- Swiss Seismological Service, Swiss Federal Institute of Technology of Zürich (ETHZ), Zürich, Switzerland
Site-specific hazard analyses and microzonation are important products for densely populated areas and facilities of special risk. The empirical amplification function is classically estimated using the standard spectral ratio (SSR) approach. The SSR simply consists in comparing earthquake recordings on soil sites with the recording of the same earthquake on a close-by rock reference. Recording a statistically significant number of earthquakes to apply the SSR can however be difficult, especially in low seismicity areas and noisy urban environments. On the contrary, computing the SSR from too few earthquakes can lead to an uncertain evaluation of the mean amplification function. Defining the minimum number of earthquake recordings in empirical site response assessment is thus important. We compute empirical amplification functions at 60 KiKnet sites in Japan from several hundred earthquakes and three Swiss sites from several tens of earthquakes. We performed statistical analysis on the amplification functions to estimate the geometric mean and standard deviation and more importantly to determine the distribution law of the amplification factors as a function of the number of recordings. Independent to the site and to the frequency, we find that the log-normal distribution is a very good approximation for the site response. Based on that, we develop a strategy to estimate the minimum number of earthquakes from the confidence interval definition. We find that 10 samples are the best compromise between minimizing the number of recordings and having a good statistical significance of the results. As a general rule, a minimum of 10 uncorrelated earthquakes should be considered, but the higher the number of earthquakes, the lower the uncertainty on the geometric mean of the site amplification function. Moreover, the linear site response is observed to be independent to the intensity of the ground motion level for the analyzed dataset.
Introduction
Site effects can significantly increase the seismic hazard and risk locally. Unconsolidated deposits such as thick and soft sediments in sedimentary basins are prone to strongly amplify the ground motion. Site effects are caused, among others, by the seismic impedance between rock and sediments, the 1D, 2D and 3D resonances, and the edge-generated surface waves. In turn, the site response can vary significantly from one site to another (site-to-site variability, e.g., Bindi et al., 2009; Hollender et al., 2015; Bindi et al., 2017; Imtiaz et al., 2018; Perron et al., 2018) and from one earthquake to another (within-site variability, e.g., Thompson et al., 2012; Ktenidou et al., 2016; Ktenidou et al., 2017; Maufroy et al., 2017; Perron, 2017; Zhu et al., 2018; Zhu et al., 2022). At large ground motion levels, non-linear effects in specific soils will increase the site response uncertainty as well (Régnier et al., 2013; Régnier et al., 2016). Understanding and reducing the ground motion estimation uncertainty is important for Probabilistic Seismic Hazard Assessment, especially at a long return period (Bommer and Abrahamson, 2006). The site-to-site and within-site variabilities have practical implications for site-specific study and microzonation, for instance on the spatial resolution and required duration of the instrumentation.
The within-site variability is very small when estimated from 1D SH site response analysis because it is a strong simplification of the real phenomena. On the contrary, approaches based on direct observations from real earthquake recordings are appropriate for analyzing the variability of the site response. One of the most commonly used approaches to measure the empirical amplification function is the standard spectral ratio (SSR) introduced by Borcherdt (1970). It consists in performing the ratio in the Fourier domain between the signal recorded at one station on sediments and the signal obtained at another station located nearby on a stiffer site condition (i.e., a rock site) for the same earthquake. However, in noisy urban areas in regions of low-to-moderate seismicity, recording earthquakes with a good signal-to-noise ratio (SNR) can require several months, if not years. It is thus important to estimate the number of earthquakes that should be recorded at the sites to evaluate the empirical site amplification function based on the desired accuracy.
The main goal of this work is to define, for the specification in the Swiss building SIA 261/1 (SIA, 2020), the minimum number of earthquakes in empirical site effect assessment. We first evaluate the stability and validity of the mean amplification as a function of the number of earthquake recordings used to compute it. The variations of the mean amplification are expected to be directly related to the within-site variability at each site. To verify that, we estimate the SSR and surface-to-borehole spectral ratio (SBSR) amplification function for stations of the Swiss strong motion network and of the Japanese KiKnet network having recorded hundreds of earthquakes. We use this large amount of data to determine the statistical distribution of the site amplification. Based on the statistical distribution, we propose an analytic equation predicting the variation of the mean amplification according to the standard deviation and to the number of recorded events. We also determined the dependence on the mean amplification functions of the ground motion intensity, measured as the peak ground acceleration (PGA).
Method and Resources
In Switzerland, we developed a waveform database covering the time period from January 1998 to September 2019. Waveforms at each Swiss site were selected according to a magnitude–distance filter. In Japan, the database is covering the time period from October 1997 to March 2016. The SSR is computed for each component individually or the mean of the two horizontal components and can be noted as follows:
where
1) Automatic quality checks of earthquake recordings and automatic picking of the P and S wave arrival (TP, TS) through a time–frequency analysis;
2) Selection of earthquakes with hypocentral distance at least five times the interstation distance (RSTA);
3) Selection of the signal window between TP and the coda defined by 3.3TS–2.3TP (Perron et al., 2017) and of the noise window before TP and of the same duration as the signal window. Site and reference use the same time windows;
4) Computation of the FAS for the noise and the signal window;
5) Computation of the horizontal mean FAS using the quadratic mean:
6) Smoothing and resampling of the horizontal mean FAS on a logarithmic scale using the Konno and Ohmachi (1998) approach with a b-value of 50;
7) Estimation of the SNR;
8) Selection of earthquakes with SNR > 5 over at least a two-octave frequency band window both at the site and at the reference;
9) Spectral ratio computation between the horizontal mean FAS at the site and at the reference for each earthquake;
10) Estimation of the within-site events geometric mean and standard deviation at each frequency;
11) Detection of outliers as a group of samples of probability <0.1% over a frequency band larger than one octave;
12) Outliers are discarded, and the geometric mean and standard deviation are recomputed
Figure 1 shows an example of the SBSR computation in Japan.
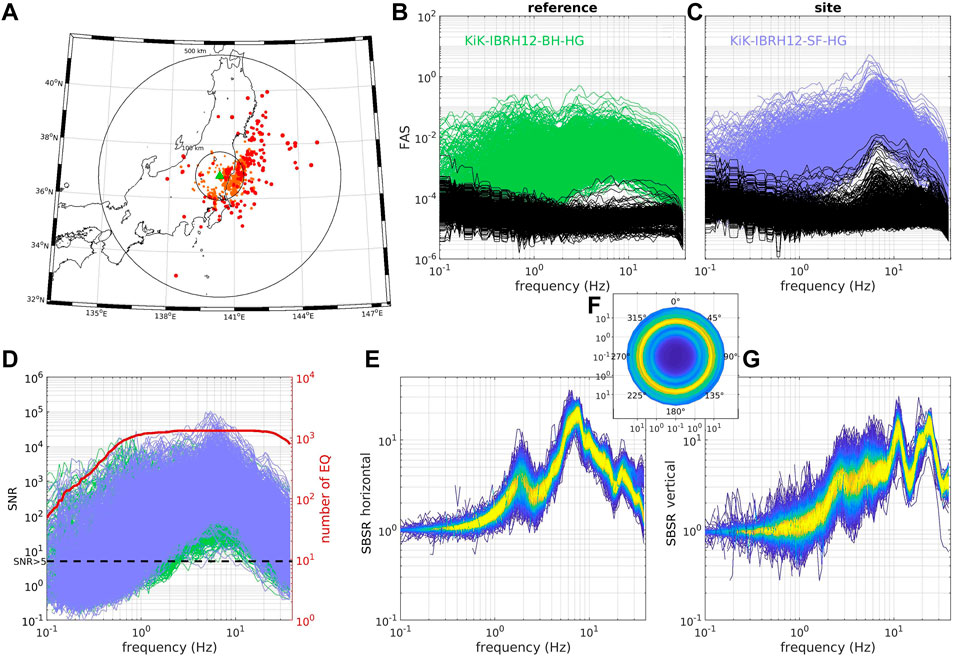
FIGURE 1. Example of the surface-to-borehole spectral ratio (SBSR) computation at KiKnet station IBRH12 in Japan. (A) The map shows the location of the site (green triangle) and the epicenters of the selected earthquakes (yellow-to-red dot according to the earthquake magnitude). Panels (B) and (C) present the power spectral density (PSD) for the noise (black lines) and for the earthquake recording on the horizontal mean component at the site (blue lines) and at the reference (green lines). Panel (D) indicates the SNR at the site (blue lines) and at the reference (green lines), as well as the number of earthquakes spectrum with SNR > 5 (red line) as a function of frequency. The distribution of the SBSR as a function of frequency for the horizontal mean component (E), for the horizontal as a function of the azimuth (F), and for the vertical (G) component. The color scale indicates the density of lines, each line corresponding to the SBSR of one single earthquake.
Standard Spectral Ratio and Surface-to-Borehole Spectral Ratio Results
In total, SSR is estimated from three pairs of stations where approximately 100 good-quality earthquakes have been recorded in Switzerland, and SBSR is computed from 60 pairs of surface-to-borehole stations with up to 2000 good-quality earthquakes in Japan. Figure 2 and Figure 3 respectively show the distribution of the SBSR for 60 pairs of surface-to-borehole stations in Japan and the SSR for the three pairs of surface stations in Switzerland. Figure 4 provides a summary of the number of good-quality earthquake recordings, geometric mean, and geometric standard deviation as a function of frequency in Japan (gray curves) and Switzerland (red curves).
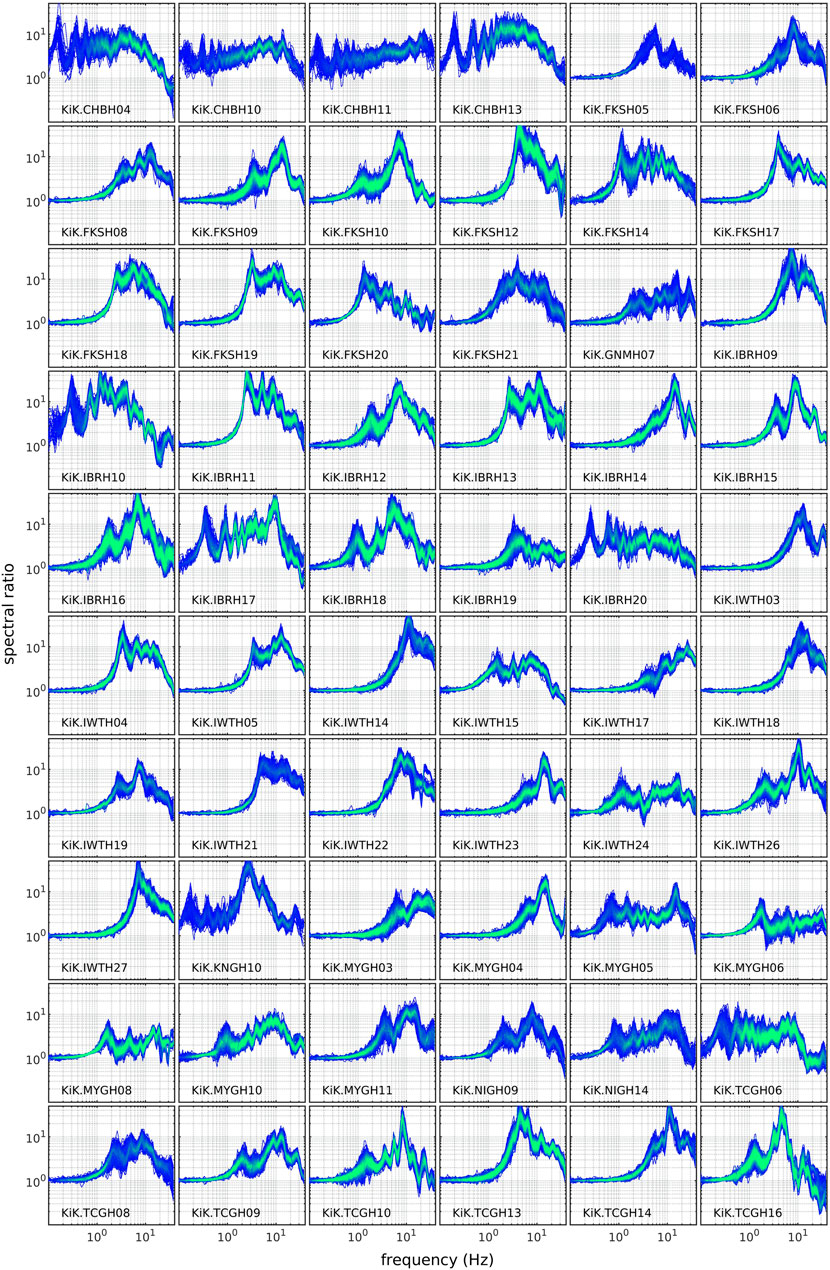
FIGURE 2. Amplification function computed from the SBSR between 60 pairs of stations in Japan. The color from dark blue to light green indicates an increasing density of curves, each curve corresponding to one single earthquake.
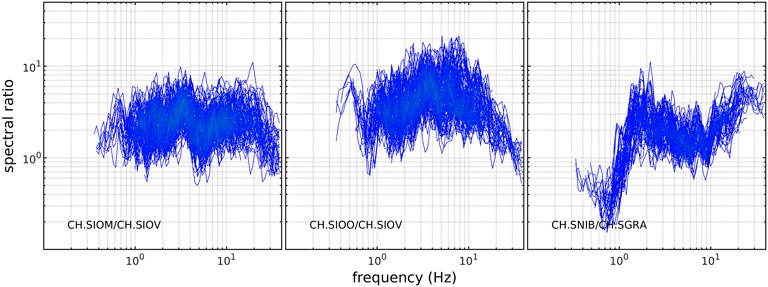
FIGURE 3. Amplification function computed from the standard spectral ratio between 3 pairs of stations in Switzerland. The color from dark blue to light blue indicates an increasing density of curves, each curve corresponding to one single earthquake.
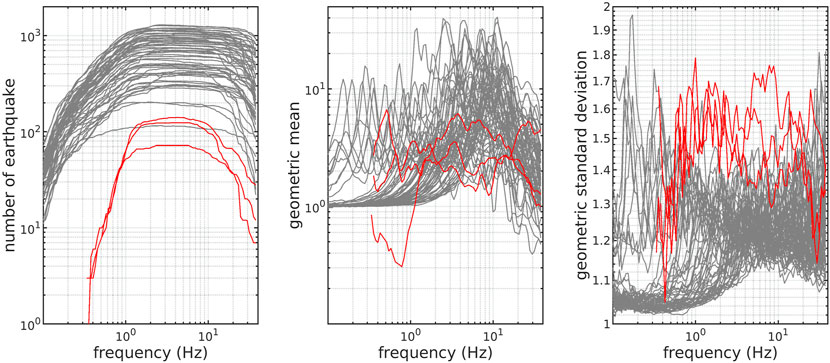
FIGURE 4. Number of good-quality earthquakes (left panel), within-site geometric mean (central panel), and within-site geometric standard deviation (right panel) as a function of frequency for 60 surface-to-borehole spectral ratios in Japan (gray curves) and three standard spectral ratio in Switzerland (red curves).
Figure 2, Figure 3, and Figure 4 clearly show that the amplification functions are different from one site to another, both in terms of mean and standard deviation. It reflects the differences in the geological conditions of the sites, which determine, among others, the fundamental resonance frequency of the site (f0), here corresponding to the first peak on the amplification function. The within-site standard deviation can also vary drastically from one site to another and depending on the frequency. In Japan, we can separate the amplification functions into two groups: the first group with f0 > 0.5 Hz, with an amplification function equal to one and low standard deviation (close to 1.05) for frequency below f0; a second group with f0 below the minimum frequency of the analysis here (0.1 Hz), and having significant amplification (above one) and high variability at low frequency. It is also clear that the variability of the site response is on average higher in Switzerland than that in Japan. For the Swiss sites, this is probably because of the SSR method imposing relatively high site-to-reference distances and non-negligible site effects at the surface reference station. In Japan, we can observe some anomalies (eye shapes departing from the log-normal distribution) in the amplification function at high frequency (e.g., for stations: KiK-IBRH13; KiK-IBRH17; KiK-TCGH16). It is not possible to clearly determine its origin, but from our experience, this is very probably an artificial artifact because of coupling issues of the borehole instrumentation or because of a modification on the instrumentation at some point due to maintenance of the station for instance.
Distribution of the Within-Site Variability
As we have seen in the previous section, both the mean and standard deviation of the amplification function as a function of frequency are dependent to the geological characteristics of the site itself. However, the nature of the site response distribution is the same independently to the site or to the frequency and has been shown to be well modeled by a log-normal distribution (Ktenidou et al., 2011). In other words, the distribution of the logarithm of the relative amplification of the ground motion between two sites is Gaussian. To qualitatively verify the log-normal distribution of the site response at every frequency, the quantile–quantile (Q–Q) plot and the histogram are represented at frequencies 0.5, 1.0, 2.5, 5.1, 9.9, and 20.6 Hz in Figure 5. The shape of the histograms of the logarithm of the amplification factors represents a Gaussian and Q–Q curves of every site at every frequency are well aligned along the 1/1 line, in particular in the interval
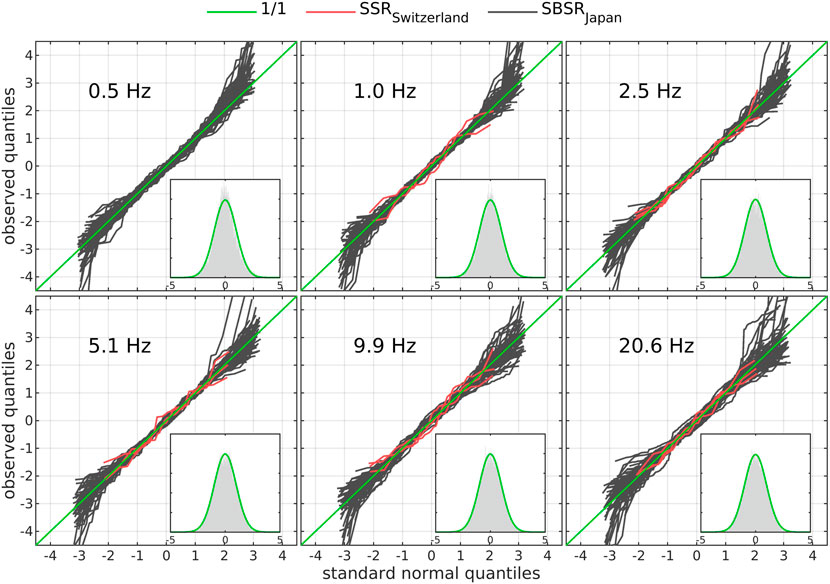
FIGURE 5. Quantile–quantile plot of the logarithm of the amplification factors for 60 surface-to-borehole spectral ratios in Japan (gray curves) and three standard spectral ratios in Switzerland (red curves) at six different frequencies (one panel per frequency). On each panel, the histogram (gray area) of the standard normal distribution computed from the logarithm of the amplification factors at all sites at the corresponding frequency is compared with the best normal distribution fit (green curve).
Proving the log-normal distribution of the amplification function is important because then peculiar statistical properties apply. For example, if a variable
where
with
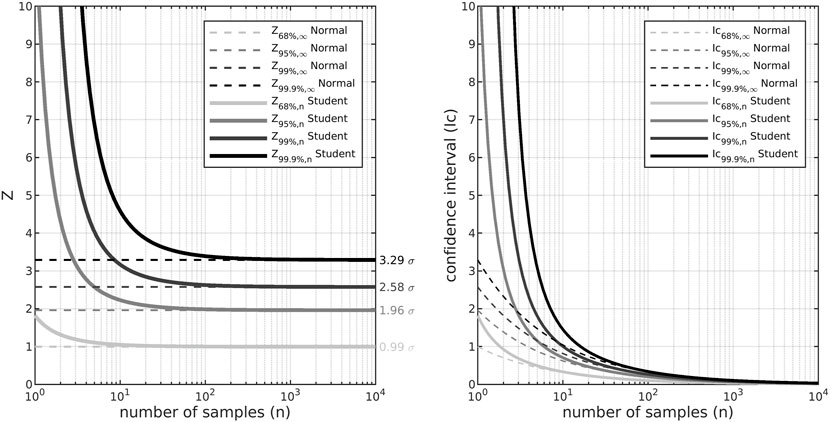
FIGURE 6. Critical value Z (left panel) and confidence interval (right panel) as a function of the number of samples for the confidence levels 68%, 95%, 99%, and 99.9% for the standard normal distribution (dashed lines), and the standard Student distribution (solid lines).
Figure 6 (right panel) shows the evolution of
Validity of the Confidence Interval Predictions
After demonstrating the validity of the log-normal assumption, we verified the validity of the prediction of
where
To estimate the reliability of the confidence interval more quantitatively, we bootstrapped the amplification factors at each frequency over
with the inequation equal to 1 when it is true and 0 otherwise.
Figure 7 shows the bootstrap estimation of
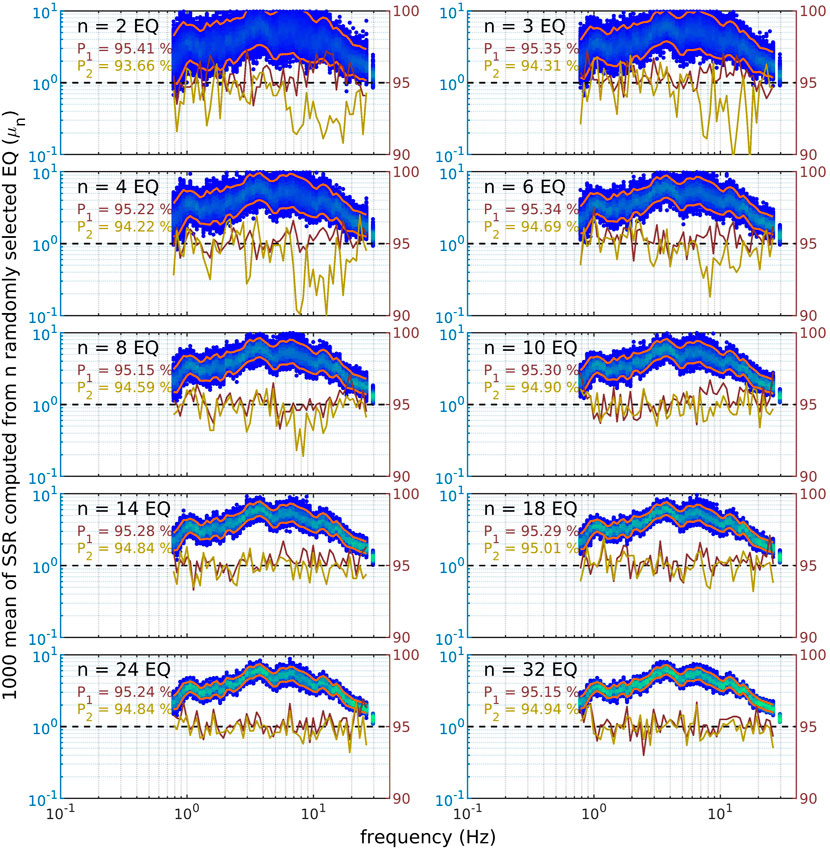
FIGURE 7. Evaluation of P1 (dark brown line) and P2 (light brown line) on the standard spectral ratio computed at Swiss station SIOO/SIOV from 1000 randomly selected subsets of
Now, we follow the same procedure for every three SSR in Switzerland and SBSR in Japan. The corresponding results are given in Figure 8. We can make a similar observation as in Figure 7,
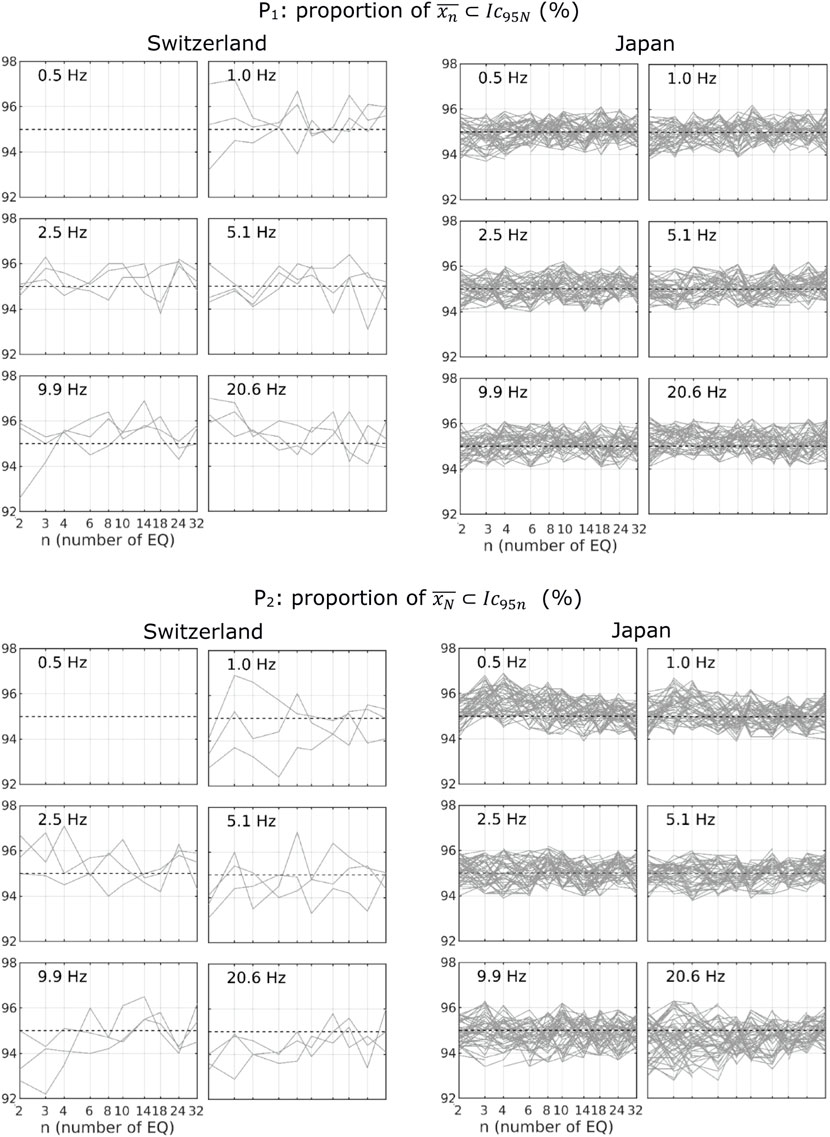
FIGURE 8. Evaluation of P1 and P2 as a function of the number of events
The confidence interval computed from a large site response dataset is a good estimator of what is going to be the behavior of the mean computed from much smaller subsets of even only three earthquakes and for any frequency. However, it is clear that using 10 recordings of earthquakes or above greatly improves the quality of the prediction and the significance of the results. In conclusion, at least 10 events should be considered to have a good statistical significance and to make good use of the confidence interval predicting power.
Variability of the Mean Amplification Function as a Function of the Number of Events
Some questions which arise when evaluating the amplification function at a specific site are as follows: Is the number of earthquake recordings sufficient to accurately estimate the amplification function? Which minimal number of earthquakes (
Provided that the geometric mean
For example, if the amplification at 1 Hz has been measured from
It is important to note that for a Student distribution,
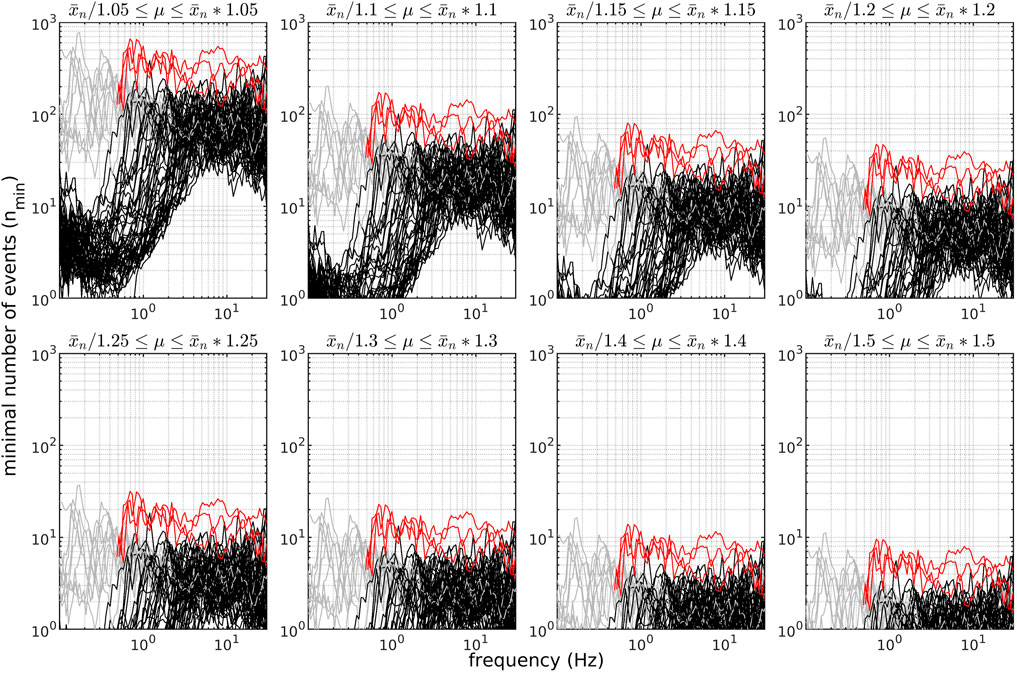
FIGURE 9. Minimum number of earthquakes as a function of frequency for the coefficient of variation
It has to be highlighted that
Dependence of the Site Response Variability on the Intensity of the Ground Motion
The dependence of the site response on the intensity of the ground motion is a complex research topic that interests the community for several decades (e.g., Sánchez-sesma, 1987; Aki, 1993). The non-linear behavior of unconsolidated soil to strong ground motion solicitations is of major interest in engineering seismology. Non-linearity tends to reduce the fundamental resonance frequency of the site, leading to an increase of the hazard at low frequency and a decrease at high frequency (Régnier et al., 2016). In extreme cases, it can also lead to liquefaction phenomena.
One question often arises when speaking about empirical site effects assessment which is: is the measured amplification function from weak ground motion representative of site response to strong ground motion? To address this question, we compute the equivalent of the standard normal distribution (
This common standard normal distribution formulation allows using the site response of every site together.
Figure 10 shows the number of events per frequency, the distribution of the PGA and the normalized amplification function for four PGA bins [(0.001 0.01), (0.01 0.1), (0.1 1), and (1 10) m/s2]. First, it should be mentioned that the number of events varies strongly from one PGA bin to another. This explains the apparent differences when looking at the normalized amplification function (black curves) of the different bin. We observe that the normalized amplification function for every PGA bin can be explained by the standard normal distribution, which indicates that no non-linear behavior is observed here. The mean is fairly equal to 0 and the standard deviation is equal to 1 for every frequency of every bin. That demonstrates, first, that the linear behavior characterizes the vast majority of the sites, and second, that the linear site response is independent to the ground motion intensity. Therefore, if we consider a specific site having a linear behavior, the amplification function observed from the weak motion of a small magnitude earthquake will be the same as the one for the strong motion of a large magnitude earthquake, all other things being the same. This highlights the importance and the validity of using the recording of low-to-moderate earthquakes to assess the anelastic amplification functions for larger earthquakes as long as there is no significant non-linear site response at the site of interest.
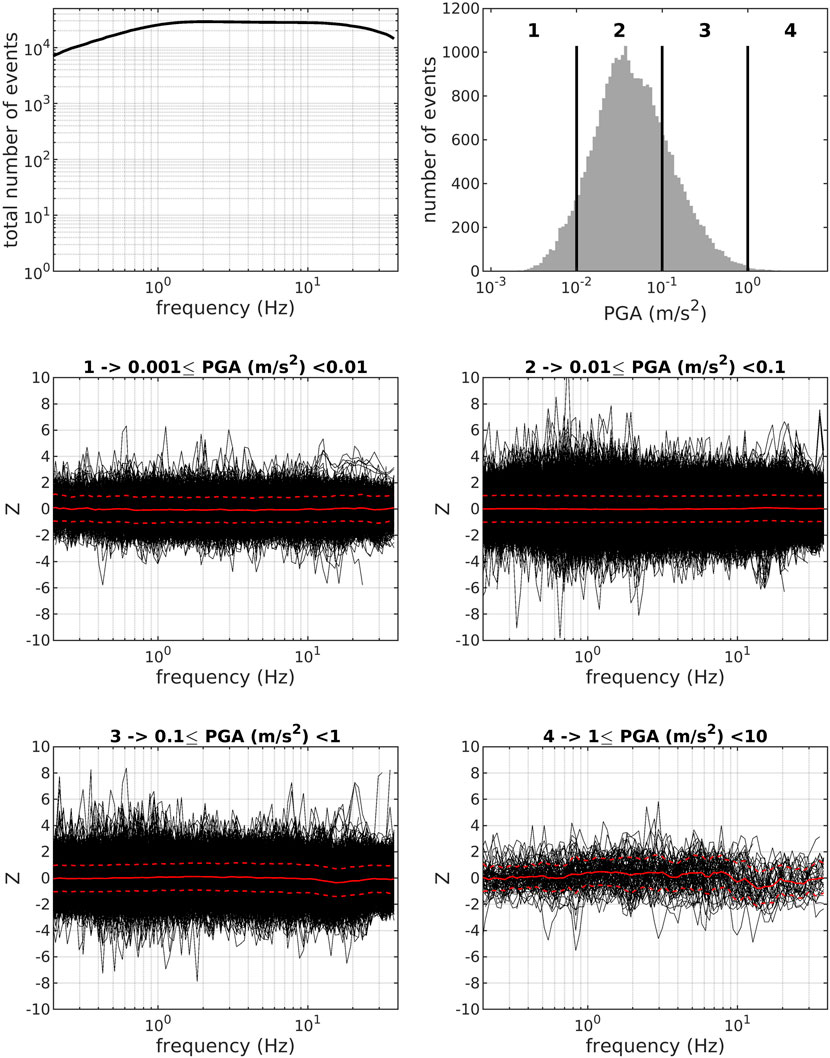
FIGURE 10. Top-left panel: Total number of normalized amplification functions obtain from 3 Swiss SSR distribution and 60 SBSR Japanese distribution and as a function of frequency. Top right: Histogram of the peak ground acceleration (PGA) distribution. From middle left to bottom-right panel: normalized amplification function for four PGA bins and as a function of frequency. The mean and mean plus/minus standard deviation are represented with solid red lines and dotted red lines respectively.
Conclusion
Site effect is a major contributor to the seismic hazard, and its evaluation at specific sites of interest generally requires the recording of several earthquakes. We address here the question of the site response variability and of the minimum necessary number of earthquakes to be recorded.
To address this question, we carefully compute empirical amplification functions at 60 KiKnet sites from several hundred earthquakes and three Swiss sites from several tens of earthquakes. We performed statistical analysis on the amplification function to estimate the geometric mean and standard deviation, and more importantly to determine the distribution law of the amplification factor at each frequency. Independent to the site and to the frequency, we found that the log-normal distribution is a very good approximation for the site response. Based on that we developed a strategy to estimate the minimum number of earthquakes from the confidence interval definition. We first demonstrate the validity of the use of the confidence interval to model the uncertainty of the geometric mean estimation. We found that between 8 and 14 earthquakes are necessary to have a good prediction by the confidence interval, that is to say, a good statistical significance. For most of the sites, 10 samples seem to be the best compromise between minimizing the number of recordings and having a good statistical significance of the results. Based on the confidence interval, we provide the analytic formula to estimate the minimum number of earthquakes to be recorded, as a function of the within-site standard deviation (Eq. 11). We used it on the Swiss and Japanese amplification function and determine, among others, that with a 95% probability: the mean varies by less than 40% for 10 earthquakes, and less than 25% for 20 events.
It is very important to point out that satisfying the minimal number of earthquakes by itself is not sufficient. The selected earthquakes should be uncorrelated and as much evenly distributed around the site as possible to cover the entire variability of the site response. Therefore, one should not use only earthquakes belonging to a single cluster of events. In our dataset, the linear site response is observed to be independent to the intensity of the ground motion. In other words, assessing the site response from the recording of low PGA and low magnitude earthquakes, provides the same amplification functions as from recording of high PGA and large magnitude earthquakes, as far as the soil behaves linearly.
As a general rule, a minimum of 10 uncorrelated earthquakes should be considered, but the higher the number of earthquakes, the lower the uncertainty on the geometric mean site response assessment. Based on our results, the specification in the Swiss building SIA 261/1 recommends taking a minimum of 10 uncorrelated earthquakes to perform site-specific studies.
Data Availability Statement
Publicly available datasets were analyzed in this study. This data can be found here: The data of the Swiss stations (CH, https://doi.org/10.12686/sed/networks/ch) can be accessed following the instruction on the webpage http://www.seismo.ethz.ch/en/research-and-teaching/products-software/waveform-data/ (last accessed April 2022). The data of the station site characterization can be accessed at http://stations.seismo.ethz.ch (last accessed April 2022). The figures were produced using MATLAB which is available at www.mathworks.com/products/matlab (last accessed August 2021). Japaness KiKnet data are available at https://www.kyoshin.bosai.go.jp/ (last accessed June 2022).
Author Contributions
VP did most of the work, as well as the writing of the article. PB helped with the statistic and reviewing work. DF is the main supervisor and fund provider.
Funding
This work received financial contributions from the Swiss Federal Office for the Environment (FOEN), the Swiss Federal Office for Civil Protection (FOCP), and the Swiss Federal Institute of Technology Zurich (ETHZ). Open access funding is provided by ETH Zurich.
Conflict of Interest
The authors declare that the research was conducted in the absence of any commercial or financial relationships that could be construed as a potential conflict of interest.
Publisher’s Note
All claims expressed in this article are solely those of the authors and do not necessarily represent those of their affiliated organizations, or those of the publisher, the editors, and the reviewers. Any product that may be evaluated in this article, or claim that may be made by its manufacturer, is not guaranteed or endorsed by the publisher.
Acknowledgments
This work was made in the framework of the Earthquake Risk Model for Switzerland project financed by contributions from the Swiss Federal Office for the Environment (FOEN), Swiss Federal Office for Civil Protection (FOCP), and Swiss Federal Institute of Technology Zurich (ETHZ).
References
Aki, K. (1993). Local Site Effects on Weak and Strong Ground Motion. Tectonophysics 218, 93–111. doi:10.1016/0040-1951(93)90262-i
Bindi, D., Luzi, L., and Pacor, F. (2009). Interevent and Interstation Variability Computed for the Italian Accelerometric Archive (ITACA). Bull. Seismol. Soc. Am. 99, 2471–2488. doi:10.1785/0120080209
Bindi, D., Spallarossa, D., and Pacor, F. (2017). Between-Event and Between-Station Variability Observed in the Fourier and Response Spectra Domains: Comparison with Seismological Models. Geophys. J. Int. 210, 1092–1104. doi:10.1093/gji/ggx217
Bommer, J. J., and Abrahamson, N. A. (2006). Why Do Modern Probabilistic Seismic-Hazard Analyses Often Lead to Increased Hazard Estimates? Bull. Seismol. Soc. Am. 96, 1967–1977. doi:10.1785/0120060043
Borcherdt, R. D. (1970). Effects of Local Geology on Ground Motion Near San Francisco Bay. Bull. Seismol. Soc. Am. 60, 29–61.
Cadet, H., Pierre-Yves, B., and Adrian, R.-M. (2012). Site Effect Assessment Using KiK-Net Data: Part 1. A Simple Correction Procedure for Surface/Downhole Spectral Ratios. Bull. Earthq. Eng. 10, 421–448. doi:10.1007/s10518-011-9283-1
Hobiger, M., Bergamo, P., Imperatori, W., Panzera, F., Marrios Lontsi, A., Perron, V., et al. (2021). Site Characterization of Swiss Strong-Motion Stations: The Benefit of Advanced Processing Algorithms. Bull. Seismol. Soc. Am. 111, 1713–1739. doi:10.1785/0120200316
Hollender, F., Cornou, C., Dechamp, A., Oghalaei, K., Renalier, F., Maufroy, E., et al. (2017). Characterization of Site Conditions (Soil Class, VS30, Velocity Profiles) for 33 Stations from the French Permanent Accelerometric Network (RAP) Using Surface-Wave Methods. Bull. Earthq. Eng. 16, 2337–2365. doi:10.1007/s10518-017-0135-5
Hollender, F., Perron, V., Imtiaz, A., Svay, A., Mariscal, A., Bard, P.-Y., et al. (2015). “Close to the Lair of Odysseus Cyclops: The SINAPS@ Post-seismic Campaign and Accelerometric Network Installation on Kefalonia Island,” in 9ème Colloque National AFPS, Marne-la-Vallée, France, 30 Nov-2 Dec 2015.
Hollender, F., Roumelioti, Z., Regnier, J., Perron, V., and Bard, P.-Y. (2018). “Respective Advantages of Surface and Downhole Reference Stations for Site Effect Studies: Lessons Learnt from the Argonet (Cephalonia Island, Greece) and Cadarache (Provence, France) Vertical Arrays,” in 16th European Conference on Earthquake Engineering (16ECEE), Thessaloniki, Greece, 18-21June 2018, 13.
Imtiaz, A., Perron, V., Hollender, F., Bard, P. Y., Cornou, C., Svay, A., et al. (2018). Wavefield Characteristics and Spatial Incoherency: A Comparative Study from Argostoli Rock‐ and Soil‐Site Dense Seismic Arrays. Bull. Seismol. Soc. Am. 108, 2839–2853. doi:10.1785/0120180025
Konno, K., and Ohmachi, T. (1998). Ground-Motion Characteristics Estimated from Spectral Ratio between Horizontal and Vertical Components of Microtremor. Bull. Seismol. Soc. Am. 88, 228–241. doi:10.1785/bssa0880010228
Ktenidou, O.-J., Chávez-García, F.-J., Raptakis, D., and Pitilakis, K. D. (2016). Directional Dependence of Site Effects Observed Near a Basin Edge at Aegion, Greece. Bull. Earthq. Eng. 14, 623–645. doi:10.1007/s10518-015-9843-x
Ktenidou, O.-J., Chavez-Garcia, F. J., and Pitilakis, K. D. (2011). Variance Reduction and Signal-To-Noise Ratio: Reducing Uncertainty in Spectral Ratios. Bull. Seismol. Soc. Am. 101, 619–634. doi:10.1785/0120100036
Ktenidou, O.-J., Roumelioti, Z., Abrahamson, N., Cotton, F., Pitilakis, K., and Hollender, F. (2017). Understanding Single-Station Ground Motion Variability and Uncertainty (Sigma): Lessons Learnt from EUROSEISTEST. Bull. Earthq. Eng. 16, 2311–2336. doi:10.1007/s10518-017-0098-6
Maufroy, E., Chaljub, E., Theodoulidis, N. P., Roumelioti, Z., Hollender, F., Bard, P. Y., et al. (2017). Source‐Related Variability of Site Response in the Mygdonian Basin (Greece) from Accelerometric Recordings and 3D Numerical Simulations. Bull. Seismol. Soc. Am. 107, 787–808. doi:10.1785/0120160107
Perron, V. (2017). Apport des enregistrements de séismes et de bruit de fond pour l’évaluation site-spécifique de l’aléa sismique en zone de sismicité faible à modérée. PhD thesis. Grenoble, France: Université Grenoble Alpes.
Perron, V., Gélis, C., Froment, B., Hollender, F., Bard, P.-Y., Cultrera, G., et al. (2018). Can Broad-Band Earthquake Site Responses Be Predicted by the Ambient Noise Spectral Ratio? Insight from Observations at Two Sedimentary Basins. Geophys. J. Int. 215, 1442–1454. doi:10.1093/gji/ggy355
Perron, V., Laurendeau, A., Hollender, F., Bard, P.-Y., Gélis, C., Traversa, P., et al. (2017). Selecting Time Windows of Seismic Phases and Noise for Engineering Seismology Applications: A Versatile Methodology and Algorithm. Bull. Earthq. Eng. 16, 2211–2225. doi:10.1007/s10518-017-0131-9
Régnier, J., Cadet, H., and Bard, P. Y. (2016). Empirical Quantification of the Impact of Nonlinear Soil Behavior on Site Response. Bull. Seismol. Soc. Am. 106, 1710–1719. doi:10.1785/0120150199
Régnier, J., Cadet, H., Bonilla, L. F., Bertrand, E., and Semblat, J.-F. (2013). Assessing Nonlinear Behavior of Soils in Seismic Site Response: Statistical Analysis on KiK-Net Strong-Motion Data. Bull. Seismol. Soc. Am. 103, 1750–1770. doi:10.1785/0120120240
Sánchez-sesma, F. J. (1987). Site Effects on Strong Ground Motion. Soil Dyn. Earthq. Eng. 6, 124–132. doi:10.1016/0267-7261(87)90022-4
SIA (2020). SIA 261 Einwirkungen auf Tragwerke. Zurich, Switzerland (in German): Schweizerischer Ingenieur- und Architekentverein, Swiss Society of Engineers and Architects. Available at: http://shop.sia.ch/normenwerk/ingenieur/sia%20261/d/2020/D/Product/(Accessed April 1, 2022).
Thompson, E. M., Baise, L. G., Tanaka, Y., and Kayen, R. E. (2012). A Taxonomy of Site Response Complexity. Soil Dyn. Earthq. Eng. 41, 32–43. doi:10.1016/j.soildyn.2012.04.005
Zhu, C., Chávez-García, F. J., Thambiratnam, D., and Gallage, C. (2018). Quantifying the Edge-Induced Seismic Aggravation in Shallow Basins Relative to the 1D SH Modelling. Soil Dyn. Earthq. Eng. 115, 402–412. doi:10.1016/j.soildyn.2018.08.025
Keywords: seismic hazard, site effects, microzonation, statistic, signal processing
Citation: Perron V, Bergamo P and Fäh D (2022) Evaluating the Minimum Number of Earthquakes in Empirical Site Response Assessment: Input for New Requirements for Microzonation in the Swiss Building Codes. Front. Earth Sci. 10:917855. doi: 10.3389/feart.2022.917855
Received: 11 April 2022; Accepted: 01 June 2022;
Published: 13 July 2022.
Edited by:
Simone Barani, University of Genoa, ItalyReviewed by:
Giovanni Forte, University of Naples Federico II, ItalyEnrico Paolucci, University of Siena, Italy
Copyright © 2022 Perron, Bergamo and Fäh. This is an open-access article distributed under the terms of the Creative Commons Attribution License (CC BY). The use, distribution or reproduction in other forums is permitted, provided the original author(s) and the copyright owner(s) are credited and that the original publication in this journal is cited, in accordance with accepted academic practice. No use, distribution or reproduction is permitted which does not comply with these terms.
*Correspondence: Vincent Perron, dmluY2VudC5wZXJyb24ubWFpbEBnbWFpbC5jb20=