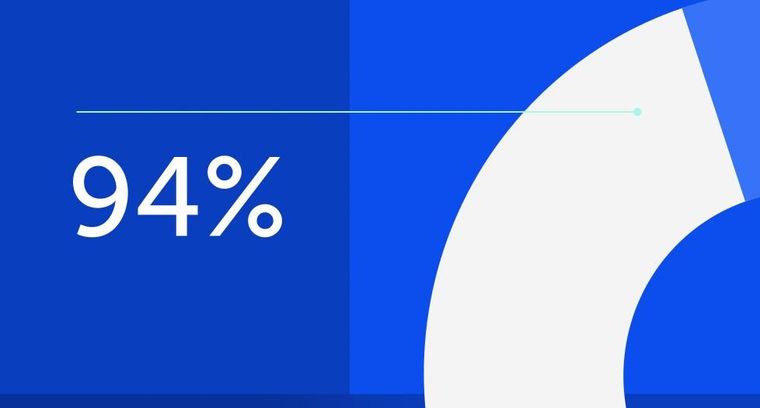
94% of researchers rate our articles as excellent or good
Learn more about the work of our research integrity team to safeguard the quality of each article we publish.
Find out more
ORIGINAL RESEARCH article
Front. Earth Sci., 24 June 2022
Sec. Biogeoscience
Volume 10 - 2022 | https://doi.org/10.3389/feart.2022.906408
Aerosol loading and cloud cover can alter the composition of radiation reaching the Earth’s surface and affect the ecosystem’s carbon cycle. In this study, we established an empirical model of the diffuse radiation fraction (Kd) based on a clearness index (Kt) to obtain the Kd of four FLUXNET forest sites in China. We focused on the relationships among the Kd, photosynthetically active radiation (PAR), light-use efficiency (LUE) and gross primary productivity (GPP) through mechanistic analysis. The relationships between carbon fluxes [including GPP, ecosystem respiration (ER), and net ecosystem exchange (NEE)] and the Kd were explored. Furthermore, we investigated the influence of environmental factors on carbon fluxes. The results showed that the Kd models were accurate in estimating Kd (R2= 0.88–0.93). Overall, the GPP first increased and then decreased with increasing Kd. When Kd< Ko (Ko, the diffuse radiation fraction corresponding to the maximum value of GPP), the direct PAR decreased as Kd increased, while the diffuse PAR increased rapidly. At this stage, the diffuse fertilization effect led to an increase in GPP. When Ko<Kd<Kdiff-max (Kdiff-max, the diffuse radiation fraction corresponding to the maximum value of diffuse PAR), as Kd increased the direct PAR still decreased and the diffuse PAR still increased, but the GPP declined. When Kd>Kdiff-max, the diffuse PAR began to decrease, and the reduction in the superimposed direct PAR caused the GPP of the canopy to drop rapidly. The LUE of the vegetation canopy was higher under diffuse light conditions than under direct light. Furthermore, with an increase in the Kd, the negative value change of the NEE was consistent with the GPP, but the ER was less affected by the Kd. Finally, the impact of temperature (TA) and vapor pressure deficit (VPD) on the GPP was unimodal, and the impact on the NEE was U-shaped. In addition, latent heat (LE) had a significant positive effect on GPP and NEE. Our study emphasized the relationship between the change in PAR composition and the Kd, as well as its impact on the carbon fluxes change, which is highly important to the study of carbon neutralization.
Solar radiation is the main source of energy exchange on the Earth’s surface and an important factor affecting the productivity of ecosystems. Among them, wavelengths between 400–700 nm are considered photosynthetically active radiation (PAR), which is the main energy source of plant photosynthesis (Tang et al., 2013; Ren et al., 2018). According to whether they can receive solar radiation, the leaves of the vegetation canopy can be divided into sunlit leaves and shaded leaves (Zhang Q. et al., 2017). For a canopy with a complex vertical structure, sunlit leaves receive both direct and diffuse radiation and photosynthesis is light-saturated, while shaded leaves receive only diffuse radiation and photosynthesis is usually limited by light (Yue and Unger, 2017; Zhou et al., 2021b; Xue et al., 2021). Aerosol pollution and the presence of clouds increase diffuse radiation (Xue et al., 2020; Zeng et al., 2021). Compared with direct radiation, diffuse radiation has a stronger ability to penetrate the vegetation canopy and a more homogeneous distribution (Ryu et al., 2019), as well as possesses a higher ratio of blue light (Urban et al., 2012), which can promote photosynthesis of shaded leaves and improve the light-use efficiency (LUE), thus improving the gross primary productivity (GPP) of the ecosystem, which is called the diffuse fertilization effect (DFE) (Wang et al., 2015; Wang et al., 2018; Gao et al., 2018; Rap et al., 2018; Xie et al., 2020; Gui et al., 2021). At the same time, however, aerosols and clouds reduce total radiation, and the balance between the drop in GPP caused by the reduction of total radiation and the effect of diffuse fertilization is uncertain (Xing et al., 2017; Han et al., 2019; Zhou et al., 2021a; Xie et al., 2021), which is bad for environment production and economic development in China (Li et al., 2022; Yang et al., 2022).
Terrestrial ecosystems absorb carbon dioxide (CO2) through photosynthesis and release carbon into the atmosphere through respiration and therefore play an important role in the global carbon cycle (Xiao et al., 2019). There are many parameters to describe carbon cycle, among which GPP, ecosystem respiration (ER) and net ecosystem exchange (NEE) are the primary component of the land-atmospheric carbon cycle and sequestration (Gui et al., 2021). These biological processes affecting the carbon cycle are not only affected by PAR and diffuse fertilization effects, but also by environmental factors (Normand et al., 2021; Shen et al., 2022a). The efficiency of photosynthetically—transpiration (respiration) is influenced by many factors, including air temperature, solar radiation, water availability, soil temperature, and forest canopy characteristics (Chen et al., 2021; Shen et al., 2022b). Oliphant et al. (2011) reported that the net effect of vapor pressure deficit (VPD) and air temperature (TA) related to sky conditions contributes to enhancing the light response, although their effect is lower than that of total PAR. Whereas, Dass et al. (2016) showed that the summer temperature explained 37.7% of the annual changes in GPP, while precipitation and cloud accounted for 20.7% and 19.3%, respectively in northern Eurasia. Numerous studies have shown that the effect of environmental factors on GPP cannot be ignored. The change of ecosystem carbon cycle is not the result of the single influence of diffuse radiation, but the result of the combined action of multiple environmental factors, and this influence has great uncertainty, so further exploration is required.
The diffuse radiation fraction (Kd), which is the ratio of diffuse radiation to total radiation, is an important index to measure cloud cover and aerosol load in the sky. It is a key variable to study the influence of diffuse radiation on carbon fluxes and a key process to analyze the impact of PAR and LUE on GPP (Yang et al., 2019; Zhang Y. et al., 2017). Due to limited observations, empirical parameters were historically used to simulate Kd (Rensheng et al., 2004; Zeng et al., 2020). The earliest Kd model was established by Liu and Jordan based on the clearness index (Kt), which is defined as the ratio of total solar radiation (H) to extraterrestrial radiation (H0) (Liu and Jordan., 1960). Since then, many researchers have used different meteorological parameters and other variables for modeling (Li et al., 2011; Dervishi and Mahdavi, 2012; Cao et al., 2017; Gao et al., 2018; Zhou et al., 2019). According to their different input parameters, these models can be divided into 1) models based on Kt, 2) models based on S, 3) models based on Kt and S coupling, and 4) models based on multiple parameters (Li et al., 2011; Li et al., 2011). The previous models mostly used quadratic fitting, for instance, Feng et al. (2018) established a variety of functions to simulate Kd in mainland China, among which quadratic functions performed best, with the average RMSE, MAE and R were 1.81 MJ m−2·d−1, 1.33 MJ m−2·d−1 and 0.79, respectively. But then Yang et al. (2020) compared 18 Kd models established in China, and the results showed that the estimated performance of the cubic function model based on Kt is better than quadratic function, RMSE is 0.08 MJ m−2·d−1 lower. The best-performing model established by Yang et al. (2020) is a binary cubic model coupled with two parameters Kt and S. Moreover, the estimated performance of Kd model coupled with temperature and relative humidity on the basis of Kt and S is slightly better than that of only based on Kt, with RMSE 0.06 MJ m−2·d−1 lower. But compared with Kd model based on Kt and S (uncoupled environmental factors), RMSE is 0.02 MJ m−2·d−1 higher. In other words, models coupled with environmental factors on the basis of Kt and S do not always perform best. Since FLUXNET sites do not provide the observed sunshine duration data and relative humidity data, the Kd model of the coupling of S and environmental factors is not suitable for our study, therefore, this study adopted the Kd model established on the basis of Kt. With the development of technology, some scholars also use machine learning methods to derive Kd (Zhou et al., 2021c). Compared with machine learning methods, the empirical model can take regional radiation conditions and sky conditions into account in the model, which can explain the mechanism of Kd to a certain extent, that is, the influence of radiation components and atmospheric transparency on Kd. In conclusion, the cubic function model based on Kt that we adopted is most appropriate, and can capture the variation of Kd better. In addition, compared with machine learning methods, it can explain the mechanism affecting the formation of Kd more clearly, and is simpler and more efficient. Moreover, few studies have applied this model to simulate the Kd of FLUXNET sites in the past. We tried to build Kd model at FLUXNET sites and got good prediction results, which provides a case of model application where there is no diffuse radiation observation data.
The current research on the impact of diffuse radiation on ecosystem productivity has mostly focused on a large spatial area; few studies are based on the mechanisms of observation sites, and few empirical models are built based on FLUXNET sites. Moreover, the relationship among the PAR, GPP and Kd is less clear. Whether the increase in the Kd is sufficient to compensate for the loss in total PAR and whether the canopy GPP is higher or lower under diffuse radiation need to be further explored. To strengthen research in this field, our study is based on the scale of the site and aims to explore the mechanisms of the interactions between the Kd and the PAR, LUE and GPP depending on the sky conditions, vegetation types and environmental conditions of different sites. In this study, the main objectives are to 1) derive the Kd value of FLUXNET sites; 2) clarify the complex relationship among Kd, PAR, and GPP, as well as Kd, LUE, and GPP, and explore the mechanism of the diffuse fertilization effect and the process of GPP change; 3) explore the response of ecosystem carbon fluxes to Kd and the influence of other environmental factors on GPP. Forest ecosystems are the main terrestrial carbon sinks, making up approximately 80% of the terrestrial carbon sink (Fang et al., 2018; Piao et al., 2022). The study of the effects of diffuse radiation on forest ecosystem carbon fluxes will provide new insight on carbon peaks and carbon neutrality.
FLUXNET is a global network composed of local regional networks that measures the exchanges of carbon dioxide, water vapor, and energy between the biosphere and atmosphere based on the eddy covariance method (Baldocchi et al., 2001; Friend et al., 2007). The daily GPP, NEE, ER, and heat fluxes, as well as meteorological data (including air temperature, shortwave radiation and VPD) were download from the FLUXNET database (https://fluxnet.org/). There are 12 FLUXNET sites in China, the distribution and overview of which can be seen in Figure 1 and Table 1, respectively. Due to the complex vertical structures of the forest canopy, it has a strong response to diffuse radiation (Strada et al., 2015; Zhang Y. et al., 2020). Therefore, we chose only four forest sites in this study: Cha, Qia, Din and MPM. We extracted flux data from the growing season (June–August) when physiological and phenological changes can be largely neglected (Alton et al., 2007). A detailed description of the geographical environment of the four sites is shown in Table 2. There are three kinds of plant functional types (PFT) at FLUXNET forest sites in China, which are mixed forests (MF), evergreen broadleaf forests (EBF) and evergreen needleleaf forests (ENF). The PFT of different sites is related to the local climate. The Cha site located in a higher latitude zone and has a temperate continental climate, forming a mixed forest of temperate coniferous forest and broad-leaved forest. The other three sites are located in the middle and low latitudes zone and have a subtropical monsoon climate, forming evergreen broad-leaved forests and evergreen coniferous forests. The vertical structure of the canopy plays an important role in diffuse fertilization effect. The response of carbon absorption to diffuse radiation is also related to canopy characteristics such as leaf area index (LAI), leaf angle and leaf optical characteristics. Some studies showed that diffuse fertilization effect increased with the increase of LAI (Gui et al., 2021). From the Table 2, Cha site has the largest LAI and canopy height (5.8 m2 m−2 and 26m, respectively), followed by Din and Qia sites. MPM sites had the smallest LAI and canopy height (1.75–2.455.8 m2 m−2 and 2–4 m, respectively). In terms of LAI and canopy height, Cha site has the most complex vertical canopy structure, while MPM site had the simplest canopy structure. In addition, it is worth paying attention to that the typical vegetation type of MPM site is different from other sites. The MPM site is a coastal mangrove ecological community, and mangrove is a woody wetland plant, which has higher requirements on hydrothermal conditions. In addition, the four sites provide data in different years. The Cha, Qia, and Din sites provide data from 2003 to 2005, and the MPM site provides data from 2016 to 2018.
FIGURE 1. The spatial distribution of the CMA radiation sites and FLUXNET sites. TP, NEC, NC, EC, SC, CC, NWC and SWC represent the Tibetan Plateau and northeastern, northern, eastern, southern, central, northwestern and southwestern China, respectively.
The in situ-measured radiation dataset was obtained from the National Meteorological Information Center of the China Meteorological Administration (CMA) (https://data.cma.cn/). It provides daily solar radiation data including daily total radiation, diffuse radiation, and sunshine duration. Quality control was carried out for all data to reduce the impact of outliers on the result analysis. Among all these sites, only 19 sites provided diffuse radiation measurements (He and Wang, 2020). The spatial distribution of radiation sites is shown in Figure 1. For this study, we use daily measurements from 19 diffuse radiation sites during 2000–2017 to establish and verify an empirical model of Kd. Among them, the measurements from 2000 to 2011 were used for Kd modeling, and data from 2012 to 2017 were used for Kd validation. On the other hand, some of these radiation sites are adjacent to FLUXNET sites but they rarely overlap and they are a certain distance away from FLUXNET sites. Therefore, when we built the Kd model of FLUXNET sites, we chose the three nearest radiation sites.
According to the previous empirical model, we selected the Kd model based on Kt. Kt represents the diffusion and absorption effects caused by the presence of the atmosphere (Bortolini et al., 2013; Khorasanizadeh and Mohammadi, 2016). As Kt decreases, sky conditions vary from clear to cloudy (Tsubo and Walker, 2004; Apeh et al., 2021). The FLUXNET sites did not provide diffuse radiation data, but there were many CMA sites around the FLUXNET sites. According to Tobler’s First Law of Geography, everything is related to everything else, but things that are located near each other are more related than things located further away (Tobler, 1970). Therefore, we considered the FLUXNET sites as center points and chose the three CMA radiation sites nearest to the FLUXNET sites. The sky conditions and radiation conditions at the radiation sites were used to approximately represent those of FLUXNET sites. We used the data provided by the radiation sites to build the Kd model and then input the data from the FLUXNET sites to obtain the corresponding Kd. Therefore, according to the distribution of the forest FLUXNET sites, seven CMA sites were chosen. To obtain Kd, we first needed to calculate Kt, which is the ratio of total solar radiation (H) to extraterrestrial radiation (H0). The equation of daily extraterrestrial radiation (H0) was given as follows (Duffie and Beckman, 2020; Yang et al., 2020):
where
Because the measurement of radiation may be subject to errors and failures, it is necessary to perform quality control on the data to identify incorrect data (Yang et al., 2020). This study adopts the data quality control method proposed by Younes (Younes et al., 2005): First, data were rejected if the solar altitude was less than 7°; Second, data were rejected if the ratio of the sunshine duration to maximum possible sunshine was greater than 1; Third, the values of the Kt and Kd ranged between 0–1; Fourth, the envelope was drawn. The results are shown in Figure 2. After the quality control process, the percentage of valid data at the CMA sites was 95.36–97.34%. The remaining datasets were divided into two parts. The data from 2000 to 2011 were used for Kd modeling, and data from 2012 to 2017 were used for Kd validation.
PAR is the main energy source for plant photosynthesis, controlling the rate of effective photosynthesis of terrestrial organisms and directly affecting the growth of vegetation (Li et al., 1997). Its diffuse component can enhance the LUE of the vegetation canopy, thereby increasing carbon uptake. However, not all FLUXNET sites provided PAR observations; only the MPM site provided photosynthesis photon flux density (PPFD), so we converted quantum systems (μmol m−2 s−1) into energy systems (W·m−2) based on Formula (4). Other sites adopted the method proposed by (Zhu et al., 2010), which was based on geographical division.
where
LUE connects solar radiation, photosynthesis and vegetation production, represents the ratio of plant fixation to light energy absorption, and represents the efficiency of converting the light energy captured by plants into chemical energy, that is, the efficiency of CO2 fixation into organic matter, which can indicate vegetation productivity and carbon fixation efficiency (Monteith, 1972; Monteith, 1977; Baldocchi and Penuelas, 2019). We attempted to quantify the LUE to analyze the impact of diffuse radiation on the vegetation LUE, which can be defined as the ratio of the GPP to PAR, as shown in Formula (5) (Strada et al., 2015; Yue and Unger, 2017; Wang et al., 2018) (Strada et al., 2015; Yue and Unger, 2017; Wang et al., 2018).
To explore the applicability of the Kd model at different FLUXNET sites, we evaluated the Kd model using observational data from the CMA radiation sites. Due to the proximity of the Din and MPM sites, the three closest CMA radiation sites were the same, so the data used for modeling and validation were the same. The Kd model based on the four FLUXNET forest sites is shown in Table 3.
Figure 3 shows the density scatterplots of the daily Kd simulations from 2012 to 2017. The results illustrated that the Kd model had excellent performance in simulating Kd, with high R2 values of 0.88–0.93, low RMSE values of 0.07–0.1 W m−2, and low MAE values of 0.06–0.07 W m−2 at the four FLUXNET sites. However, at the Cha site, the model overestimated the low value and underestimated the high value of the Kd, while at the Qia, Din and MPM sites, there was a slight underestimation of the Kd. The land cover, cloud fraction and aerosol conditions varied from site to site, so the model behaved differently across different regions. Compared with the quadratic function model based on Kd established by Yang et al. (2020), our RMSE decreased by 0.46–0.81 MJ m−2·d−1, the estimated performance is obviously better.
FIGURE 3. Density scatterplots of the model-simulated Kd. (A–C) are Cha, Qia, Din and MPM sites; the dashed black and solid red lines indicate the 1:1 line and the linear regression line, respectively.
The PAR data from all the sites except for the MPM site were based on previous empirical formulas. Therefore, it was necessary to simulate PAR. We evaluated the empirical model of PAR using observations at the MPM site because only this site had observation data. Figure 4 shows the density scatterplots of the simulated PAR. The R2 of the results obtained by the empirical model and the measured results at the FLUXNET site was 0.79, indicating that the model can estimate the PAR of the FLUXNET site well. Its RMSE and MAE were relatively low, at 13.60 and 10.79 W m−2, respectively. Even if the factors affecting PAR are complex and changeable, the empirical model was based on certain climatological methods, so it could reasonably simulate PAR.
FIGURE 4. Density scatterplots of the model-simulated PAR. The solid black and solid red lines indicate the 1:1 line and the line regression line, respectively.
Kd is affected by solar radiation, aerosols and clouds, so it changes over time. Exploring the temporal change of the Kd helps assess its impact on GPP. We analyzed the differences in FLUXNET sites from the monthly changes in Kd. Figure 5 is the monthly variation in the Kd simulated at the four forest sites, and the changes in the Kd at the four sites are different. At a monthly scale, the Kd of the Cha sites was the highest in July and the lowest in January, showing a single-peak trend throughout the year. This is because the Cha site has a typical temperate continental climate, with strong radiation and high cloud cover in summer and the opposite in winter. In contrast to the Cha sites, the Qia sites had the lowest Kd in July due to the low cloud cover during the dry season and the highest Kd in January, which may be due to the presence of more anthropogenic aerosols in winter. The Din sites had the lowest Kd in October due to the low cloud cover during the dry season and the highest Kd in March due to radiation increasing gradually in spring. However, the Kd of the MPM site was the highest in April and the lowest in December, but there was little change throughout the year, which was mainly due to the low latitude of the MPM site and the slight annual change in solar radiation. In this study, we mainly studied the effects of diffuse radiation on vegetation productivity during the growing season (summer, including June, July and August), and the Kd of the four forest sites in summer from high to low were Din (0.75), MPM (0.66), Qia (0.65) and Cha (0.65).
FIGURE 5. The monthly variations in Kd simulated at four forest sites. (A–D) are Cha, Qia, Din and MPM sites, respectively.
Figure 6 shows the spatial distribution of the Kd simulated at all FLUXNET sites. Spatially, the Kd of the four seasons was roughly zonal in distribution: high at low latitudes, low at high latitudes, and gradually decreasing from low latitudes to high latitudes. The Tibetan Plateau was also a low value area. This was mainly due to the zonal distribution of solar radiation and cloud cover. Solar radiation in low latitudes is strong, and cloud cover is high, so the diffuse radiation is greater and the Kd is higher. The opposite pattern is true at higher latitudes. The Tibetan Plateau has a high altitude, low cloud coverage and strong direct radiation, so the Kd is also low. Our research focused on forest sites, but to understand the spatial distribution of the Kd at all FLUXNET sites and all vegetation types, it is helpful to understand the Kd levels at the four forest sites. Figure 6 shows that the Kd values of these four forest sites are higher than those of the other sites in all seasons.
FIGURE 6. The spatial distribution of the Kd simulated at all FLUXNET sites. (A–D) are spring (March-April-May), summer (June-July-August), autumn (September-October-November) and winter (Dec-Jan-Feb), respectively.
Changes in aerosol load and cloud cover not only change the PAR reaching the surface but also change the proportion of diffuse radiation, with uncertain overall effects on global plant productivity and the terrestrial carbon sink.
We first explored the relationship among the Kd, PAR and GPP, and the result is shown in Figure 7. Clouds and aerosols can increase diffuse radiation, thereby promoting Kd. As the Kd increased, GPP first increased and then decreased. It was closely related to PAR, and changes in the Kd caused changes in PAR and its components. Overall, the total PAR decreased with increasing Kd. However, the changes in its direct and diffuse components were more complicated during the different ranges of Kd. When Kd gradually increased to Ko (Kd corresponds to the maximum value of GPP), the direct PAR was higher than the diffuse PAR but decreased rapidly, while the diffuse PAR showed the opposite results and increased rapidly, and the two radiation components reached a balanced state near Ko. At this stage, the growth in GPP was mainly caused by the increase in diffuse PAR. The presence of clouds and aerosols increased the diffuse PAR, and the diffuse PAR in the canopy had a stronger transmittance, which can spread more incident radiation to shaded leaves, greatly promoting the photosynthesis of the shaded leaves. This diffuse fertilization effect leads to an increase in canopy GPP. Due to differences in vegetation PFTs, aerosol load and cloud cover, different regions had an optimal Kd (Ko) when the GPP reached a maximum value. The optimal Kd (Ko) values of the Cha (MF), Qia (ENF), Din (EBF), and MPM (EBF) sites were 0.54, 0.56, 0.57, and 0.43, respectively, and the sky conditions at this time were the most favorable for carbon assimilation. This result is consistent with the optimal Kd range of forests obtained by previous studies, which was between 0.4–0.6 (Alton et al., 2007; Alton, 2008; Zhang J. et al., 2020).
FIGURE 7. The relationship among Kd, PAR and GPP. (A–D) are Cha, Qia, Din and MPM sites, respectively.
When the Kd exceeds Ko, the amount of aerosols and clouds in the sky is too high, which greatly reduces the total PAR reaching the surface, so the GPP begins to decrease with an increase in Kd. However, the root cause of the reduction in total PAR is the substantial reduction in direct PAR. The diffuse PAR first increases and then decreases with increasing Kd. This is because the moderate increase in aerosols and cloud cover promotes diffuse PAR. Once the increase becomes excessive, it greatly reduces the total PAR, resulting in no excess PAR becoming scattered. When the Kd is between Ko and Kdiff-max (Kd corresponds to the maximum value of diffuse PAR), although the diffuse PAR is higher than the direct PAR, it grows slowly, while the direct PAR still decreases, and the diffuse fertilization effect is not enough to compensate for the loss of GPP caused by the reduction of direct PAR, so the overall GPP at this stage has a downward trend. When the Kd is greater than the Kdiff-max, the diffuse PAR also begins to decrease, and because the total PAR has been drastically reduced, there is not enough radiation for the aerosols and clouds to scatter. The drop in diffuse PAR causes a weakening of photosynthesis in shaded leaves, while the direct PAR is still in a reduced state, which leads to a weakening of photosynthesis in sunlit leaves. The reduction in the superposition of the two radiation components results in the rapid decline of canopy GPP.
Hence, the net effect on the canopy carbon assimilation depends on a balance between the reduction in direct PAR (which tends to reduce photosynthesis) and the increase in diffuse PAR (which tends to increase photosynthesis).
Figure 7 shows that the LUE increases as the Kd increases. Furthermore, we compared the LUE under direct and diffuse radiation conditions. The sky conditions were separated into categories of predominantly diffuse (Kd ≥ 0.5) and predominantly direct (Kd < 0.5) sunlight. We divided the sky condition based on the Kd, and considered direct radiation to be dominant when the Kd was less than 0.5 and diffuse radiation to be dominant when the Kd was greater than 0.5 (Alton, 2008). The LUE of FLUXNET forest sites under different radiation conditions is shown in Figure 8. The LUE of the four forest sites from high to low was: Cha, MPM, Qia and Din. At all sites, the LUE was significantly higher under diffuse radiation dominated conditions than direct radiation dominated conditions. Specifically, when the sky radiance was dominated by diffuse sunlight, the LUE was found to be 5.52%, 3.38%, 2.58%, and 3.77% higher than that under direct sunlight at the Cha, Qia, Din and MPM sites, respectively. This observation indicated an enhanced LUE, with the greatest increase occurring in dense foliage, such as the Cha site dominated by MF. Table 2 shows that the Cha site has the most complex vertical structure among the four forest sites because it has the highest LAI (5.8 m2·m−2) and the highest canopy height (26 m), so it is more sensitive to the diffuse radiation response.
Under clear-sky conditions, there were fewer clouds and aerosols, and the Kd was low, so solar radiation was dominated by direct light and sunlight was too concentrated on sunlit leaves. This situation led to the sunlit leaves in the canopy being usually light-saturated, and since extra sunlight cannot be used efficiently for photosynthesis, the LUE was low. At the same time, shaded leaves are more efficient at using light, but they were limited by the low incident radiation that they have received. In contrast, under cloudy or aerosol sky conditions, sunlight is more scattered and incoming radiation is more diffuse, the irradiance of the canopy is more uniform, and shaded leaves can receive much more sunlight from all directions, so the light-saturated part of the canopy is reduced and the LUE increases. This also demonstrates the diffuse fertilization effect. In conclusion, canopy photosynthesis tends to be significantly more light-use efficient under diffuse sunlight than under direct sunlight. The LUE enhancement under diffuse sunlight can be explained by the sharing of the canopy radiation load, which is reduced under direct sunlight. A modest reduction in direct radiation does not reduce photosynthesis in sunlit leaves because the LUE remains light saturated. However, when the amount of the Kd is too high, the total PAR is greatly reduced, and both the diffuse and direct PAR components are reduced (Figure 7). Even though the LUE still increases, the available light is reduced, so the canopy GPP also decreases.
The terrestrial ecosystem’s carbon cycle, especially ecosystem photosynthesis and respiration, is quite sensitive to aerosol loading and cloud fraction (Doughty et al., 2010). Figure 9 shows the relationship among the Kd and terrestrial ecosystem carbon fluxes, including GPP, NEE and ER. NEE represents the difference between gross photosynthetic uptake and respiratory losses. The ecosystem is a net carbon sink when the NEE is less than 0, and it becomes a carbon source when the NEE is larger than 0. Therefore, the higher the negative NEE value is, the more carbon is absorbed by the ecosystem. Ecosystem respiration is a biological process that converts organic carbon into CO2. It is a compound flux consisting of the aboveground respiration of leaves and woody tissues, the underground respiration of roots (autotrophic soil respiration), and the underground respiration of soil organisms (heterotrophic soil respiration). ER represents a fundamental biospheric function and plays a major role in the global carbon cycle and growth rate of atmospheric CO2.
FIGURE 9. The relationship among Kd and ecosystem carbon fluxes. (A–D) are Cha, Qia, Din and MPM sites, respectively.
As the aerosol loading and cloud cover increase, the Kd increases continuously, and the negative NEE value shows a consistent trend with the GPP; that is, the negative NEE value first increases and then decreases. This shows that the carbon uptake of the ecosystem first increases and then decreases, but when the Kd approaches 1, the NEE of the four sites appears to be higher than 0, demonstrating that the ecosystem has switched from a carbon sink to a carbon source. The increase in carbon uptake is primarily a result of the diffuse fertilization effect. The photosynthesis and LUE of shaded leaves are greatly promoted by diffuse PAR. When the Kd is too high (around Ko), clouds and aerosols cause a large reduction in total PAR. There is not enough PAR for photosynthesis, so the GPP and NEE decrease. The Kd values corresponding to the maximum NEE were 0.56, 0.57, 0.59, and 0.42 at the Cha, Qia, Din and MPM sites, respectively. Except for at the MPM site, where the Kd value was lower than Ko, the Kd values at the other sites were larger than Ko, indicating that the NEE response to Kd was later than that of the GPP at most sites. This delay effect is caused by ecosystem respiration. The ER response to Kd has great uncertainty. The Kd has little influence on ER, and ER shows a weak downward trend with increasing Kd. This is mainly because the factors influencing ER are complex and diverse, among which the Kd is not the leading influencing factor.
The ecosystem carbon cycle consists of biological processes such as photosynthesis and respiration, which are not only affected by PAR and diffuse fertilization effects but also regulated by various environmental factors. Temperature and moisture are important conditions for vegetation growth, so we considered the impact of environmental factors such as temperature (TA), atmospheric vapor pressure deficit (VPD) and latent heat (LE) on the carbon cycle. The result is shown in Figure 10.
FIGURE 10. The relationship among environmental factors and ecosystem carbon fluxes. (i–iv) for Cha, Qia, Din and MPM sites; (A–C) for TA, VPD and LE, respectively.
Temperature had a significant impact on the GPP of the Cha, Qia, Din, and MPM sites. Among them, the TA of the Qia, Din and MPM sites had an obvious single-peak change in the impact on vegetation, which increased in GPP at first and then decreased. However, the single-peak characteristics at the Cha sites were not significant, showing that the GPP increased with increasing TA. The Cha sites are located in northeastern China, where thermal conditions restrict vegetation growth. Studies have shown that temperature is the main environmental control factor for the increase in GPP in northern Eurasia (Dass et al., 2016). The Qia, Din and MPM sites have lower latitudes and better thermal conditions. Due to higher temperatures there, there was a single-peak change in the impact on vegetation. Studuies have shown that temperature and VPD can limit photosynthesis and respiration (Moazenzadeh et al., 2018). Some researchers believe that vegetation growth has an optimal temperature, and at the most suitable temperature, productivity is the highest. Gui et al. (2021) pointed out that when considering the coeffects of the VPD and TA, the strongest positive effect of diffuse fertilization effects was found at 0–5 hPa and 20–25°C. Zhang Y. et al., 2020 further found that 5–20°C and a VPD < 10 hPa were the optimum ranges for the strongest positive effects of diffuse light on canopy photosynthesis, reflecting that the effect may decrease with increasing stomatal resistance to leaf carbon uptake. Our research shows that when the TA was 26–27°C at the Qia site, the GPP was the highest, the Din site had the highest GPP at approximately 31–32°C, and the MPM site had the highest GPP at approximately 30–31°C. When the temperature is too high, it affects the activity of vegetation enzymes, which in turn affects photosynthesis (Kanniah et al., 2011). At the Cha, Din, and MPM sites, the increase in temperature promoted ER. At the Din and MPM sites, with the increase in temperature, NEE also showed a negative increasing trend, indicating that the increase in temperature at these two sites was conducive to carbon uptake.
VPD is identified as a critical driver of ecosystem function, and the VPD affects the stomatal conductance of the canopy. Similar to the results of previous studies, the GPP we obtained varied with VPD as a unimodal curve, which was mainly manifested at all the FLUXNET forest sites. Studies have shown that when the VPD threshold is 6–8 hPa, and it determines whether the GPP response transitions from a significant increase to a decrease (Chen et al., 2021). Our results suggested that an increasing VPD under climate change could reduce forest CO2 uptake (Sulman et al., 2016). The increase in VPD may exceed the threshold of stomatal openings, which may have a negative impact on GPP. Our research shows that when the VPD of the Cha site was 10–11 hPa, the GPP was the highest, while the Qia and Din sites were 15–16 hPa, and the MPM site was 9–10 hPa. When the VPD is within the appropriate threshold, GPP will increase. However, when the VPD is large, plants will close part of their stomata to reduce the loss caused by transpiration. Studies have shown that in forest ecosystems, GPP is not always restricted by the VPD, which is mainly due to forests having a stronger water holding capacity and deeper root systems (Beer et al., 2007; Chen et al., 2021; Gui et al., 2021). The GPP in these places is mainly affected by radiation or temperature. At the Cha and Din sites, the VPD was helpful in promoting ER, but it was not significant at the other sites. In contrast to the GPP, the impact of the VPD on NEE was U-shaped at the Cha, Din, and MPM sites but it was essentially the same. As the VPD increased, carbon absorption first increased and then decreased.
LE is the heat exchange of moisture between the underlying surface and the atmosphere, it has important effects on material and energy fluxes between ecosystems and the atmosphere. High LE can provide energy, water and heat exchange for vegetation (Kasurinen et al., 2014). At all FLUXNET forest sites, the GPP and LE showed a linear increase relationship, while the NEE showed a linear decrease. Among these sites, this pattern was particularly prominent at the Din and MPM sites. These two EBF sites are located in subtropical regions. The vegetation evapotranspiration at these sites is high because of their dense and complex canopy structure. Therefore, the vegetation growth at these sites had a high demand for water and heat. The LE of the MPM site was significantly higher than that of the other sites. The MPM site is dominated by mangroves. Mangroves are tropical wetland vegetation that grow in a high-temperature and humid environment and have high requirements for water and heat, so the latent heat flux deeply influences carbon fluxes (Lugo, 1987). However, the effect of LE on ER is not obvious in general. But at the MPM site, the LE had a positive effect on ER.
In this work, the effect of diffuse radiation on the terrestrial ecosystem carbon fluxes of FLUXNET forest sites was investigated. First, we established a Kd empirical model based on Kt to simulate the Kd at the Cha, Qia, Din and MPM sites, and the results showed that the Kd model was accurate in estimating the Kd (R2= 0.88–0.93). The monthly variation in Kd was different among the FLUXNET sites. The spatial distribution of Kd showed a latitudinal zonality, decreasing gradually from low latitudes to high latitudes. Furthermore, we found changes exist in the relationship between Kd, PAR, and GPP and the stage characteristics of GPP. With increasing Kd, GPP first increased and then decreased. When Kd<Ko, the direct PAR decreased rapidly, while the diffuse PAR increased rapidly. When Ko<Kd<Kdiff-max, the direct PAR still decreased, and the diffuse PAR still increased, but the total PAR was greatly reduced by excessive aerosols and clouds, so the GPP declined. When Kd>Kdiff-max, the diffuse PAR began to decrease, and the reduction of the superimposed direct PAR caused the GPP of the canopy to drop rapidly. Moreover, under conditions dominated by diffuse light, the LUE of the vegetation canopy was higher than that under direct light. In addition, the results indicated that the ecosystem switches from a carbon sink to a carbon source when the Kd approaches 1. Finally, we found that the impact of TA and VPD on GPP is unimodal, and correspondingly, the impact on NEE is U-shaped. However, LE is a combination of hydrothermal conditions that play a positive role in ecosystem productivity and carbon uptake.
Previous studies mostly focused on the effect of the Kd or PAR on GPP, and few studies could explain the mechanism by integrating the three factors. Our research clarified the relationship among the Kd, PAR, and GPP, as well as Kd, LUE, and GPP through mechanistic analysis. It indicated that the diffuse PAR does not always increase with increasing Kd but decreases when the Kd is too high. In conclusion, the net effect on canopy carbon uptake depends on a balance between the reduction in total PAR and the change in diffuse PAR. However, the Kd model based on Kt needs to be further improved. In previous studies, it has been concluded that the estimated performance of Kd model coupled Kt and S is the best. Whereas, due to the limitations of the existing observation data of FLUXNET sites, we failed to establish the Kd model of Kt and S coupled. In future, we will obtain sunshine duration through modeling or other methods to further improve our model. In addition, the Kt that input Kd model is also calculated based on the empirical model, because the data used to calculate Kt is difficult to be measured due to technical limitations, so the uncertainty may affect the research results (El Mghouchi et al., 2016; Zhang Y. et al., 2017; Shen et al., 2022a). Forests are an important part of the carbon cycle in terrestrial ecosystems. Clarifying the impact of diffuse radiation on the carbon assimilation of forest ecosystems will help improve forest management and promote forest sinks to better achieve the goal of carbon neutrality.
The original contributions presented in the study are included in the article/Supplementary Material, further inquiries can be directed to the corresponding author.
XD: Conceptualization, Methodology, Software, Formal Analysis, Writing—Original Draft; JZ: Conceptualization, Methodology, Supervision, Project Administration, Funding Acquisition, Review and Editing; YC, LZ, TL, and TH: Methodology, Software, Review and Editing.
This research was supported by the National Natural Science Foundation of China (41575144) and the National Key R&D Program of China (2017YFA0603603).
The authors declare that the research was conducted in the absence of any commercial or financial relationships that could be construed as a potential conflict of interest.
All claims expressed in this article are solely those of the authors and do not necessarily represent those of their affiliated organizations, or those of the publisher, the editors and the reviewers. Any product that may be evaluated in this article, or claim that may be made by its manufacturer, is not guaranteed or endorsed by the publisher.
The authors thank the FLUXNET global network (http://fluxnet.fluxdata.org/) for providing the carbon fluxes observations and the National Information Centre (http://data.cma.cn/site/index.html) of the China Meteorological Administration for providing the meteorological data in this study.
Alton, P. B., North, P. R., and Los, S. O. (2007). The Impact of Diffuse Sunlight on Canopy Light-Use Efficiency, Gross Photosynthetic Product and Net Ecosystem Exchange in Three Forest Biomes. GCB Bioenergy 13, 776–787. doi:10.1111/J.1365-2486.2007.01316.X
Alton, P. B. (2008). Reduced Carbon Sequestration in Terrestrial Ecosystems under Overcast Skies Compared to Clear Skies. Agric. For. Meteorology 148, 1641–1653. doi:10.1016/J.AGRFORMET.2008.05.014
Apeh, O. O., Overen, O. K., and Meyer, E. L. (2021). Monthly, Seasonal and Yearly Assessments of Global Solar Radiation, Clearness Index and Diffuse Fractions in Alice, South Africa. Sustainability 13, 2135. doi:10.3390/SU13042135
Baldocchi, D., Falge, E., Gu, L., Olson, R., Hollinger, D., Running, S., et al. (2001). FLUXNET: A New Tool to Study the Temporal and Spatial Variability of Ecosystem-Scale Carbon Dioxide, Water Vapor, and Energy Flux Densities. Bull. Amer. Meteor. Soc. 82, 2415–2434. doi:10.1175/1520-0477(2001)082<2415:FANTTS>2.3.CO;2
Baldocchi, D., and Penuelas, J. (2019). The Physics and Ecology of Mining Carbon Dioxide from the Atmosphere by Ecosystems. Glob. Change Biol. 25, 1191–1197. doi:10.1111/GCB.14559
Beer, C., Reichstein, M., Ciais, P., Farquhar, G. D., and Papale, D. (2007). Mean Annual GPP of Europe Derived from its Water Balance. Geophys. Res. Lett. 34, L05401. doi:10.1029/2006GL029006
Bortolini, M., Gamberi, M., Graziani, A., Manzini, R., and Mora, C. (2013). Multi-location Model for the Estimation of the Horizontal Daily Diffuse Fraction of Solar Radiation in Europe. Energy Convers. Manag. 67, 208–216. doi:10.1016/J.ENCONMAN.2012.11.008
Cao, F., Li, H., Yang, T., Li, Y., Zhu, T., and Zhao, L. (2017). Evaluation of Diffuse Solar Radiation Models in Northern China: New Model Establishment and Radiation Sources Comparison. Renew. Energy 103, 708–720. doi:10.1016/J.RENENE.2016.11.004
Chen, N., Song, C., Xu, X., Wang, X., Cong, N., Jiang, P., et al. (2021). Divergent Impacts of Atmospheric Water Demand on Gross Primary Productivity in Three Typical Ecosystems in China. Agric. For. Meteorology 307, 108527. doi:10.1016/J.AGRFORMET.2021.108527
Dass, P., Rawlins, M. A., Kimball, J. S., and Kim, Y. (2016). Environmental Controls on the Increasing GPP of Terrestrial Vegetation across Northern Eurasia. Biogeosciences 13, 45–62. doi:10.5194/BG-13-45-2016
Dervishi, S., and Mahdavi, A. (2012). Computing Diffuse Fraction of Global Horizontal Solar Radiation: A Model Comparison. Sol. Energy 86, 1796–1802. doi:10.1016/J.SOLENER.2012.03.008
Doughty, C. E., Flanner, M. G., and Goulden, M. L. (2010). Effect of Smoke on Subcanopy Shaded Light, Canopy Temperature, and Carbon Dioxide Uptake in an Amazon Rainforest. Glob. Biogeochem. Cycles 24, GB3015. doi:10.1029/2009GB003670
El Mghouchi, Y., El Bouardi, A., Choulli, Z., and Ajzoul, T. (2016). Models for Obtaining the Daily Direct, Diffuse and Global Solar Radiations. Renew. Sustain. Energy Rev. 56, 87–99. doi:10.1016/J.RSER.2015.11.044
Fang, J., Yu, G., Liu, L., Hu, S., and Chapin, F. S. (2018). Climate Change, Human Impacts, and Carbon Sequestration in China. Proc. Natl. Acad. Sci. U.S.A. 115, 4015–4020. doi:10.1073/pnas.1700304115
Feng, L., Lin, A., Wang, L., Qin, W., and Gong, W. (2018). Evaluation of Sunshine-Based Models for Predicting Diffuse Solar Radiation in China. Renew. Sustain. Energy Rev. 94, 168–182. doi:10.1016/J.RSER.2018.06.009
Friend, A. D., Arneth, A., Kiang, N. Y., Lomas, M., Ogée, J., Rödenbeck, C., et al. (2007). FLUXNET and Modelling the Global Carbon Cycle. Glob. Change Biol. 13, 610–633. doi:10.1111/J.1365-2486.2006.01223.X
Gao, X., Gu, F., Mei, X., Hao, W., Li, H., Gong, D., et al. (2018). Light and Water Use Efficiency as Influenced by Clouds And/or Aerosols in a Rainfed Spring Maize Cropland on the Loess Plateau. Crop Sci. 58, 853–862. doi:10.2135/CROPSCI2017.06.0341
Gui, X., Wang, L., Su, X., Yi, X., Chen, X., Yao, R., et al. (2021). Environmental Factors Modulate the Diffuse Fertilization Effect on Gross Primary Productivity across Chinese Ecosystems. Sci. Total Environ. 793, 148443. doi:10.1016/J.SCITOTENV.2021.148443
Han, J., Zhang, L., Li, S., Wen, X., Li, Q., and Wang, H. (2019). Effects of Sky Conditions on Net Ecosystem Productivity of a Subtropical Coniferous Plantation Vary from Half-Hourly to Daily Timescales. Sci. Total Environ. 651, 3002–3014. doi:10.1016/J.SCITOTENV.2018.10.190
He, Y., and Wang, K. (2020). Variability in Direct and Diffuse Solar Radiation Across China From 1958 to 2017. Geophys. Res. Lett. 47, e2019GL084570. doi:10.1029/2019GL084570
Kanniah, K. D., Beringer, J., and Hutley, L. B. (2011). Environmental Controls on the Spatial Variability of Savanna Productivity in the Northern Territory, Australia. Agric. For. Meteorology 151, 1429–1439. doi:10.1016/J.AGRFORMET.2011.06.009
Kasurinen, V., Alfredsen, K., Kolari, P., Mammarella, I., Alekseychik, P., Rinne, J., et al. (2014). Latent Heat Exchange in the Boreal and Arctic Biomes. Glob. Change Biol. 20, 3439–3456. doi:10.1111/GCB.12640
Khorasanizadeh, H., and Mohammadi, K. (2016). Diffuse Solar Radiation on a Horizontal Surface: Reviewing and Categorizing the Empirical Models. Renew. Sustain. Energy Rev. 53, 338–362. doi:10.1016/j.rser.2015.08.037
Li, H., Ma, W., Wang, X., and Lian, Y. (2011). Estimating Monthly Average Daily Diffuse Solar Radiation with Multiple Predictors: A Case Study. Renew. Energy 36, 1944–1948. doi:10.1016/J.RENENE.2011.01.006
Li, X., Xue, W., Wang, K., Che, Y., and Wei, J. (2022). Environmental Regulation and Synergistic Effects of PM2.5 Control in China. J. Clean. Prod. 337, 130438. doi:10.1016/J.JCLEPRO.2022.130438
Li, Z., Moreau, L., and Cihlar, J. (1997). Estimation of Photosynthetically Active Radiation Absorbed at the Surface. J. Geophys. Res. 102, 29717–29727. doi:10.1029/97JD01219
Liu, B. Y. H., and Jordan, R. C. (1960). The Interrelationship and Characteristic Distribution of Direct, Diffuse and Total Solar Radiation. Sol. Energy 4, 1–19. doi:10.1016/0038-092X(60)90062-1
Moazenzadeh, R., Mohammadi, B., Shamshirband, S., and Chau, K.-w. (2018). Coupling a Firefly Algorithm with Support Vector Regression to Predict Evaporation in Northern iran. Eng. Appl. Comput. Fluid Mech. 12, 584–597. doi:10.1080/19942060.2018.1482476
Monteith, J. L. (1977). Climate and the Efficiency of Crop Production in Britain. Phil. Trans. R. Soc. Lond. B 281, 277–294. doi:10.1098/RSTB.1977.0140
Monteith, J. L. (1972). Solar Radiation and Productivity in Tropical Ecosystems. J. Appl. Ecol. 9, 747. doi:10.2307/2401901
Normand, A. E., Turner, B. L., Lamit, L. J., Smith, A. N., Baiser, B., Clark, M. W., et al. (2021). Organic Matter Chemistry Drives Carbon Dioxide Production of Peatlands. Geophys. Res. Lett. 48, e2021GL093392. doi:10.1029/2021GL093392
Oliphant, A. J., Dragoni, D., Deng, B., Grimmond, C. S. B., Schmid, H.-P., and Scott, S. L. (2011). The Role of Sky Conditions on Gross Primary Production in a Mixed Deciduous Forest. Agric. For. Meteorology 151, 781–791. doi:10.1016/j.agrformet.2011.01.005
Piao, S., He, Y., and Wang, X. (2022). Estimation of China’s Terrestrial Ecosystem Carbon Sink: Methods, Progress and Prospects.. Sci. China Earth Sci. 65, 641–651. doi:10.1007/s11430-021-9892-6
Rap, A., Scott, C. E., Reddington, C. L., Mercado, L., Ellis, R. J., Garraway, S., et al. (2018). Enhanced Global Primary Production by Biogenic Aerosol via Diffuse Radiation Fertilization. Nat. Geosci. 11, 640–644. doi:10.1038/s41561-018-0208-3
Ren, X., He, H., Zhang, L., and Yu, G. (2014). Estimation of Diffuse Photosynthetically Active Radiation and the Spatiotemporal Variation Analysis in China from 1981 to 2010. J. Geogr. Sci. 24, 579–592. doi:10.1007/S11442-014-1107-1
Ren, X., He, H., Zhang, L., and Yu, G. (2018). Global Radiation, Photosynthetically Active Radiation, and the Diffuse Component Dataset of China, 1981-2010. Earth Syst. Sci. Data 10, 1217–1226. doi:10.5194/ESSD-10-1217-2018
Rensheng, C., Ersi, K., Jianping, Y., Shihua, L., Wenzhi, Z., and Yongjian, D. (2004). Estimation of Horizontal Diffuse Solar Radiation with Measured Daily Data in China. Renew. Energy 29, 717–726. doi:10.1016/J.RENENE.2003.09.012
Ryu, Y., Berry, J. A., and Baldocchi, D. D. (2019). What Is Global Photosynthesis? History, Uncertainties and Opportunities. Remote Sens. Environ. 223, 95–114. doi:10.1016/J.RSE.2019.01.016
Shen, X., Liu, B., Henderson, M., Wang, L., Jiang, M., and Lu, X. (2022a). Vegetation Greening, Extended Growing Seasons, and Temperature Feedbacks in Warming Temperate Grasslands of China. J. Clim. 35, 1–51. doi:10.1175/JCLI-D-21-0325.1
Shen, X., Liu, Y., Liu, B., Zhang, J., Wang, L., Lu, X., et al. (2022b). Effect of Shrub Encroachment on Land Surface Temperature in Semi-arid Areas of Temperate Regions of the Northern Hemisphere. Agric. For. Meteorology 320, 108943. doi:10.1016/J.AGRFORMET.2022.108943
Spitters, C. J. T., Toussaint, H. A. J. M., and Goudriaan, J. (1986). Separating the Diffuse and Direct Component of Global Radiation and its Implications for Modeling Canopy Photosynthesis Part I. Components of Incoming Radiation. Agric. For. Meteorology 38, 217–229. doi:10.1016/0168-1923(86)90060-2
Strada, S., Unger, N., and Yue, X. (2015). Observed Aerosol-Induced Radiative Effect on Plant Productivity in the Eastern United States. Atmos. Environ. 122, 463–476. doi:10.1016/J.ATMOSENV.2015.09.051
Sulman, B. N., Roman, D. T., Yi, K., Wang, L., Phillips, R. P., and Novick, K. A. (2016). High Atmospheric Demand for Water Can Limit Forest Carbon Uptake and Transpiration as Severely as Dry Soil. Geophys. Res. Lett. 43, 9686–9695. doi:10.1002/2016GL069416
Tang, W., Qin, J., Yang, K., Niu, X., Zhang, X., Yu, Y., et al. (2013). Reconstruction of Daily Photosynthetically Active Radiation and its Trends over China. J. Geophys. Res. Atmos. 118, 13292–13302. doi:10.1002/2013JD020527
Tobler, W. R. (1970). A Computer Movie Simulating Urban Growth in the Detroit Region. Econ. Geogr. 46, 234. doi:10.2307/143141
Tsubo, M., and Walker, S. (2004). Relationships between Photosynthetically Active Radiation and Clearness Index at Bloemfontein, South Africa. Theor. Appl. Climatol. 80, 17–25. doi:10.1007/S00704-004-0080-5
Urban, O., Klem, K., Ač, A., Havránková, K., Holišová, P., Navrátil, M., et al. (2012). Impact of Clear and Cloudy Sky Conditions on the Vertical Distribution of Photosynthetic CO2uptake within a Spruce Canopy. Funct. Ecol. 26, 46–55. doi:10.1111/J.1365-2435.2011.01934.X
Wang, S., Huang, K., Yan, H., Yan, H., Zhou, L., Wang, H., et al. (2015). Improving the Light Use Efficiency Model for Simulating Terrestrial Vegetation Gross Primary Production by the Inclusion of Diffuse Radiation across Ecosystems in China. Ecol. Complex. 23, 1–13. doi:10.1016/J.ECOCOM.2015.04.004
Wang, S., Ibrom, A., Bauer-Gottwein, P., and Garcia, M. (2018). Incorporating Diffuse Radiation into a Light Use Efficiency and Evapotranspiration Model: An 11-year Study in a High Latitude Deciduous Forest. Agric. For. Meteorology 248, 479–493. doi:10.1016/J.AGRFORMET.2017.10.023
Wong, F. K. K., and Fung, T. (2013). Combining Hyperspectral and Radar Imagery for Mangrove Leaf Area Index Modeling. Photogramm. Eng. remote Sens. 79, 479–490. doi:10.14358/PERS.79.5.479
Xiao, J., Chevallier, F., Gomez, C., Guanter, L., Hicke, J. A., Huete, A. R., et al. (2019). Remote Sensing of the Terrestrial Carbon Cycle: A Review of Advances over 50 Years. Remote Sens. Environ. 233, 111383. doi:10.1016/J.RSE.2019.111383
Xie, H., Zhao, J., Wang, K., and Peng, H. (2021). Long-term Variations in Solar Radiation, Diffuse Radiation, and Diffuse Radiation Fraction Caused by Aerosols in China during 1961-2016. PLoS One 16, e0250376. doi:10.1371/journal.pone.0250376
Xie, X., Wang, T., Yue, X., Li, S., Zhuang, B., and Wang, M. (2020). Effects of Atmospheric Aerosols on Terrestrial Carbon Fluxes and CO2 Concentrations in China. Atmos. Res. 237, 104859. doi:10.1016/J.ATMOSRES.2020.104859
Xing, J., Wang, J., Mathur, R., Wang, S., Sarwar, G., Pleim, J., et al. (2017). Impacts of Aerosol Direct Effects on Tropospheric Ozone through Changes in Atmospheric Dynamics and Photolysis Rates. Atmos. Chem. Phys. 17, 9869–9883. doi:10.5194/ACP-17-9869-2017
Xue, W., Zhang, J., Ji, D., Che, Y., Lu, T., Deng, X., et al. (2021). Aerosol-induced Direct Radiative Forcing Effects on Terrestrial Ecosystem Carbon Fluxes over China. Environ. Res. 200, 111464. doi:10.1016/J.ENVRES.2021.111464
Xue, W., Zhang, J., Qiao, Y., Wei, J., Lu, T., Che, Y., et al. (2020). Spatiotemporal Variations and Relationships of Aerosol-Radiation-Ecosystem Productivity over China during 2001-2014. Sci. Total Environ. 741, 140324. doi:10.1016/J.SCITOTENV.2020.140324
Yang, L., Cao, Q., Yu, Y., and Liu, Y. (2020). Comparison of Daily Diffuse Radiation Models in Regions of China without Solar Radiation Measurement. Energy 191, 116571. doi:10.1016/J.ENERGY.2019.116571
Yang, X., Li, J., Yu, Q., Ma, Y., Tong, X., Feng, Y., et al. (2019). Impacts of Diffuse Radiation Fraction on Light Use Efficiency and Gross Primary Production of Winter Wheat in the North China Plain. Agric. For. Meteorology 275, 233–242. doi:10.1016/J.AGRFORMET.2019.05.028
Yang, Z., Li, Q., Xue, W., and Xu, Z. (2022). Impacts of Nature Reserves on Local Residents' Income in China. Ecol. Econ. 199, 107494. doi:10.1016/J.ECOLECON.2022.107494
Younes, S., Claywell, R., and Muneer, T. (2005). Quality Control of Solar Radiation Data: Present Status and Proposed New Approaches. Energy 30, 1533–1549. doi:10.1016/J.ENERGY.2004.04.031
Yue, X., and Unger, N. (2017). Aerosol Optical Depth Thresholds as a Tool to Assess Diffuse Radiation Fertilization of the Land Carbon Uptake in China. Atmos. Chem. Phys. 17, 1329–1342. doi:10.5194/ACP-17-1329-2017
Zeng, Z., Wang, Z., Ding, M., Zheng, X., Sun, X., Zhu, W., et al. (2021). Estimation and Long-Term Trend Analysis of Surface Solar Radiation in Antarctica: A Case Study of Zhongshan Station. Adv. Atmos. Sci. 38, 1497–1509. doi:10.1007/S00376-021-0386-6
Zeng, Z., Wang, Z., Gui, K., Yan, X., Gao, M., Luo, M., et al. (2020). Daily Global Solar Radiation in China Estimated From High-Density Meteorological Observations: A Random Forest Model Framework. Earth Space Sci. Sci. 7, e2019EA001058. doi:10.1029/2019EA001058
Zhang, J., Ding, J., Zhang, J., Yuan, M., Li, P., Xiao, Z., et al. (2020). Effects of Increasing Aerosol Optical Depth on the Gross Primary Productivity in China during 2000-2014. Ecol. Indic. 108, 105761. doi:10.1016/J.ECOLIND.2019.105761
Zhang, L., Yu, G., Sun, X., Wen, X., Ren, C., Song, X., et al. (2006). Seasonal Variation of Carbon Exchange of Typical Forest Ecosystems along the Eastern Forest Transect in China. Sci. China Ser. D. 49, 47–62. doi:10.1007/S11430-006-8047-2
Zhang, Q., M. Chen, J., Wang, H., Qiu, F., Yang, F., et al. (2017). Improving the Ability of the Photochemical Reflectance Index to Track Canopy Light Use Efficiency through Differentiating Sunlit and Shaded Leaves. Remote Sens. Environ. 194, 1–15. doi:10.1016/J.RSE.2017.03.012
Zhang, Y., Bastos, A., Maignan, F., Goll, D., Boucher, O., Li, L., et al. (2020). Modeling the Impacts of Diffuse Light Fraction on Photosynthesis in ORCHIDEE (V5453) Land Surface Model. Geosci. Model. Dev. 13, 5401–5423. doi:10.5194/GMD-13-5401-2020
Zhang, Y., Li, Q., Huang, H., Wu, W., Du, X., and Wang, H. (2017). The Combined Use of Remote Sensing and Social Sensing Data in Fine-Grained Urban Land Use Mapping: A Case Study in Beijing, China. Remote Sens. 9, 865. doi:10.3390/RS9090865
Zhou, H., Yue, X., Lei, Y., Tian, C., Ma, Y., and Cao, Y. (2021a). Aerosol Radiative and Climatic Effects on Ecosystem Productivity and Evapotranspiration. Curr. Opin. Environ. Sci. Health 19, 100218. doi:10.1016/J.COESH.2020.10.006
Zhou, H., Yue, X., Lei, Y., Tian, C., Ma, Y., and Cao, Y. (2021b). Large Contributions of Diffuse Radiation to Global Gross Primary Productivity During 1981-2015. Glob. Biogeochem. Cycles 35, e2021GB006957. doi:10.1029/2021GB006957
Zhou, H., Yue, X., Lei, Y., Zhang, T., Tian, C., Ma, Y., et al. (2021c). Responses of Gross Primary Productivity to Diffuse Radiation at Global FLUXNET Sites. Atmos. Environ. 244, 117905. doi:10.1016/J.ATMOSENV.2020.117905
Zhou, Y., Xiang, Y., and Shan, F. (1984). A Climatological Study on the Photosynthetically Active Radiation. Acta. Meteorol. Sin. 4, 387–397. doi:10.11676/qxxb1984.046
Zhou, Y., Wang, D., Liu, Y., and Liu, J. (2019). Diffuse Solar Radiation Models for Different Climate Zones in China: Model Evaluation and General Model Development. Energy Convers. manage. 185, 518–536. doi:10.1016/J.ENCONMAN.2019.02.013
Keywords: FLUXNET, diffuse radiation fraction, forest ecosystem, diffuse fertilization effect, carbon fluxes
Citation: Deng X, Zhang J, Che Y, Zhou L, Lu T and Han T (2022) The Effect of Diffuse Radiation on Ecosystem Carbon Fluxes Across China From FLUXNET Forest Observations. Front. Earth Sci. 10:906408. doi: 10.3389/feart.2022.906408
Received: 28 March 2022; Accepted: 03 June 2022;
Published: 24 June 2022.
Edited by:
Xiangjin Shen, Northeast Institute of Geography and Agroecology (CAS), ChinaReviewed by:
Meng Guo, Northeast Normal University, ChinaCopyright © 2022 Deng, Zhang, Che, Zhou, Lu and Han. This is an open-access article distributed under the terms of the Creative Commons Attribution License (CC BY). The use, distribution or reproduction in other forums is permitted, provided the original author(s) and the copyright owner(s) are credited and that the original publication in this journal is cited, in accordance with accepted academic practice. No use, distribution or reproduction is permitted which does not comply with these terms.
*Correspondence: Jing Zhang, amluZ3poYW5nQGJudS5lZHUuY24=
Disclaimer: All claims expressed in this article are solely those of the authors and do not necessarily represent those of their affiliated organizations, or those of the publisher, the editors and the reviewers. Any product that may be evaluated in this article or claim that may be made by its manufacturer is not guaranteed or endorsed by the publisher.
Research integrity at Frontiers
Learn more about the work of our research integrity team to safeguard the quality of each article we publish.