- 1Department of Exact and Natural Sciences, Institute of Interdisciplinary Research, “Alexandru Ioan Cuza” University of Iaşi, Iaşi, Romania
- 2Department of Biology, Case Western Reserve University, Cleveland, OH, United States
One of the most applied tools for documenting cultural variability and for tracing cultural trajectories within the environmental context is eco-cultural niche modeling and its associated methodology. The niche breadth characterization quantitatively evaluates the links between a given adaptive system and ecological constraints, which provides valuable information for archeology. For this purpose, in this study, 10 independent climatic and topographic environmental variables were interpolated, and eco-cultural niche modeling techniques were used to determine whether these differences in geographic distributions and niche breadth are consequences of differences in five Cucuteni–Trypillia groups that flourished in Eastern Europe during the Eneolithic (cal. 5,400/5,300–2,800/2,700 BCE). Our results showed that the eco-cultural niches of Cucuteni–Trypillia groups are significantly overlapping, and the expansion trend of the last two cultural groups (Late Eneolithic–cal. 4,100/4,000–2,800/2,700 BCE) into the northeastern steppe regions was not due to ecological niche differences but rather a result of other cultural factors. Furthermore, we highlighted that the first three Cucuteni–Trypillia groups (Early-to-Middle Eneolithic—cal. 5,400/5,300–4,100/4,000 BCE) had slightly more constrained ecological niches in the mid-Holocene ecosystems than the Late Eneolithic groups. The results have significant implications for understanding the geographical range dynamics and distribution of the last great Chalcolithic society of Old Europe and contribute to the characterization of ecological niches they have exploited during the cultural evolutionary process.
Introduction
One of the broad goals of modern archeology is to understand and describe the behaviors of prehistoric populations by documenting cultural variability and tracing cultural trajectories within the environmental context (Banks et al., 2006; Banks et al., 2013; d'Errico and Banks, 2013; Banks, 2017). In recent decades, the development of Geographic Information System (GIS) software (Anderson and Raza, 2010), geostatistical models based on the R package for statistics (R Core Team, 2015), and the tools for estimating species distributions (Phillips et al., 2006; Phillips and Dudík, 2008; Elith et al., 2010), together with free access to archeological datasets (Harper et al., 2019), has attracted the interest of researchers in implementing new or upgraded software extensions to approach the links among 1) environmental conditions (Argyriou et al., 2017; Noviello et al., 2018; Mihu-Pintilie and Nicu, 2019; Tapete and Cigna, 2019), 2) bioclimatic conditions (Lemmen et al., 2011; Birks et al., 2015; Krauß et al., 2017; Ivanova et al., 2018), and 3) cultural evolutionary processes of past human populations (Tallavaara et al., 2015; Pétillon et al., 2016; Harper et al., 2019; Whitford, 2019). One of these GIS-based applications, frequently used by archaeologists within interdisciplinary studies (e.g., geo-archaeological research), is eco-cultural niche modeling (ECNM) and its associated methodology (Banks et al., 2006).
Broadly, ECNM has been adopted by archeologists (Banks, 2017) to explore the interactions between cultural and natural systems and to understand how ecological dynamics influenced the adaptations and movements of prehistoric populations (Banks et al., 2008; Banks et al., 2009). Therefore, even if the concept of ecological niche modeling (ENM) is derived from and commonly used in biodiversity (Soberón and Peterson, 2005; Bobrowski et al., 2018; Gherghel et al., 2019) and geographic ecology (Peterson et al., 2011), ECNM applies the same methodology to analyses of the archaeological record and prehistoric human culture using GIS. The usefulness of ECNM for archeology is that it offers the opportunity to quantitatively evaluate the hypothesis that a given adaptive system was influenced by ecological constraints or alternatively that the characteristics and geographic distribution may have been influenced more by non-ecological processes (e.g., cultural factors) (Banks et al., 2011). At the same time, the ability to identify and characterize ECNM that prehistoric populations exploited during the different cultural periods is an important aspect of any environmental modeling effort in conjunction with cultural variability analysis, especially when significant climate change was recorded (Banks et al., 2013; Banks, 2017).
The Eneolithic (cal. ∼ 5,000–3,000 BCE in Eastern Europe) has been broadly categorized as a period of transition from that of continuous spatial dynamics to relatively sedentary settlement-based agriculture (Harper et al., 2019; Mihu-Pintilie and Nicu, 2019). Prehistoric populations adapted to the environment through complex, often specialized, subsistence strategies, allowing human cultures to spread across relatively heterogeneous landscapes (Banks et al., 2006). For this reason, successive human migration and colonization of resource-rich areas with productive loess soils (e.g., Chernozem) (Argyriou et al., 2017; Noviello et al., 2018; Tapete and Cigna, 2019) and high mineral resources (e.g., salt, flint, and clay) (Lemmen et al., 2011; Krauß et al., 2017; Brigan and Weller, 2018; Ivanova et al., 2018) have been found all over Europe (Tallavaara et al., 2015; Pétillon et al., 2016; Harper et al., 2019). In this context, one of the first approaches to apply ECNM in geographical and archaeological studies was to identify the links between agricultural practices derived from large- (Banks et al., 2008) to middle-scale (Ivanova et al., 2018; Whitford, 2019) environmental and bioclimatic patterns, with the cultural evolution of the most representative prehistoric communities (Peterson et al., 2011). Despite this, only a few studies on the niche construction theory (NCT) have been developed for the same Eneolithic cultural unity in Eastern Europe (Harper et al., 2019; Whitford, 2019), even if the amount of archeological evidence meets the requirements for ECNM methodology. This is also the case in the current territories of Romania, Moldova, and Ukraine, where the entire geographic area has a high density of Eneolithic archaeological sites corresponding to Precucuteni/Cucuteni (in Romania and Moldova) and Trypillia (in Ukraine) culture or Cucuteni–Trypillia cultural unity (CTU), together known as the last great Chalcolithic society of Old Europe (cal. 5,400/5,300–2,800/2,700 BCE) (Gimbutas, 1974; Monah and Monah, 1997; Chapman et al., 2019) (Figure 1). However, by constructing, characterizing, and comparing the niche breadth of CTU groups, we aimed to test for ECNM applicability, contributing to a broader perspective on the ecological adaptation and spatiotemporal dynamics inside the most representative culture of Old Europe.
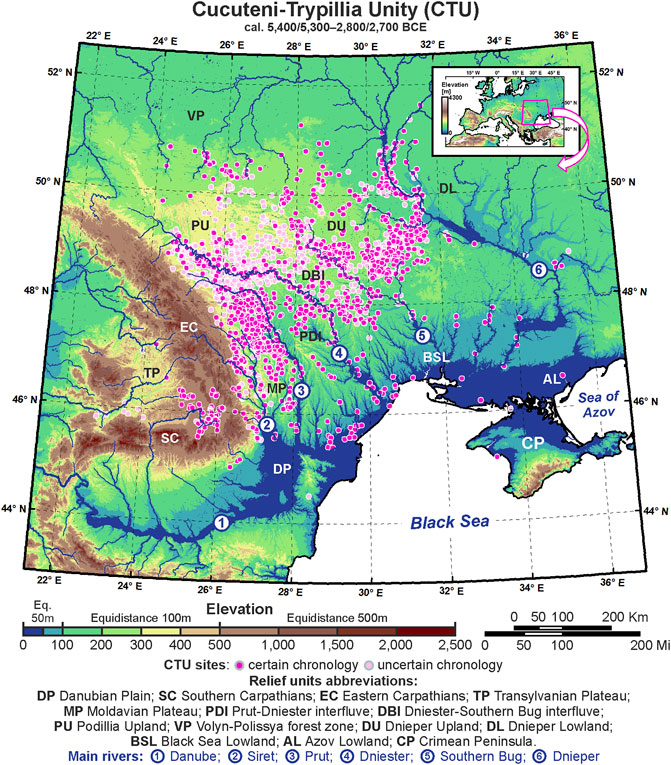
FIGURE 1. Elevation in Eastern Europe and the distribution of Cucuteni–Trypillia cultural unity (CTU) sites; the relief units and major rivers are highlighted for the study area.
Consistent with this study’s main goal, we tested the hypothesis that the observed differences in the geographic distribution of CTU groups reflect the exploitation of different ecological niches inside of the same Eneolithic culture. We provided the first GIS-based Eneolithic ECNM for a vast area in Eastern Europe among Eastern Carpathians to the west, the Dnieper river valley to the east and north, and the Black Sea to the south (Figure 1). To this end, the location of more than 2,200 Eneolithic archaeological sites belonging to the CTU complex, divided into five chronological cultural groups (cal. 5,400–2,700 BCE) (Mantu, 1998; Shukurov et al., 2015), was investigated. The correlation of archaeological data with environmental conditions was made using 10 ecological variables, of which five were terrain variables (Moore et al., 1991; Riley et al., 1999; Sørensen et al., 2006; De Reu et al., 2013; Burrough et al., 2015; Mihu-Pintilie and Nicu, 2019; Whitford, 2019) and five were bioclimatic variables selected from three different global climatic model databases corresponding to the mid-Holocene climatic conditions (Hijmans et al., 2005). This information should allow us to identify the differences in eco-cultural niche breadth among CTU groups and analyze possible links between human adaptive systems and the ecological niches exploited in the heterogeneous environment of the study area. Also, based on niche characterization, we aimed to answer the following questions: 1) Did the heterogeneous ecological and bioclimatic characteristics in Eastern Europe play a significant role in the evolutionary process of successive phases of the CTU? and 2) Were these particular traits of the successive cultural groups correlated with modifying habitation practices (e.g., subsistence agriculture and population growth) and the subsequent occupation of other territories?
Data and Methods
Figure 2 summarizes the workflow chart followed in this study describing the archeological geodatabase construction in GIS, the ecological variable-obtaining process, the key steps for ECNM modeling, and the statistical tools and metrics used for niches’ interpretation. Each methodological step will be detailed in the subsections as follows.
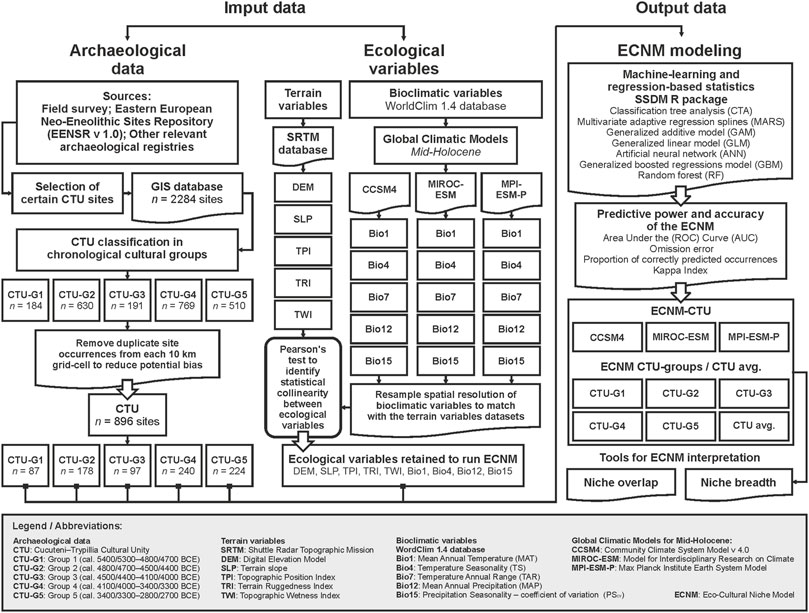
FIGURE 2. Workflow chart followed in this study to obtain the eco-cultural niche (ECNM) breadth of the Cucuteni–Trypillia cultural unity (CTU) during the Eneolithic in Eastern Europe.
Study Area Delineation
Spanning approximately two and a half millennia (cal. ∼ 5,400–2,700 BCE), the CTU complex spreads from a small area in the Eastern Carpathian lowland, defined by the Siret and Prut watersheds which are the last Danube tributaries. They came to occupy a territory between the eastern bank of the Dnieper to the east, the Volyn Polissya forest zone of modern Ukraine to the north, and the northwestern coast of the Black Sea to the south. To the west, due to the Eastern Carpathians, which acted as an orographic barrier, the expansion was hampered, and only a small group managed to cross the mountains through the Curvature Carpathians passages and settle in the intra-Carpathian depressions (Transylvanian Plateau). Therefore, the dominant characteristic of the CTU complex was the extension to the eastern forest-steppe zone, defining the transition from continuous spatial dynamics to a relatively sedentary settlement-based agriculture located on more productive soils. Starting with 4,400 BCE, respectively, during the transition from Middle-to-Late Eneolithic, this fact is also highlighted by the demographic development when the significant regional differences in settlement structure and spatial organization between west and east have been attested by archeologists (Harper et al., 2019). Overall, the dominant population movements inside the CTU culture were from west to the northeast and east or from the sub-Carpathians region to the upper and middle basins of Siret, Prut, Dniester, Southern Bug, and Dnieper river basins. In these geographical settings, the major landform units’ distribution (e.g., Eastern Carpathian, Black Sea shoreline, and main watercourse) and CTU sites’ distribution were used to avoid overrating the eco-cultural niche extents and losing their resolutions used as optimal landmarks for geographical niche delineation. Therefore, the study area we referred to in this work is located in the northeastern region of Old Europe, or more precisely, between 44°N–52°N latitude and 22°E–36°E longitude (Figure 1).
Archeological Data Occurrence
In order to identify the links among archaeological settlement placement, human adaptive systems, and the ecological niches exploited by prehistoric populations during the Eneolithic, a geodatabase was created using Esri ArcGIS 10.6 based on field surveys–only in Romania (Mihu-Pintilie and Nicu, 2019), along with relevant archaeological documentation and registries–for the entire study area (Harper et al., 2019). We only used the CTU settlements where independent archaeological documentation was well-grounded in reports, scientific publications (e.g., articles, supplementary material, and data in journal articles), radiocarbon-based chronology, and georeferenced archeological maps (Nikitin et al., 2010; Diachenko and Menotti, 2012; Brigan and Weller, 2013, 2018; Chapman et al., 2014; Chapman et al., 2019; Harper et al., 2019; Mihu-Pintilie and Nicu, 2019). The main source for CTU sites located outside the Romanian territory was the Eastern European Neo-Eneolithic Sites Repository (EENSR) version 1.0 database, published for the first time by Harper et al. (2019), which contains information about more than 8,000 Neo-Eneolithic sites. The EENSR database was constructed based on information recorded in national-level registries such as the Register of Tripoli Culture Monuments (RPTK)–Ukraine, Register of Monuments of the Republic of Moldova (RMRM), and National Archaeological Record of Romania (RAN), along with various other studies (Harper et al., 2019). Therefore, after the EENSR was thoroughly revised to include only archaeological sites with remains recovered from intact stratigraphic contexts and combined with our database (Mihu-Pintilie and Nicu, 2019), only 2,284 sites could be certainly identified as belonging to the specific CTU.
Being one of the most important and best-explored early farming communities in Eastern Europe, the CTU was discovered independently in Eastern Romania (Cucuteni) and Central-Western Ukraine (Trypillia) in the late 19th century. For these reasons, the CTU cultural stages were studied separately by various authors for a long time (Shukurov et al., 2015) and broadly classified into Ariuşd, Cucuteni, and Eastern Tripolye (ETC) and Western Tripolye (WTC) cultures (Harper et al., 2019). However, to overcome this generalized geographical–archaeological classification, the selected CTU sites were chronologically united and classified into five groups, according to Mantu (1998) and Shukurov et al. (2015): CTU-G1 (cal. 5,400/5,300–4,800/4,700 BCE); CTU-G2 (cal. 4,800/4,700–4,500/4,400 BCE); CTU-G3 (cal. 4,500/4,400–4,100/4,000 BCE); CTU-G4 (cal. 4,100/4,000–3,400/3,300 BCE); and CTU-G5 (cal. 3,400/3,300–2,800/2,700 BCE). The last step in archaeological data processing was to remove duplicate site occurrences from each grid cell in order to reduce potential bias and to ensure that the training and testing occurrence datasets in the ECNM were spatially unique (Anderson and Gonzalez, 2011; Boria et al., 2014; Vidal-Cordasco and Nuevo-López, 2021). Furthermore, the occurrence data were reduced to ensure a minimum distance of 10 km between any pair of occurrence points, preventing oversampling of environmental conditions from certain areas and reducing spatial autocorrelation (Figure 2). Ultimately, 896 CTU archaeological sites were retained to run the ECNM (Figure 3). More details on chronology, cultural stage names in different countries, and CTU groups’ abbreviation used in this study are found in Table 1.
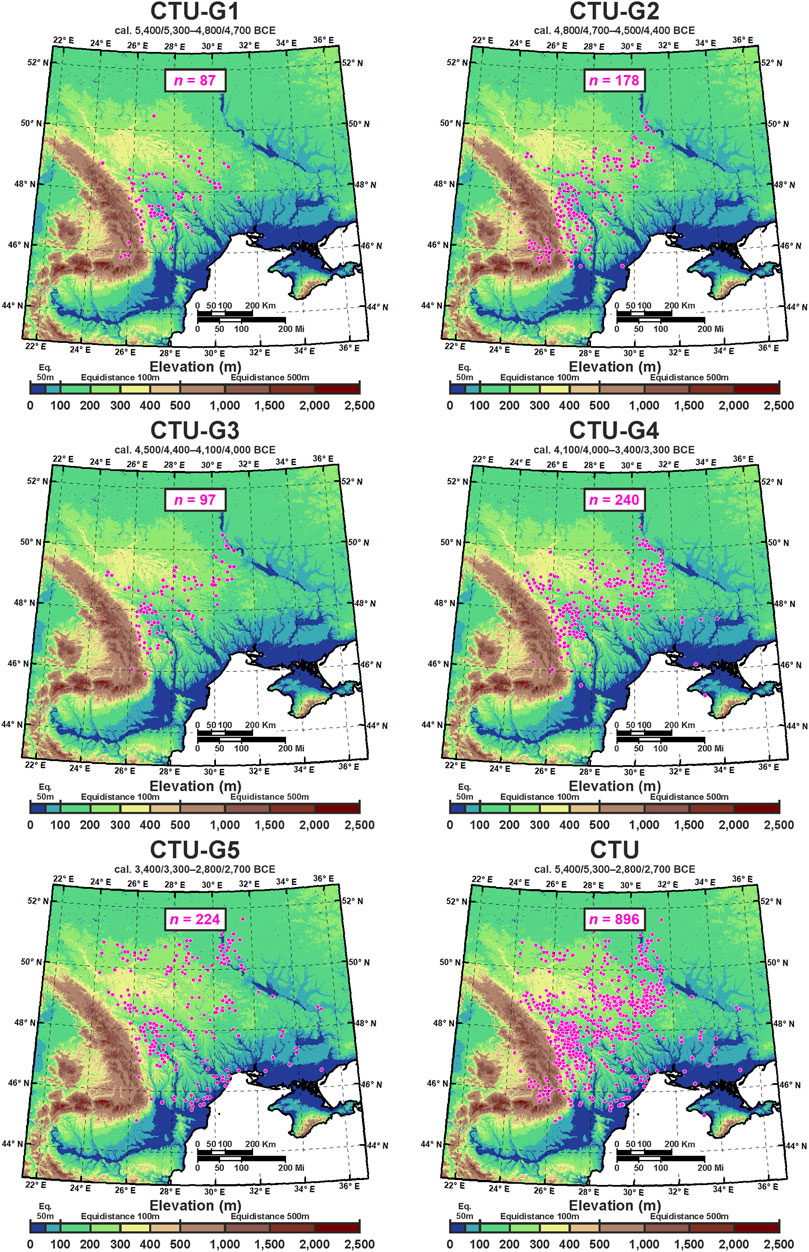
FIGURE 3. CTU groups’ (see Table 1) and total CTU sites’ distribution were used to run the ECNM; duplicate site occurrences from each grid cell were removed to reduce potential bias.

TABLE 1. Cucuteni–Trypillia Unity (CTU) chronology during the Eneolithic in Eastern Europe (after Mantu, 1998; Shukurov et al., 2015), number of total archaeological sites per cultural stage, and number of sites retained to run the ECNM and CTU groups’ abbreviations used in this study.
Ecological Variables
To estimate the eco-cultural niches, we initially used a combination of 10 ecological variables selected based on probable relevance for the CTU site’s location in Eastern Europe. Of these, five are terrain variables: the elevation above the sea level (DEM), terrain slope (Burrough et al., 2015), topographic position index (TPI) (De Reu et al., 2013; Mihu-Pintilie and Nicu, 2019), terrain ruggedness index (TRI) (Riley et al., 1999), and topographic wetness index (TWI) (Moore et al., 1991; Sørensen et al., 2006); and five are WorldClim 1.4, 2022 bioclimatic variables (Hijmans et al., 2005): Bio1/mean annual temperature (MAT), Bio4/temperature seasonality (TS), Bio7/temperature annual range (TAR), Bio12/mean annual precipitation (MAP), and Bio15/precipitation seasonality–coefficient of variation (PScv). Overall, the ecological variables were selected for ECNM for several reasons: first, to include as much as possible the ecological variability in the study area; second, for their applicability for species distribution modeling and their direct affiliation to habitation practice during the Eneolithic; and third, based on their resolution and ease of availability (Whitford, 2019). Therefore, the terrain variables provide information on the physical setting of the area extent concerning both space and suitability for the construction of sedentary settlements during the Eneolithic, and the bioclimatic variables indicate their impacts on the length of the growing season (e.g., changes in weather, ecology, and amount of daylight) and in influencing the hydrological settings (e.g., drought seasonality and floods).
Regarding the spatial database used for ecological variable modeling, the terrain variables are DEM-derived (30 m) rasters from the Shuttle Radar Topographic Mission (SRTM) terrain data (Farr et al., 2007), and the bioclimatic variables represent interpolations of the current climatic conditions projected on global climatic models (GCMs) corresponding to the mid-Holocene (Hijmans et al., 2005) when the CTU in its different phases flourished (Shukurov et al., 2015). In the case of bioclimatic variables, three different mid-Holocene climatic reconstructions based on three different paleoclimate GCMs were used: Community Climate System Model Version 4 (CCSM4) (Gent et al., 2011), Model for Interdisciplinary Research on Climate (MIROC-ESM) (Watanabe et al., 2011), and Max Planck Institute Earth System Model (MPI-ESM-P) (Gutjahr et al., 2019) (Figure 4). Using multiple paleoclimate reconstructions, we were able to account for inter-model variations in the paleoclimate record and not rely only on one model, which increases the overall accuracy of our estimates and improves the interpretation of the results. We obtained the interpolated climatic variables from the WorldClim 1.4, 2022 database (Hijmans et al., 2005; Fick and Hijmans, 2017), widely used in estimating species distribution (Booth et al., 2014). The WorldClim 1.4 database has been previously used to predict the potential geographic ranges and eco-cultural niches of other cultures, showing high accuracy in estimating ecological niches (Banks et al., 2013; Birks et al., 2015; Krauß et al., 2017; Vidal-Cordasco and Nuevo-López, 202; Whitford, 2019). The WorldClim database comprises 19 bioclimatic variables (Booth et al., 2014) characterizing the temperature and precipitation regimes of the studied area from which we have chosen the annually based variables to increase the generality of our predictions and accuracy in interpretation of the results. All the bioclimatic variables were resampled from 30 s or approximately 1 km2 spatial resolution (Gent et al., 2011; Watanabe et al., 2011; Gutjahr et al., 2019) to 654 m2 in order to match the spatial resolution of terrain datasets (Farr et al., 2007) for the ECNM procedure (Table 2).
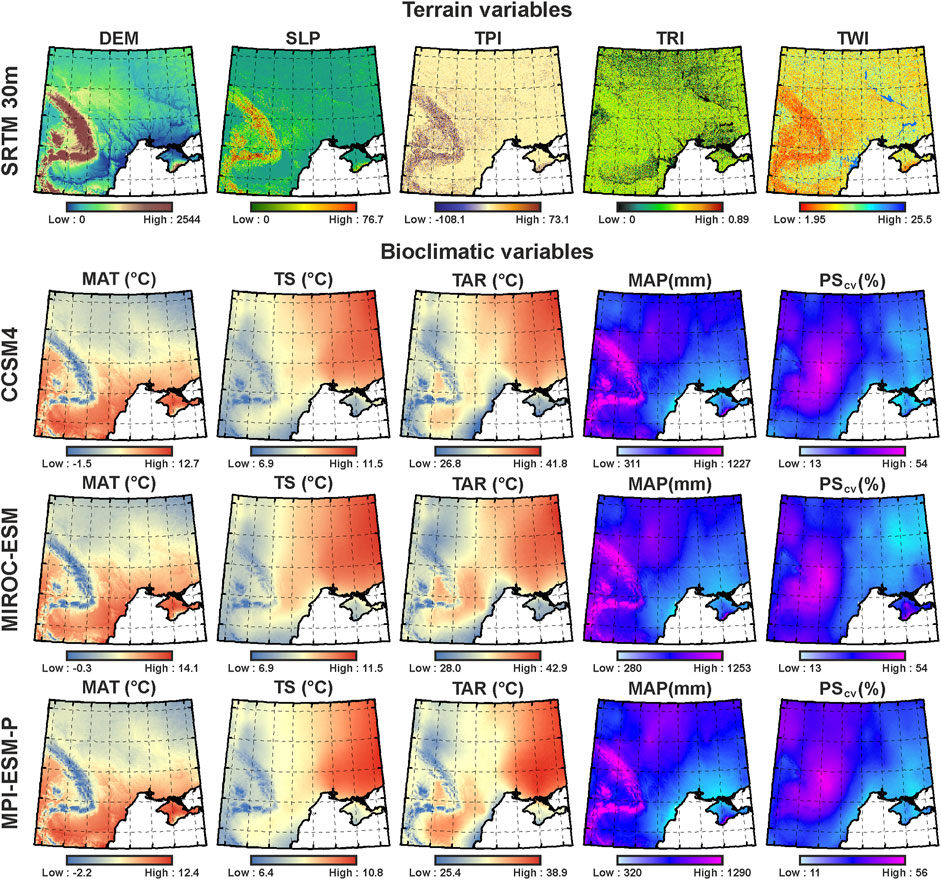
FIGURE 4. Independent ecological variables selected to run the ECNM: terrain variables generated using DEM-derived raster from SRTM 30 m; mid-Holocene bioclimatic variables selected from three global climatic models (CCSM4, MIROC-ESM, and MPI-ESM-P) provided by the WorldClim 1.4 database.
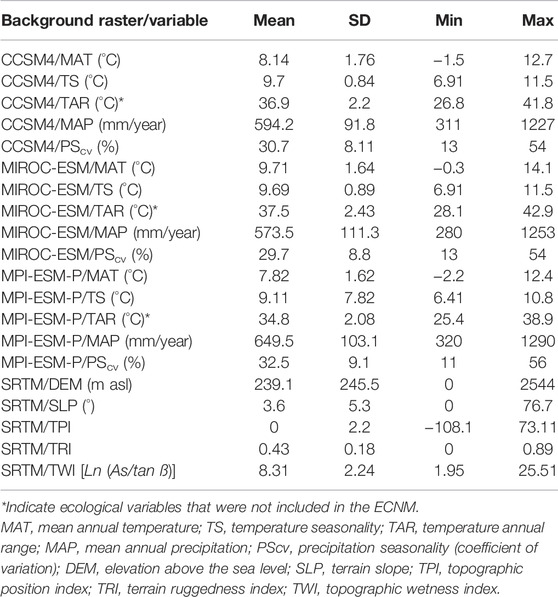
TABLE 2. Summary statistics of the background raster datasets used for ECNM of the CTU and CTU groups.
Since collinearity can induce errors in estimates, we ran Pearson’s correlation coefficient (r2) to identify collinearity among variables, and all the variables with an r2 greater than 0.8 were removed from the models (Table 3). Nine ecological variables were retained to run the ECNM, from which five are based on the local topography (DEM, SLP, TPI, TRI, and TWI) and four on the climatic datasets (MAT, TS, MAP, and PScv).
Eco-Cultural Niche Modeling
To model the eco-cultural niches of the five phases of CTU, we used seven machine learning and regression-based statistical methods used in estimating species ecological niches: the classification tree analysis (CTA), multivariate adaptive regression splines (MARS), generalized additive model (GAM), generalized linear model (GLM), artificial neural network (ANN), generalized boosted regressions model (GBM), and random forest (RF) (Prasad, 2018). Using more than one statistical method to estimate the eco-cultural niches and the geographic distributions of CTU groups, we decreased the errors associated with each statistical tool (Sillero and Barbosa, 2019). Stacking resulted in models produced by different modeling methods, minimizing errors, and increasing results and interpretations’ accuracy. Ten thousand randomly selected background pseudo-absences were used to produce the models. The models were tested independently using 25% of the occurrence data (not used to train the modeling process). Also, the predictive power and accuracy were assessed using a few metrics, including the area under the ROC curve (AUC), the omission error, the proportion of correctly predicted occurrences, and the Kappa Index (Oliveira et al., 2014) (Figure 2). The final model stack was ensemble only for the models that were shown to have a high predictive power by only considering models with an AUC higher than 0.75. We also assessed the percentage contribution of each environmental predictor by using a Pearson correlation coefficient to evaluate the importance of each ecological variable to the ECNMs. The predictions were then exported as probabilities into a GIS, where they were visualized and maps of each prediction were produced in ArcGIS 10.6. All the models were produced and evaluated in the SSDM R package (Schmitt et al., 2017; R Core Team, 2015).
Geographic Ranges and Niches
To study the range shifts in the geographic distribution of different phases of the CTU, we used the relative centroid change to indicate the direction of the range shifts. We calculated all the niche shifts in ArcGIS 10.6. We also compared the eco-cultural niches of the different phases of the CTU by calculating Schoener’s D (Schoener, 1968), Warren I statistics (van der Vaart, 1998; Warren et al., 2008), and relative rank (Warren and Seifert, 2011) for niche overlap and niche breadth. The rank, D, and I niche statistics range from 0 to 1, where 0 shows no overlap and 1 shows total overlap. We calculated all the niche breadth and overlap statistics using the ENMToolbox 1.4.4 (Warren et al., 2008) (Figure 2).
Results and Discussion
Environmental Conditions During the Eneolithic
During the Eneolithic, the Cucuteni–Trypillia groups occupied a latitudinal band between 44°N and 52°N latitude in Eastern Europe, with mean annual temperatures (MAT) ranging between 5.1°C and 12°C and mean annual precipitations (MAP) ranging between 377 mm/year and 810 mm/year. The temperature seasonality (TS) or the standard deviation of the mean monthly temperature indicates variability between 9.41°C and 10.9°C. The precipitation seasonality (PScv) calculated as a coefficient of variation (%) in monthly precipitation totals over the year indicates mean values between 17.3 and 70.5%. The terrain elevation (DEM) varied between 0 m (Black Sea level) and 855 m, and terrain slope (SLP) varied between flat areas and 31°. The topographic position index (TPI), which indicates if the CTU settlement was placed on positive (e.g., hill and ridge) or negative (e.g., valley and depression) landforms, varies between 13.4 and −12.9 (mean 0.29). The terrain ruggedness index (TRI), a hardness indicator of the CTU population’s movement through the study area, varies between 0 and 0.9 (mean 0.46). The topographic wetness index (TRI), commonly used to quantify topographic control on hydrological processes (e.g., floodable areas and arid lands), varies between 4.43 and 20.5 (mean 7.82).
The bioclimatic and terrain datasets were analyzed from one cultural group to another in these environmental conditions depending on the settlement’s spatial distribution inside each cultural phase. Therefore, significant differences in terms of annual and seasonal temperature were observed between the Early Eneolithic (CTU-G1) and first phase of Middle Eneolithic (CTU-G2) groups (cal. 5,400/5,300–4,500/4,400 BCE), where mean annual temperatures (MAT) were +0.23°C degrees higher than the second phase of the Middle/Late Eneolithic period (cal. 4,500/4,400–2,800/2,700 BCE) in which the CTU-G3, CTU-G4, and CTU-G5 groups flourished. These thermal characteristics are also observed in the case of temperature seasonality (TS) which increased with +0.13°C by comparing the CTU-G1 and CTU-G2 conditions with the last three CTU phases. However, the general thermal trends were the decrease in annual mean temperature and a slight increase in seasonal temperature variation from the first phase of the CTU culture to the last one. This phenomenon can be explained by the expansion of CTU populations from the west to the northeast, where the occupied steppe region (Black Sea lowland and southern Bug–Dnieper upper basins) showed more significant temperature variations than the Eastern Carpathian lowlands. According to the mean annual precipitation (MAP), the rainiest period was during the Early-to-Middle Eneolithic, when the maximum amount of precipitation ranged between 732 and 810 mm/year. Also, the precipitation seasonality (PScv) indicates that the beginning of the Late Eneolithic or during the Cucuteni B (1–3)/Trypillia BII + CI phase (CTU-G4) was the time interval with the most significant difference in precipitation amount (59.9% coefficient of variation). Overall, the mean amount of precipitation during the Eneolithic was around 600 mm/year, and the precipitation seasonality in terms of the coefficient of variation was 44.5%.
The climatic conditions are closely related to the terrain variable characteristics, and several differences in CTU habitation practices during the Eneolithic have been observed. Therefore, the mean elevation of CTU sites decreases from the Early/Middle Eneolithic to Late Eneolithic period, respectively, from 200.5 m (CTU-G1) and 254 m (CTU-G2) to 158.3 m (CTU-G5). This trend was determined by the migration from the hilly region in the Eastern Carpathian lowland (Siret, Prut, and upper Dniester basins) to the Southern Bug and Dnieper’s lower areas as well to the Black Sea lowlands (Figure 3). The largest elevation difference (848 m) between CTU sites occurred during the cal. 4,800/4,700–4,500/4,400 BCE (CTU-G2) due to the Ariuşd phase (Cucuteni A), which migrated to the west and occupied the intra-Carpathian depressions. However, the upper limit of CTU sites’ altitude did not exceed 600 m than in a few isolated cases referring here to the sub-Carpathian and intra-Carpathian sites. Regarding the terrain slope of CTU settlements, the average values ranging between 3.51° and 4.6° indicate that there are no significant differences from one cultural stage to another and attest that suitable habitat areas have not changed over time. These terrain characteristics also result from the mean values of the topographic position index (TPI), which is positive for all the CTU groups (0.07–0.44), from the mean values of the topographic ruggedness index (TRI), which is constant at around 0.47 value, and from the mean values of topographic wetness index (TWI), which slightly ranges between 7.6 and 8.12 (Table 4).
Ecological Variables’ Performance and Contributions for Eco-Cultural Niche Modeling
The eco-cultural niche models were produced in the SSDM R package, Maxent, and ENMToolbox 1.4.4 using the optimum combination of modeling parameters described earlier (Figure 2). First, one model each was produced from the CTU/CCSM4, CTU/MIROC-ESM, and CTU/MPI-ESM-P datasets combined with DEM-derived terrain variables in order to test the performance of global climatic models (GCMs) (Baker and Taylor, 2016) for ECNM (Figure 5). The models’ performance provided in Table 5 indicate that there are no significant differences (AUC: 0.78–0.79; omission rate: 0.21–0.22; proportionally correct: 0.78–0.79; and Kappa: 0.54–0.55) between GCMs used to run ECNM for the entire Cucuteni–Trypillia culture. The same conclusion results from the analysis of ecological variable contributions where the bioclimatic variables (MAT, TS, MAP, and PScv) have relatively similar percentages (±3.59%) in each model obtained by run ECNM procedure: CTU/CCSM4—77.29%; CTU/MIROC-ESM—75.95%; and CTU/MPI-ESM-P—73.7% (Figure 6). However, by choosing to account for inter-model variations in the paleoclimate record, the construction quality and interpretation improved (Baker and Taylor, 2016).
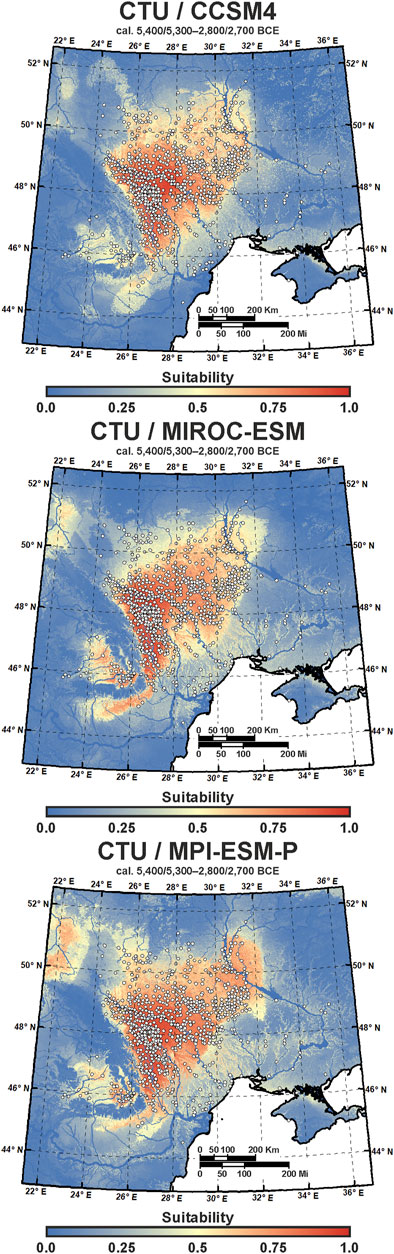
FIGURE 5. ECNM reconstructions of CTU (cal. 5,400/5,300–2,800/2,700 BCE) using terrain variables and mid-Holocene CCSM4, MIROC-ESM, and MPI-ESM-P bioclimatic variables; colors range from blue (0) to yellow (0.5) and to red (1), or low (0) to high (1) suitability, respectively; the white dots represent CTU sites used for niche reconstruction.

TABLE 5. Model performance of CTU niches reconstructed using bioclimatic variables provided by CCSM4, MIROC-ESM, MPI-ESM-P, and DEM-derived terrain variables.
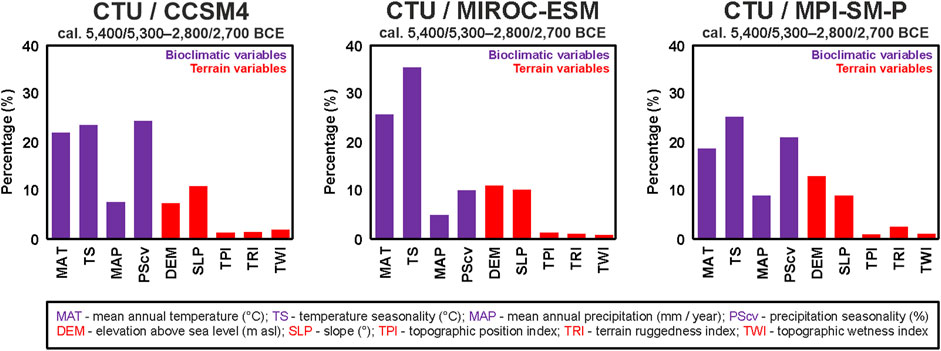
FIGURE 6. Variable contributions to CTU niches reconstructed using bioclimatic variables derived from CCSM4, MIROC-ESM, and MPI-ESM-P global climatic models (GCM) and DEM-derived terrain variables.
To generate the final eco-cultural niche models for each CTU group (CTU-G1—CTU-G5) and for the entire CTU period, the average values of bioclimatic variables obtained from each GCM were used together with all the selected terrain variables (Figure 7). The model’s performance assessment provided in Table 6 using four different metrics (AUC, omission rate, proportionally correct, and Kappa index) indicates high predictive power and accuracy for each resulting ECNM: AUC between 0.78 and 0.84, omission rate between 0.16 and 0.22, proportionally correct between 0.78 and 0.83, and Kappa between 0.47 and 0.58. Regarding ecological variable contributions to eco-cultural niche models provided for each CTU group, bioclimatic conditions dominate: CTU-G1—88.34%, CTU-G2—82.5%, CTU-G3—77.02%, CTU-G4—78.04%, and CTU-G5—74.85% (CTU—75.65%). Within the bioclimatic variables, the variation of the precipitation seasonality coefficient (PSCV) has the most significant contributions to ECNM in the case of first three cultural phases (36.55–55.3%), the mean annual temperature (MAT 24.5%) in the case of the ECN model of CTU-G4, and the temperature seasonality (TS 41.15%) in the case of the ECN model of the last CTU phase (CTU-G5). The overall analysis of the variable contributions for the entire culture (CTU) niche indicates a relative balance among MAT (22.07%), TS (28.02%), and PSCV (18.43%) and a significant increase in elevation (DEM—10.41%) and terrain slope (SLP—9.95%) contributions to ECNM compared to each CTU group models (Figure 8). Accordingly, the answer to the first question stated at the end of the Introduction section of this study is affirmative: yes, the heterogeneous ecological characteristics in Eastern Europe play a significant role in the evolutionary process of each successive phase of the CTU, but the bioclimatic variables were more important for habitation suitability than terrain variables during the Eneolithic.
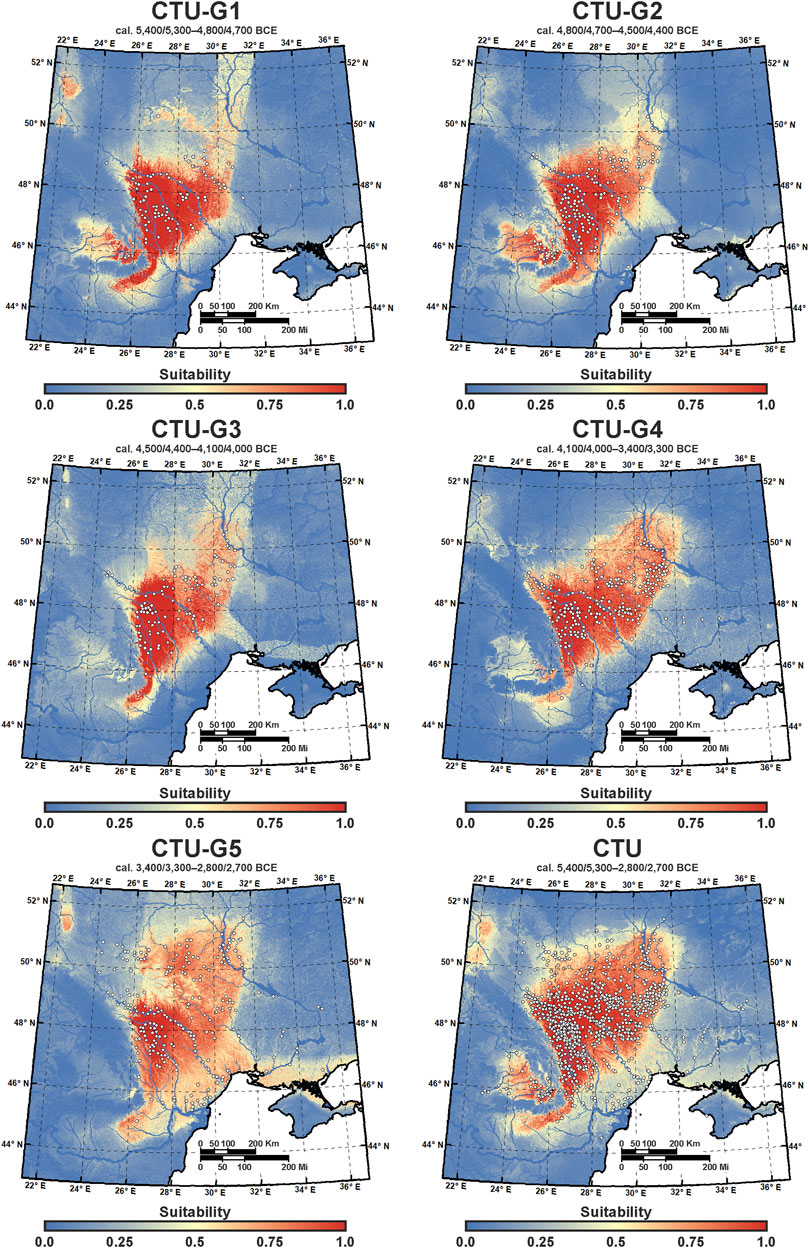
FIGURE 7. ECNM reconstructions of CTU groups (see Table 1) and for the entire CTU culture (cal. 5,400/5,300–2,800/2,700 BCE); colors range from blue (0) to yellow (0.5) and to red (1), or low (0%) to middle (50%) and to high (100%) suitability, respectively; the white dots represent the archaeological sites.
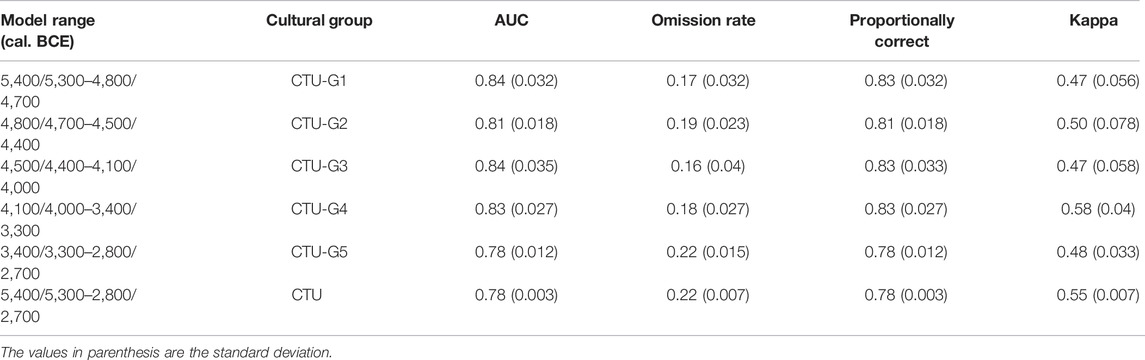
TABLE 6. Model performance of CTU groups and CTU niches reconstructed using average values of bioclimatic variables provided by CCSM4, MIROC-ESM, and MPI-ESM-P, and DEM-derived terrain variables.
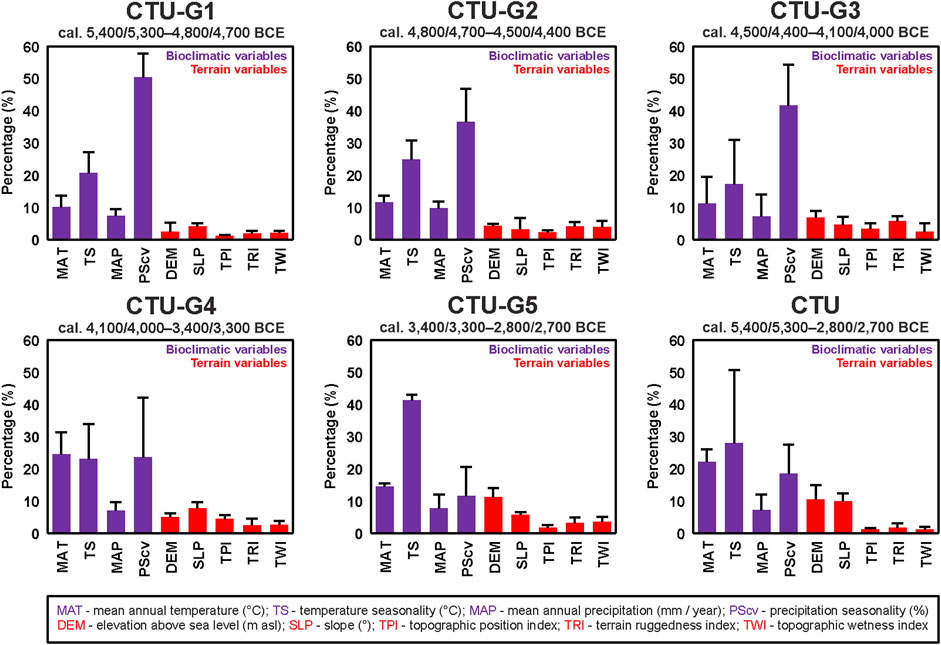
FIGURE 8. Variable contributions to CTU and CTU groups’ niches reconstructed using GCM-derived bioclimatic variables (see Figure 5) and DEM-derived terrain variables.
Eco-Cultural Niche Modeling Breadth and Overlaps Between Cucuteni–Trypillia Cultural Unity Groups
As we showed before, the AUC values indicate the significant predictive ability (Table 6) of the CTU groups’ ECNM (Figure 7). Although Late Eneolithic groups (CTU-G4 and CTU-G5) have more extended areas with high ecological suitability than Early-to-Middle Eneolithic groups (CTU-G1, CTU-G2, and CTU-G3), the ECNM’s predictions yield similar geographic patterns for the entire Cucuteni–Trypillia culture. In other words, the highest niche probability areas for CTU groups are observed in the same regions: 1) along the Eastern Carpathian lowland (Moldavian Plateau–Podillia Upland), particularly in the upper Siret, Prut, and Dniester basins; 2) in the Middle Prut–Dniester–Southern Bug interfluves up to the Volyn Polissya forest zone; and 3) in the Dnieper Upland (Middle Dnieper basin) (see Figure 1). Similarly, the outside of these areas, respectively, Black Sea lowland, Danubian Plain, Transylvanian Plateau, and Volyn Polissya forest zone, show a lower niche probability for all the CTU groups, except for the Transylvanian Plateau, where the niche probabilities are higher for Early Eneolithic groups (CTU-G1 and CTU-G2) than for Middle-to-Late Eneolithic groups (CTU-G3, CTU-G4, and CTU-G5). These outcomes indicate that CTU groups generally preferred lowland areas and wide river valleys instead of higher areas for their niche selection, with slightly ecological niche breadth between them (±0.01 inverse concentration; ± 0.15 uncertainty) (Table 7).

TABLE 7. Niche breadth statistics (after Levins, 1968; Whitford, 2019).
Statistical analysis of the niche overlap between the successive Cucuteni–Trypillia groups during the Eneolithic reveals that the population did not occupy exclusive ECNM (Table 8). Although CTU-G1 and CTU-G2 groups (cal. 5,400/5,300–4,500/4,400 BCE) have a geographic distribution that includes a broader range of terrain conditions (e.g., elevation and terrain slope) compared with CTU-G3, CTU-G4, and CTU-G5 groups (cal. 4,500/4,400–2,800/2,700 BCE), there is a significant overlap in their ECNMs: Schoener’s D between 0.728 and 0.798; I statistic between 0.932 and 0.962; and rank between 0.644 and 0.82. More than that, by analysis of the niche overlaps between the ECNM of the entire Cucuteni–Trypillia culture with the ECN models of each CTU group, the results also indicate significant overlapping: Schoener’s D between 0.726 and 0.794; I statistic between 0.933 and 0.96; and rank between 0.724 and 0.82 (Table 9). Accordingly, the answer to the second question is stated at the end of the Introduction section in connection with the fact that the particular trails of the successive CTU groups can be correlated with the modification of habitation practices and that the subsequent occupation of other territories is partially affirmative due to the characteristics of the niche. Therefore, the ECNMs’ breadth and overlap results indicate a close connection between habitation practices and human migrations to the other territories but as a consequence of cultural evolution (e.g., population growth) and not as the one related to environmental conditions. We supported this statement because the CTU groups did not have exclusive eco-cultural niches, and the main difference between them was found just in their ECNM breadths and not in their overlap.
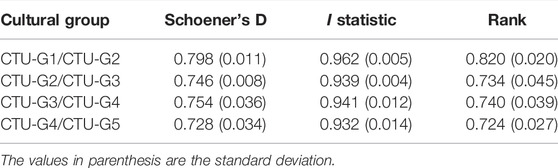
TABLE 8. Schoener’s D, I statistic, and relative rank niche overlap statistics (0 = no overlap; 1 = perfect overlap) for consecutive CTU groups.
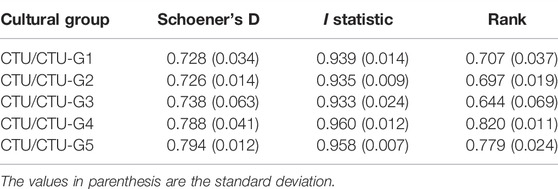
TABLE 9. Schoener’s D, I statistic, and relative rank niche overlap statistics (0 = no overlap; 1 = perfect overlap) between the CTU model and each cultural group.
Chronological Considerations and Limitation to Eco-Cultural Niche Modeling
The ECNM methodology, which was adopted by archeologists and from the bio-computational architecture to explore the interactions between cultural and natural systems (Banks, 2017), offers a high potential to understand how and when the environmental conditions and ecological dynamics influenced adaptation and movement of prehistoric populations through the inhabited territories (Banks et al., 2008; Banks et al., 2009; d'Errico and Banks, 2013; Vidal-Cordasco and Nuevo-López, 2021; Whitford, 2019). The outcomes obtained in this work reveal that the eco-cultural niche of all the CTU groups significantly overlapped. However, the first three cultural phases from Early-to-Middle Eneolithic (CTU-G1, CTU-G2, and CTU-G3) had slightly more restricted ecological niches than prehistoric populations of the last two CTU groups from Late Eneolithic (CTU-G4 and CTU-G5). Consequently, the population which belonged to the Precucuteni I, II, and III/Trypillia A (1–2), Cucuteni A (1–4)/Trypillia BI, and Cucuteni A–B (1–2)/Trypillia BI/BII cultural stages had higher probabilities of occupying cooler and drier environments like the sub-Carpathians or inner landscapes of the Eastern Carpathians. Conversely, the population which belonged to the Cucuteni B (1–3)/Trypillia BII + CI and Erbiceni/Trypillia CII-γII groups had ecological niches that were more restricted to Eastern Carpathian areas. However, there is a higher probability of occupying the eastern steppe territories between the Middle Dnieper basin and Black Sea lowlands (Figure 7). These results have significant implications for understanding the geographical range and distribution of the last great Chalcolithic society of Old Europe (Chapman et al., 2019).
Overall, according to the large number of CTU sites discovered in Eastern Europe, it might be suggested that the populations belonging to different Eneolithic groups could have adapted to different ecological settings. However, the results obtained in this study suggest that CTU groups did not occupy exclusive ecological niches and that the difference in their geographic distribution is rather a consequence of their ecological niche breadths. Accordingly, although the most suitable areas overlap with all the CTU groups, respectively, Siret, Prut, and Dniester watersheds, the broader ecological niche breadth of the last two CTU groups probably made it easier for them to occupy large territories, including Southern Bug and Dniester watersheds. Therefore, the increase in population during the Late Eneolithic period probably allowed the expansion of the ecological niches and reduced their vulnerability to changes in the ecosystems. On the other hand, the larger niche breadth of the CTU-G5 group may be essential to understanding the transition of the Cucuteni–Trypillia culture to the Early Bronze Age (EBA).
The ECNM methodology used in this approach has some limitations that should be explored in future studies. We treated Cucuteni–Trypillia phases as a uniform culture in Eastern Europe, but there were some differences, especially among the Ariuşd, Cucuteni, Eastern Tripolye (ETC) groups, and Western Tripolye (WTC) groups. Therefore, it would be helpful for future works to expand this research by focusing on more specific geographic regions for these two cultural stages and comparing the resulting niches. Also, it should be considered that despite ECNM constituting a well-established approach, all the reconstructed niches are partial because they must focus only on a limited number of ecological variables. It would be desirable that future works based on ECNM methodology compare reconstructed niches presented here with the distribution models of some vegetation or animal species consumed by Eneolithic groups.
Conclusion
In this study, we applied for the first time the ECNM methodology to explore the socio-ecological dynamics inside the Cucuteni–Trypillia culture (CTU), which flourished in Eastern Europe during the Eneolithic (cal. 5,400/5,300–2,800/2,700 BCE). The interpretation of the eco-cultural niche breadth and overlaps of the successive phases has provided valuable insight into the relationship between the culture (site distributions) and ecology (bioclimatic and terrain variables) and its impacts on the cultural development process. Therefore, the ECNM significantly overlapped, and the expansion trend of the last two CTU groups (Late Eneolithic—cal. 4,100/4,000–2,800/2,700 BCE) into the northeastern steppe regions or even to the Black Sea lowland was not due to ecological niche differences but rather a result of other cultural factors (e.g., population growth, subsistence agriculture, and mixed with other cultures). However, the first three CTU groups (Early and Middle Eneolithic—cal. 5,400/5,300–4,100/4,000 BCE) had more restricted ecological niches than the last two groups (Last Eneolithic). In other words, the broader ecological niches of the last CTU groups probably allowed them to occupy large steppe territories and exclude the inner areas of Eastern Carpathians, whereas the narrower eco-cultural niche breadth of the first CTU groups made the prehistoric population more vulnerable to the climatic changes (e.g., temperature seasonality variation) that affect the northeastern steppe ecosystems. These results have significant implications for understanding the geographical range and distribution of the last great Chalcolithic society of Old Europe and contribute to the characterization of ecological niches they have exploited during the cultural evolutionary process.
Data Availability Statement
The original contributions presented in the study are included in the article/supplementary material; further inquiries can be directed to the corresponding author.
Author Contributions
All the authors have equal contributions in conceptualization, methodology and formal analysis, data validation, investigation, data curation, writing, original draft preparation, and reviewing and editing of the manuscript.
Funding
Authors are thankful to Romanian Ministry of Research, Innovation and Digitization, within Program 1—Development of the national RD system, Subprogram 1.2—Institutional Performance—RDI excellence funding projects, Contract no. 11PFE/30.12.2021, for financial support.
Conflict of Interest
The authors declare that the research was conducted in the absence of any commercial or financial relationships that could be construed as a potential conflict of interest.
Publisher’s Note
All claims expressed in this article are solely those of the authors and do not necessarily represent those of their affiliated organizations, or those of the publisher, the editors, and the reviewers. Any product that may be evaluated in this article, or claim that may be made by its manufacturer, is not guaranteed or endorsed by the publisher.
Acknowledgments
All the data were processed in the Geoarchaeology Laboratory (Coordinator: AM-P) of the Institute of Interdisciplinary Research, Department of Exact and Natural Sciences, “Alexandru Ioan Cuza” University of Iaşi (UAIC), Romania. Ryan Martin (Case Western Reserve University, Cleveland, Ohio) is kindly acknowledged for the English language editing of the manuscript. Our gratitude goes to the reviewers who helped us in improving the manuscript.
References
Anderson, R. P., and Gonzalez, I. (2011). Species-specific Tuning Increases Robustness to Sampling Bias in Models of Species Distributions: an Implementation with Maxent. Ecol. Model. 222 (15), 2796–2811. doi:10.1016/j.ecolmodel.2011.04.011
Anderson, R. P., and Raza, A. (2010). The Effect of the Extent of the Study Region on GIS Models of Species Geographic Distributions and Estimates of Niche Evolution: Preliminary Tests with Montane Rodents (Genus Nephelomys) in Venezuela. J. Biogeogr. 37 (7), 1378–1393. doi:10.1111/j.1365-2699.2010.02290.x
Argyriou, A. V., Teeuw, R. M., and Sarris, A. (2017). GIS-based Landform Classification of Bronze Age Archaeological Sites on Crete Island. PLoS ONE 12, e0170727. doi:10.1371/journal.pone.0170727
Baker, N. C., and Taylor, P. C. (2016). A Framework for Evaluating Climate Model Performance Metrics. J. Clim. 29 (5), 1773–1782. doi:10.1175/JCLI-D-15-0114.1
Banks, W. E., Aubry, T., d’Errico, F., Zilhão, J., Lira-Noriega, A., and Townsend Peterson, A. (2011). Eco-cultural Niches of the Badegoulian: Unraveling Links between Cultural Adaptation and Ecology during the Last Glacial Maximum in France. J. Anthropol. Archaeol. 30 (3), 359–374. doi:10.1016/j.jaa.2011.05.003
Banks, W. E., d'Errico, F., Dibble, H. L., Krishtalka, L., West, D., Olszewski, D., et al. (2006). Eco-Cultural Niche Modeling: New Tools for Reconstructing the Geography and Ecology of Past Human Populations. PalaeoAnthropology 4 (6), 68–83.
Banks, W. E., d'Errico, F., Peterson, A. T., Vanhaeren, M., Kageyama, M., Sepulchre, P., et al. (2008). Human Ecological Niches and Ranges during the LGM in Europe Derived from an Application of Eco-Cultural Niche Modeling. J. Archaeol. Sci. 35 (2), 481–491. doi:10.1016/j.jas.2007.05.011
Banks, W. E., d'Errico, F., and Zilhão, J. (2013). Human-climate Interaction during the Early Upper Paleolithic: Testing the Hypothesis of an Adaptive Shift between the Proto-Aurignacian and the Early Aurignacian. J. Hum. Evol. 64 (1), 39–55. doi:10.1016/j.jhevol.2012.10.001
Banks, W. E. (2017). The Application of Ecological Niche Modeling Methods to Archaeological Data in Order to Examine Culture-Environment Relationships and Cultural Trajectories. Quaternaire 28 (2), 271–276. doi:10.4000/quaternaire.7966
Banks, W. E., Zilhão, J., d'Errico, F., Kageyama, M., Sima, A., and Ronchitelli, A. (2009). Investigating Links between Ecology and Bifacial Tool Types in Western Europe during the Last Glacial Maximum. J. Archaeol. Sci. 36 (12), 2853–2867. doi:10.1016/j.jas.2009.09.014
Birks, H. H., Gelorini, V., Robinson, E., and Hoek, W. Z. (2015). Impacts of Palaeoclimate Change 60 000-8000 Years Ago on Humans and Their Environments in Europe: Integrating Palaeoenvironmental and Archaeological Data. Quat. Int. 378, 4–13. doi:10.1016/j.quaint.2014.02.022
Bobrowski, M., Bechtel, B., Böhner, J., Oldeland, J., Weidinger, J., and Schickhoff, U. (2018). Application of Thermal and Phenological Land Surface Parameters for Improving Ecological Niche Models of Betula Utilis in the Himalayan Region. Remote Sens. 10, 6814. doi:10.3390/rs10060814
Booth, T. H., Nix, H. A., Busby, J. R., and Hutchinson, M. F. (2014). Bioclim: the First Species Distribution Modelling Package, its Early Applications and Relevance to Most currentMaxEntstudies. Divers. Distrib. 20, 1–9. doi:10.1111/ddi.12144
Boria, R. A., Olson, L. E., Goodman, S. M., and Anderson, R. P. (2014). Spatial Filtering to Reduce Sampling Bias Can Improve the Performance of Ecological Niche Models. Ecol. Model. 275, 73–77. doi:10.1016/j.ecolmodel.2013.12.012
Brigand, R., and Weller, O. (2018). Neo-Eneolithic Settlement Pattern and Salt Exploitation in Romanian Moldavia. J. Archaeol. Sci. Rep. 17, 68–78. doi:10.1016/j.jasrep.2017.10.032
Brigand, R., and Weller, O. (2013). Neolithic and Chalcolithic Settlement Patterns in Central Moldavia (Romania). Doc. Praeh. 40, 195–207. doi:10.4312/dp.40.15
Burrough, P. A., McDonnell, R. A., and Lloyd, C. D. (2015). Principles of Geographical Information Systems –. third edition. Oxford: Oxford University Press.
Chapman, J. C., Videiko, M. Y., Gaydarska, B., Burdo, N., and Hale, D. (2014). Architectural Differentiation on a Trypillia Mega-Site: Preliminary Report on the Excavation of a Mega-Structure at Nebelivka, Ukraine. J. Neolit. Archaeol. 16, 135–157. doi:10.12766/jna.2014.4
Chapman, J., Gaydarska, B., and Nebbia, M. (2019). The Origins of Trypillia Megasites. Front. Digit. Humanit. 6, 10. doi:10.3389/fdigh.2019.00010
de Oliveira, G., Rangel, T. F., Lima-Ribeiro, M. S., Terribile, L. C., and Diniz-Filho, J. A. F. (2014). Evaluating, Partitioning, and Mapping the Spatial Autocorrelation Component in Ecological Niche Modeling: A New Approach Based on Environmentally Equidistant Records. Ecography 37 (7), 637–647. doi:10.1111/j.1600-0587.2013.00564.x
De Reu, J., Bourgeois, J., Bats, M., Zwertvaegher, A., Gelorini, V., De Smedt, P., et al. (2013). Application of the Topographic Position Index to Heterogeneous Landscapes. Geomorphology 186, 39–49. doi:10.1016/j.geomorph.2012.12.015
d’Errico, F., and Banks, W. E. (2013). Identifying Mechanisms behind Middle Paleolithic and Middle Stone Age Cultural Trajectories. Curr. Anthropol. 54 (S8), S371–S387. doi:10.1086/673388
Diachenko, A., and Menotti, F. (2012). The Gravity Model: Monitoring the Formation and Development of the Tripolye Culture Giant-Settlements in Ukraine. J. Archaeol. Sci. 39, 2810–2817. doi:10.1016/j.jas.2012.04.025
Elith, J., Kearney, M., and Phillips, S. (2010). The Art of Modelling Range-Shifting Species. Methods Ecol. Evol. 1 (4), 330–342. doi:10.1111/j.2041-210X.2010.00036.x
Farr, T. G., Rosen, P. A., Caro, E., Crippen, R., Duren, R., Hensley, S., et al. (2007). The Shuttle Radar Topography Mission. Rev. Geophys. 45, RG2004. doi:10.1029/2005RG000183
Fick, S. E., and Hijmans, R. J. (2017). WorldClim 2: New 1‐km Spatial Resolution Climate Surfaces for Global Land Areas. Int. J. Climatol. 37 (12), 4302–4315. doi:10.1002/joc.5086
Gent, P. R., Danabasoglu, G., Donner, L. J., Holland, M. M., Hunke, E. C., Jayne, S. R., et al. (2011). The Community Climate System Model Version 4. J. Clim. 24 (19), 4973–4991. doi:10.1175/2011JCLI4083.1
Gherghel, I., Brischoux, F., and Papeş, M. (2019). Refining Model Estimates of Potential Species' Distributions to Relevant Accessible Areas. Prog. Phys. Geogr. Earth Environ. 44 (10), 449–460. doi:10.1177/0309133319881104
Gutjahr, O., Putrasahan, D., Lohmann, K., Jungclaus, J. H., von Storch, J.-S., Brüggemann, N., et al. (2019). Max Planck Institute Earth System Model (MPI-ESM1.2) for the High-Resolution Model Intercomparison Project (HighResMIP). Geosci. Model. Dev. 12, 3241–3281. doi:10.5194/gmd-12-3241-2019
Harper, T. K., Diachenko, A., Rassamakin, Y. Y., and Kennett, D. J. (2019). Ecological Dimensions of Population Dynamics and Subsistence in Neo-Eneolithic Eastern Europe. J. Anthropol. Archaeol. 53, 92–101. doi:10.1016/j.jaa.2018.11.006
Hijmans, R. J., Cameron, S. E., Parra, J. L., Jones, P. G., and Jarvis, A. (2005). Very High Resolution Interpolated Climate Surfaces for Global Land Areas. Int. J. Climatol. 25, 1965–1978. doi:10.1002/joc.1276
Ivanova, M., De Cupere, B., Ethier, J., and Marinova, E. (2018). Pioneer Farming in Southeast Europe during the Early Sixth Millennium BC: Climate-Related Adaptations in the Exploitation of Plants and Animals. PLoS One 13 (5), e0197225. doi:10.1371/journal.pone.0197225
Krauß, R., Marinova, E., De Brue, H., and Weninger, B. (2018). The Rapid Spread of Early Farming from the Aegean into the Balkans via the Sub-mediterranean-aegean Vegetation Zone. Quat. Int. 496, 24–41. doi:10.1016/j.quaint.2017.01.019
Lemmen, C., Gronenborn, D., and Wirtz, K. W. (2011). A Simulation of the Neolithic Transition in Western Eurasia. J. Archaeol. Sci. 38 (12), 3459–3470. doi:10.1016/j.jas.2011.08.008
Mantu, C.-M. (1998). Cultura Cucuteni. Evolutie, Cronologie, Legaturi. Piatra Neamt: Centrul Internaional de Cercetare a culturii Cucuteni.
Mihu-Pintilie, A., and Nicu, I. C. (2019). GIS-based Landform Classification of Eneoli Thic Archaeological Sites in the Plateau-Plain Transition Zone (NE Romania): Habitation Practices vs. Flood Hazard Perception. Remote Sens. 118, 915. doi:10.3390/rs11080915
Monah, D., and Monah, F. (1997). “The Last Great Chalcolithic Civilization of Old Europe,” in Cucuteni. The Last Great Chalcolithic Civilization of Europe. Editors C.-M. Mantu, G. Dumitroaia, and A. Tsaravopoulos (Thessaloniki: Athena Printing House), 15–95.
Moore, I. D., Grayson, R. B., and Ladson, A. R. (1991). Digital Terrain Modelling: A Review of Hydrological, Geomorphological, and Biological Applications. Hydrol. Process. 5, 3–30. doi:10.1002/hyp.3360050103
Nikitin, A., Sokhatsky, M. P., Sokhatsky, M., Kovaliukh, M., and Videiko, M. (2010). Comprehensive Site Chronology and Ancient Mitochondrial DNAAnalysis from Verteba Cave - a Trypillian Culture Site of Eneolithic Ukraine. Iansa I (1–2), 9–18. doi:10.24916/iansa.2010.1-2.1
Noviello, M., Cafarelli, B., Calculli, C., Sarris, A., and Mairota, P. (2018). Investigating the Distribution of Archaeological Sites: Multiparametric vs Probability Models and Potentials for Remote Sensing Data. Appl. Geogr. 95, 34–44. doi:10.1016/j.apgeog.2018.04.005
Peterson, A. T., Soberón, J., Pearson, R. G., Anderson, R. P., Martínez-Meyer, E., Nakamura, M., et al. (2011). Ecological Niches and Geographic Distributions. Princeton: Princeton University Press.
Pétillon, J.-M., Laroulandie, V., Costamagno, S., and Langlais, M. (2016). Testing Environmental Determinants in the Cultural Evolution of Hunter-Gatherers: a Three-Year Multidisciplinary Project on the Occupation of the Western Aquitaine Basin during the Middle and Upper Magdalenian (19-14 Kyr Cal BP). Quat. Int. 414, 1–8. doi:10.1016/j.quaint.2016.04.035
Phillips, S. J., Anderson, R. P., and Schapire, R. E. (2006). Maximum Entropy Modeling of Species Geographic Distributions. Ecol. Model. 190 (3–4), 231–259. doi:10.1016/j.ecolmodel.2005.03.026
Phillips, S. J., and Dudík, M. (2008). Modeling of Species Distributions with Maxent: New Extensions and a Comprehensive Evaluation. Ecography 31 (2), 161–175. doi:10.1111/j.0906-7590.2008.5203.x
Prasad, A. M. (2018). “Machine Learning for Macroscale Ecological Niche Modeling - a Multi-Model, Multi-Response Ensemble Technique for Tree Species Management under Climate Change,” in Machine Learning for Ecology and Sustainable Natural Resource Management. Editors G. Humphries, D. Magness, and F. Huettmann (Cham: Springer), 123–139. doi:10.1007/978-3-319-96978-7_6
R Core Team (2015). R: A Language and Environment for Statistical Computing. Vienna, Austria: R Foundation for Statistical Computing. http://www.R-project.org/(Accessed March 1, 2022).
Riley, S. J., DeGloria, S. D., and Elliot, R. (1999). A Terrain Ruggedness Index that Quantifies Topographic Heterogeneity. Int. J. Sci. 5, 23–27.
Schmitt, S., Pouteau, R., Justeau, D., Boissieu, F., and Birnbaum, P. (2017). Ssdm : An R Package to Predict Distribution of Species Richness and Composition Based on Stacked Species Distribution Models. Methods Ecol. Evol. 8, 1795–1803. doi:10.1111/2041-210X.12841
Schoener, T. W. (1968). The Anolis Lizards of Bimini: Resource Partitioning in a Complex Fauna. Ecology 49 (4), 704–726. doi:10.2307/1935534
Shukurov, A., Sarson, G., Videiko, M., Henderson, K., Shiel, R., Dolukhanov, P., et al. (2015). Productivity of Premodern Agriculture in the Cucuteni-Trypillia Area. Hum. Biol. 87 (3), 235–282. doi:10.13110/humanbiology.87.3.0235
Sillero, N., and Barbosa, A. M. (2021). Common Mistakes in Ecological Niche Models. Int. J. Geogr. Inf. Sci. 35 (2), 213–226. doi:10.1080/13658816.2020.1798968
Soberon, J., and Peterson, A. T. (2005). Interpretation of Models of Fundamental Ecological Niches and Species' Distributional Areas. Biodiv. Inf. 2, 1–10. doi:10.17161/bi.v2i0.4
Sørensen, R., Zinko, U., and Seibert, J. (2006). On the Calculation of the Topographic Wetness Index: Evaluation of Different Methods Based on Field Observations. Hydrol. Earth Syst. Sci. 10, 101–112. doi:10.5194/hess-10-101-2006
Tallavaara, M., Luoto, M., Korhonen, N., Järvinen, H., and Seppä, H. (2015). Human Population Dynamics in Europe over the Last Glacial Maximum. Proc. Natl. Acad. Sci. U.S.A. 112 (27), 8232–8237. doi:10.1073/pnas.1503784112
Tapete, D., and Cigna, F. (2019). COSMO-SkyMed SAR for Detection and Monitoring of Archaeological and Cultural Heritage Sites. Remote Sens. 1111, 1326. doi:10.3390/rs11111326
Vidal-Cordasco, M., and Nuevo-López, A. (2021). Difference in Ecological Niche Breadth between Mesolithic and Early Neolithic Groups in Iberia. J. Archaeol. Sci. Rep. 35, 102728. doi:10.1016/j.jasrep.2020.102728
Warren, D. L., Glor, R. E., Turelli, M., and Funk, D. (2008). Environmental Niche Equivalency versus Conservatism: Quantitative Approaches to Niche Evolution. Evolution 62 (11), 2868–2883. doi:10.1111/j.1558-5646.2008.00482.x
Warren, D. L., and Seifert, S. N. (2011). Ecological Niche Modeling in Maxent: the Importance of Model Complexity and the Performance of Model Selection Criteria. Ecol. Appl. 21 (2), 335–342. doi:10.1890/10-1171.1
Watanabe, S., Hajima, T., Sudo, K., Nagashima, T., Takemura, T., Okajima, H., et al. (2011). MIROC-ESM 2010: Model Description and Basic Results of CMIP5-20c3m Experiments. Geosci. Model. Dev. 4, 845–872. doi:10.5194/gmd-4-845-2011
Whitford, B. R. (2019). Characterizing the Cultural Evolutionary Process from Eco-Cultural Niche Models: Niche Construction during the Neolithic of the Struma River Valley (C. 6200-4900 BC). Archaeol. Anthropol. Sci. 11, 2181–2200. doi:10.1007/s12520-018-0667-x
WorldClim 1.4 (2022). Global Paleoclimate Data, Free Climate Data for Ecological Modeling and GIS. Availabe at: http://www.worldclim.com/paleo-climate1 (Accessed March 3, 2022).
Glossary
GIS Geographic Information System
ECNM eco-cultural niche modeling
ENM ecological niche modeling
NCT niche construction theory
BCE before common era
CTU Cucuteni–Trypillia cultural unity
CTU-G1 Group 1: Precucuteni I, II, III/Trypillia A (Early Eneolithic)
CTU-G2 Group 2: Cucuteni A (1–4)/Trypillia BI (Middle Eneolithic)
CTU-G3 Group 3: Cucuteni A-B (1–2)/Trypillia BI/BII (Middle Eneolithic)
CTU-G4 Group 4: Cucuteni B (1–3)/Trypillia BII + CI (Late Eneolithic)
CTU-G5 Group 5: Trypillia CII-γII (Late Eneolithic)
EENSR Eastern European Neo-Eneolithic Sites Repository
RPTK Register of Tripoli Culture Monuments–Ukraine
RMRM Register of Monuments of the Republic of Moldova
RAN National Archaeological Record of Romania
ETC Ariuşd, Cucuteni, and Eastern Tripolye cultures
WTC Western Tripolye culture
DEM digital elevation model
SLP terrain slope
TPI topographic position index
TRI terrain ruggedness index
TWI topographic wetness index
MAT mean annual temperature
TS temperature seasonality
TAR temperature annual range
MAP mean annual precipitation
PScv precipitation seasonality–coefficient of variation
GCM global climatic models
CCSM4 Community Climate System Model version 4
MIROC-ESM Model for Interdisciplinary Research on Climate
MPI-ESM-P Max Planck Institute Earth system model
Keywords: eco-cultural niche modeling, Eneolithic, Cucuteni–Tripolye cultural complex, spatial distribution, ecological and bioclimatic variables, niche breadth and overlap, Old Europe
Citation: Mihu-Pintilie A and Gherghel I (2022) Eco-Cultural Niche Breadth and Overlap Within the Cucuteni–Trypillia Culture Groups During the Eneolithic. Front. Earth Sci. 10:910836. doi: 10.3389/feart.2022.910836
Received: 01 April 2022; Accepted: 16 May 2022;
Published: 30 June 2022.
Edited by:
Athos Agapiou, Cyprus University of Technology, CyprusReviewed by:
Catalin Lazar, University of Bucharest, RomaniaBulat Usmanov, Kazan Federal University, Russia
Copyright © 2022 Mihu-Pintilie and Gherghel. This is an open-access article distributed under the terms of the Creative Commons Attribution License (CC BY). The use, distribution or reproduction in other forums is permitted, provided the original author(s) and the copyright owner(s) are credited and that the original publication in this journal is cited, in accordance with accepted academic practice. No use, distribution or reproduction is permitted which does not comply with these terms.
*Correspondence: Alin Mihu-Pintilie, bWlodS5waW50aWxpZS5hbGluQGdtYWlsLmNvbQ==