reservoirs are compartmentalized by 2% porosity and 0.002 mD permeability. Note that the platform margin with more protected lithofacies greatly influenced karstification. Vertical differences in the distribution and development of reservoirs may relate to karst differentiation in pattern and depth. The violet-blue polyline implies the possible lower bound of karstification. For section location, see Figure 6A. (B) statistic differences in number and diameter of vugs between the platform margin and interior (data cited from Tian et al. (2020)).
5.3 Hydrocarbon Charging
Notwithstanding the advantageous conditions for hydrocarbon accumulation, exploration practices illustrate notable differences between the platform margin and interior, that is, reservoirs are more developed in scale and quality in the platform margin than the platform interior, and the tested gas productions also vary vastly (Tian et al., 2020). This is a further reminder of the strong heterogeneity in reservoir distribution that may be the result of multi-factor functions. Aside from the differences in sedimentary facies, paleogeomorphology, and karst alteration expounded earlier, the hydrocarbon charging process may be another vital function for reservoir differences between the platform margin and intraplatform regions.
The natural gas in the Z2dn in the GMA is derived from multiple sources (Wei et al., 2015; Zheng D. et al., 2021). Analyses for hydrocarbon inclusions formed during hydrocarbon expulsion of the Z2dn in the GMA suggested a U-Pb isotopic age of 416 ± 23 Ma and a clumped isotopic temperature of 84–120°C. As the burial depth of the Є1q was 1 km shallower than that of the Z2dn, the calibrated hydrocarbon expulsion temperature may have been constrained by the burial depth of the Є1q and ranged from 70 to 100°C, exceeding the hydrocarbon-generating threshold (Shen et al., 2021). Adjacent to the GMA, the Ziyang-Weiyuan area (ZWA) went through two major hydrocarbon charging processes. Re–Os hydrocarbon dating showed two isochron ages for bitumen and 78 ± 37 Ma for pyrobitumen, which indicate the generating and thermal cracking timings of liquid hydrocarbons, respectively (Shi et al., 2020a). The hydrocarbon expulsion time of the source rocks was 414 ± 44 Ma, denoting the late Silurian which is consistent with the results for the Є1q. Furthermore, the Mn/Fe ratios of bitumen in the Z2dn and Є1q are most related (Shi et al., 2020b). Biomarker chromatograms for the reservoir bitumen and the source rocks from Є1q and Z2dn revealed comparable sterane distributions, implying that the reservoir bitumen of the Z2dn is composed of mixed sources derived from the Є1q and the Z2dn (Zheng D. et al., 2021).
With respect to the Z2dn4, source rocks from the lower Sinian Doushantuo Formation, the Є1q of the lower Cambrian, and the Z2dn3 supply most of the hydrocarbon, with the small remainder being produced by the muddy dolomites of Z2dn4 itself (Yang et al., 2020). The major source of hydrocarbon for Z2dn4 is mud-shale in the Є1q, which is distinguished by a high TOC content ranging from 0.83% to 4.28% (Wei et al., 2017), high maturity with Ro values between 1.5% and 5.7% (Zou et al., 2010), and a large thickness that is favorable for large-scale hydrocarbon generation and effective sealing (Li et al., 2018; Yang et al., 2020). Moreover, due to the Mianyang-Changning intracratonic sag lying to the west of the GMA, the deposited thickness of the Є1q was much thicker compared to the crystallizing regional hydrocarbon-generating center right above the GMA (Figure 9B).
The GMA displays excellent spatial dispositions amongst source rocks and reservoirs. The Є1q source rock, together with very thin Є1m, clings to the flank and top of the Z2dn4, ideating patterns of lateral migration and downward charging for hydrocarbons. The two spatial disposition patterns display direct contact between source rocks and convex mound-shoal reservoirs from two sides, which is beneficial to hydrocarbon accumulation in mound-shoal complexes of the platform margin (Yang et al., 2020). In addition, source rocks from the underlying Z2dn3 and Doushantuo Formation present another pattern of upward charging (Figure 10). Thus, the Z2dn4 in the GMA is surrounded by source rocks from three sides: the west flank, top, and bottom and exhibits vast exploration potential.
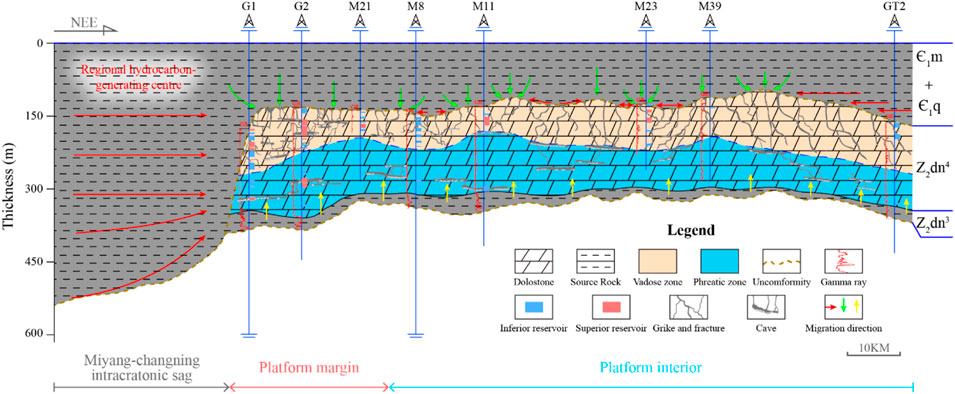
FIGURE 10. Sketched map of hydrocarbon migration of the Z2dn4 in the GMA. For the section location, see Figure 11. Note that the inferior and superior reservoirs are compartmentalized by 5% porosity and 0.25 mD permeability.
The three charging patterns mentioned earlier intrinsically lead to different degrees of reservoir accumulation. First, the potential for hydrocarbon generation of source rocks differs tremendously; the lower Cambrian Є1q is the major donor for natural gas of the Z2dn (Zheng D. et al., 2021). Second, the thickest part of the Є1q (also called the hydrocarbon-generating center) lies in the Mianyang-Changning intracratonic sag that is adjacent to the GMA on the west and constitutes a lateral migrating assemblage with reservoirs of Z2dn4. It is intuitively plausible that hydrocarbons expulsed in the regional generation center tend to laterally transfer and preferentially accumulate in mound-shoal complexes of the platform margin that are nearest to the intracratonic sag. Moreover, this postulated insight is confirmed by the solid bitumen distribution.
Solid bitumen exists prevailingly in reservoir spaces in the Z2dn in the GMA (Gao et al., 2018). Its occurrence in the matrix porosities demonstrates direct evidence for the existence of paleo-reservoirs (Wang Z. Y. et al., 2020; Liu et al., 2022), while its content and distribution can indicate the dominant migration path of hydrocarbons (Mastalerz et al., 2018). The contents of solid bitumen in single boreholes of Z2dn4 in the GMA were evaluated quantitatively by Song et al. (2021) via a multi-mineral volumetric inversion pattern established by five conventional logging curves (viz. AC, CNL, DEN, RT, and RXO) and data from cores and thin sections. The plane distribution of bitumen contents in boreholes reveals a high bitumen content in the platform margin that tends to gradually decrease from west to east (Figure 11). This implies a predominant role of lateral migration and the charging of hydrocarbons from west to east (with a non-negligible contribution from the nearly transmeridional fault system). This may be because the capacity for lateral migration reduces with increasing distance away from the regional hydrocarbon-generating center, i.e., the intracratonic sag. Lower matrix porosity in intraplatform might also block the pathway of hydrocarbon transfer. This thus accounts for the differences in hydrocarbon entrapment between the platform margin and intraplatform region.
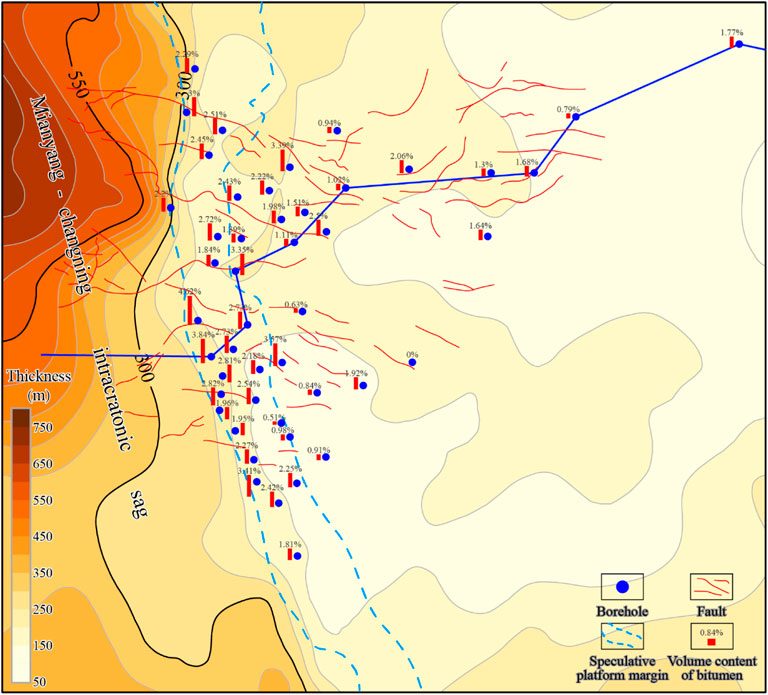
FIGURE 11. Combined source rock thickness of the lower Cambrian Є1m and Є1q. Single-borewell volume content of solid bitumen and faults, of the Z2dn4, are added to the thickness map. The volume contents of bitumen in the boreholes within the speculative platform margin intuitively exceed those in the intraplatform region. The fault system plays an important role in lateral hydrocarbon migration. The volume content of solid bitumen is cited from Song et al. (2021).
With regard to the upward hydrocarbon-charging pattern, the gas sources were derived majorly from mudstones from the underlying Z2dn3 and the lower Sinian Doushantuo Formation. The TOC content of the Z2dn3 ranged from 0.5% to 4.73% and that of the Doushantuo Formation was in the range of 0.56%–14.17% (Wei et al., 2015). These are, therefore, seemingly adequate source rocks. However, both strata are too thin compared to the Є1q as their mean thickness was less than 30 m, thus limiting their potential as source rocks. Hydrocarbons from the two strata have limited effect on the transmeridionally differentiated accumulation framework of the Z2dn4, let alone the prior reception and entrapment of the Z2dn2 for hydrocarbon from the Doushantuo Formation.
Regarding the downward charging process, the key is that the formation pressure in overlying source rocks exceeds the sum of the underlying formation pressure, hydrocarbon buoyancy, and migrating resistance along the faults (Cong et al., 2016; Fu et al., 2017). The trends in the formation pressure of the Є1q showed that the overpressure started with hydrocarbon expulsion in the late Permian and culminated in the middle Jurassic with a maximum pressure coefficient of 2.4, before eventually stabilizing with a pressure coefficient of 2.2 (Liu et al., 2018). The pressure coefficients of the Є1q after hydrocarbon generation clearly exceeded those (1.11–1.59) of the Z2dn4 (Liu et al., 2022). This may be evidence supporting a downward charging pattern; however, the capillary pressure and hydrocarbon buoyancy are not quantitively constrained, and further investigation is required concerning the superimposed effect of the hydrocarbon expelling zone, source rock overpressure area, and hydrocarbon conducting faults in the GMA. In the intraplatform region, hydrocarbons from superjacent Є1q can be entrapped and reserved by local geomorphic highs and form a considerable number of reservoirs. Furthermore, the hydrocarbon potential is higher in the intracratonic sag than right above the GMA due to larger depositional thickness (Yang et al., 2020), which is one of the reasons responsible for the heterogeneous distribution of reservoirs in the GMA.
6 Conclusion
The distribution of carbonate reservoirs is generally known as a comprehensive consequence of multiple factors. Based on paleogeomorphological reconstruction using the elevation method, the slope angles of each borehole were calculated, showing a slope belt surrounding the platform. In the light of FMI data in boreholes, four electrofacies (host rock, vug, fracture, and cave) were identified. The random forest algorithm was employed to identify the four electrofacies in borewells that lacked FMI data, and the result suggested that the vuggy and cave sections of boreholes are thicker in the platform margin than in the platform interior, which is consistent with the postulated greater erosion and karstification in the platform margin. The slope gradients, thicknesses of the four electrofacies in every borehole, and multiple physical property parameters of reservoirs were used for factor analysis. Four rotated factors with a total variance contribution greater than 80% were obtained, and the comprehensive score of each borehole was calculated via the cumulative sum of the four factors according to their respective ratios of variance proportions. This score depicts the favorability and development of reservoirs and shows that the most advantageous reservoirs are distributed in the mound-shoal complexes of the platform margin that is adjacent to the Mianyang-Changning intracratonic sag in the west of the GMA. The development and distribution of reservoirs are predominantly subjected to the following three factors:
(1) Favorable sedimentary facies are the material foundations for high-quality reservoirs. Both the quantity and quality of vugs in mound-shoal complexes of the platform margin are notably superior to those in the intraplatform region of the Z2dn4. Furthermore, the porosities of various sedimentary facies are apparently biased. In the platform margin where the mound-shoal complexes dominate, porosities are higher than in the tidal flats and lagoons that were most common in the inner platform. This indicates differences in the primary deposition that provide substantial bases for later karst alteration and hydrocarbon charging.
(2) In episode Ⅱ of the Tongwan Movement, the Sichuan Basin including the GMA was uplifted above sea level. Massive exposure of the Z2dn4 platform led to extensive karstification. The prolonged karstification differs in the platform margin compared to the intraplatform region due to differences in depositional bases and karst fluids. The mixing karst zone, which involved both seawater and freshwater, is superimposed with the platform margin and slope terrain with higher hydraulic gradients. This resulted in more intensive karstification and more developed vugs and caverns that are conductive to hydrocarbon storage in the platform margin.
(3) Source rocks of the Z2dn4 in the GMA incorporate the lower Cambrian Є1q, the underlying Z2dn3, and the lower Sinian Doushantuo Formation, forming three types of charging processes: the lateral migration pattern and the downward and upward charging patterns. The Є1q together with the very thin Є1m in the Mianyang-Changning intracratonic sag abutting the platform margin of the GMA is the regional hydrocarbon-generating center. This area constitutes a lateral migrating and accumulating assemblage with mound-shoal complexes in the platform margin. The concentrations of bitumen in restored single-borehole solid bitumen contents were gradually decreased with increasing distance from the sag, implying that lateral migration played a dominant role. Moreover, the bulk lateral migrating hydrocarbons are entrapped in the platform margin, accounting for the heterogeneous distribution of the reservoirs.
Data Availability Statement
The raw data supporting the conclusions of this article will be made available by the authors, without undue reservation.
Author Contributions
ZKZ data curation and writing original draft; XZW, XYY, and LW project administration and supervision; WZW, DMZ, and MYW resources and investigation; FH and SYX reviewing and editing. All authors read and approved the final paper.
Funding
This work was financially supported by the National Science and Technology Major Project (grant number 2016ZX05004-005) and the Major Science and Technology Project of PetroChina Co., Ltd. (grant number 2016E-0602).
Conflict of Interest
Authors LW and WW were employed by PetroChina Southwest Oil and Gas Field Company.
The remaining authors declare that the research was conducted in the absence of any commercial or financial relationships that could be construed as a potential conflict of interest.
Publisher’s Note
All claims expressed in this article are solely those of the authors and do not necessarily represent those of their affiliated organizations, or those of the publisher, the editors, and the reviewers. Any product that may be evaluated in this article, or claim that may be made by its manufacturer, is not guaranteed or endorsed by the publisher.
Acknowledgments
The authors thank the Exploration and Development Research Institute of PetroChina Southwest Oil and Gas Field for their support, plentiful data, and permission to use and publish the data. Special thanks are extended to Yang Li for his enlightening and insightful suggestions and help, which have made this article better.
References
Abarca, E., Idiart, A., Grandia, F., Rodríguez-Morillas, N., Pellan, C., Zen, M., et al. (2019). 3D Reactive Transport Modeling of Porosity Evolution in a Carbonate Reservoir through Dolomitization. Chem. Geol. 513, 184–199. doi:10.1016/j.chemgeo.2019.03.017
Al-Rbeawi, S., and Kadhim, F. (2017). The Impact of Hydraulic Flow Unit & Reservoir Quality Index on Pressure Profile and Productivity Index in Multi-Segments Reservoirs. Petroleum 3, 414–430. doi:10.1016/j.petlm.2017.05.004
Bai, J., Li, Y., Li, J., Yang, X., Jiang, Y., and Xia, S.-T. (2022). Multinomial Random Forest. Pattern Recognit. 122, 108331. doi:10.1016/j.patcog.2021.108331
Biteau, J.-J., Le Marrec, A., Le Vot, M., and Masset, J.-M. (2006). The Aquitaine Basin. Pet. Geosci. 12 (3), 247–273. doi:10.1144/1354-079305-674
Breiman, L., Friedman, J., Stone, C., and Olshen, R. A. (1984). Classification and Regression Trees. Belmont, California: Wadsworth International Group.
Burchette, T. P. (1996). Unconformities and Porosity in Carbonate Strata. Mar. Pet. Geol. 13 (5), 596–597. doi:10.1016/0264-8172(96)88336-x
Calner, M., Lehnert, O., and Nõlvak, J. (2010). Palaeokarst Evidence for Widespread Regression and Subaerial Exposure in the Middle Katian (Upper Ordovician) of Baltoscandia: Significance for Global Climate. Palaeogeogr. Palaeoclimatol. Palaeoecol. 296, 235–247. doi:10.1016/j.palaeo.2009.11.028
Che, Z., Tan, X., Deng, J., and Jin, M. (2019). The Characteristics and Controlling Factors of Facies-Controlled Coastal Eogenetic Karst: Insights from the Fourth Member of Neoproterozoic Dengying Formation, Central Sichuan Basin, China. Carbonates Evaporites 34 (4), 1771–1783. doi:10.1007/s13146-019-00524-0
Chen, L., Zhang, H., Cai, Z., Hao, F., Xue, Y., and Zhao, W. (2022). Petrographic, Mineralogical and Geochemical Constraints on the Fluid Origin and Multistage Karstification of the Middle-Lower Ordovician Carbonate Reservoir, NW Tarim Basin, China. J. Pet. Sci. Eng. 208 (C), 109561. doi:10.1016/j.petrol.2021.109561
Cong, L., Zhao, T. Q., Liu, Y., Hu, X. L., and Wang, W. F. (2016). Conditions of Oil-Gas Downward Migration in Vertical and Lateral and Their Differences in Accumulation Laws. J. China Univ. Min. Technol. 45 (05), 951–957. doi:10.13247/j.cnki.jcumt.000494 (in Chinese with English Abstract).
Dai, X., Du, B., Zhang, M., Li, J., Tang, T., Xu, Y., et al. (2020). Reunderstanding and Significance of High-Quality Reservoirs of the Inner Dengying Formation in the Anyue Gas Field. Nat. Gas. Ind. B 7 (2), 109–119. doi:10.1016/j.ngib.2020.03.001
Dong, Y. H., Duan, R. Q., Li, Z., Lü, X. X., Cai, C. F., Liu, J. Q., et al. (2020). Quantitative Evaluation of Hydrothermal Fluids and Their Impact on Diagenesis of Deep Carbonate Reservoirs: Insights from Geochemical Modeling. Mar. Pet. Geol. 124, 104797. doi:10.1016/j.marpetgeo.2020.104797
Duan, J. B., Dai, L. C., Li, B. S., Zhu, X., and Li, H. (2019). Reservoir Characteristics and Their Controlling Factors of the Fourth Member of Upper Sinian Dengying Fm in the Northern Sichuan Basin. Nat. Gas. Ind. 39 (07), 9–20. doi:10.3787/j.issn.1000-0976.2019.07.002 (in Chinese with English Abstract).
Dyman, T. S., Crovelli, R. A., Bartberger, C. E., and Takahashi, K. I. (2002). Worldwide Estimates of Deep Natural Gas Resources Based on the U.S. Geological Survey World Petroleum Assessment 2000. Nat. Resour. Res. 11 (3), 207–218. doi:10.1023/a:1019860722244
Fritz, R. D., Wilson, J. L., and Yurewicz, D. A. (1993). Paleokarst Related Hydrocarbon Reservoirs. New Orleans: SEMP Core Workshop.
Fu, G., Li, S. Z., and Yang, D. X. (2017). A Method Forecasting Distribution Areas of Fault Transporting Oil-Gas Migration and its Application. Acta Sedimentol. Sin. 35 (03), 592–599. doi:10.14027/j.cnki.cjxb.2017.03.016 (in Chinese with English Abstract).
Gao, P., Liu, G., Lash, G. G., Li, B., Yan, D., and Chen, C. (2018). Occurrences and Origin of Reservoir Solid Bitumen in Sinian Dengying Formation Dolomites of the Sichuan Basin, SW China. Int. J. Coal Geol. 200, 135–152. doi:10.1016/j.coal.2018.11.001
Gomez-Rivas, E., Corbella, M., Martín-Martín, J. D., Stafford, S. L., Teixell, A., Bons, P. D., et al. (2014). Reactivity of Dolomitizing Fluids and Mg Source Evaluation of Fault-Controlled Dolomitization at the Benicàssim Outcrop Analogue (Maestrat Basin, E Spain). Mar. Pet. Geol. 55, 26–42. doi:10.1016/j.marpetgeo.2013.12.015
Halbouty, M. T. (2003). Giant Oil and Gas Fields of the Decade 1990–1999, 78. Tulsa: AAPG Memoir, 1–340.
Han, C., Lin, C., Wei, T., Dong, C., Ren, L., Zhang, X., et al. (2019). Paleogeomorphology Restoration and the Controlling Effects of Paleogeomorphology on Karst Reservoirs: a Case Study of an Ordovician-Aged Section in Tahe Oilfield, Tarim Basin, China. Carbonates Evaporites 34 (1), 31–44. doi:10.1007/s13146-017-0382-1
Hao, Y., Yang, X., Wang, Y. F., Chen, W., Gu, M. F., and Hou, G. F. (2017). Supergene Karstification in the Sinian Dengying Formation,Sichuan Basin. Sediment. Geol. Tethyan Geol. 37 (01), 48–54. doi:10.3969/j.issn.1009-3850.2017.01.007 (in Chinese with English Abstract).
Hou, L. H., Yang, F., Yang, C., and Wang, J. H. (2021). Characteristics and Formation of Simian (Ediacaran)carbonate Karstic Reservoirs in Dengying Formation in Sichuan Basin,China. Pet. Res. 6 (2), 14. doi:10.1016/j.ptlrs.2020.11.003
Jia, A., Wei, Y., and Jin, Y. (2016). Progress in Key Technologies for Evaluating Marine Shale Gas Development in China. Pet. Explor. Dev. 43 (6), 1035–1042. doi:10.1016/s1876-3804(16)30120-3
Jiang, P., Zhang, H., Wang, L., Wang, X. G., and Xiang, Y. H. (2021). An Evaluation Method of Reservoir “Sweet Spots” in Low Permeability Oilfields of Beibuwan Basin. Acta Pet. Sin. 42 (06), 751–764. (in Chinese with English abstract). doi:10.7623/syxb202106005
Jin, M., Li, B., Zhu, X., Dai, L., Jiang, Z., Wu, H., et al. (2020). Characteristics and Main Controlling Factors of Reservoirs in the Fourth Member of Sinian Dengying Formation in Yuanba and its Peripheral Area, Northeastern Sichuan Basin, SW China. Pet. Explor. Dev. 47 (6), 1172–1182. doi:10.1016/s1876-3804(20)60127-1
Jin, M., Tan, X., Tong, M., Zeng, W., Liu, H., Zhong, B., et al. (2017). Karst Paleogeomorphology of the Fourth Member of Sinian Dengying Formation in Gaoshiti-Moxi Area, Sichuan Basin, SW China: Restoration and Geological Significance. Pet. Explor. Dev. 44 (1), 58–68. doi:10.1016/s1876-3804(17)30008-3
Kaiser, H. F. (1958). The Varimax Criterion for Analytic Rotation in Factor Analysis. Psychometrika 23 (3), 187–200. doi:10.1007/bf02289233
Kayastha, P., Dhital, M. R., and De Smedt, F. (2013). Application of the Analytical Hierarchy Process (AHP) for Landslide Susceptibility Mapping: A Case Study from the Tinau Watershed, West Nepal. Comput. Geosci. 52 (MAR.), 398–408. doi:10.1016/j.cageo.2012.11.003
Lawley, D. N., and Maxwell, A. E. (1962). Factor Analysis as a Statistical Method. J. R. Stat. Soc. 12 (3), 209–229. doi:10.2307/2986915
Li, A. P., Gao, D., Hu, M. Y., Zhao, Y. R., Zhu, C. Y., and Dai, Y. C. (2021c). Deposition Model and Main Factors Controlling Depositional Processes for Microbialites in the Fourth Member, Dengying Formation, Central Sichuan Basin. Acta Sedimentol. Sin. doi:10.14027/j.issn.1000-0550.2021.156 (in Chinese with English Abstract).
Li, R., Chen, Q., Deng, H., Fu, M., Hu, L., Xie, X., et al. (2021a). Quantitative Evaluation of the Carbonate Reservoir Heterogeneity Based on Production Dynamic Data: A Case Study from Cretaceous Mishrif Formation in Halfaya Oilfield, Iraq. J. Petroleum Sci. Eng. 206, 109007. doi:10.1016/j.petrol.2021.109007
Li, W.-P., Zheng, Y.-F., and Zhao, Y.-Y. (2017). Geochemical Evidence from Marine Carbonate for Enhanced Terrigenous Input into Seawater during the Ediacaran-Cambrian Transition in South China. Precambrian Res. 291, 83–97. doi:10.1016/j.precamres.2017.01.015
Li, X., Jiang, Z., Wang, P., Song, Y., Li, Z., Tang, X., et al. (2018). Porosity-preserving Mechanisms of Marine Shale in Lower Cambrian of Sichuan Basin, South China. J. Nat. Gas. Sci. Eng. 55, 191–205. doi:10.1016/j.jngse.2018.05.002
Li, Y., Xue, Z. J., Cheng, Z., Jiang, H. J., and Wang, R. Y. (2020). Progress and Development Directions of Deep Oil and Gas Exploration and Development in China. China Pet. Explor. 25 (01), 45–57. (in Chinese with English Abstract). doi:10.3969/j.issn.1672-7703.2020.01.005
Li, Y., Wang, X., Feng, M., Zeng, D., Xie, S., Fan, R., et al. (2019). Reservoir Characteristics and Genetic Differences between the Second and Fourth Members of Sinian Dengying Formation in Northern Sichuan Basin and its Surrounding Areas. Pet. Explor. Dev. 46 (1), 54–66. doi:10.1016/s1876-3804(19)30005-9
Li, Y., Zhang, T., Dai, Z., Huang, L., Xu, Y., Wang, X., et al. (2021b). Quantitative Evaluation Methods of Tight Reservoirs Based on Multi-Feature Fusion: A Case Study of the Fourth Member of Shahejie Formation in Liaohe Depression. J. Petroleum Sci. Eng. 198, 108090. doi:10.1016/j.petrol.2020.108090
Li, Z. Q., Liu, J., Li, Y., Hang, W. Y., Hong, H. T., Ying, D. L., et al. (2015). Formation and Evolution of Weiyuan-Anyue Extension-Erosion Groove in Sinian System, Sichuan Basin. Pet. Explor. Dev. 42 (1), 26–33. doi:10.1016/s1876-3804(15)60003-9
Liu, H., Luo, S., Tan, X., Li, L., Lian, C., Zeng, W., et al. (2015). Restoration of Paleokarst Geomorphology of Sinian Dengying Formation in Sichuan Basin and its Significance, SW China. Pet. Explor. Dev. 42 (3), 311–322. doi:10.1016/s1876-3804(15)30021-5
Liu, S. G., Wang, Y. S., Sun, W., Zhong, Y., Hong, H. T., Deng, B., et al. (2016). Control of Intracratonic Sags on the Hydrocarbon Accumulations in the Marine Strata across the Sichuan Basin, China. J. Chengdu Univ. Technol. 43 (01), 1–23. (in Chinese with English Abstract). doi:10.3969/j.issn.1671-9727.2016.01.01
Liu, S., Li, Z., Deng, B., Sun, W., Li, Z., Ding, Y., et al. (2022). Occurrence Morphology of Bitumen in Dengying Formation Deep and Ultra-Deep Carbonate Reservoirs of the Sichuan Basin and its Indicating Significance to Oil and Gas Reservoirs. Nat. Gas. Ind. B 9, 73–83. doi:10.1016/j.ngib.2022.01.001
Liu, W., Qiu, N. S., Xu, Q. C., and Chang, J. (2018). The Quantitative Evaluation of the Pressurization Caused by Hydrocarbon Generation in the Cambrian Qiongzhusi Formation of the Gaoshiti-Moxi Area, Sichuan Basin. Pet. Sci. Bull. 3 (03), 262–271. (in Chinese with English Abstract).
Loucks, R. G., and Anderson, J. H. (1985). Depositional Facies, Diagenetic Terranes, and Porosity Development in Lower Ordovician Ellenburger Dolomite, Puckett Field, West Texas. New York: Springer.
Loucks, R. G. (1999). Origin and Attributes of Paleocave Carbonate Reservoirs(in Karst Modeling; Proceedings of the Symposium). Karst Waters Institute Special Publication 5, 59–64.
Luo, B., Luo, W. J., Wang, W. Z., Wang, Z. H., and Shan, S. J. (2015). Formation Mechanism of the Sinian Natural Gas Reservoir in the Leshan-Longnvsi Paleouplift, Sichuan Basin. Nat. Gas Geosci. 26, 444–455.
Luo, B., Yang, Y., Luo, W., Wen, L., Wang, W., and Chen, K. (2017). Controlling Factors of Dengying Formation Reservoirs in the Central Sichuan Paleo-Uplift. Pet. Res. 2 (1), 54–63. doi:10.1016/j.ptlrs.2017.06.001
Ma, Y. S., Li, M. W., Cai, X. Y., Xu, X. H., Hu, D. F., Qu, S. L., et al. (2020). Mechanisms and Exploitation of Deep Marine Petroleum Accumulations in China: Advances, Technological Bottlenecks and Basic Scientific Problems. Oil Gas. Geol. 41, 655–683. (in Chinese with English abstract). doi:10.11743/ogg20200401
Mastalerz, M., Drobniak, A., and Stankiewicz, A. B. (2018). Origin, Properties, and Implications of Solid Bitumen in Source-Rock Reservoirs: A Review. Int. J. Coal Geol. 195, 14–36. doi:10.1016/j.coal.2018.05.013
Moore, C. H., and Wade, W. J. (2013). “Carbonate Reservoirs — Porosity and Diagenesis in a Sequence Stratigraphic Framework,” in Developments in Sedimentology. Amsterdam: Academic Press, Elsevier, 67, 1–374.
Morad, D., Nader, F. H., Gasparrini, M., Morad, S., Rossi, C., Marchionda, E., et al. (2018). Comparison of the Diagenetic and Reservoir Quality Evolution between the Anticline Crest and Flank of an Upper Jurassic Carbonate Gas Reservoir, Abu Dhabi, United Arab Emirates. Sediment. Geol. 367, 96–113. doi:10.1016/j.sedgeo.2018.02.008
Perrin, C., Marquez, X., Flores, J., and Berthereau, G. (2020). Porosity Depth Saturation (PDS) Model: Quantification of Porosity Preservation with Burial in Carbonate Oil Reservoirs, and Application to Infer Oil Charging Time. Mar. Pet. Geol. 120, 104515. doi:10.1016/j.marpetgeo.2020.104515
Purdy, E. G., and Winterer, E. L. (2001). Origin of Atoll Lagoons. Gsa Bull. 113 (7), 837–854. doi:10.1130/0016-7606(2001)113<0837:ooal>2.0.co;2
Quinlan, J. R. (1992). C4.5: Programs for Machine Learning. San Francisco: Morgan Kaufmann Publishers Inc.
Reyes, O., Morell, C., and Ventura, S. (2015). Scalable Extensions of the ReliefF Algorithm for Weighting and Selecting Features on the Multi-Label Learning Context. Neurocomputing 161, 168–182. doi:10.1016/j.neucom.2015.02.045
Ronchi, P., Ortenzi, A., Borromeo, O., Claps, M., and Zempolich, W. G. (2010). Depositional Setting and Diagenetic Processes and Their Impact on the Reservoir Quality in the Late Visean-Bashkirian Kashagan Carbonate Platform (Pre-Caspian Basin, Kazakhstan). AAPG (Am. Assoc. Pet. Geol.) Bull. 94, 1313–1348. doi:10.1306/01051009130
Sayago, J., Di Lucia, M., Mutti, M., Cotti, A., Sitta, A., Broberg, K., et al. (2012). Characterization of a Deeply Buried Paleokarst Terrain in the Loppa High Using Core Data and Multiattribute Seismic Facies Classification. AAPG Bull. 96 (10), 1843–1866. doi:10.1306/02271211137
Schlager, W. (2005). Carbonate Sedimentology and Sequence Stratigraphy. Tulsa: SEPM Concepts in Sedimentology and Paleontology.
Shan, X., Zhang, J., Zhang, B., Liu, J., Zhou, H., Wang, Y., et al. (2017). Characteristics of Dolomite Karstic Reservoir in the Sinian Dengying Formation, Sichuan Basin. Pet. Res. 2 (1), 13–24. doi:10.1016/j.ptlrs.2017.06.003
Shen, A. J., Zhao, W. Z., Hu, A. P., Wang, H., Liang, F., and Wang, Y. S. (2021). The Dating and Temperature Measurement Technologies for Carbonate Minerals and Their Application in Hydrocarbon Accumulation Research in the Paleouplift in Central Sichuan Basin, SW China. Pet. Explor. Dev. 48 (03), 555–568. doi:10.1016/s1876-3804(21)60045-9
Shi, C., Cao, J., Luo, B., Hu, W., Tan, X., and Tian, X. (2020b). Major Elements Trace Hydrocarbon Sources in Over-mature Petroleum Systems: Insights from the Sinian Sichuan Basin, China. Precambrian Res. 343, 105726. doi:10.1016/j.precamres.2020.105726
Shi, C., Cao, J., Selby, D., Tan, X., Luo, B., and Hu, W. (2020a). Hydrocarbon Evolution of the Over-Mature Sinian Dengying Reservoir of the Neoproterozoic Sichuan Basin, China: Insights from Re-Os Geochronology. Mar. Pet. Geol. 122, 104726. doi:10.1016/j.marpetgeo.2020.104726
Song, Z. Z., Liu, G. D., Luo, B., Zeng, Q. C., Tian, X. W., Dai, X., et al. (2021). Logging Evaluation of Solid Bitumen in Tight Carbonate in Deepburied and Ultra-deep-buried Strata of the Central Sichuan Basin. Acta Sedimentol. Sin. 39 (01), 197–211. doi:10.14027/j.issn.1000-0550.2020.127 (in Chinese with English Abstract).
Su, J., Wang, X., Yang, H., Yu, F., Li, Y., Ma, S., et al. (2021). Hydrothermal Alteration and Hydrocarbon Accumulations in Ultra-deep Carbonate Reservoirs along a Strike-Slip Fault System, Tarim Basin, NW China. J. Pet. Sci. Eng. 203 (10), 108605. doi:10.1016/j.petrol.2021.108605
Szabó, N. P. (2011). Shale Volume Estimation Based on the Factor Analysis of Well-Logging Data. Acta Geophys. 59 (5), 935–953. doi:10.2478/s11600-011-0034-0
Tan, X. C., Xiao, D., Chen, J. S., Li, L., and Liu, H. (2015). New Advance and Enlightenment of Eogenetic Karstification. J. Paleogeogr. 17 (04), 441–456. (in Chinese with English Abstract).
Tang, J. G., Hu, W. S., Li, W., and Zhang, G. Y. (2013). Prediction of Weathering Paleokarst Reservoirs by Combining Paleokarst Landform with Unconformity: A Case Study of Sinian Dengying Formation in Leshan−Longnvsi Paleo-Uplift, Sichuan Basin. Pet. Explor. Dev. 40 (06), 674–681. doi:10.1016/s1876-3804(13)60097-x
Tian, F., Luo, X., and Zhang, W. (2019). Integrated Geological-Geophysical Characterizations of Deeply Buried Fractured-Vuggy Carbonate Reservoirs in Ordovician Strata, Tarim Basin. Mar. Pet. Geol. 99, 292–309. doi:10.1016/j.marpetgeo.2018.10.028
Tian, X. W., Peng, H. L., Wang, Y. L., Yang, D. L., Sun, Y. T., Zhang, X. H., et al. (2020). Analysis of Reservoir Difference and Controlling Factors between the Platform Margin and the Inner Area of the Fourth Member of Sinian Dengying Formation in Anyue Gas Field,central Sichuan. Nat. Gas. Geosci. 31 (09), 1225–1238. doi:10.11764/j.issn.1672-1926.2020.04.007 (in Chinese with English Abstract).
Vacher, H. L., and Mylroie, J. E. (2002). Eogenetic Karst from the Perspective of an Equivalent Porous Medium. Carbonates Evaporites 17 (2), 182–196. doi:10.1007/bf03176484
Wang, J., He, Z., Zhu, D., Liu, Q., Ding, Q., Li, S., et al. (2020a). Petrological and Geochemical Characteristics of the Botryoidal Dolomite of Dengying Formation in the Yangtze Craton, South China: Constraints on Terminal Ediacaran "Dolomite Seas". Sediment. Geol. 406, 105722. doi:10.1016/j.sedgeo.2020.105722
Wang, Y., and Lu, Y. (2021). Diagenetic Facies Prediction Using a LDA-Assisted SSOM Method for the Eocene Beach-Bar Sandstones of Dongying Depression, East China. J. Pet. Sci. Eng. 196, 108040. doi:10.1016/j.petrol.2020.108040
Wang, Z. C., Jiang, H., Wang, T. S., Lu, W. H., Gu, Z. D., Xu, A. N., et al. (2014). Paleogeomorphology Formed during Tongwan Tectonization in Sichuan Basin and its Significance for Hydrocarbon Accumulation. Pet. Explor. Dev. 41 (3), 305–312. doi:10.1016/s1876-3804(14)60038-0
Wang, Z. Y., Ma, C., Gong, D. Y., and Jiang, H. (2020b). The Origin of Bitumen and its Significance for Hydrocarbon Accumulation Process. Acta Geol. Sin. 94 (11), 13. doi:10.19762/j.cnki.dizhixuebao.2020253 (in Chinese with English Abstract).
Wei, G. Q., Wang, Z. H., Li, J., Yang, W., and Xie, Z. Y. (2017). Characteristics of Source Rocks, Resource Potential and Exploration Direction of Sinian and Cambrian in Sicuhan Basin. Nat. Gas. Geosci. 28 (1), 1–13. doi:10.11764/j.issn.1672-1926.2016.11.015
Wei, G. Q., Yang, W., Xie, W. R., Xie, Z. Y., Zeng, F. Y., Mo, W. L., et al. (2016). Formation Conditions, Accumulation Models and Exploration Direction of Large-Scale Gas Fields in Sinian-Cambrian, Sichuan Basin, China. Nat. Gas. Geosci. 26 (05), 785–795. doi:10.1016/j.jnggs.2016.04.002
Wei, G., Xie, Z., Song, J., Yang, W., Wang, Z., Li, J., et al. (2015). Features and Origin of Natural Gas in the Sinian-Cambrian of Central Sichuan Paleo-Uplift, Sichuan Basin, SW China. Pet. Explor. Dev. 42 (6), 768–777. doi:10.1016/s1876-3804(15)30073-2
Wen, L., Wang, W. Z., Zhang, J., and Luo, B. (2017). Classification of Sinian Dengying Formation and Sedimentary Evolution Mechanism of Gaoshiti-Moxi Area in Central Sichuan Basin. Acta Petrol. Sin. 33 (4), 1285–1294.
White, W. B. (1988). Geomorphology and Hydrology of Karst Terrains. New York: Oxford UniversityOxford Press, 464.
White, W. B. (2002). Karst Hydrology: Recent Developments and Open Questions. Eng. Geol. 65 (2), 85–105. doi:10.1016/s0013-7952(01)00116-8
Xie, X., Lu, H., Deng, H., Yang, H., Teng, B., and Li, H. A. (2019). Characterization of Unique Natural Gas Flow in Fracture-Vuggy Carbonate Reservoir: A Case Study on Dengying Carbonate Reservoir in China. J. Pet. Sci. Eng. 182, 106243. doi:10.1016/j.petrol.2019.106243
Yan, H. J., He, D. B., Xu, W. Z., Wang, G. T., Ji, G., Tian, G. Q., et al. (2016). Paleotopography Reconstruction Method and its Controlling Effect on Fluid Distribution: A Case Study of the Gas Reservoir Evaluation Stage in Gaoqiao, Ordos Basin. Acta Pet. Sin. 37 (12), 1483–1494. doi:10.7623/syxb201612004 (in Chinese with English Abstract).
Yan, H. J., Peng, X., Xia, Q. Y., Xu, W., Luo, W. J., Li, X. Y., et al. (2020). Distribution Features of Ancient Karst Landform in the Fourth Member of the Dengying Formation in the Gaoshiti-Moxi Region and its Guiding Significance for Gas Reservoir Development. Acta Pet. Sin. 41 (6), 658–670+752. doi:10.7623/syxb202006002
Yang, P., Liu, K., Liu, J., Yu, S., Yu, B., Hou, M., et al. (2021). Petroleum Charge History of Deeply Buried Carbonate Reservoirs in the Shuntuoguole Low Uplift, Tarim Basin, West China. Mar. Pet. Geol. 128, 105063. doi:10.1016/j.marpetgeo.2021.105063
Yang, W., Wei, G., Xie, W., Jin, H., Zeng, F., Su, N., et al. (2020). Hydrocarbon Accumulation and Exploration Prospect of Mound-Shoal Complexes on the Platform Margin of the Fourth Member of Sinian Dengying Formation in the East of Mianzhu-Changning Intracratonic Rift, Sichuan Basin, SW China. Pet. Explor. Dev. 47 (6), 1262–1274. doi:10.1016/s1876-3804(20)60134-9
Yang, Y., Wen, L., Luo, B., Wang, W., and Shan, S. (2016). Hydrocarbon Accumulation of Sinian Natural Gas Reservoirs, Leshan-Longnüsi Paleohigh, Sichuan Basin, SW China. Pet. Explor. Dev. 43 (2), 197–207. doi:10.1016/s1876-3804(16)30023-4
Yu, T., Liu, H., Liu, B. W., Tang, S., Tang, Y. Z., and Yin, C. (2021). Restoration of Karst Paleogeomorphology and its Significance in Petroleum Geology—Using the Top of the Middle Triassic Leikoupo Formation in the Northwestern Sichuan Basin as an Example. J. Pet. Sci. Eng. 208 (2022), 109638. doi:10.1016/j.petrol.2021.109638
Zhang, G., Lin, C., Ren, L., Li, S., Cui, S., Wang, K., et al. (2022). Seismic Characterization of Deeply Buried Paleocaves Based on Bayesian Deep Learning. J. Nat. Gas Sci. Eng. 97, 104340. doi:10.1016/j.jngse.2021.104340
Zhang, P., Hua, H., and Liu, W. (2014). Isotopic and REE Evidence for the Paleoenvironmental Evolution of the Late Ediacaran Dengying Section, Ningqiang of Shaanxi Province, China. Precambrian Res. 242 (3), 96–111. doi:10.1016/j.precamres.2013.12.011
Zhao, J. Y., Liu, H. F., Shi, Z. W., Xie, S., He, L., Wu, Y., et al. (2018). A New Interpretation Mode for Pre-Carboniferous Paleo-Geomorphology of the Jingbian Gas Field, Ordos Basin. Nat. Gas. Ind. 38 (04), 53–58. (in Chinese with English Abstract). doi:10.3787/j.issn.1000-0976.2018.04.005
Zheng, D., Pang, X., Luo, B., Chen, D., Pang, B., Li, H., et al. (2021b). Geochemical Characteristics, Genetic Types, and Source of Natural Gas in the Sinian Dengying Formation, Sichuan Basin, China. J. Pet. Sci. Eng. 199, 108341. doi:10.1016/j.petrol.2020.108341
Zheng, W., Tian, F., Di, Q., Xin, W., Cheng, F., and Shan, X. (2021a). Electrofacies Classification of Deeply Buried Carbonate Strata Using Machine Learning Methods: A Case Study on Ordovician Paleokarst Reservoirs in Tarim Basin. Mar. Pet. Geol. 123, 104720. doi:10.1016/j.marpetgeo.2020.104720
Zhou, J. G., Zhang, J. Y., Deng, H. Y., Chen, Y. N., Hao, Y., Li, W. Z., et al. (2017). Lithofacies Paleogeography and Sedimentary Model of Sinian Dengying Fm in the Sichuan Basin. Nat. Gas. Ind. B 37 (01), 24–31. doi:10.1016/j.ngib.2017.07.023
Zhou, Y., Yang, F., Ji, Y., Zhou, X., and Zhang, C. (2020). Characteristics and Controlling Factors of Dolomite Karst Reservoirs of the Sinian Dengying Formation, Central Sichuan Basin, Southwestern China. Precambrian Res. 343, 105708. doi:10.1016/j.precamres.2020.105708
Zhou, Z., Wang, X., Yin, G., Yuan, S., and Zeng, S. (2016). Characteristics and Genesis of the (Sinian) Dengying Formation Reservoir in Central Sichuan, China. J. Nat. Gas Sci. Eng. 29, 311–321. doi:10.1016/j.jngse.2015.12.005
Zhu, L., Wang, Z., Zhang, B., Wei, L., Feng, Q., Lei, X., et al. (2019). Characteristics of Paleogeomorphology and Paleokarstification and the Impact on Natural Gas Accumulation: a Case Study of Upper Assemblage of Majiagou Formation in Central Sulige Gas Field, Ordos Basin, China. Carbonates Evaporites 34, 1353–1366. doi:10.1007/s13146-018-0434-1
Zhu, L., Zhou, X., and Zhang, C. (2021). Rapid Identification of High-Quality Marine Shale Gas Reservoirs Based on the Oversampling Method and Random Forest Algorithm. Artif. Intell. Geosci. 2, 76–81. doi:10.1016/j.aiig.2021.12.001
Keywords: reservoir evaluation, paleogeomorphological reconstruction, random forest algorithm, factor analysis, Deng member, Gaoshiti-Moxi area
Citation: Zhou Z, Wang X, Yang X, Wen L, Wang W, Zeng D, Wei M, Xie S and Huo F (2022) Multi-Factor Evaluation of Deep Karst Dolomite Reservoir Based on Paleogeomorphological Reconstruction, a Case Study From the 4th Member of the Dengying Formation in the Central Sichuan Basin, China. Front. Earth Sci. 10:930269. doi: 10.3389/feart.2022.930269
Received: 27 April 2022; Accepted: 17 May 2022;
Published: 30 June 2022.
Edited by:
Chen Zhang, Chengdu University of Technology, ChinaReviewed by:
Zheng Cao, Chongqing University of Science and Technology, ChinaZuozhen Han, Shandong University of Science and Technology, China
Demin Zhang, SINOPEC Petroleum Exploration and Production Research Institute, China
Copyright © 2022 Zhou, Wang, Yang, Wen, Wang, Zeng, Wei, Xie and Huo. This is an open-access article distributed under the terms of the Creative Commons Attribution License (CC BY). The use, distribution or reproduction in other forums is permitted, provided the original author(s) and the copyright owner(s) are credited and that the original publication in this journal is cited, in accordance with accepted academic practice. No use, distribution or reproduction is permitted which does not comply with these terms.
*Correspondence: Xiyan Yang, eXh5c2dzdEAxNjMuY29t; Fei Huo, aHVvZmVpMzQyMDk5MjA2QDE2My5jb20=