- 1Centro de Ciências e Tecnologias da Água (CTA), Universidade do Algarve, Faro, Portugal
- 2CERIS, Instituto Superior Técnico, Universidade de Lisboa, Lisboa, Portugal
- 3National Centre for Groundwater Research and Training, Flinders University, Groundwater Modelling Decision Support Initiative (GMDSI), Adelaide, SA, Australia
The Vale do Lobo sector of the Campina de Faro aquifer system in the Algarve (Portugal) is at risk of seawater intrusion. Managed Aquifer Recharge (MAR) is being considered to avoid groundwater quality deterioration. Numerical modelling was undertaken to assess the feasibility of several proposed MAR schemes. Although some data is available, many aspects of system behaviour are not well understood or measured. We demonstrate the use of a structurally simple but parametrically complex model for decision-making in a coastal aquifer. Modelling was designed to facilitate uncertainty reduction through data assimilation where possible, whilst acknowledging that which remains unknown elsewhere. Open-source software was employed throughout, and the workflow was scripted (reproducible). The model was designed to be fast-running (rapid) and numerically stable to facilitate data assimilation and represent prediction-pertinent uncertainty (robust). Omitting physical processes and structural detail constrains the type of predictions that can be made. This was addressed by assessing the effectiveness of MAR at maintaining the fresh-seawater interface (approximated using the Ghyben-Herzberg relationship) below specified thresholds. This enabled the use of a constant-density model, rather than attempting to explicitly simulating the interaction between fresh and seawater. Although predictive uncertainty may be increased, it is outweighed by the ability to extract information from the available data. Results show that, due to the limit on water availability and the continued groundwater extraction at unsustainable rates, only limited improvements in hydraulic heads can be achieved with the proposed MAR schemes. This is an important finding for decision-makers, as it indicates that a considerable reduction in extraction in addition to MAR will be required. Our approach identified these limitations, avoiding the need for further data collection, and demonstrating the value of purposeful model design.
1 Introduction
Seawater intrusion is a global issue exacerbated by increasing dependence on coastal groundwater resources, sea level rise and climate change. Most severe cases of salinization occur where groundwater levels fall below mean sea level and the groundwater flow direction turns landward (Werner, 2017). The interactions between fresh and saline groundwater involve complex density-dependent and hydrochemical processes, and are therefore inherently difficult and expensive to monitor, investigate and manage (Werner et al., 2013).
Corrective measures for aquifers already impacted by, or at risk of, seawater intrusion essentially comprise two options: reducing extraction rates, or artificially increasing recharge (Abarca et al., 2006). Both are often prevented or limited due to regulatory issues. It is often not possible to revoke existing groundwater abstraction licences, and decisions to reduce groundwater use have far-reaching economic, political, and social consequences. Managed Aquifer Recharge (MAR) includes a suite of methods to enhance aquifer recharge that are increasingly used to maintain, enhance, and secure groundwater systems under stress (Dillon et al., 2019). However, many countries lack detailed regulations making implementation challenging (Yuan et al., 2016). Although global implementation of MAR is increasing, it is not keeping pace with increasing groundwater extraction (Dillon et al., 2019).
MAR is expensive, particularly for seawater intrusion barriers, or where deep recharge boreholes are needed (Vanderzalm et al., 2022). Further pre-treatment of water prior to discharge is often necessary, particularly where urban wastewater or storm water is used (Dillon et al., 2019). Such schemes have high capital and operational costs. However, in comparison to desalination of seawater as an alternative water source, additional treatment of wastewater incurs lower energy costs, and fewer environmental impacts (Koussis et al., 2010).
Given the costs associated with MAR, and the challenges in predicting seawater intrusion, identifying the appropriate course of action is difficult. It is hard to demonstrate to stakeholders why, and when, action is necessary. However, decision-making under uncertainty is the norm for most decisions of consequence in groundwater management (Caers, 2011). Notwithstanding, decision-makers need to be informed of the risks surrounding their decisions. This requires quantifying the uncertainty of decision outcomes. Modelling supports decision making by providing the means to consolidate available data and information to both quantify, and reduce, the uncertainty surrounding outcomes of a management action (Doherty and Moore, 2021).
The subsurface is complex, and data on aquifer properties and boundary conditions are typically very limited. Expressing their uncertainty requires the use of many parameters to allow spatial variability to emerge through history-matching of the model to the historical behaviour of the system. The methods employed by industry standard tools for history-matching, PEST (Doherty, 2020) and PESTPP (White et al., 2020) software suites, need to run models many times to calculate parameter sensitivities. Therefore, incorporating existing information on system properties and past system behaviour into a model that is capable of quantifying and reducing uncertainty, requires a model that is fast and stable. To accomplish this, model development and deployment must be purposefully designed to achieve these two goals (Caers, 2011; Doherty and Moore, 2021). Models that simulate the effects of density changes between fresh and seawater require fine spatial and time discretization, have long run times, and are susceptible to numerical instability (Dausman et al., 2010). As a result, model-based data assimilation and uncertainty quantification become difficult, if not impossible (Carrera et al., 2010). Therefore, an alternative approach is needed.
Using a simpler model of a coastal aquifer introduces limitations in the types of questions that the model can answer. These limitations can be overcome by purposeful design of the modelling workflow and the prediction. We present a case study of decision-support groundwater modelling to assess MAR as a solution to seawater intrusion in the Vale do Lobo aquifer, Portugal. We demonstrate the development and use of a constant-density, highly-parameterized model, building upon the methods described in Hugman and Doherty, 2022. This approach enables the assimilation of information from both expert knowledge and field measurements to quantify and reduce predictive uncertainty. The effectiveness of MAR is assessed in terms of whether MAR can raise hydraulic heads sufficiently to levels preventative of seawater intrusion; a simple metric enabling this feasibility stage assessment of MAR.
Details of the study area and conceptual model are provided in Section 2, an outline of the problem, modelling rationale and design are described in Section 3. The numerical model configuration is described in Section 4, the data assimilation and uncertainty quantification process in Section 5, with discussion and conclusions presented in Section 6.
2 Study Area
The study area is located to the west of Faro, capital of the Algarve province of Portugal, and comprises the western part of the Campina de Faro aquifer system, known as the Vale do Lobo (VL) sector as shown in Figure 1. The aquifer covers an area of 32 km2. Groundwater from this coastal aquifer has been used extensively for irrigation over the last 50 years, for golf, tourism, and agricultural purposes. Long term annual average rainfall is approximately 600 mm/yr largely falling between November and April, whilst potential evapotranspiration is approximately 1,600 mm/yr with a substantial excess over rainfall during the summer months (DRAP-ALGARVE, 2021). Most irrigation is applied between the months of March and October and is almost entirely supplied from groundwater. Consequently, hydraulic heads are now below sea level across much of the aquifer (0 to -9 m above sea level (asl)), and several boreholes can no longer be used due to chloride concentrations of 927–2,242 mg/l measured in 2019 (Fernandes et al., 2020)). Currently the VL sector does not meet the regulatory requirement of “good” quantitative status under the EU Water Framework Directive (WFD), where groundwater extraction is required to be less than 90% of average annual recharge. The aquifer is at risk of further deterioration and losing “good” status based on water quality considerations considering that the Portuguese threshold value for chloride is 250 mg/l (APA, 2016). Stakeholders are interested in understanding to what extent Managed Aquifer Recharge (MAR) can be part of the solution to reverse the decline in hydraulic heads and prevent further seawater intrusion, recognising that achieving an aquifer-scale solution to SWI is a very ambitious aim, likely to require a combination of methods.
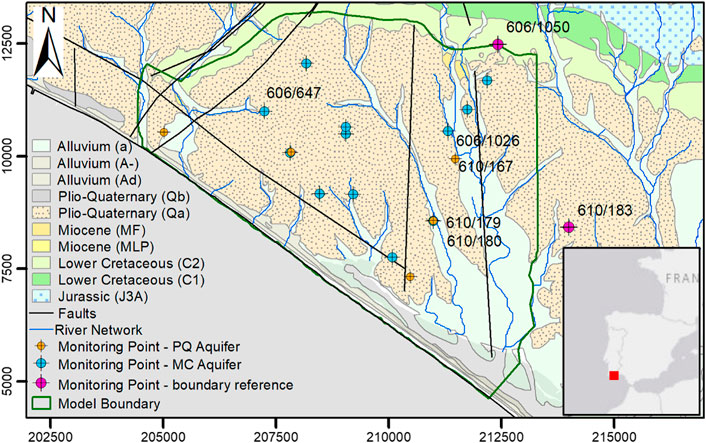
FIGURE 1. Location and main hydrogeological features of the Vale do Lobo aquifer system, including piezometer locations.
The VL sector is bounded to the east by an administrative boundary which divides the western sector of the aquifer (at risk of seawater intrusion), from the Faro sector to the east, where the problems affecting groundwater status are related with excess nitrates due to agriculture (Stigter et al., 2011). These sectors were defined to enable appropriate independent measures for each sector to be defined in the River Basin Management Plans (RBMPs) (APA, 2016) to meet WFD requirements. To the northwest, the VL boundary is defined by the Carcavai fault zone. An outcrop of Lower Cretaceous strata form the northern boundary of the VL sector, with Jurassic sediments forming a karstic aquifer further to the north.
The aquifer is formed of a thick sedimentary sequence of superimposed sedimentary basins of Mesozoic and Cenozoic age, underlain by Palaeozoic basement. The VL sector is comprised of two aquifers, an upper phreatic sand to sandy clay aquifer of Plio-Quaternary (PQ) age, and a lower semi-confined aquifer of calcareous sandstones and limestones of mainly Miocene (MC) age. A clay aquitard, with an average thickness of 10 m, separates these two aquifers. The PQ is absent at the northern boundary of the VL and increases to a maximum thickness of around 70 m in the south-east, where it is postulated that PQ sediments infilled a karstic depression in the MC surface (Carvalho et al., 2012). The PQ is highly heterogenous with 5 distinct layers mapped (Manuppella et al., 2007).
Although deep borehole records are limited, correlation with offshore and onshore borehole logs suggest that the MC aquifer reaches a depth of 350 m below mean sea level at the coast. It is underlain by the same low permeability marls of Lower Cretaceous age that form the northern boundary of the aquifer (Lopes et al., 2006). In addition to the faulted north-western boundary of the aquifer, two NNW-SSE-oriented faults transect the eastern part of the VL area (Manuppella et al., 2007). Their locations are somewhat uncertain; it is possible that their alignment is closer to that of the streams than depicted in Figure 1. A strike-slip fault is also located parallel to the coast approximately 1 km inland.
Most groundwater extraction is now from the MC aquifer, although the PQ aquifer was exploited historically by shallow, large diameter wells (Almeida et al., 2000). Current groundwater extraction is estimated at 6.45 Mm3/yr (APA, 2016), based on measured extraction for the major groundwater users, and estimated extraction based on land cover and crop type for the smaller users who are not required to submit extraction returns. Detailed borehole construction records are limited, and it is often unclear if, and where, extraction is occurring from the phreatic aquifer.
The environmental regulator, the Agência Portuguesa do Ambiente (APA), estimates long term annual diffuse recharge to the VL sector is 3.46 Mm3/yr. However, diffuse recharge is limited by the weathered red clays found at the surface, and it is recognized that a major, but unquantified, source of water to the aquifer is likely to be groundwater flowing laterally from the northern boundary from Cretaceous and Jurassic strata (Almeida et al., 2000; Hugman, 2016).
Hydraulic heads are regularly monitored by APA and are available for boreholes in the long-term monitoring network (SNIRH, 2021). Additional heads measured monthly by the groundwater users in piezometers and extraction boreholes were also made available for use in this study. The location of hydraulic head time series used for history matching are shown in Figure 1.
Piezometric contours of the MC aquifer, along with selected hydraulic head time series, are shown on Figure 2A. The contours show that hydraulic heads are below sea level (between 0 and −9 m asl) across most of the aquifer, with the lowest values in the centre and north of the aquifer, resulting in radial flow towards this depression. Time series from three boreholes with the longest period of record are shown in Figure 2B, indicating that hydraulic heads were already declining during the 1980s, possibly reaching a new equilibrium since the late 1990s with higher seasonal variation, in both the PQ and MC aquifers. Hydraulic heads in the PQ are only measured in 5 locations, but these generally show slightly higher heads with reduced seasonal fluctuations compared to heads in the MC. Piezometers 610/179 (MC) and 610/180 (PQ) are adjacent to one another and represent the only location where heads are measured simultaneously in both aquifers.
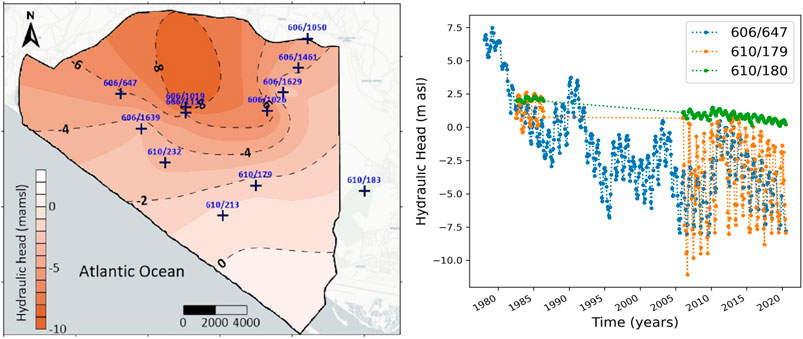
FIGURE 2. Hydraulic head contours from semi-confined aquifer, October 2018 (A), Selected hydraulic head time series at piezometer locations 606/647 (semi-confined), 610/179 (semi-confined), and 610/180 (phreatic) (B).
Time series measurements of chloride concentrations over time are only available at four locations in the VL sector, with 2 of these exhibiting increasing trends (SNIRH, 2021). A monitoring program during 2019/2020 encountered chloride concentrations up to 2,200 mg/l in extraction boreholes, with land managers reporting that several boreholes are no longer used as their chloride concentrations are too high for irrigation (Fernandes et al., 2020).
Previous numerical modelling studies covering this area have included density-driven flow (DDF) models to investigate seawater intrusion (Hugman, 2016), assessment of nitrate contamination in the eastern sector of the Campina de Faro (Costa et al., 2021), and to assess the potential of using greenhouse runoff as water source for MAR (Costa et al., 2020). More recently, (Hugman et al., 2021) investigated sustainable extraction rates to avoid seawater intrusion.
3 The Problem
It is clear the current rates of extraction from the VL sector are unsustainable. Meeting the water balance requirement of the WFD will not prevent seawater intrusion. Without other mitigation measures, groundwater extraction would need to be reduced to 30% of current rates in VL, possibly even less (Hugman and Doherty, 2022). This would be exceedingly difficult to achieve in practice. There are few viable alternatives, and these are expensive, i.e., replacing groundwater use with desalinated seawater.
MAR has been identified as a potential mitigation measure. However, additional treatment is likely to make it an expensive option, the water available for MAR is limited, and legal issues would need to be overcome. Before committing to further investment in investigating MAR options, decision-makers need to understand whether it is likely to prevent seawater intrusion in this aquifer.
3.1 MAR Design and Water Availability
Two types of water are potentially available for MAR in this area: 1) ephemeral river flow, and 2) treated wastewater. The Ribeira da São Lourenço 1) flows from north to south close to the eastern boundary of the aquifer, with average annual flow of 1.25 Mm3/yr between 1996 and 2008. Flow occurs on average 77 days per year. No flow is recorded in some years. Preliminary pre-settlement basin designs limit the average MAR recharge from this source to 0.5 Mm3/yr (Standen et al., 2021).
Treated wastewater 2) is available from three treatment works in the area: Quinta do Lago, Vale do Lobo and Faro Noroeste. In 2020, available volumes were 0.76, 0.16 and 1.50 Mm3/yr respectively (written communication, Águas do Algarve, S.A.).
The preferred MAR design would use surface infiltration basins recharging into the PQ, thereby avoiding direct injection into the MC, and allowing soil-aquifer treatment in the unsaturated zone. However, the current understanding of the permeability of the PQ and the presence of the aquitard suggests this option is unlikely to be feasible. Therefore, recharge is proposed by boreholes into the MC, at locations close to the water sources, as shown on Figure 3.
3.2 Modelling Rationale and Prediction
3.2.1 Rationale
To model the physical coastal aquifer processes requires density-coupled flow and transport models. These require fine spatial and time discretization, with typically very long run times, and are susceptible to numerical instability. They also require the offshore part of the system to be characterized and included in the model, yet these aspects of the system are often poorly known. Sharp-interface codes offer an alternative, but simulated outcomes can be quite sensitive to initial conditions, definition of the coastal boundary condition, and they still require the offshore portion to be modelled explicitly (Bakker and Schaars, 2013; Coulon et al., 2021).
To achieve a fast and stable numerical model, process complexity is reduced by using a constant-density model. We assume that the changes in density do not play a large role in the aquifer response during the simulation period. Although this simplification will introduce some error, this will likely be small in comparison to other sources of uncertainty in the model (Caers, 2011; Doherty and Moore, 2021). The prediction cannot be based on chloride concentrations; therefore, an alternative prediction is described in Section 3.2.2 below.
The model structure is simplified in terms of reducing the model layers and extent. The rigid structure of the offshore portion of the model is replaced by flexible parameters which represent the offshore extent. This allows us to stochastically represent the uncertainty in aquifer structure and properties offshore through physically abstract parameters. It removes the need for an offshore extent entirely, reducing the number of grid cells significantly, whilst also avoiding hard wiring assumed (but unknown) offshore structure and properties into the model. The number of layers is then limited to main hydro-stratigraphic units that are likely to control the hydraulic head response to the current pressures, and the proposed artificial recharge.
Initial assessment of the water volumes available compared to the estimated aquifer water balance indicates that these volumes may be insufficient to achieve the aquifer-scale improvements in hydraulic heads necessary to prevent SWI. However, given the uncertainties in the water balance, and the interest from regulators and stakeholders in MAR, the modelling presented herein investigates the feasibility of MAR in more detail.
3.2.2 The Prediction
Modelling undertaken herein aims to determine whether MAR can prevent seawater intrusion, an ambitious but important aim. The depth of the fresh-seawater interface as a function of hydraulic head can be obtained using the Ghyben-Herzberg relationship (Bear and Verruijt, 1987), based on the assumptions of static equilibrium, stationary seawater, and assuming that a sharp interface exists between fresh and salt water:
where z is the position of the interface below sea level [m], α [-] is defined as ρf /(ρs - ρf ), where ρf [M/L3] and ρs [M/L3] are the fresh water and sea water densities respectively, and h is the hydraulic head [m]. The minimum value of hydraulic head that ensures the fresh-seawater interface does not rise above a specified depth can be calculated using Eq. 1.
The effectiveness of MAR is assessed on its ability to maintain hydraulic heads at levels that ensure that the interface remains deeper than a critical value at specified locations (e.g., deeper than the base of existing extraction boreholes). This is admittedly a coarse metric. It ignores the effects of dispersion, the (potentially wide) transition zone between fresh and seawater, and up-coning in response to individual extractions. However, it is a metric that allows preliminary assessment at the aquifer scale of the feasibility of the scheme. Modelling in this context cannot ensure that MAR will be successful; however, it can determine if MAR will not be successful. As the purpose of this exercise is to assess whether it is worth exploring these schemes further, such a prediction is sufficient, and more robust, than attempting to simulate the full complexity of processes and structure.
4 Numerical Model Development
The groundwater model was constructed using MODFLOW6 (MF6) (Langevin et al., 2021), using the open source Flopy environment (v.3.3.4) (Bakker et al., 2016). The model has three stress periods: an initial steady state period to obtain representative heads and extraction rates for the start of the second stress period; a transient period from October 2000 to October 2020. A third stress period extends the model for 20 years incorporating MAR, with the same hydrological inputs and extraction rates from the calibration period. It was not possible to start from a pre-development scenario, due to a lack of head data from this time.
The model was discretized with 400 × 400 m cell size, with quadtree mesh refinement applied using the open-source software, GRIDGEN (Lien et al., 2015). Cell sizes were reduced adjacent to potential drains and the MAR borehole locations. The model has three layers representing the phreatic aquifer, the aquitard, and the semi-confined aquifer (all layers were necessary to capture the hydraulic head response under MAR). To avoid discontinuous layers, the upper layer was assigned a minimum thickness where necessary.
The lumped parameter recharge model, LUMPREM (Doherty J., 2021), was used to estimate both recharge and groundwater withdrawal for irrigation, based on daily rainfall and potential evapotranspiration from the Faro-Patacão meteorological station (DRAP-ALGARVE, 2021). Recharge is applied to layer 1 (the phreatic aquifer), at rates depending on rainfall, irrigation, evapotranspiration, and the capacity and current volume of the soil-moisture store. Recharge occurs up to the potential evapotranspiration rate until the soil moisture store is empty, with rates decreasing as the volume of the soil moisture store decreases (the shape of this function is controlled by the gamma parameter). Transfer of rainfall-recharge to layer 3 (the semi-confined aquifer) is limited by the presence of the clay aquitard separating the two aquifers.
Using LUMPREM allowed estimation of groundwater extraction based on irrigation demand, thereby accounting for missing extraction data. It can be integrated with MF6 and PEST by the python package Lumpyrem (Hugman, 2021). The combined model (MF6 + LUMPREM) includes LUMPREM models for each of the major groundwater users, the extensive agriculture (non-metered) group, and non-irrigated land. Recharge was applied to areas defined by grid intersection with the respective land uses. Total groundwater withdrawal for irrigation was applied as time-varying total extraction rates for each group, these are then sub-divided between individual extraction wells. The locations of irrigated areas and extraction boreholes/wells are shown on Figure 4 in relation to the model grid and boundaries.
The inland boundaries are represented by Cauchy (i.e., general head) boundary conditions applied to the semi-confined aquifer. These represent the inflows to the MC aquifer from the Jurassic aquifer to the north, and the eastern sector of the Campina de Faro aquifer to the east). The heads vary according to time series measured at 606/1050 and 610/183 for the northwestern, and eastern boundaries respectively (at locations shown on Figure 1). Definition of the coastal boundary condition for the semi-confined aquifer is described in Section 4.1, whilst for the phreatic aquifer, a head correction of 1.0124 was applied, based on the method of Lu et al. (2015). For all the boundaries, conductance is time-invariant, as are heads for the coastal boundary.
4.1 Coastal Boundary
As previously described, there is little to no data on hydraulic properties or system behaviour in the offshore portion of the aquifer system. Rather than attempt to simulate it explicitly, we represent the offshore conditions implicitly with a general head boundary, using the approach described in Hugman and Doherty (2022). This enables us to limit the model domain to the onshore portion of the system, where freshwater conditions are assumed to prevail. In turn, this allows us to ignore the effects of density differences and use a fast-running model that enables data assimilation and uncertainty analysis.
General head boundaries require specification of head and conductance parameters. Conceptually, these parameters represent the linkage between the model and the offshore portion of the system. However, they omit the effects of changes in offshore storage and assume that the dynamics of offshore flow do not change significantly during the simulated period. As such, these head and conductance parameters take on a somewhat “abstract” nature. As they are no longer physically-based, these parameters are no longer useful recipients for expert knowledge, And as they are not informed by measured data, uncertainty can be large.
The approach described in Hugman and Doherty (2022) enables the transfer of expert knowledge to these abstract parameters through the use of a simple-complex model pair. The “complex” model simulates physical process which are omitted from the “simple” model. The complex model is simulated for an ensemble representative of stresses and hydraulic properties. Values for the abstract parameters in a corresponding “simple” model are calculated for each realization. This allows the statistical distribution of abstract parameters to be characterized.
For the VL, this is achieved with use of a complementary two-dimensional DDF model (using SEAWAT) of the VL semi-confined aquifer. It was run for a long pre-development period (during which flow is towards the sea), followed by a post-development (land-ward flow) period. A total of 100 stochastic realisations were created, sampling from the prior probability distribution of aquifer properties and inland heads based on the aquifer conceptualization (expert knowledge). By recording head and flow for both pre- and post- development conditions for each realization, values of head and conductance at the coastal boundary were obtained through the following equations:
For sea-ward flow and land-ward flow conditions respectively. These two equations can be solved for the two unknowns h and c. The solutions are:
Where H is the freshwater head at the coastline [m], q is groundwater flow under the coastline [m3/d], and for a general head boundary along the coastline, h represents the head [m], and c the conductance [m2/d]. The subscripts o and i represent outflow (pre-development) and inflow (post-development) conditions respectively. Values of Ho and Hi and values of qo and qi are obtained from the complex model for each realization. The mean and covariance of the heads and conductance can be calculated to form the combined covariance matrix:
Where c is the value of log10 conductance [log10 m2/d] at the coastal boundary,
The values of h and c for a single point are used to characterize the full length of the coastal boundary by pilot points. However, values of h and c are expected to show some degree of spatial correlation along the boundary. Therefore, a joint probability distribution is required. Values were selected from a probability distribution that has the mean of
5 Data Assimilation and Uncertainty Quantification
5.1 Methods
For the combined model a solution of minimum error variance (MEV) was sought using PEST_HP (Doherty, 2020), employing a highly-parameterized approach. A unique solution was obtained using Tikhonov (preferred value) regularization. This was followed by history-matching and uncertainty quantification (and reduction) using PESTPP-IES (White, 2018).
5.1.1 Parameterisation and Prior Information
An array of 962 pilot points distributed across the model domain, layers and boundaries allowed spatial variation of parameters. For aquifer properties these included horizontal hydraulic conductivity (K) for all layers (and thus for ratio-linked vertical hydraulic conductivity), specific yield (layer 1), and storativity (layer 3). Pilot points were placed manually, located between observation points and extraction well/borehole locations, and between these features and the model boundaries. Pilot points were also included along model boundaries and drains to allow spatial variation in boundary condition parameters.
Recharge and groundwater withdrawal for irrigation vary by land use zones linked to LUMPREM models, the parameters of which are adjustable. Prior to coupling LUMPREM and MF6, LUMPREM model parameters were first calibrated against measured extraction rates. Obtained values were subsequently used as initial parameter values when calibrating the combined model. LUMPREM provided a time series of groundwater extraction totals for each major groundwater user, and these were subdivided into groundwater extraction rates at each extraction point with a multiplier. As the extraction rates at each well were unknown, the multipliers were allowed to vary if needed during the calibration process.
The prior estimates of parameters, including the LUMPREM parameters, are shown in Supplementary Table S1 of the Supplementary Material, with the mean of the prior probability distribution representing preferred values in the regularization. The model is parameterized with a total of 1,437 adjustable parameters. Parameter field uniqueness is achieved through numerical regularization which seeks minimum departure of each parameter from a user-specified “preferred value.” For spatially varying parameters, covariance matrices are used instead of regularization weights to ensure smoothness of emergent parameter fields.
5.1.2 Observations and Weighting
In total, 5,103 observations were included as history matching targets. A total of 12 hydraulic head time series from the semi-confined, and 5 from the phreatic aquifer were used as history-matching targets. At one location, head differences between the two aquifers were also included as observations (610/179 and 610/180 in Figure 2B). Metered quantities of groundwater extraction reported to APA from 2010 onwards were also included as observations.
First-order temporal variations were calculated by subtracting each observation from the previous observation, giving equal importance to the temporal changes in the observation borehole time series as the actual measurement value (White et al., 2014; Foster et al., 2021; Hugman et al., 2021).
Soft data was also incorporated, with drains set at ground level across the entire model domain, and observations of zero flow included, where appropriate.
The weighting scheme aimed to give equal importance to matching of heads and extraction rates in the history-matching process. Heads were sub-divided into several observation groups to increase the weight of boreholes in different layers, and those that exhibited different responses. Groundwater extraction observations also were sub-divided to account for large difference in the temporal resolution of observations between the groups.
5.1.3 History Matching and Uncertainty Quantification
The PESTPP-IES iterative ensemble smoother generates alternative, calibration-constrained, parameter realizations, by sampling from a selected probability distribution (White, 2018). The parameter realizations are then iteratively adjusted until the model outputs attain a better fit to observations. In this case, the linear approximation to the posterior probability distribution was used as the starting point for PESTPP-IES, as often this can provide a better starting point for the process (Gallagher and Doherty, 2020).
Noise was added to the non-zero weighted observations by replacing the observation weights used during the history matching process with the inverse of the standard deviation of measurement noise. These were applied to heads (0.1 m) and pumping rates (0.5–2.5 m3/d ), with larger uncertainty applied to the non-metered groundwater users. The PEST utility RANDOBS was used to generate realisations containing noise-enhanced observations. The number of realisations (200) was selected to be more than double the number of uniquely identifiable pieces of information in the calibration dataset (90) identified by the PEST utility SUPCALC (Doherty J. E., 2021) following other recent studies (Hayley et al., 2019).
5.2 Results
5.2.1 Calibration
The resulting MEV parameter set achieved a good fit to measured observations of both hydraulic heads and groundwater extraction. In general, a better fit was obtained for heads in the semi-confined aquifer compared to the phreatic (as shown in Figure 5). This is not surprising, as there are fewer head observation points in the phreatic aquifer. The PQ formation is known to be highly heterogeneous, and it is difficult to determine if, and where, extraction is occurring from this phreatic aquifer. As the fit of 610/167 only improved once extraction was permitted from both aquifers, this suggests that extraction is occurring from the PQ in this area.
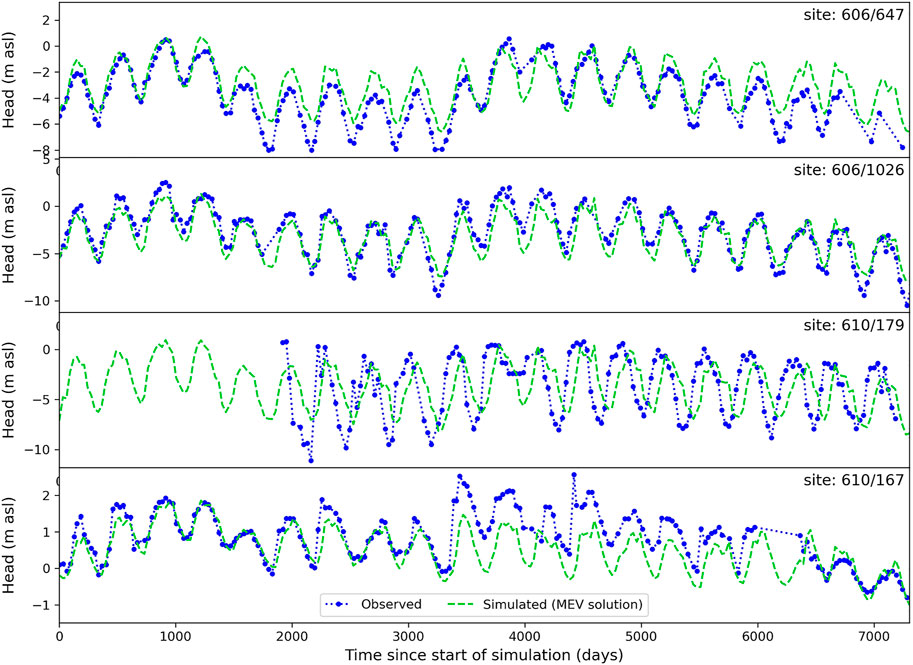
FIGURE 5. Measured and simulated hydraulic heads for 606/647, 606/1026 and 610/179 from the semi-confined aquifer, and 610/167 from the phreatic aquifer.
Simulated and observed extraction rates are shown in Figure 6. In general, simulated extractions match measured extractions well, particularly in the central and eastern parts of the model.
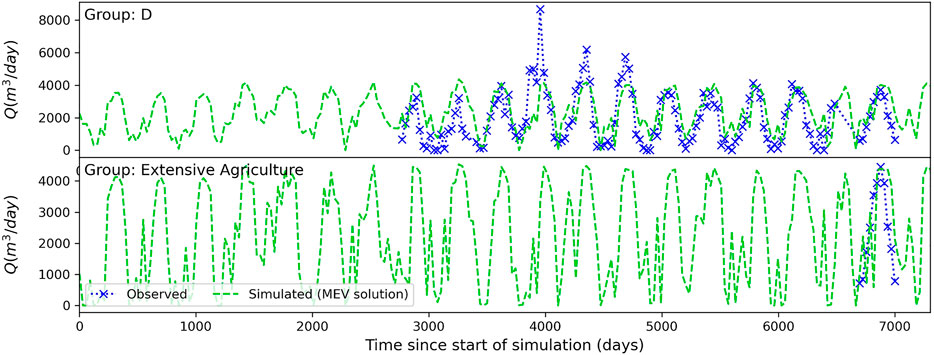
FIGURE 6. Measured and simulated extraction rates for user group D, and the extensive agriculture group.
Calibrated total annual average recharge values of 0.33–0.59 Mm3/yr, with an average of 0.44 Mm3/yr, were obtained. These values are an order of magnitude lower than the APA estimate (3.46 Mm3/yr), which has been recognised as an over-estimate by several authors (Almeida et al., 2000; Hugman, 2016). The calibrated recharge values reflect the conceptual understanding that weathered red clays at the ground surface are of low permeability, limiting diffuse rainfall-recharge to the phreatic aquifer. The lowest recharge rates occur under the non-irrigated land (2 mm/yr), which accounts for 25 km2 of the total 32 km2. The other land uses have higher recharge rates (4–295 mm/yr) and include irrigation return. Diffuse recharge is largely prevented from reaching the semi-confined aquifer by the presence of the aquitard, with the majority of inflow occurring at depth from the adjacent aquifer systems.
5.2.2 History-Matching
Of the 200 realizations, 138 resulted in model convergence. The remaining model runs generally failed due to convergence issues related to drying of the upper layers. History matching results are shown in Figure 7 for the same piezometers as Figure 5, along with the MEV results. The ensemble encompasses almost all the observations, apart from piezometer 610/179 where heads recover earlier in the year than the model predicts, indicating that extraction in this location perhaps ceases earlier in the year than expected by the soil-moisture balance. The ensemble resulted in a wider distribution of heads in the phreatic aquifer, as shown by 610/167, where although the temporal variation in heads matches the measured data well, there is a large range of predicted groundwater levels in this location. This occurred despite increasing the weight of the phreatic aquifer observations.
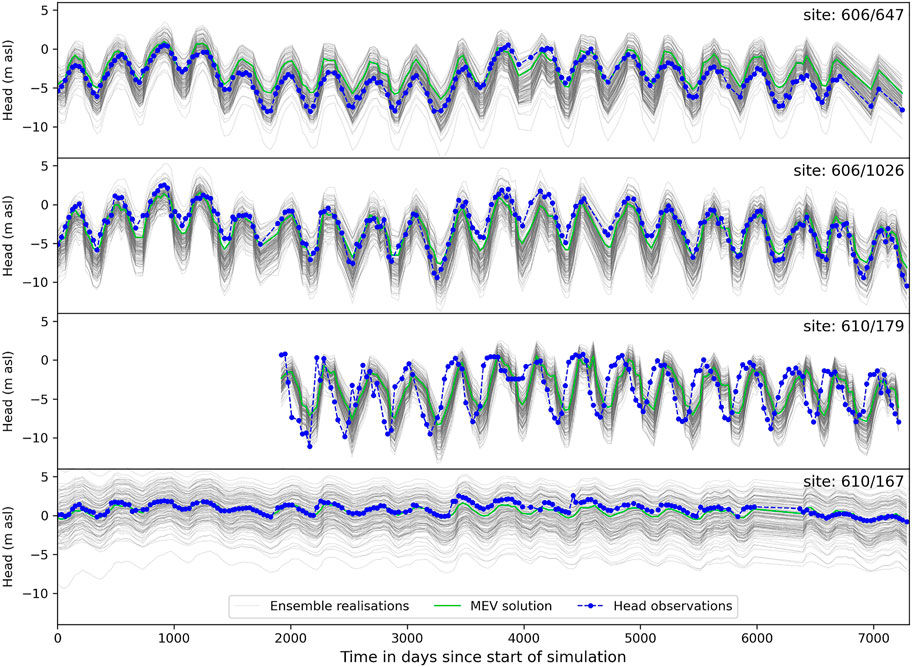
FIGURE 7. Measured and ensemble of simulated hydraulic heads for 606/647, 606/1026 and 610/179 from the semi-confined aquifer, and 610/167 from the phreatic aquifer.
5.2.3 MAR Scenario Results
The impact of MAR at the locations denoted Marsl (Ribeira da São Lourenço), Marww1 (Quinta do Lago), Marww2 (Vale do Lobo) and Marww3 (Faro Noroeste) is shown in Figure 8, where the ensemble of predicted heads is plotted against the minimum head required at each location. Results at extraction boreholes are not shown, as the impact of MAR is negligible.
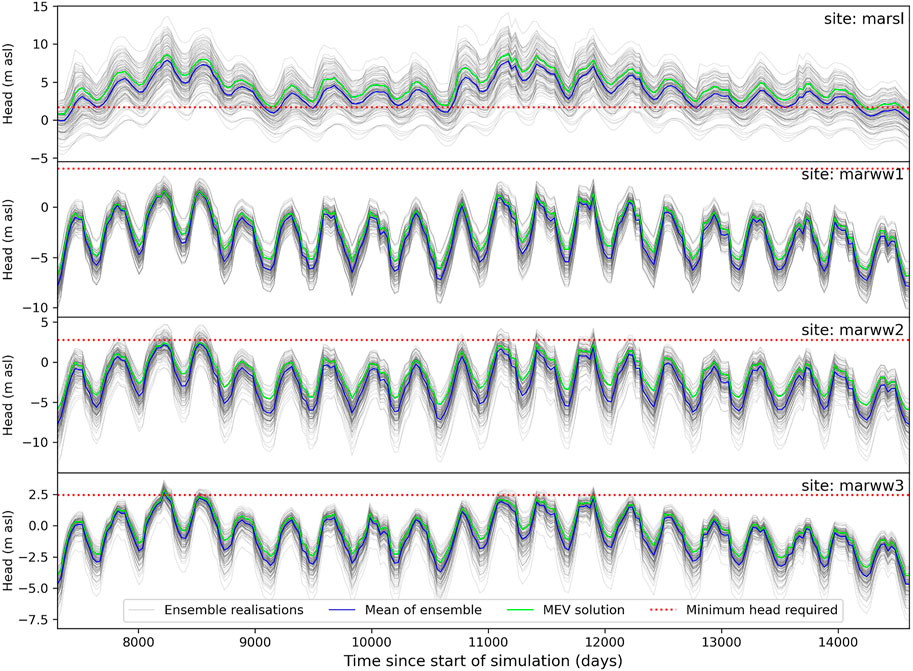
FIGURE 8. Predicted hydraulic heads at MAR locations, showing MEV model results (green), each ensemble member (grey), mean of ensemble (blue), and the minimum head requirement at that location (red dashed).
At Marsl, the heads are highly dependent on the variability of ephemeral flow, with large increases occurring during recharge periods. However, these are short-lived, falling rapidly to levels similar to the minimum head requirement when additional recharge is not occurring. This indicates that MAR is probably not necessary at this location; a location further downstream would be more beneficial.
At the other MAR locations, the minimum head requirement is only met during limited times and for some realisations. A no-MAR scenario was run to identify the head improvements resulting from MAR. The time-averaged head differences show only limited improvement in hydraulic heads as shown in Table 1 (averages are not appropriate for Marsl due to the ephemeral flow variability and are not presented). Time series of head differences, in the Supplementary Figure S1, indicate that head improvements occur rapidly after the implementation of MAR.
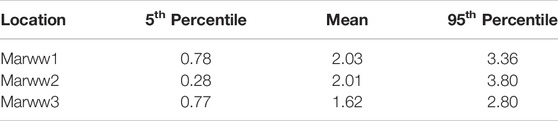
TABLE 1. Average head differences (m) at MAR locations during 20 years simulation period (MAR scenario minus no-MAR scenario).
These results raise the question whether it is possible to reach the minimum heads under any scenario, remembering that heads for the pre-development period are unknown. This was examined by undertaking an additional scenario with no extraction (and no MAR). The minimum heads required at each extraction borehole were compared to the predicted heads (5th percentile of the ensemble) at those locations, confirming that the minimum heads could be met with a small number of exceptions (see Supplementary Table S2). These occurred where extraction boreholes are deep (up to 200 m), or where the boreholes were located close to the eastern boundary. Here, the average heads are already low (1.1 m asl), preventing the minimum head requirement from being met close to the boundary. This provides confidence that somewhere between no-extraction and current extraction plus MAR, a management solution to protect the aquifer exists.
5.2.4 Insights From Linear Analysis
The spatial distributions of hydraulic conductivity for each layer from the MEV parameter set are plotted in Figure 9, along with the corresponding values of the relative parameter uncertainty variance reduction (RUPVR) (Doherty J. E., 2021). This ratio varies from 0 to 1, with higher values indicating the locations where posterior parameter uncertainty has been reduced in comparison to the prior during history-matching. Of particular interest is the area in the centre of the model, which appears to have relatively higher K in both layer 1 and layer 2, where the RPUVR shows that the uncertainty has been reduced to a greater extent than the surrounding area. This is an important insight, which could justify further site investigation for a potential infiltration basin MAR scheme in this location.
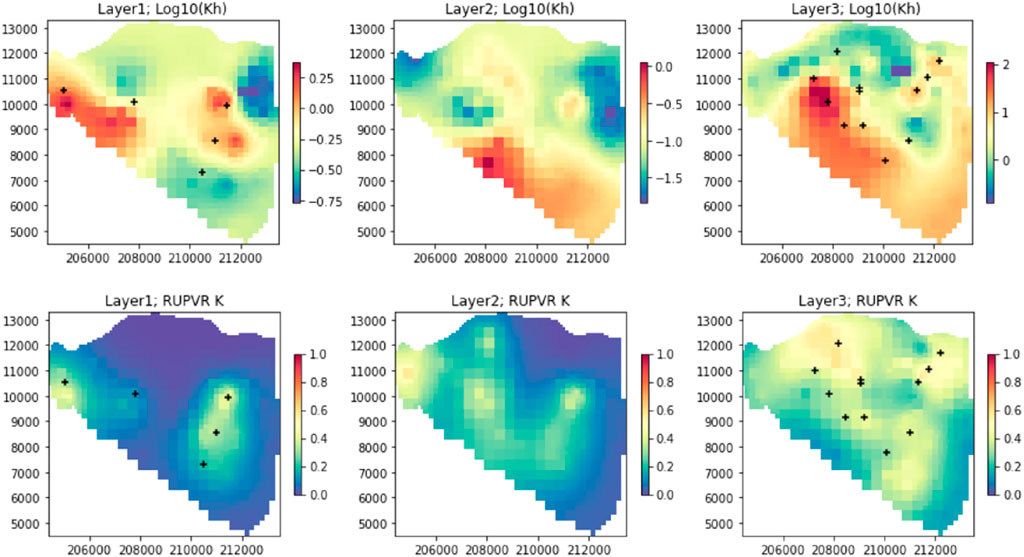
FIGURE 9. Spatial distribution of (log) hydraulic conductivity in layers 1 (phreatic), 2 (aquitard) and 3 (semi-confined) (top, left to right), and RPUVR of hydraulic conductivity in layers 1, 2 and 3 (bottom, left to right), location of hydraulic head observations for each layer indicated by black crosses.
Values of RUPVR were low for pilot points along the boundary conditions, with mean values of 3 × 10−2 to 6 × 10−6 obtained for conductance and head values, indicating that history matching was not effective in reducing uncertainty in the boundary condition parameters, outlining the importance of constraining the prior probability distributions by the method described in Section 4.1.
6 Discussion and Conclusions
6.1 The Workflow
The combined model was scripted, and therefore reproducible. Furthermore, conceptual changes identified during the model construction and calibration process could easily be altered in the base model. Whilst the model was designed to have a fast run time, the scripting involved significant time investment and cognitive effort for the modeller in moving away from GUI based methods to the open-source tools described herein, but it was considered to be worth it for the resulting flexibility and reproducibility.
The model development and deployment were considered simultaneously, with reduced process complexity (constant-density) and structural complexity (modelling the offshore extent and processes as described in Section 4.1). The resulting model was capable of uncertainty quantification and reduction, but with limitations in terms of the predictions it can make. For an initial first-order assessment, evaluating the effectiveness of MAR against minimum heads was an acceptable compromise. This relatively simple metric quickly identified that MAR was not likely to be successful and thus no further effort was put into a more comprehensive analysis. If this had not been the case, further efforts into designing adequate metrics would have been warranted. An alternative (or complementary) analysis could use the results from the complementary DDF model to determine the relation between fresh-saltwater interface response to changes in flux across the GHB coastal boundary. If a defensible relation between change in flux and gradient reversal could be established, this would allow the magnitude of change in GHB flux to be used as a metric for effectiveness.
Calibrating with PEST_HP was time-consuming. Balancing the weights requiring subjective expert knowledge about the important features of the system. To obtain an acceptable fit across all observation groups required testing of multiple weighting strategies. However, calibration allowed the use of linear analysis. This identified (with the RPUVR statistic) that the uncertainty of coastal boundary parameters was not reduced by history-matching. This provided further justification for the method used to stochastically characterise the coastal boundary, which constrained the prior probability distribution. It also enabled the linearized posterior probability distribution to be used as the starting point for PESTPP-IES, reducing the number of model convergence failures during this process (one-third of realizations failed to converge even with this workflow).
Where decisions need to be made relatively quickly to protect the aquifer, the use of a simpler model is beneficial. If building a complex model takes too long, decisions are likely to be taken before such a model is available (Caers, 2011). Furthermore, if a complex model cannot quantify and reduce uncertainty, a likely outcome given the nature of DDF models, then the decision-support such a model can provide is limited.
6.2 The VL Sector
This case study demonstrates the development of a decision-support groundwater model to assess the effectiveness of MAR to prevent seawater intrusion in a coastal aquifer system, whilst allowing reduction of prediction uncertainty through data assimilation in a highly-parameterized framework. Process complexity was reduced using a constant-density model, along with a complementary 2D DDF model, to allow stochastic characterisation of the head and conductance along the boundary. This allowed us to achieve the fast run times necessary to undertake history-matching and reduce predictive uncertainty.
Evaluating MAR by the ability to achieve minimum heads that prevent the seawater interface encroaching above the base of the current extraction boreholes is pragmatic. It permits a preliminary, aquifer-wide assessment, and allows regulators and stakeholders to understand the benefits and limitations of MAR with a simple metric. The results demonstrate that MAR cannot increase the hydraulic heads sufficiently to attain the minimum heads required, even locally. Therefore, the proposed MAR schemes cannot prevent the interface from reaching the base of the existing extraction boreholes, and seawater intrusion in the VL cannot be mitigated by MAR alone.
The minimum heads can be met for the majority of locations in a “no-extraction” scenario, the exception being deep boreholes close to the eastern boundary. Here heads are not sufficiently high enough to prevent seawater intrusion, indicating that the VL sector cannot be entirely protected from seawater intrusion even under this scenario without concurrent management action in the eastern part of the Campina de Faro.
This modelling, in conjunction with that of Hugman and Doherty (2022), identifies for the first time, the true scale of the problem in this area, and how difficult it will be to resolve. A significant reduction in extraction will be needed in addition to, or as an alternative to MAR. Hugman and Doherty (2022) have shown that extraction rates would need to be reduced at least to 30% of current rates in VL, possibly even less. Required reduction in extraction would be less in conjunction with MAR. An integrated approach to water management in the VL sector could use the available treated waste-water directly for irrigation as an alternative to MAR. Although this has not been explicitly modelled, the implication of our model results is that the waste-water volumes remain insufficient, and further reductions in extraction would still be required.
Predicted climate change impacts on rainfall indicate that for the RCP4.5 scenario, rainfall is expected to decrease by 10% in the south of Portugal, with an associated reduction in wet days of 10–20%, which will lead to associated reductions in recharge (Soares et al., 2017). River flows in the Mediterranean region are likely to be even more intermittent in the future due to climate change, with an increasing number of zero flow events (Schneider et al., 2013), reducing the availability of water for MAR from this source. Meanwhile, socio-economic and agricultural development in the region will result in increased water demand for irrigation (Stigter et al., 1998; Hugman et al., 2017). These compounding factors will result in higher demand at a time when less water is available. Without action, the aquifer will face even more severe pressures in the future.
Collecting further information on the aquifer properties and state of seawater intrusion, such as geophysics and further water quality studies, adds to the available body of knowledge, but it is time-consuming and expensive. Meanwhile, decisions are not taken. The existing data is already rich in prediction-specific information, as measured water levels are available close to where water level predictions are required. We have demonstrated an approach and associated model to support decision-making with the data currently available. This modelling has limitations, but we are still able to state with a relative degree of confidence that investing in MAR on its own is not going to solve the problem. In conjunction with Hugman & Doherty (2022), we have demonstrated that substantial further actions are needed to protect groundwater quality in the VL sector.
Data Availability Statement
Publicly available datasets were analyzed in this study. This data can be found here: The raw data is available from the websites referenced in the text (SNIRH and DRAP-ALGARVE). The remainder of the data was provided directly by the Agência Portuguesa do Ambiente, elements of which are confidential.
Author Contributions
KS and RH conceived the idea, KS analysed the data. KS (with support and guidance from RH) set up the modelling workflows and undertook all simulations and their postprocessing. RH completed the linear algebra to characterise the coastal boundary. KS and RH discussed the results and contributed to the final manuscript. JPM provided supervision, reviewed the manuscript and obtained the MARSoluT research funding.
Funding
KS received funding from the European Union’s Horizon 2020 research and innovation programme under the Marie Skłodowska-Curie grant agreement no. 814066 (Managed Aquifer Recharge Solutions Training Network—MARSoluT). RH received funding through the “Groundwater Modelling Decision Support Initiative” (GMDSI), conducted under the auspice of the National Centre for Groundwater Research and Training (NCGRT), Flinders University, South Australia, with funding from BHP and Rio Tinto.
Conflict of Interest
The authors declare that the research was conducted in the absence of any commercial or financial relationships that could be construed as a potential conflict of interest.
Publisher’s Note
All claims expressed in this article are solely those of the authors and do not necessarily represent those of their affiliated organizations, or those of the publisher, the editors and the reviewers. Any product that may be evaluated in this article, or claim that may be made by its manufacturer, is not guaranteed or endorsed by the publisher.
Acknowledgments
We thank Edite Reis of the Agência Portuguesa do Ambiente for providing additional data used in this study, and John Doherty for this advice during the project.
Supplementary Material
The Supplementary Material for this article can be found online at: https://www.frontiersin.org/articles/10.3389/feart.2022.904271/full#supplementary-material
References
Abarca, E., Vázquez-Suñé, E., Carrera, J., Capino, B., Gámez, D., and Batlle, F. (2006). Optimal Design of Measures to Correct Seawater Intrusion. Water Resour. Res. 42, 1–14. doi:10.1029/2005WR004524
Almeida, C., Mendonça, J. L., Jesus, M. R., and Gomes, A. J. (2000). Sistemas Aquíferos de Portugal Continental. Lisboa: INAG, Instituto da Água.
APA (2016). Plano de Gestão de Região Hidrográfica Das Ribeiras Do Algarve (Rh8) Parte 2 - Caracterização e Diagnóstico. Available at: https://apambiente.pt/sites/default/files/_SNIAMB_Agua/DRH/PlaneamentoOrdenamento/PGRH/2016-2021/PTRH8/PGRH_2_RH8_Parte2_Anexos.pdf.
Bakker, M., Post, V., Langevin, C. D., Hughes, J. D., White, J. T., and Starn, J. J. (2016). Scripting MODFLOW Model Development Using Python and FloPy. Groundwater 54, 733–739. doi:10.1111/gwat.12413
Bakker, M., and Schaars, F. (2013). Modeling Steady Sea Water Intrusion with Single-Density Groundwater Codes. GroundWater 51, 135–144. doi:10.1111/j.1745-6584.2012.00955.x
Bear, J., and Verruijt, A. (1987). Modeling Groundwater Flow and Pollution - Theory and Application of Transport in Porous Media. Dordrecht, Holland: D. Reidel Publishing Company.
Carrera, J., Hidalgo, J. J., Slooten, L. J., and Vázquez-Suñé, E. (2010). Computational and Conceptual Issues in the Calibration of Seawater Intrusion Models. Hydrogeology J. 18, 131–145. doi:10.1007/s10040-009-0524-1
Carvalho, J., Ramalho, E., Dias, R., Pinto, C., and Ressurreição, R. (2012). A Geophysical Study of the Carcavai Fault Zone, Portugal. Pure Appl. Geophys. 169, 183–200. doi:10.1007/s00024-011-0318-y
Costa, L. R. D., Hugman, R. T., Stigter, T. Y., and Monteiro, J. P. (2021). Predicting the Impact of Management and Climate Scenarios on Groundwater Nitrate Concentration Trends in Southern Portugal. Hydrogeology J., 2501–2516. doi:10.1007/s10040-021-02374-4
Costa, L. R. D., Monteiro, J. P. P. G., and Hugman, R. T. (2020). Assessing the Use of Harvested Greenhouse Runoff for Managed Aquifer Recharge to Improve Groundwater Status in South Portugal. Environ. Earth Sci. 79, 1–15. doi:10.1007/s12665-020-09003-5
Coulon, C., Pryet, A., Lemieux, J. M., Yrro, B. J. F., Bouchedda, A., and Gloaguen, E. (2021). A Framework for Parameter Estimation Using Sharp-Interface Seawater Intrusion Models. J. Hydrology 600, 126509. doi:10.1016/j.jhydrol.2021.126509
Dausman, A. M., Langevin, C., Bakker, M., and Schaars, F. (2010). A Comparison between SWI and SEAWAT – the Importance of Dispersion , Inversion and Vertical Anisotropy. São Miguel, Azores, Portugal: 21st Salt Water Intrusion Meeting, 271–274.
Dillon, P., Stuyfzand, P., Grischek, T., Lluria, M., Pyne, R. D. G., and Jain, R. C. (2019). Sixty Years of Global Progress in Managed Aquifer Recharge. Hydrogeology J. 27, 1–30. doi:10.1007/s10040-018-1841-z
Doherty, J. E. (2021b). PEST Model-independent Parameter Estimation User Manual Part II: PEST Utility Support Software, Watermark Numerical Computing. Brisbane.
Doherty, J. E. (2020). PEST_HP - PEST for Highly Parallelized Computing Environments. Brisbane: Watermark Numerical Computing.
Doherty, J., and Moore, C. (2021). in Decision Support Modelling Viewed through the Lens of Model Complexity (Adelaide: National Centre for Groundwater Research and Training, Flinders University). doi:10.25957/p25g-0f58A GMDSI Monograph. South Australia
Doherty, J. (2021a). Version 2 of the LUMPREM Groundwater Recharge Model. Brisbane: Watermark Numerical Computing.
DRAP-ALGARVE (2021). Weather Station Data for Patação-Faro. Available at: https://www.drapalgarve.gov.pt/ema/pat.htm (Accessed December 22, 2021).
Fernandes, J., Midões, C., Ferreira, A., Castanheira, A., Monteiro, F., and Pereira, A. (2020). GeoEra TACTIC project, Pilot description and assessment: Campina de Faro Aquifer System. Portugal.
Foster, L. K., White, J. T., Leaf, A. T., Houston, N. A., and Teague, A. (2021). Risk-Based Decision-Support Groundwater Modeling for the Lower San Antonio River Basin. Groundwater 59, 581–596. doi:10.1111/gwat.13107
Gallagher, M., and Doherty, J. (2020). Water Supply Security for the Township of Biggenden: A GMDSI Worked Example Report. South Australia: Flinders University. doi:10.25957/5f4c5815fc0d1
Hayley, K., Valenza, A., Whilte, E., Hutchinson, B., and Schumacher, J. (2019). Application of the Iterative Ensemble Smoother Modeling Case Study. Water 11, 1649. doi:10.3390/w11081649
Hugman, R., Doherty, J., and Standen, K. (2021). Model-Based Assessment of Coastal Aquifer Management Options. A GMDSI Worked Example Report. South Australia: Flinders University. doi:10.25957/a476-x588
Hugman, R., and Doherty, J. (2022). Complex or Simple-Does a Model Have to be One or the Other?. Frontiers in Earth Sci. 10, 1–12. doi:10.3389/feart.2022.867379
Hugman, R. (2021). Lumpyrem. Available at: https://github.com/rhugman/lumpyrem (Accessed March 15, 2021).
Hugman, R. (2016). Numerical Approaches to Simulate Groundwater Flow and Transport in Coastal Aquifers – from Regional Scale Management to Submarine Groundwater Discharge. Portugal: Universidade do Algarve.
Hugman, R., Stigter, T., Costa, L., and Monteiro, J. P. (2017). Numerical Modelling Assessment of Climate-Change Impacts and Mitigation Measures on the Querença-Silves Coastal Aquifer (Algarve, Portugal). Hydrogeology J. 25, 2105–2121. doi:10.1007/s10040-017-1594-0
Koussis, A. D., Georgopoulou, E., Kotronarou, A., Lalas, D. P., Restrepo, P., and Destouni, G. (2010). Cost-efficient Management of Coastal Aquifers via Recharge with Treated Wastewater and Desalination of Brackish Groundwater: General Framework. Hydrological Sci. J. 55, 1217–1233. doi:10.1080/02626667.2010.512467
Langevin, C. D., Hughes, J. D., Banta, E. R., Provost, A. M., and Panday, S. (2021). MODFLOW 6 Modular Hydrologic Model. doi:10.5066/F76Q1VQV
Lien, J.-M., Liu, G., and Langevin, C. D. (2015). GRIDGEN Version 1 . 0 : A Computer Program for Generating Unstructured Finite-Volume Grids. doi:10.3133/ofr20141109
Lu, C., Xin, P., Li, L., and Luo, J. (2015). Seawater Intrusion in Response to Sea-Level Rise in a Coastal Aquifer with a General-Head Inland Boundary. J. Hydrology 522, 135–140. doi:10.1016/j.jhydrol.2014.12.053
Manuppella, G., Ramalho, M., Antunes, M. T., and Pais, J. (2007). Carta Geologia de Portugal na escala de 1:50,000: Notícia Explicativa da folha 53-A Faro. Lisboa.
Schneider, C., Laizé, C. L. R., Acreman, M. C., and Flörke, M. (2013). How Will Climate Change Modify River Flow Regimes in Europe? Hydrology Earth Syst. Sci. 17, 325–339. doi:10.5194/hess-17-325-2013
SNIRH (2021). Sistema Nacional de Informação de Recursos Hidricos, Agência Portuguesa Do Ambiente. Available at: https://snirh.apambiente.pt/index.php?idMain=1&idItem=1.4&uh=M&sa=M12 - CAMPINA DE FARO (Accessed December 20, 2020).
Soares, P. M. M. M., Cardoso, R. M., Lima, D. C. A., Miranda, P. M. A. A., and Daniela, C. A. L. (2017). Future Precipitation in Portugal: High-Resolution Projections Using WRF Model and EURO-CORDEX Multi-Model Ensembles. Clim. Dyn. 49, 2503–2530. doi:10.1007/s00382-016-3455-2
Standen, K., Hugman, R., and Monteiro, J. P. (2021). “Managed Aquifer Recharge as a Solution for an Over-exploited Aquifer in South Portugal: Development of a Decision-Support Groundwater Model (Abstract),” in 47th International Association of Hydrogeologists Conference, Brussels, September 6th – 10th, 2021.
Stigter, T. Y., Carvalho Dill, A. M. M., and Ribeiro, L. (2011). Major Issues Regarding the Efficiency of Monitoring Programs for Nitrate Contaminated Groundwater. Environ. Sci. Technol. 45, 8674–8682. doi:10.1021/es201798g
Stigter, T. Y., van Ooijen, S. P. J., Post, V. E. A., Appelo, C. A. J., and Carvalho Dill, A. M. M. (1998). A Hydrogeological and Hydrochemical Explanation of the Groundwater Composition under Irrigated Land in a Mediterranean Environment, Algarve, Portugal. J. Hydrology 208, 262–279. doi:10.1016/S0022-1694(98)00168-1
Vanderzalm, J., Page, D., Dillon, P., Gonzalez, D., and Petheram, C. (2022). Assessing the Costs of Managed Aquifer Recharge Options to Support Agricultural Development. Agric. Water Manag. 263, 107437. doi:10.1016/j.agwat.2021.107437
Werner, A. D., Bakker, M., Post, V. E. A., Vandenbohede, A., Lu, C., and Ataie-Ashtiani, B. (2013). Seawater Intrusion Processes, Investigation and Management: Recent Advances and Future Challenges. Adv. Water Resour. 51, 3–26. doi:10.1016/j.advwatres.2012.03.004
Werner, A. D. (2017). On the Classification of Seawater Intrusion. J. Hydrology 551, 619–631. doi:10.1016/j.jhydrol.2016.12.012
White, J. T. (2018). A Model-independent Iterative Ensemble Smoother for Efficient History-Matching and Uncertainty Quantification in Very High Dimensions. Environ. Model. Softw. 109, 191–201. doi:10.1016/j.envsoft.2018.06.009
White, J. T., Doherty, J. E., and Hughes, J. D. (2014). Quantifying the Predictive Consequences of Model Error with Linear Subspace Analysis. Water Resour. Res. 50, 1152–1173. doi:10.1002/2013WR014767
White, J. T., Hunt, R. J., Fienen, M. N., Doherty, J. E., and Survey, U. S. G. (2020). Approaches to Highly Parameterized Inversion: PEST++ Version 5, a Software Suite for Parameter Estimation, Uncertainty Analysis, Management Optimization and Sensitivity Analysis. Tech. Methods 64. doi:10.3133/tm7c26
Keywords: uncertainty, data-assimilation, complexity, MAR (managed aquifer recharge), numerical modelling
Citation: Standen K, Hugman R and Monteiro JP (2022) Decision-Support Groundwater Modelling of Managed Aquifer Recharge in a Coastal Aquifer in South Portugal. Front. Earth Sci. 10:904271. doi: 10.3389/feart.2022.904271
Received: 25 March 2022; Accepted: 11 May 2022;
Published: 31 May 2022.
Edited by:
Michael Fienen, United States Geological Survey, United StatesReviewed by:
Ali Al-Maktoumi, Sultan Qaboos University, OmanJohn Masterson, United States Geological Survey (USGS), United States
Copyright © 2022 Standen, Hugman and Monteiro. This is an open-access article distributed under the terms of the Creative Commons Attribution License (CC BY). The use, distribution or reproduction in other forums is permitted, provided the original author(s) and the copyright owner(s) are credited and that the original publication in this journal is cited, in accordance with accepted academic practice. No use, distribution or reproduction is permitted which does not comply with these terms.
*Correspondence: Kath Standen, ay5lLnN0YW5kZW5AdWFsZy5wdA==