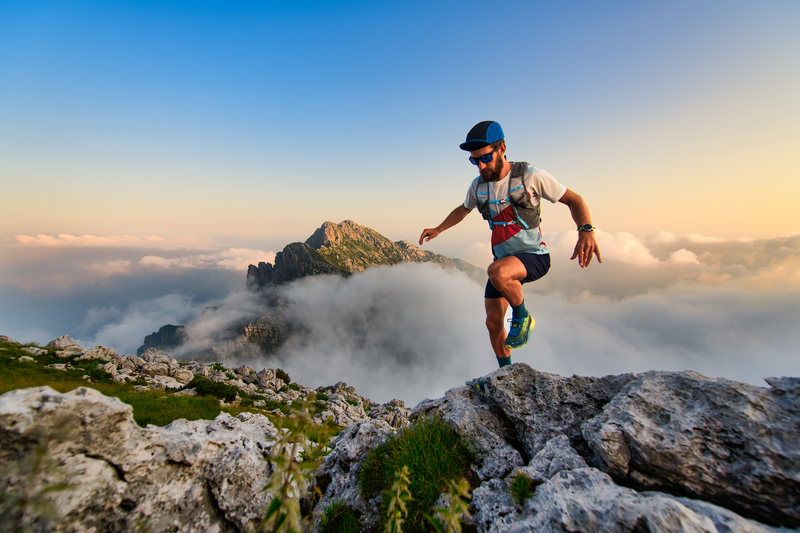
94% of researchers rate our articles as excellent or good
Learn more about the work of our research integrity team to safeguard the quality of each article we publish.
Find out more
ORIGINAL RESEARCH article
Front. Earth Sci. , 19 August 2022
Sec. Geohazards and Georisks
Volume 10 - 2022 | https://doi.org/10.3389/feart.2022.897315
This article is part of the Research Topic Volcanism in the Central Volcanic Zone of the Andes View all 10 articles
Recent advances in the modeling of volcanic phenomena have allowed scientists to better understand the stochastic behavior of volcanic systems. Eruptions can produce various types of volcanic phenomena of different sizes. The size of a given volcanic phenomenon dominates its spatial distribution and is commonly represented by volume/mass parameters in the models that reproduce their behavior. Multi-hazard assessments depend on first-order parameters to forecast hazards at a given geographic location. However, few multi-hazard assessments consider the size of the eruption (e.g., tephra fallout) to co-parameterize the size of the accompanying phenomena (e.g., mass flows) in a given eruptive scenario. Furthermore, few studies simulate multi-phenomenon eruptive scenarios with semi-continuous variations in their size, something that allows a better quantification of the aleatoric variability of the system. Here, we present a multi-hazard assessment of the San Pedro volcano, a high-threat volcano from northern Chile, that produced two large-size Plinian eruptions (VEI 5 and 6) in the last 16 ka, and ten Strombolian eruptions (VEI 2) between 1870 and 2021 CE, with the latest occurring on 2 December 1960 CE. We use intra-scenarios (i.e., subdivisions of eruptive scenarios) to explore the size variability of explosive volcanic phenomena. The size of intra-scenarios is extrapolated from the largest-size deposits of each type of phenomenon from the geologic record of the San Pedro volcano. We simulate explosive intra-scenarios for tephra fallout, concentrated PDCs, and lahars, and effusive scenarios for blocky lava flows. On the local scale, mass flows are likely (66–100%) to affect transport and energy infrastructure within a 14 km radius of the volcano. On the regional scale, large-size eruptions (VEI 5) in the rainy season are about as likely as not (33–66%) to accumulate 1 cm of tephra on energy, transport, and mining infrastructure over a 50 km radius, and these same eruptions are unlikely (10–33%) to accumulate 1 cm of tephra on the city of Calama. This work shows how multi-phenomenon intra-scenarios can be applied to better quantify the aleatoric variability of the type and size of volcanic phenomena in hazard assessments.
The primary goal of volcanic hazard assessments is to forecast the occurrence of volcanic phenomena in a given geographical location (Decker, 1986; Blong, 2000; Newhall and Hoblitt, 2002; Marzocchi and Bebbington, 2012; Martí, 2017; Poland and Anderson, 2020). Volcanic systems have complex stochastic behaviors that can lead to the generation of various types of volcanic phenomena, such as tephra fallout, volcanic ballistic projectiles (VBPs), pyroclastic density currents (PDCs), water-sediment flows (lahars), and lava flows, among other types. During an eruption, volcanic phenomena can also be generated with different sizes. The spatial distribution of each volcanic phenomenon is dominated by their size, and it is directly related to the first-order parameters in the computational models used to reproduce their behavior. The spatial distribution of volcanic phenomena is controlled by external first-order parameters such as terrain elevation and atmospheric wind data. However, their spatial distribution is also dominated by internal first-order parameters, for example, tephra fallout is controlled by the erupted mass parameter in Tephra2 (Bonadonna et al., 2005); lahars by the volume parameter in Laharz-py (Schilling, 2014) and LaharFlow (Woodhouse et al., 2016); PDCs by the initial velocity and flow duration parameters in VolcFlow (Kelfoun et al., 2009; Kelfoun, 2017), and by the collapse height and mobility parameters in the Energy Cone model (Malin and Sheridan, 1982); VBPs by the initial velocity and launch angle parameters in Ballistics (Bertin, 2017); lava flows by the effusion rate and viscosity parameters in FlowGo/Q-LavHA (Harris and Rowland, 2001; Mossoux et al., 2016), and by the mean length parameter in Decreasing Probability/Q-LavHA (Bonne et al., 2008; Mossoux et al., 2016), among other examples. These parameters are related to the aleatoric variability of the volcanic system. Aleatoric variability comes from the random behavior of the system, and it is one of the uncertainties that can be quantified in volcanic hazard assessments (Marzocchi et al., 2004; Marzocchi et al., 2021).
The volume of a given volcanic phenomenon is a parameter that appears in some of these models, directly or indirectly. For instance, volume appears directly as a parameter in the lahar models, but it appears indirectly in the tephra fallout and PDCs models (i.e., it cannot be entered directly). Nonetheless, erupted mass can be converted to erupted volume by using a constant mean density value of 1,000 kg m−3 for pumice ash (Pyle, 2015), and volume can be approximated from the initial velocity and flow duration parameters in VolcFlow (Kelfoun et al., 2009). These conversions can be proven useful for producing a co-parameterization of the volume of multiple hazards in the same eruptive scenario. In this context, knowing the volume of each phenomenon produced during the largest eruption of a given volcano is crucial to co-parameterize the size of synchronous volcanic phenomena in the same eruptive scenario.
One of the most important sources of aleatoric variability in volcanic systems is the size of future volcanic phenomena. Many model-based volcanic hazard assessments do not explore the variability of the size of volcanic phenomena in their approaches. Most hazard assessments, subjectively, select and simulate a unique set of sizes (three or more) from the volcanic explosivity index (VEI) scale, this is known as the eruptive scenario methodology. However, this methodology does not account for many of the sizes that could occur for a given phenomenon within the same eruptive scenario selected, as previous works have proposed, for the case of tephra fallout (Sandri et al., 2016). This could be a byproduct of the use of the VEI scale, something that is done with two objectives, to reduce the computational time costs (by producing fewer simulations) and to better communicate the results (Selva et al., 2018). Nevertheless, hazard assessments should strive to obtain the spatial distribution probabilities of volcanic phenomena through the simulation of several scenarios that should be continuous or semi-continuous in size, and non-dependent on the major divisions of the VEI scale (e.g., Sandri et al., 2016; Biass et al., 2017; Charbonnier et al., 2020; Clarke et al., 2020; Tadini et al., 2022). Therefore, the gaps between the size of the selected and simulated scenarios should not differ by order of magnitude (e.g., 107, 108, 109 m3), instead, the gaps should be smaller, and more scenarios should be produced.
In the last decade, a variety of computational frameworks have been published in the scientific literature that greatly improve the accessibility of methodologies and reduce computational time costs. For example, probabilistic toolboxes for analytical models of tephra fallout (Tephra2-TephraProb) (Bonadonna et al., 2005; Biass et al., 2016b), easily accessible statistical/empirical models of lava flows (Q-LavHA) (Felpeto et al., 2001; Bonne et al., 2008; Mossoux et al., 2016), and real-time deterministic numerical models for concentrated PDCs (VolcFlow) (Patra et al., 2005; Kelfoun et al., 2009) and lahars (LaharFlow) (Woodhouse et al., 2016). Even though the eruptive scenario methodology is done to reduce computational time costs, this could be no longer necessary in the future, as the computational power of personal computers and the advances in computational frameworks could be able to reduce simulation time costs, thus enabling the production of more scenarios.
The aleatoric variability within the volume of a volcanic phenomenon has been explored in various previous studies (Sandri et al., 2016; Biass et al., 2017; Charbonnier et al., 2020; Tadini et al., 2022). For example, Sandri et al. (2016) explore the intra-size variability of tephra fallout by following the discretization of the erupted mass parameters into bins with an interval of 0.1 on the magnitude (M) scale (Pyle, 2015); Biass et al. (2017) explore the intra-size variability of tephra fallout by producing thousands of simulations with different erupted mass values chosen stochastically (Monte Carlo method) from within a range of values; Tadini et al. (2022) explore the intra-size variability of tephra fallout by simulating a continuous distribution of erupted mass values; Charbonnier et al. (2020) explore the intra-size variability of PDCs by producing a discretization of the volume, by increasing by 106 m3 the volume of all simulations.
Most model-based volcanic hazard assessments focus on a single type of volcanic phenomena, for example, tephra fallout (Biass and Bonadonna, 2013; Biass et al., 2014, 2016a, 2017; Bonasia et al., 2014; Sandri et al., 2016; Selva et al., 2018; Vásquez et al., 2019; Hayes et al., 2020; Michaud-Dubuy et al., 2021; Tadini et al., 2022; Titos et al., 2022) or PDCs (Sandri et al., 2018; Tierz et al., 2018; Charbonnier et al., 2020; Clarke et al., 2020; Esposti Ongaro et al., 2020; Flynn and Ramsey, 2020; Spiller et al., 2020). Double-hazard assessments focus on tephra fallout along with other types of volcanic phenomena, such as lava flows (Marrero et al., 2019; Gjerløw et al., 2022) or PDCs (Alcorn et al., 2013; Tennant et al., 2021). Multi-hazard assessments focus on three or more types of volcanic phenomena, such as tephra fallout, PDCs, lava flows, lahars, VBPs, or others (Felpeto et al., 2007; Capra et al., 2008; Scaini et al., 2012; Becerril et al., 2014, 2017; Sandri et al., 2014; Jiménez et al., 2020; Reyes-Hardy et al., 2021; Mead et al., 2022; Warwick et al., 2022). Multi-hazard assessments can quantify a wider range of the aleatoric variability of the behavior of a given volcanic system than single- or double-hazard assessments.
Here, we present a multi-hazard assessment of the San Pedro volcano, a high-threat volcano in northern Chile (Lara et al., 2011; SERNAGEOMIN, 2020). Even though San Pedro is a remote volcano, located in the middle of the Atacama Desert (21°S), a previous hazard assessment (Bertin and Amigo, 2015) has shown that the distribution of tephra fallout from large-size eruptions (VEI 5) could reach localities and infrastructure widely distributed throughout the northeastern quadrant of the Antofagasta region. Within a 100 km radius of the San Pedro volcano, the locality with the highest population density is the city of Calama (≈80 km SW), with an estimated population larger than ≈150,000 people (INE, 2017; MINVU and IDE, 2017). Plus, there are less populated localities, such as towns, villages, and railway stations located at different distances from the volcanic crater. Furthermore, there is transport (e.g., highways, railways, and airports), electrical (e.g., electrical transmission towers and a geothermal power plant), and some of the largest copper mining infrastructure in the world (e.g., El Abra, Radomiro Tomic, Chuquicamata, and Ministro Hales mines). A probabilistic tephra fallout assessment at the San Pedro volcano is essential for the copper mining industry, to estimate the likelihood of tephra accumulation on key infrastructures for the economic development of the country, as mining is the most important source of economic growth in Chile. Moreover, an analysis of the distribution of mass flows can be useful for land-use planning of future transport and energy infrastructure that is required to be constructed near the San Pedro volcano.
We evaluate four types of volcanic phenomena, three types of explosive phenomena (i.e., tephra fallout, concentrated PDCs, and lahars), and one effusive phenomenon (i.e., blocky lava flows). In the case of the explosive scenarios, we quantify the intra-variability of the size of a given volcanic phenomenon by formulating several eruptive scenarios that have continuous/semi-continuous volumes within the sizes of the VEI scale, which we call: eruptive intra-scenarios. The volume values of the different volcanic phenomena in these intra-scenarios are co-parameterized and correlate with each other. We based the size of the largest intra-scenario on the assumption that the largest deposits of the different explosive phenomena (tephra fallout, concentrated PDCs, and lahars) were created synchronously during the largest eruption in the geological record of the San Pedro volcano. We extrapolated volumes for concentrated PDCs and lahars from the volume of tephra fallout. Thus, the methodology is based on the volume of the largest deposit from each type of volcanic phenomenon generated by the volcano, a key parameter that could be possibly found in local stratigraphic field-based studies of volcanoes, or extrapolated from analogous volcanoes (Tierz et al., 2019).
Hazard assessments require the confection of maps to visualize and explain the likelihood of volcanic hazards to the governmental stakeholders and communities exposed to volcanic threats (Doyle et al., 2014; Lindsay and Robertson, 2018; Thompson et al., 2015, 2021). Hazard cartography is crucial for increasing resilience and mitigating volcanic disaster risk in vulnerable communities (Guimarães et al., 2021; Nieto-Torres et al., 2021). Volcanic systems often require hazard assessments with complementary approaches to bring forth alternative perspectives on hazard forecasting. For example, new studies can complement previous assessments through various means: 1) complement previous hazard zones of a given type of volcanic phenomena by using another computational model to produce simulations; 2) complement previous hazard zones by simulating a type of volcanic phenomena not simulated in previous hazard assessments, or 3) implement new hazard zones by simulating a type of volcanic phenomena not taken in consideration in previous hazard assessments. Our contribution is a complement to the current volcanic hazard assessment of the San Pedro volcano from these three perspectives (Bertin and Amigo, 2015).
The expansion and improvement of volcanic hazard assessments are relevant for future risk studies at high-threat volcanoes in northern Chile (e.g., Reyes-Hardy et al., 2021). The probabilistic methods employed in this study will robust the results of future risk assessments produced at the San Pedro volcano, and we hope it will be useful for volcanic hazard assessment at volcanoes in other data-scarce regions of the world. Another goal of this work is to show how to reproduce the methodology for volcanic hazard assessment, including a detailed description of the procedures and tools used to create the hazard maps with geographic information systems (GIS).
The San Pedro volcano (21°53′S; 68°24′W; code 355070) (GVP, 2013a) is a historically active Pleistocene–Holocene compound stratovolcano from the Central Volcanic Zone of the Andean volcanic arc (CVZA) (Stern, 2004; Tilling, 2009). San Pedro is in the northeastern quadrant of the Antofagasta Region of northern Chile, 35 km W of the Chile–Bolivia international border, and 80 km NE of the city of Calama (Figure 1).
FIGURE 1. Ubication map showing locations and infrastructures at different radial distances from the San Pedro volcano. ‘World imagery’ and ‘Terrain hillshade’ base maps courtesy of ESRI and others. Volcanic data from Global Volcanism Program database, version 4.10.4 (GVP, 2013c). Additional cartography data was obtained from the OpenStreetMap database.
Several types of studies have been carried out on the San Pedro volcano, for example, geological (Francis et al., 1974; O’Callaghan and Francis, 1986; Francis and Wells, 1988; De Silva and Francis, 1991; González-Ferrán, 1995), petrographic (Francis et al., 1974; O’Callaghan and Francis, 1986; Godoy et al., 2017; González-Maurel et al., 2019a; González-Maurel et al., 2020), geochronological (O’Callaghan and Francis, 1986; Mamani et al., 2008; Godoy et al., 2014, 2017; Delunel et al., 2016; Bertin and Amigo, 2019; González-Maurel et al., 2019b), historical eruption recordings (Casertano, 1963; González-Ferrán, 1995; Petit-Breuilh, 2004; Siebert et al., 2010; Bertin and Amigo, 2015, 2019), regional (Lara et al., 2011; Amigo et al., 2012) and local volcanic hazard assessments (Bertin and Amigo, 2015), emergency crisis plans (ONEMI, 2019), seismic (Pritchard et al., 2014), surface deformation (Pritchard and Simons, 2004; Pritchard et al., 2014), morphometric (Grosse et al., 2014; Aravena et al., 2015), spectral thermal anomalies (Jay et al., 2013), volatile fluxes (Aguilera et al., 2020), and glaciological (Barcaza et al., 2017). In northern Chile, model-based double-hazard assessments have been produced regionally for a series of volcanoes (Amigo et al., 2012). Besides, model-based multi-hazard assessments have been done for single volcanoes, such as Láscar (Gardeweg and Amigo, 2015), San Pedro (Bertin and Amigo, 2015), and Guallatiri (Jorquera et al., 2019; Reyes-Hardy et al., 2021).
The historical record of the San Pedro volcano comprises 11 eruptions between the years 1870–2021 CE (Siebert et al., 2010; GVP, 2013a; Bertin and Amigo, 2019), and the last eruption occurred more than 60 years ago, on 2 December 1960 CE (Casertano, 1963). However, the description of these events is incomplete, and only six eruptions have been confirmed and assigned the predetermined size of VEI 2 (Newhall and Self, 1982; GVP, 2013a). No geological record of historical eruptions has been found to date, and most eruptions are interpreted to be phreatic explosions by previous authors (Bertin and Amigo, 2015, 2019) as no significant details or visual descriptions of these events have been given in the literature. Despite this, the San Pedro volcano has the second-most abundant historical record of eruptions in northern Chile, just after the Lascar volcano—the most active volcano of the CVZA—which has a total of 29 confirmed eruptions in its record between the years 1848–2021 CE (GVP, 2013b).
The geologic record of the San Pedro volcano dates to ca. 310 ka (Bertin and Amigo, 2019). Recent geological studies have found that it has produced two Plinian eruptions in the last 16 ka (Sellés and Gardeweg, 2017; Bertin and Amigo, 2019). Estimations of the volume of tephra fallout deposits, yield eruptions of VEI5/M5.7 (ca. 15.45 ka BP) and VEI6/M6.0 (ca. 11.55 ka BP) (Bertin and Amigo, 2015, 2019). The M6.0 eruption is the largest in the geological record of the San Pedro volcano (Bertin and Amigo, 2019), and one of the largest tephra fallout deposits in the last 12 ka in northern Chile. Many authors interpret that this eruption generated PDCs as well, which traveled up to 14 km W and produced the ‘El Encanto’ ignimbrite (Francis et al., 1974; O’Callaghan and Francis, 1986; Bertin and Amigo, 2019). Two studies have provided estimates for the volume of the tephra fallout deposit by applying different methodologies. Francis et al. (1974) estimated a volume of 2 km3, while Bertin and Amigo (2019) estimated, through different methodologies, a volume of 10 km3 (Bonadonna and Houghton, 2005) and 26 km3 (Sulpizio, 2005), although, the former value was selected to classify the eruption. This volume is comparable to the 1600 eruption of the Huaynaputina volcano in Peru, which has been classified as a VEI 6 eruption with an erupted volume of 11 km3: the largest historical eruption in the CVZA (Tilling, 2009). In addition, the San Pedro volcano has produced at least four domes in the last 11 ka (Bertin and Amigo, 2019), from which two of these collapsed and generated PDCs toward the northern flank of the volcano. The recurrence and magnitude of these events positions San Pedro as one of the most geologically and historically active volcanoes of northern Chile and the CVZA (Lara et al., 2011).
According to hazard-related studies, volcanic phenomena such as tephra fallout, PDCs, lava flows, and VBPs are likely to occur in future eruptions of the San Pedro volcano (Lara et al., 2011; Amigo et al., 2012; Bertin and Amigo, 2015, 2019), whereas the perceived potential for the volcano to produce lahars is unlikely (Lara et al., 2011). Primarily, due to the absence of an ice cap (Bertin and Amigo, 2015) and the seasonal variation of the area covered by snow at the volcanic summit.
A priori, tephra fallout is one of the most important phenomena that could be produced by the San Pedro volcano, due to the large distances it can travel. Even though San Pedro is a remote volcano, and the population density of the Atacama Desert is small, previous volcanic hazard assessments (Bertin and Amigo, 2015) have shown that the distribution of tephra fallout from a large-size eruption (VEI 5–6) could reach localities and infrastructure widely distributed throughout the northeastern quadrant of the Antofagasta region.
Recent threat rankings placed San Pedro in 41st place out of a total of 92 geologically active volcanoes from all the country, including the Central, Southern, and Austral volcanic zones of the Andean volcanic arc. Nonetheless, it is in the fifth position out of 32 geologically active volcanoes in northern Chile (Lara et al., 2011; SERNAGEOMIN, 2020). Furthermore, a recent hazard ranking has placed San Pedro in fifth place out of 8 historically active volcanoes in northern Chile (Guimarães et al., 2021). Consequently, even though it is not considered a priority on a national scale, it is important on a local and regional scale (Antofagasta Region).
The volcano is currently being monitored by the Southern Andes Volcanological Observatory (in Spanish: Observatorio Volcanológico de Los Andes del Sur, OVDAS)1 and monitoring reports are produced monthly (e.g., OVDAS, 2022; Amigo, 2021). Regarding monitoring studies, no deformation was detected during InSAR surveys (Pritchard and Simons, 2004), but there are records of seismic disturbances (Pritchard et al., 2014; OVDAS, 2022), small thermal anomaly hotspots (Jay et al., 2013), and fumarolic degassing (Aguilera et al., 2020). Moreover, a single gas plume has been observed by various authors in the modern western cone throughout the last 50 years (Francis et al., 1974; O’Callaghan and Francis, 1986; Bertin and Amigo, 2019; Aguilera et al., 2020).
Within a 20 km radius, less than ≈20 people are living at the maintenance stations of the Chile–Bolivia freight railway (San Pedro and Ascotán stations) (Figure 2) (INE, 2017; MINVU and IDE, 2017). Regarding electric infrastructure, there is a chain of high-voltage electrical transmission towers connecting the Cerro Pabellón geothermal power plant to the power grid of the Antofagasta Region (Figures 1, 2). Additionally, there are critical transport routes, such as the international highway (CH–21) and the trails of the Chile–Bolivia freight railway (Figures 1, 2).
FIGURE 2. Simplified geological map of the San Pedro volcano. Map based on the mapping and geochronology produced by Bertin and Amigo (2019). This map shows in color the deposits of blocky lava flows, PDCs, tephra fallout, and lahars (ca. 140 ka–present) and in gray the volcanic avalanche deposit (ca. 140 ka) (1:110,000 scale). The ‘Terrain: multi-directional hillshade’ and the ‘Terrain: hillshade’ base maps are courtesy of ESRI and others, taken from the Living Atlas database (https://livingatlas.arcgis.com). Additional cartography data was obtained from the OpenStreetMap database with the QuickOSM plugin for QGIS (https://github.com/3liz/QuickOSM).
Within a 20–50 km radius, there are more than ≈150 people in the towns of Conchi (≈28 km SW), Ayquina (≈44 km S), Lasana (≈49 km W), and Toconce (≈49 km SE) (Figure 1) (MINVU and IDE, 2017). In addition, there are crucial mining and energy infrastructures: the Cerro Pabellón geothermal power plant (≈26 km E) and the El Abra open-pit copper mine (≈44 km W) (Figure 1).
Within a 50–100 km radius, more than ≈155,000 people are living mostly in the city of Calama (≈80 km SW), and in small localities such as Chiu Chiu (≈56 km SW), Caspana (≈55 km S), Ollagüe (≈75 km NE), and Río Grande (≈89 km W) (Figure 1) (INE, 2017; MINVU and IDE, 2017). Likewise, there are at least three copper mines within this distance from the volcano, including Radomiro Tomic (≈63 km SW), Chuquicamata (≈68 km SW), and Ministro Hales (≈76 km SW) (Figure 1). Further, mining activity generates a weekly high influx of workers traveling through the El Loa international airport (≈81 km SW), promoting a floating population in the city of Calama.
The geological history of the San Pedro volcano is divided into Stage I (ca. 310–140 ka) and Stage II (ca. 140 ka to the present) (Francis et al., 1974; O’Callaghan and Francis, 1986; Bertin and Amigo, 2019). Its whole volcanic edifice is composed of two superimposed coalescent cones (Francis et al., 1974). The ancestral eastern cone (≈6,149 m a.s.l.) was built during San Pedro Stage I, while the modern western cone (≈5,971 m a.s.l.) was built during San Pedro Stage II (Figure 2) (Bertin and Amigo, 2019). These evolution stages are separated by the sector collapse of the ancestral eastern cone that occurred during the middle Pleistocene (ca. 140 ka) (O’Callaghan and Francis, 1986). This collapse produced a debris avalanche with an estimated volume of 6 km3 that deposited volcanic materials up to 15 km NW of the volcano (Figure 2) (O’Callaghan and Francis, 1986; Bertin and Amigo, 2019). The products of the volcano cover an area between 124 and 150 km2, while the whole edifice has an estimated volume between 40 and 56 km3 (De Silva and Francis, 1991; Grosse et al., 2014; Aravena et al., 2015; Bertin and Amigo, 2019). In the following paragraphs, we only review Stage II of San Pedro, as it spans the same time interval evaluated in this assessment (ca. 140 ka to present).
The construction of the modern western cone begins during the middle to late Pleistocene when effusive eruptions occurred in the collapsed escarpment of the ancestral eastern cone. These eruptions produced at least twelve blocky lava flows with runout lengths between 7.2 and 12.4 km toward the NW and W, and between 20 and 100 m of front thicknesses (Figure 2) (Bertin and Amigo, 2019). These blocky lava flows have an andesitic to dacitic composition and an age constrained at ca. 140–60 ka by several authors through Ar40/Ar36 geochronology (O’Callaghan and Francis, 1986; Mamani et al., 2008; Godoy et al., 2014, 2017; Delunel et al., 2016; Bertin and Amigo, 2019; González-Maurel et al., 2019b).
During the late Pleistocene (ca. >55–37 ka), at least four lahar discharges occurred toward the W and S. Two deposits stand out due to their length, reaching 20 and 25 km toward the W, and even impacting the Loa River valley (Figure 2) (Bertin and Amigo, 2019). At ca. 60–15 ka, a transition to more differentiated effusive products occurred, leading to five lava domes and six non-flowing domes (Bertin and Amigo, 2019). The lava domes have runout lengths between 1.5 and 6.5 km toward the NW and SW (Bertin and Amigo, 2019).
From the late Pleistocene to the early Holocene (ca. >15–11 ka), at least seven explosive eruptions formed tephra fallout deposits that can be found up to 25 km NE and SE of the volcano (Figure 2) (Bertin and Amigo, 2019). Some of these deposits have been identified near the Ascotán station (≈27 km NE) and near the Chile–Bolivia border (≈38 km SE) (Figure 1) (Bertin and Amigo, 2019). Previous works attribute the largest deposit to a VEI 6 Plinian eruption (M6.0) constrained at ca. 11.55 ka BP (through radiocarbon dating C14), whereas the second-largest deposit is attributed to a VEI 5 Plinian eruption (M5.7) constrained at ca. 15.45 ka BP (C14) (Bertin and Amigo, 2019). At ca. 15 ka, San Pedro produced PDCs that traveled up to 14 km toward the SE, and to the SW and N. This deposit has a thickness between 0.1 and 0.3 m and a scoriaceous composition (Bertin and Amigo, 2019). Previous authors propose that this deposit is synchronous with the tephra fallout deposits of the VEI 5 Plinian eruption (M5.7), whereas the other five deposits have smaller but unknown magnitudes (Bertin and Amigo, 2019).
During the early Holocene (ca. 11 ka), San Pedro produced large PDCs with a runout length of up to 14 km toward the W, that formed a pumice-rich deposit called ‘El Encanto’ ignimbrite (Figure 2) (Sellés and Gardeweg, 2017; Bertin and Amigo, 2019). Authors suggest that this deposit formed synchronously with the tephra fallout deposits of the Plinian VEI 6 eruption (M6.0) (Bertin and Amigo, 2019). The volume of the Encanto ignimbrite has been estimated at 1.5 km3 (O’Callaghan and Francis, 1986) and 0.75 km3 (Bertin and Amigo, 2019), being the largest PDCs deposit in the geological record of the volcano. At ca. <11 ka, three non-flowing dacitic domes and one lava dome were produced (Bertin and Amigo, 2019). The lava domes have runout lengths between 1.0 and 2.5 km toward the N, thicknesses between 130 and 150 m, and well-developed ogive structures (Bertin and Amigo, 2019). At least two of these domes collapsed partially during their emplacement, generating PDCs with runout lengths between 9 and 10 km toward the N, between 1 and 2 m thick, and a block-and-ash composition (Francis et al., 1974; Bertin and Amigo, 2019).
We applied a methodology comprising four steps: 1) the selection of eruptive scenarios and intra-scenarios, 2) the selection of the computational models, 3) the parameterization of the models and simulations of the explosive intra-scenarios and effusive scenarios, and 4) the preparation and visualization of likelihood and hazard zone maps.
We separated the eruptive scenarios into explosive intra-scenarios and effusive scenarios. Explosive intra-scenarios account for tephra fallout, concentrated PDCs, and syn-eruptive lahars generated by snowcap melting, whereas effusive scenarios only account for blocky lava flows.
This methodology is based on the estimated volume of the largest deposits of each volcanic phenomenon produced by the volcano. This methodology assumes that the largest deposits of each phenomenon were created and deposited during the same eruption, i.e., the largest eruption in the geologic record of the volcano. Thus, we assume that the largest tephra fallout deposit is synchronous with the largest PDC and lahar deposits of the volcano. In the case of the San Pedro volcano, this is true for the largest tephra fallout and PDCs deposits that were created during the M6.0 Plinian eruption (ca. 11.55 ka BP) (Francis et al., 1974; O'Callaghan and Francis 1986; Bertin and Amigo, 2019). Even though the largest lahar deposit is thought to have an older age (ca. >55–37 ka), we assume that a M6.0 eruption is able to produce this large lahar deposit. The simulations produced in this work are based on the complete time interval of San Pedro Stage II (ca. 140 ka to present).
For better comprehension and communication of the results, we selected three explosive scenarios representing small-size (VEI 3), medium-size (VEI 4), and large-size eruptions (VEI 5). However, each explosive scenario is composed of various intra-scenarios (see Supplementary Material):
1) The small-size scenario (VEI 3) goes from magnitudes 3.0 to 4.0, and it is composed of 5 tephra fallout, 10 concentrated PDCs, and 3 lahar intra-scenarios.
2) The medium-size scenario (VEI 4) goes from magnitudes 4.0 to 5.0, and it is composed of 5 tephra fallout, 10 concentrated PDCs, and 5 lahar intra-scenarios.
3) The large-size scenario (VEI 5) goes from magnitudes 5.0 to 6.0, and it is composed of 5 tephra fallout and 10 concentrated PDCs intra-scenarios; it does not contain lahar intra-scenarios.
Most eruptive scenario methodologies select and simulate a low number of scenarios, as they use three to four values of the VEI scale (Newhall and Self, 1982). However, we produce a higher number of scenarios by selecting and simulating scenarios located within the values of the VEI scale, by using the magnitude scale (Pyle, 2015), as done by previous authors (Sandri et al., 2016). We divided explosive scenarios into intra-scenarios to quantify the intra-variability of the mass/volume of each phenomenon. The volume/mass parameters used in the simulations of these explosive intra-scenarios are presented in Table 1.
The following computational models were used to simulate the volcanic phenomena:
1) The Tephra2 model (v. 1.2)2 within the TephraProb toolbox (v. 1.7)3 for tephra fallout (Bonadonna et al., 2005; Biass et al. 2016b).
2) The VolcFlow single-fluid model (v. 3.5.0)4 for concentrated PDCs (Kelfoun et al., 2009).
3) The LaharFlow5 model for syn-eruptive or ‘hot’ lahars generated by snow cap melting (Woodhouse et al., 2016; Tierz et al., 2017; Espín Bedón et al., 2019).
4) The Decreasing Probability model within the Q-LavHA framework (v. 3.0)6 for blocky lava flows (Felpeto et al., 2001; Bonne et al., 2008; Mossoux et al., 2016).
Tephra2 is an analytical model (Folch, 2012) of tephra dispersal and accumulation (Bonadonna et al., 2005), and TephraProb is a framework of functions for MATLAB that uses the Tephra2 model (Biass et al., 2016b). TephraProb can produce complex probabilistic eruptive scenario simulations efficiently for the user, such as in the ‘eruptive range scenario’ setting (Biass et al., 2016b). In this setting, a Monte Carlo sampling approach is applied to obtain random values for the first-order parameters from within a range of input values given by the user. Then, TephraProb produces simulations using the sampled values of the first-order parameters of the Tephra2 model (Biass et al., 2016b). These first-order parameters are erupted mass (kg), column height (m a.s.l.), eruption duration (h), and total grain-size distribution (TGSD) (φ-scale) (Biass et al., 2017). The Tephra2 model has been selected due to its extensive use and validation in the literature. It has been applied to many volcanoes, including Hekla in Iceland (Biass et al., 2014), La Fossa in Italy (Biass et al., 2016b), Puyehue–Cordón Caulle in southern Chile (Biass et al., 2016b), and Sakurajima in Japan (Biass et al., 2017), among others.
PDCs have two transport regimes (Lube et al., 2020): concentrated PDCs and diluted PDCs. Concentrated PDCs are considered to follow the topography and are analogous to pyroclastic flows, whereas diluted PDCs do not necessarily follow the topography and they are analogous to pyroclastic surges (Kelfoun, 2017). VolcFlow single-fluid is a deterministic model for simulating concentrated PDCs over a Digital Elevation Model (DEM) (Kelfoun et al., 2009; Kelfoun, 2017). VolcFlow considers the friction within the flow and between the flow and the topography of the volcano (Ogburn and Calder, 2017), which is something that other models have included, such as Titan2D (Patra et al., 2005). Nonetheless, VolcFlow single fluid uses an empirically defined parameter called ‘constant retarding stress’ (Pa), which is interpreted to be better suited than a frictional behavior parameter for modeling concentrated PDCs (Charbonnier and Gertisser, 2012). Additionally, Ogbun and Calder (2017) found that VolcFlow is more suitable to model concentrated PDCs of larger size than Titan2D. We have selected VolcFlow because it is a real-time model from which its final volume is constrained by the initial velocity and the flow duration parameters. Furthermore, other parameters within the model had been clearly described by several studies, for example, VolcFlow has been used to reproduce PDCs at Tungurahua in Ecuador (Kelfoun et al., 2009), Merapi in Indonesia (Charbonnier and Gertisser, 2012; Charbonnier et al., 2013; Kelfoun et al., 2017), Soufrière Hills in Montserrat (Gueugneau et al., 2019), Mount Pélee in Martinique (Gueugneau et al., 2020), and El Misti in Peru (Charbonnier et al., 2020).
LaharFlow is a deterministic model that can produce real-time simulations of the distribution of lahars over a DEM (Woodhouse et al., 2016). It includes an empirical model for erosion and depositation and a phenomenological model for the variation of the basal stress as a function of the flow composition (Tierz et al., 2017). The parameters of LaharFlow were calibrated by using data from the 1985 Nevado del Ruiz lahar (Pierson et al., 1990). Even though LaharFlow is a relatively recent model, it has already been tested to model lahars in the literature (Tierz et al., 2017; Espín Bedón et al., 2019). The first-order parameters in LaharFlow are the DEM resolution and the volume. LaharFlow occupies the Shuttle Radar Topography Mission (SRTM) 30-m global DEM (Farr et al., 2007), which can be extrapolated to create lower-resolution DEMs, with the intent to decrease computational time costs. Other important parameters of the model are the erosion rate, the erosion depth, and the granular friction, although no published works have produced sensitivity analyses to identify the variability of results from the use of different values in these parameters (Tierz et al., 2017; Espín Bedón et al., 2019). We have selected LaharFlow because it is a real-time model that has an initial volume parameter, which can be used to co-parameterize the volume of different phenomena in the same intra-scenario.
Q-LavHA is a framework of four different models that can simulate the distribution of ‘a’ā lava flows over a DEM (Mossoux et al., 2016). One of the reasons for its selection is the accessibility of Q-LavHA, as it is a free QGIS plug-in written in Python, that can integrate smoothly with the GIS tools used in this methodology. Q-LavHA simulates the spatial propagation of an ‘a’ā lava flow and the terminal length of a channelized flow over a DEM (Mossoux et al., 2016), based on the probabilistic steepest slope method proposed by Felpeto et al. (2001). Q-LavHA improves on this method, by adding a second corrective factor (Hp) for calculating the path of lava flows. Q-LavHA hosts various models within its framework: the Manhattan Length, Euclidean Length, the Decreasing Probability, and the Thermo-rheological (FlowGo) models. We used the Decreasing Probability model that was devised and tested by Bonne et al. (2008) at Mount Cameroon in Cameroon. Other works have used this model by simulating ‘a’ā flows in several volcanic vents of the Gran Canaria Island in Spain (Rodriguez-Gonzalez et al., 2021).
The explosive scenarios include tephra fallout, concentrated PDCs, and lahars. In this section, we describe how we obtained the volume/mass values of each phenomenon in the largest explosive intra-scenarios, i.e., the parameter values linking the three models used.
For the tephra fallout assessment, we applied the ‘eruption range scenario’ setting (TephraProb). This requires a range of values as the input for the erupted mass parameter for each intra-scenario (i.e., minimum and maximum values).
To obtain the maximum erupted mass value of the largest tephra fallout intra-scenario, we considered the volume of the tephra fallout deposit from the Plinian VEI 6 eruption (M6.0) (Bertin and Amigo, 2019). Its erupted volume (VTF, m3) was converted into erupted mass (m, kg), by assuming a constant mean density (ρ, kg m−3) value of 1,000 kg m−3 for the whole deposit by using Eq. (1) (following Bonadonna et al., 2005; Pyle, 2015; Biass et al., 2016b; 2017). This resulted in a maximum erupted mass value of 1.0 × 1013 kg (Table 1).
To obtain the minimum erupted mass value of the largest tephra fallout intra-scenario, we converted the maximum erupted mass value (1.0 × 1013 kg) to an integer of the magnitude scale (M, adimensional) following Eq. (2) (Pyle, 2015). This results in a maximum magnitude value of 6.0. Then, this value (6.0) was reduced by 0.1 to obtain a minimum magnitude value of 5.9. We converted this value to erupted mass by using Eq. (3) (Pyle, 2015). This resulted in a minimum erupted mass value of 7.9 × 1012 kg (Table 1).
This equation allowed us to select continuous values of magnitude/erupted mass for the other tephra fallout intra-scenarios (Supplementary Material). We selected a continuous range of erupted mass values for 30 intra-scenarios, ranging from M3.0–3.1 to M5.9–6.0, and we computed minimum and maximum erupted mass values for each one of these (Supplementary Material), following Sandri et al. (2016). Due to the high computational time cost of this methodology (Selva et al., 2018), we only produced probabilistic simulations for half the intra-scenarios (15). We selected the intra-scenarios that had an uneven value for the minimum magnitude (Table 1).
Regarding other tephra fallout parameters, the minimum and maximum column height values were obtained from Mastin et al. (2009) by using Eq. (4), with column height (HTF, m a.s.l.) and erupted volume (VTF, in this case, in km3). We only simulated the climactic phase of the eruptions (following Sandri et al., 2016; Biass et al., 2017), with a chosen eruption duration of 1–6 h for all scenarios. All tephra fallout scenarios assume that a vent will open at the summit crater of the modern western cone San Pedro, as this location has a vent currently emitting a gas plume (Aguilera et al., 2020).
The wind data were obtained from the European Center for Medium-Range Weather Forecasts (ECMWF) Re-Analysis 5 (ERA5) dataset. The ERA5 dataset has atmospheric wind direction (°) and velocity (m s−1) data from the year 1979 to the present, it has a global spatial resolution of 32 km and a temporal resolution of 6 h. Additionally, there are 37 vertical vectorial wind fields (atmospheric pressures) between 4.9 and 30.1 km (a.s.l.). We selected 11 years of wind data, between 2008 and 2018. At 4 wind profiles per day, this time interval has 16,072 wind profiles. A visual representation of the mean values of this wind data set can be seen in Figure 3. The advised time interval for wind data is 10 years, used in most tephra fallout assessments (e.g., Scollo et al., 2013; Biass et al., 2014, 2016b, 2017; Sandri et al., 2016).
FIGURE 3. San Pedro ERA5 wind data set. The figure shows wind field data obtained from the ERA5 dataset and plotted with the TephraProb toolbox.
TephraProb can account for seasonality in the wind data by making a separate stochastic selection of wind conditions for rainy and dry seasons. Wind seasonality was evaluated in all tephra fallout scenarios. The wind data set was separated into two seasons: 1) the rainy season and 2) the dry season. The rainy season spans from January to March, whereas the dry season spans from April to December. At 10–15 km a.s.l., the E-ward winds of the dry season are considerably faster (<50 m s−1) than in the rainy season (<30 m s−1) (Figure 3). Besides, the rainy season has relatively low-velocity (<20 m s−1) winds with W-ward, S-ward, and N-ward components, whereas these are negligible in the dry season (Figure 3). At 20 km a.s.l., the rainy season develops relatively moderate (<30 m s−1) W-ward winds, whereas the dry season develops both E-ward and W-ward relatively low-velocity winds (<20 m s−1). Wind seasonality in northern Chile has been attributed to the southward movement of the South American Summer Monsoon (SASM), which originates in the Amazonian rainforest (Garreaud, 2009; Cavalcanti, 2012; Carvalho, 2020).
For the concentrated PDCs assessment, we used the estimated volume of the largest PDC deposit of the San Pedro volcano: the Encanto ignimbrite. The area covered by the Encanto ignimbrite was estimated to be a minimum of 4.95 × 107 m2, based on the area of mapped deposits (Figure 2) (Bertin and Amigo, 2019). Bertin and Amigo (2019) estimated the volume of this deposit using a mean thickness of 15 m, resulting in a mean volume of 7.43 × 108 m3. If the middle value of the volume of the largest tephra fallout intra-scenario is multiplied by 8.3%, we obtained a value similar (7.45 × 108 m3) to the volume of the Encanto ignimbrite (7.43 × 108 m3). We used the 8.3% value to estimate the volumes of the other 30 concentrated PDCs intra-scenarios (Table 1 and Supplementary Material).
We used an 11.91-m resolution DEM produced by the ‘TerraSAR–X add-on for digital elevation measurement’ (TanDEM–X) and developed by the German Aerospace Center (Deutsches Zentrum für Luft- und Raumfahrt, DLR). The flow duration and the initial velocity were changed accordingly to produce the expected final volumes for all concentrated PDCs intra-scenarios. The duration of the flow was set between 65 and 2,500 s, while the initial velocity was set between 0.05 and 0.35 m s−1 depending on the simulation volume (Kelfoun et al., 2009) (Supplementary Material). A constant retarding stress was selected at 5,000 Pa for all simulations (Kelfoun et al., 2009), and all other parameters in the model remained at constant values (Supplementary Material). Finally, we simulated all 30 intra-scenarios.
For the lahar assessment, the area covered by the largest lahar deposit of the San Pedro volcano was estimated at 2.12 × 107 m2, according to the area of mapped deposits produced by previous authors (Bertin and Amigo, 2019) (Figure 2). The mean thickness of this deposit is estimated at 1.5 m (Bertin and Amigo, 2019), resulting in an estimated lahar deposit volume of 3.18 × 107 m3. We assume that this deposit was produced during the largest M6.0 Plinian eruption at the San Pedro volcano, along with the largest tephra fallout and PDCs deposits.
To estimate the volume of the largest lahar intra-scenario, we assume a volume of solids of 40% and a volume of water of 60% (Thouret et al., 2020), and we consider tephra as its only solid component. To estimate the volume of solids, we used the same principle as in the concentrated PDCs, although we multiplied the middle value of the volume of the largest tephra fallout intra-scenario by 0.14% (see Supplementary Material). This results in a volume of solids of 1.25 × 106 m3, which corresponds to 40% of the final volume of the largest lahar intra-scenario. The other 60% of the final volume is estimated to be incorporated by melted water from the snow cap. This results in a water volume of 1.88 × 106 m3 and a final volume of 3.14 × 107 m3, analogous to the calculated volume of the largest lahar deposit described before (3.18 × 107 m3). Finally, we used the 0.14% value to estimate the volumes of the other lahar intra-scenarios.
Landsat 8 images from 2018 to 2020 (n = 50) show that the extension of the present snow cap varies throughout the year, as its area changes depending on the season. Meanwhile, the literature suggests that the San Pedro volcano had an ice cap during the late Pleistocene, during the timespan when the largest lahar deposit is thought to have been formed (ca. >55–37 ka). This interpretation is supported by moraine deposits at ca. 3,000 m a.s.l. at the southern flank of the volcano (Francis et al., 1974), and rock glaciers near the summit of the volcano (Payne, 1998; Barcaza et al., 2017). In addition, previous assessments have mentioned that it is unlikely for syn-eruptive lahars to be generated in this decade, due to the lack of an ice cap on the volcano (Bertin and Amigo, 2015).
To obtain the estimated mean radius of the snow cap at the San Pedro volcano, we measured the mean radius of the snow cap by using Landsat 8 images (n = 50), resulting in a mean snowcap radius of 775 ± 381 m. Then, we estimated the mean volume of this snow cap, with an assumed maximum snow thickness of 0.40 m, which resulted in a volume of 7.54 × 105 m3. This is the estimated volume of water that can be produced given the average current snow conditions at the summit of the San Pedro volcano. This water volume agrees with the volume of water (60%) obtained for the lahar intra-scenario number 8 (Table 1). Thus, we used a 775-m radius for the source cap in this scenario, and we calculated the radius (r) of the source caps in the other intra-scenarios by using an assumed maximum snow thickness (t) value of 0.4 m (see Supplementary Material) and the inverse of the cylinder volume (Vw) formula, shown in Eq. (5). We think that this is the maximum thickness value for the snow cap of the volcano, and we use it to not underestimate the amount of snow that could be produced at the volcano during anomalous snowing days. Nevertheless, due to the climatic conditions, a lower snow thickness could be likely at the summit of the San Pedro volcano.
It is important to note that the occurrence of the largest intra-scenario would be very unlikely because of the amount of ice/snow melted water needed to produce it. Because of this, we did not simulate the intra-scenarios of large-size (intra-scenarios 11–15). Instead, we simulated the medium and small-size scenarios (Table 1). Therefore, the medium-size scenario with the largest size has a lahar volume of 3.14 × 106 m3 (i.e., intra-scenario 10), one order of magnitude lower than the volume of the largest lahar deposit (3.14 × 107 m3). We simulated a total of 8 scenarios in 7 different cap source locations on top of the western and eastern cones of the volcano. We produced a hexagonal grid of 700 m in diameter, with their respective centroids, in the same way as for blocky lava flows (see Section 3.4 and Supplementary Material). We set the DEM resolution of the LaharFlow model to 40 m for all simulations with the intent of reducing computational time costs. All other parameters in the model remained at their predetermined values (Supplementary Material).
The effusive scenarios include only blocky lava flows. We used the 11.91-m resolution TanDEM–X DEM in all simulations. For each effusive scenario, a different vent was used at the summit crater of the modern western cone. To obtain the distribution of these vents, we produced a hexagonal tessellation grid on the summit crater of the modern western cone of the San Pedro volcano by using the GDAL function (create grid). Then, we produced centroids for each hexagon with the GDAL function (centroids) (Supplementary Material). We tested different diameters for the hexagonal polygons of the tessellation, but we decided to use a diameter of 200 m because this resulted in 32 source vent locations, a fair number of scenarios, and low computational time costs. All scenarios were simulated with the ‘point’ setting in the vent type parameter. The coordinates of the vent locations can be seen in Supplementary Material.
The simulations were made with the Decreasing Probability model and using the Euclidean length of the lava flow deposits. We measured the 12 blocky lava flow deposits identified by Bertin and Amigo (2019). We obtained a mean Euclidean length of 7,903 with a standard deviation of 2,376 (7,903 ± 2,376 m), with a minimum of 4,611 m (11th lava flow deposit, Figure 2), and a maximum of 12,103 m (12th lava flow deposit, Figure 2). All measurements can be seen in Table 2. The values used for the Hc and Hp parameters were 12 and 20 m for all simulations, respectively. Mossoux et al. (2016) estimated that these correction values can be associated with the minimum and maximum thickness of a lava flow. Bertin and Amigo (2019) describe a front thickness of 20 m for the eighth lava flow deposit (Figure 2; Table 2).
TABLE 2. Physical and geochemical data of the 12 lava flow deposits of the San Pedro volcano. aFrancis et al. (1974a); bBertin and Amigo (2019); cMamani et al. (2008); dGodoy et al. (2014); eGodoy et al. (2017); fO’Callaghan and Francis (1986); gDelunel et al. (2016); hGonzález-Maurel et al., 2019b. Front thickness measurements were taken from Bertin and Amigo (2019). Manhattan and Euclidean lengths were measured with QGIS. The Abbreviations: wt.% means weight percentage and SD means standard deviation.
Each cell of the probability rasters has values that range from 0 to 1, which are the conditional probabilities of a given volcanic phenomenon reaching a particular location (or the likelihood of phenomenon distribution). The probabilistic model-toolbox Tephra2-TephraProb outputs probabilistic rasters, therefore the mean of these rasters (
The probability rasters of each tephra fallout scenario are obtained by exporting the ESRI ASCII (.asc) probability rasters from MATLAB, using the TephraProb toolbox and importing them to QGIS. We selected the probability rasters with a tephra accumulation threshold of 10 kg m−2. Then, these rasters are interpolated with the GRASS function (r.resamp.interp) from a low resolution (6.0, 4.0, and 2.5 km) to a higher resolution (2.0 km). For instance, the small-size tephra fallout scenario has five intra-scenarios. To obtain the probability raster of this scenario, we add the probability rasters of its five intra-scenarios and divide them by the total number of small-size intra-scenarios (n = 5) by using Eq. (6) with the GDAL raster calculator. The probability rasters of the medium and large-size scenarios are obtained following the same procedure.
The probability raster of concentrated PDCs is obtained by exporting ESRI ASCII (.asc) rasters from MATLAB by using the ArcGridWrite function7. Then, these rasters are loaded in ArcGIS ArcMap and corrected by flipping them upside down along the horizontal axis by using the ArcMap function (flip raster), because the ArcGridWrite function wrongfully flips the horizontal axis of the ASCII raster matrices, then, these raster matrices are imported to QGIS. All thickness values above zero are converted to one with the GDAL raster calculator (code: ‘raster’ > 0 = 1). The mean probabilities of all 30 simulations of PDCs are obtained by using Eq. (6) with the GDAL raster calculator (n = 30). Then, the values are normalized with the SAGA function (raster normalization), so that all cells had values ranging from zero to one. Finally, we smoothed the values of the rasters with the GRASS function (r.neighbors).
The probability raster of lahars is obtained by downloading the ‘maximum height’ vector data files (.kml) from LaharFlow and importing them to QGIS. These files have an error in the thickness column as it is populated with text values (e.g., ‘h = 0.234’) instead of numbers. To correct this, a new column labeled ‘thickness’ with only the thickness value must be created. To eliminate the ‘h_max =' portion we created a new column (code: replace(Name, ‘h_max =', ' ')). Then, these vector files are converted to raster format by using the GDAL function (rasterizer), with the ‘thickness’ column as the selected field value that will populate the cells of the raster. Then, Eq. (6) is applied (although, with n = 56). The same normalization and smoothing procedures described to the VolcFlow probability rasters are applied to the LaharFlow rasters.
The probability raster of blocky lava flows is obtained by filling with zeros the no-data cell values of the rasters by using the GRASS function (r.null). Then, all probability values above zero are converted to one with the GDAL raster calculator. Then, Eq. (6) is applied (although, with n = 32). Likewise, the same normalization and smoothing procedures used for the VolcFlow and LaharFlow probability rasters are applied to the Decreasing Probability/Q-LavHA rasters.
To communicate our results, we use the five-stage likelihood classification of the IPCC (Intergovernmental Panel on Climate Change) (Mastrandrea et al., 2010) to distinguish between hazard zones, as done in other works (Tierz et al., 2017). We assigned a five-color palette from ColorBrewer8 to each hazard zone (Brewer, 2003), these zones are:
1) Likely (66–100%) or high hazard zone (red).
2) About as likely as not (33–66%) or moderate hazard zone (orange).
3) Unlikely (10–33%) or low hazard zone (yellow).
4) Very unlikely (1–10%) or very low hazard zone (light-yellow).
5) Exceptionally unlikely (0–1%) or exceptionally low hazard zone (white)
These maps represent the likelihood of phenomenon distribution, whether by inundation (lahars, blocky lava flows), invasion (concentrated PDCs), or accumulation (tephra fallout).
Figure 4 shows the likelihood (isarithmic) maps of 1 cm of tephra fallout accumulation. In these maps, four labeled contours (i.e., 1, 10, 33, and 66%) are used to draw the qualitative limits of the likelihood of tephra fallout distribution. Each one of these contours connects the raster points where the probabilities of 1 cm (or 10 kg m−2) of tephra accumulation are equal. In this case, 10 kg m−2 equals 1 cm of tephra accumulation because we used a 1,000 kg m−3 density of pumice tephra in all simulations. Contours were computed with the GDAL function (contours). In addition to contours, a continuous color gradient was employed (red, orange, yellow, and light-yellow). Thompson et al. (2015) have shown that this way of representing the hazard of tephra fallout is better understood by both communities and stakeholders alike. In the case of mass flows, the likelihood maps of concentrated PDCs (Figure 5A), lahars (Figure 5C), and blocky lava flows (Figure 5E) are presented in Figure 5.
FIGURE 4. Likelihood maps of 1 cm of tephra fallout accumulation (alternatively: 10 kg m−2 or 10 mm). (A,B), likelihood maps of small-size eruptions of magnitude 3.0–4.0 (VEI 3) (1:500,000 scale); (C,D), likelihood maps of medium-size eruptions of magnitude 4.0–5.0 (VEI 4) (1:1,000,000 scale); (E,F), likelihood maps for large-size eruptions of magnitude 5.0–6.0 (VEI 5) (1:2,000,000 scale). (A,C,E) represent eruptions during the rainy season (from January to March); (B,D,F) depict eruptions during the dry season (from April to December).
FIGURE 5. (A) likelihood map of concentrated PDCs. (B) hazard zone map of concentrated PDCs. (C) likelihood map of lahars. (D) hazard zone map of lahars. (E) likelihood map of blocky lava flows. (F) hazard zone map of blocky lava flows. (all maps in 1:100,000 scale).
To create a final multi-hazard map, we decided to stack hazard zones of each phenomenon, instead of obtaining the mean of all the three probability rasters. To do this, vector polygons (e.g., shapefiles, .shp) are needed. For instance, to obtain the high hazard zone polygon from the probability raster of concentrated PDCs, we calculated a new raster where all the values above 66% were converted to one, and all values below 66% were converted to 0. Then, we converted this new raster to a vector polygon with the GDAL function (polygonize). Additionally, all gaps inside the limits of this high hazard zone polygon were included inside the high hazard zone to improve the readability of the map. All other hazard zones of PDCs were obtained in the same manner and following the same qualitative intervals from the likelihood classification presented above (Mastrandrea et al., 2010). We obtained the hazard zone maps of lahars and blocky lava flows following the same procedure. The hazard zone maps of concentrated PDCs (Figure 5B), lahars (Figure 5D), and blocky lava flows (Figure 5F) are presented in Figure 5, while Figure 6 shows the final multi-hazard map. The multi-hazard map was created by stacking the hazard zone polygons of each one of these single maps in the following manner: all three very low hazard zones polygons first, then the three low hazard zones, followed by the three moderate hazard zones, and finally the three high hazard zones. Furthermore, we included the conditional likelihoods of tephra accumulation from the medium and large-size eruptions of both seasons (Figures 4C–F). The rainy season eruptions were assigned a red color, while dry season eruptions were assigned a purple color to be easily distinguished (Figure 6).
FIGURE 6. Final integrated multi-hazard zone map of tephra fallout and mass flows (1:120,000 scale).
All three scenarios of tephra fallout considered the seasonality of wind conditions, i.e., the variations in wind direction and velocity between the rainy and dry seasons in northern Chile. The left column of the tephra fallout probability maps shows the results for the rainy season (January–March) (Figures 4A,C,E), while the right column shows the results for the dry season (April–December) (Figures 4B,D,F). The results show a higher likelihood of tephra accumulation in Chilean localities during the rainy season (January–March) than during the dry season (April–December) (Figures 4B,D,F).
Large-size eruptions in the rainy season (Figure 4E) are likely (66–100%) to accumulate 1 cm of tephra on the San Pedro and Ascotán stations; and on the Cerro Pabellón power plant. However, these eruptions are about as likely as not (33–66%) to settle 1 cm of tephra on the Conchi locality. Moreover, these eruptions are unlikely (10–33%) to deposit 1 cm of tephra on the Lasana, Chiu Chiu, Aiquina, Toconce, and Caspana localities; and on the El Abra Copper Mine. Furthermore, these eruptions are very unlikely (1–10%) to accumulate 1 cm of tephra on the Calama, Ollagüe, Río Grande localities; on the Radomiro Tomic, Chuquicamata, and Ministro Hales copper mines; and on the El Loa international airport.
Large-size eruptions in the dry season (Figure 4F) are likely (66–100%) to settle 1 cm of tephra on the Cerro Pabellón power plant, and about as likely as not (33–66%) to do so on the Ascotán station. Further, these eruptions are unlikely (10–33%) to deposit 1 cm of tephra on the Toconce locality and the San Pedro station. Besides, they are very unlikely (1–10%) to accumulate 1 cm of tephra in the Conchi, Caspana, Aiquina, Lasana, and Chiu Chiu localities; and on the El Abra copper mine.
Medium-size eruptions in the rainy season (Figure 4C) are about as likely as not (33–66%) to settle 1 cm of tephra on the Cerro Pabellón power plant and the electrical towers and power lines, whereas medium-size eruptions in the dry season (Figure 4D) are likely (66–100%) to do so.
Small-size eruptions in the rainy season (Figure 4A) are very unlikely (1–10%) to deposit 1 cm of tephra on the Cerro Pabellón power plant, although, small-size eruptions in the dry season (Figure 4B) are unlikely (10–33%) to do so.
During the rainy season, large-size eruptions are likely (66–100%) to accumulate 1 cm of tephra on the CH–21 highway, the freight railway, electrical towers, and power lines, whereas, medium-size eruptions are about as likely as not (33–66%) to do so, and small-size eruptions are very unlikely (1–10%) to do so.
Regarding highly populated centers, the accumulation of 1 cm of tephra in Calama after small to medium-size eruptions is exceptionally unlikely (0–1%) during both seasons (Figures 4A–D). Nonetheless, the accumulation of 1 cm of tephra in Calama after large-size eruptions is very unlikely (1–10%) during the rainy season (Figure 4E) and exceptionally unlikely (0–1%) during the dry season (Figure 4F).
Other localities such as Cosca, San Pedro de Atacama, Quillagüa, Huatacondo, María Elena, Sierra Gorda, Toconao, Tocopilla, and Antofagasta are exceptionally unlikely (0–1%) to be covered by 1 cm of tephra fallout after eruptions of all sizes in both seasons.
Concentrated PDCs produced by future eruptions are about as likely as not (33–66%) to invade the San Pedro de Inacaliri river valley; they are unlikely (10–33%) to invade the CH–21 highway, freight railway, and electrical towers surrounding the volcano; and very unlikely (1–10%) to invade the San Pedro station (Figures 5A,B).
Syn-eruptive lahars generated by future eruptions are exceptionally unlikely (0–1%) to inundate the San Pedro station, or the transport and energy infrastructure surrounding the volcano (Figures 5C,D).
Blocky lava flows produced during future effusive eruptions are likely (66–100%) to inundate the northern and southwestern flanks of the San Pedro volcano (Figures 5E,F). Further, blocky lava flows are likely (66–100%) to inundate various sections of the international highway, freight railway, along with some electrical transmission towers (Figures 5E,F).
Volcanic systems often require many hazard assessments with complementary approaches to bring forth alternative perspectives on hazard forecasting (Calder et al., 2015; Martí, 2017). The previous hazard assessment of the San Pedro volcano (Bertin and Amigo, 2015) produced simulations for tephra fallout by using the Tephra2 model (Bonadonna et al., 2005), and simulations of PDCs by using the Energy Cone model (Malin and Sheridan, 1982). However, the previous assessment did not produce simulations for lahars or blocky lava flows, the latter were not considered because the authors evaluated a different time interval (50 ka to present) in the geological history of the volcano (Bertin and Amigo, 2015).
Our tephra fallout assessment complements the 2015 hazard assessment (Bertin and Amigo, 2015) for multiple reasons. First, the 2015 assessment considered medium (VEI 4–5) and large-size (VEI 5–6) scenarios, whereas the present assessment considers 15 eruptive intra-scenarios from magnitudes 3.0 to 6.0, synthesized into three eruptive scenarios of small (VEI 3), medium (VEI 4), and large size (VEI 5). Second, the present work uses a different visualization of the hazard zones of tephra fallout than the previous hazard assessment, which aids stakeholders and the civil community to understand them, in agreement with previous works (Thompson et al., 2015). Moreover, the representation of wind seasonality is synthesized into only two seasons (rainy and dry seasons), whereas, in the 2015 assessment, this representation is done for the four standard climate seasons (summer, fall, winter, and spring). Third, the 2015 assessment simulated a greater time interval for wind data (16 years) than the present work (11 years), although the number of simulated wind profiles is greater in the present assessment than in the 2015 assessment. Even though both works used the same model (Tephra2), the probabilistic toolbox, TephraProb (Biass et al., 2016b), facilitated the production of a high number of simulations for each intra-scenario in the present assessment, something that was not available for the previous authors, as TephraProb was made available years after the development of the 2015 assessment.
The 2015 assessment used the Energy Cone model introduced by Malin and Sheridan (1982) to simulate PDCs (Bertin and Amigo, 2015). The main difference between the Energy Cone and the VolcFlow models is that the Energy cone is a statistical/empirical model, whereas VolcFlow is a numerical model. Even though the empirical relationships between the parameters of the Energy Cone model are well constrained (Newhall and Hoblitt, 2002), it does not consider the initial volume of the simulated deposits (Ogburn and Calder, 2017), which is crucial for the co-parameterization of phenomena size between the same intra-scenarios in the present assessment. Finally, the hazard zones PDCs in the present assessment are a complement to the hazard zones proposed in the 2015 assessment.
Our blocky lava flow assessment is an improvement because the previous assessment (Bertin and Amigo, 2015) did not simulate blocky lava flows. Even though the Decreasing Probability model simulates ‘a'ā lava flows more effectively, we think it can also simulate blocky lava flows because it was able to reproduce the length of the real lava flow deposits of the San Pedro volcano. Additionally, even though the recent geological history of the San Pedro volcano had migrated to higher-silica phenomena (i.e., lava domes), a blocky lava flow assessment can still be useful for the effusive phases of future eruptions.
Our lahar assessment can be seen as a complement to hazard zones proposed in the 2015 assessment because previous zones were not delimited with lahar models of any type because they were estimated from the distribution of geological deposits (Bertin and Amigo, 2015). In the present work, the probabilistic use of LaharFlow (Woodhouse et al., 2016), is an improvement in the forecasting of future lahars at the San Pedro volcano. The LaharFlow model allowed us to co-parameterize its volume with other models of volcanic phenomena within the same simulated intra-scenario.
We did not consider other volcanic phenomena, such as VBPs, diluted PDCs, and volcanic avalanches by sector collapse, as they are not within the scope of this study. In the case of VBPs, there are two limitations to their implementation in this methodology. First, mass/volume is not a parameter in models of VBPs (Mastin, 2001; Biass et al., 2016c; Bertin 2017). Second, recent works have demonstrated that the launch velocity of VBPs, one of the first-order parameters in VBPs models, does not correlate with the size of eruptions (Maeno et al., 2013; Taddeucci et al., 2017). Therefore, we could not integrate models of VBPs with the simulated explosive intra-scenarios in the present assessment. In the case of sector collapse, we did not produce simulations for volcanic avalanches because of the low recurrence of this phenomenon globally and regionally (Sandri et al., 2014). In the case of diluted PDCs, we only could produce an assessment with the single-fluid version of VolcFlow, which models concentrated PDCs, but not diluted PDCs (Kelfoun et al., 2009). Therefore, the results of these simulations are limited, as they are not representative of the whole areas that real PDCs could cover. However, they are representative of the areas where the largest portion of the volume of PDCs could be deposited (Ogburn and Calder, 2017). Even though the areas where diluted PDCs could be distributed are not simulated, the areas that this phenomenon could cover are delimited by the hazard zones of simulated blocky lava flows in the final multi-hazard map (Figure 6).
In the case of the distribution of tephra fallout, the rainy season has low-velocity winds with W-ward, S-ward, and N-ward components, which causes simulated tephra to be transported equally toward the W and E (Figures 4A,C,E). Conversely, the dry season has fast E-ward winds, causing simulated tephra to distribute toward the E (Figures 4B,D,F). Plus, the larger the size of the eruption, the more it is affected by wind conditions (see Figure 6). This is because larger size eruptions have higher column heights (Mastin et al., 2009), therefore, they are affected by the high-altitude high-velocity W-ward winds of the rainy season (Figure 3). The San Pedro and Ascotán freight railway stations have the highest likelihood of tephra accumulation of all localities evaluated in this study (Figure 4). From the results, we suggest that a special emphasis should be placed on the Toconce locality, due to its location, as it seems to have a similar likelihood of tephra accumulation after large eruptions independent of the season (Figures 4E,F); being unlikely (10–33%) to be covered by 1 cm of tephra in both seasons, something that does not happen in other localities.
Regarding the distribution of mass flows, the northern and southwestern flanks are likely to be the areas most affected by all types of mass flows. In the case of the northern flank, we think this is because it connects to the crater of the modern western cone with a high-slope angle. Meanwhile, the southern flank has an incision on the southwestern face of the summit of the modern western cone, again, with a high-slope angle. The current topography of the volcano promotes the distribution of mass flows toward the northern and southwestern flanks, which increases the probability of mass flows reaching those locations. However, the topography surrounding the volcano controls the probability of mass flow distribution at farther distances from the crater. The general trend of the topography surrounding the volcano is to decrease in elevation toward the W. Therefore, mass flows tend to have a W-ward distribution; this can be seen especially in the distribution of concentrated PDCs (Figures 5A,B) and blocky lava flows (Figures 5E,F). The current deposits of blocky lava flows will be key in containing, blocking, or redirecting the distribution of future mass flows, both in the northern and southwestern flanks of the San Pedro volcano (Figure 6). In the simulations, the fourth deposit of blocky lava flows bounds the distribution of mass flows on the northern flank (Figure 2). Likewise, the 12th lava flow interrupts the distribution of mass flows on the southwestern flank (Figure 2).
In the case of the estimation of probabilities, we treated the empirical/statistical Decreasing Probability model as a deterministic model. Thus, we obtained the mean probability raster through Eq. (6). This is done because one simulation of the Q-LavHA model reproduces the direction of a one pixel-wide lava flow event instead of the full-width of a lava flow event (hazard footprint), as analytical or numerical models do. Therefore, 5000 iterations were used to reproduce the distribution of one lava flow event. If we did not make this correction, the probabilities were anomalously concentrated between 0 and 10% in the probability raster, thus making the delimitation of hazard zones subjective. Our treatment of this model as deterministic agrees with how the probabilities of empirical/statistical models have been estimated in other hazard assessments, for example, for the Energy Cone model (Tierz et al., 2018; Clarke et al., 2020).
Regarding the source locations of phenomena, we suggest that a hexagonal tessellation is more efficient than a square tessellation at discretizing the volcanic crater area. This is because source locations are more equally distributed than in a square tessellation. Even though we did not test the influence of the hexagon diameters chosen (200 and 700 m), we selected these values considering that the number of hexagons that fitted inside the crater areas would result in a fair computational time cost for both models. Furthermore, we assumed that all possible source locations have a uniform probability of occurrence.
The thickness values of the blocky lava flow deposits are relatively high (Table 2). With the TanDEM–X DEM, we found that the larger thicknesses were found near the front of the lava flows. The simulation of these front thickness values would not be advisable because they are an overestimation of the front thickness of the lava flow as it is flowing down the volcano during an eruption. The TanDEM–X DEM shows that most thickness values of the largest lava flow deposits (fourth and fifth; Figure 2) are within the 20–40 m range if they are measured at half of their Manhattan length. The eighth deposit has almost half the Manhattan length of the fourth and fifth deposits, and its front thickness value is 20 m. Therefore, we used this field-measured value (20 m) as the maximum thickness corrective value (Hp). We think this value is a better representation of the front thickness a blocky lava flow would have as it is flowing down the flanks of the volcano during an effusive eruption.
The San Pedro volcano has the fifth highest-threat index among northern Chile volcanoes. Its historically active behavior and the magnitude of its recent eruptions have shown the importance of forecasting the potential distribution of future volcanic phenomena at this volcano. In this assessment, four types of volcanic phenomena were simulated using accessible computational models. This assessment helps to quantify the hazard through multi-phenomena eruptive scenarios with continuous sizes. The hazard zones proposed in this work are a complement to previous hazard assessments at the San Pedro volcano. The following conclusions were reached:
1) There is a higher likelihood of tephra impact in Chilean localities and infrastructure during the rainy season (January–March) than during the dry season (April–December).
2) Large-size eruptions (VEI 5) in the rainy season (January–March) are very unlikely (1–10%) to accumulate 1 cm of tephra in the city of Calama (and its surrounding mining sites).
3) Large-size eruptions (VEI 5) in the rainy season are exceptionally unlikely (0–1%) to deposit 1 cm of tephra in the cities of María Elena, Sierra Gorda, San Pedro de Atacama, Tocopilla, and Antofagasta.
4) Electrical (power lines and towers) and transport (highway and railways) infrastructure, along with river valleys surrounding the volcano, could be impacted by both tephra fallout and mass flows during future eruptions.
5) The Cerro Pabellón power plant has the highest likelihood of tephra fallout impact than any other infrastructure surrounding the volcano, and the likelihood is higher during the dry season.
Both vulnerable communities and governmental entities can help mitigate the impact of potential future eruptions by being aware of the hazards, educating the community, and developing an effective emergency plan for the San Pedro volcano. Volcanic hazard maps can provide preemptive information to governmental entities for future land-use planning, civil protection, and management of volcanic crises in the Antofagasta Region.
The original contributions presented in the study are included in the article/Supplementary Material, further inquiries can be directed to the corresponding author.
NA-V conceived the study, produced the simulations, and wrote the manuscript with inputs from M-PR-H and AE. FA acquired funding for the research. All authors read, reviewed, and approved the final manuscript.
This research has been funded by the ANID FONDAP 15110017 project, National Research Center for Integrated Natural Disaster Management (CIGIDEN), granted by the Chilean National Agency for Research and Development (ANID) and the FIC–R BIP 30488832-0 project, Mitigation of Risk Associated to Volcanic Processes in the Antofagasta Region, granted by the Innovation Fund for Regional Competitiveness (FIC–R) of the Antofagasta Regional Government, Chile (GORE Antofagasta). M-PR-H is funded by the SNSF 188757 project, A new probabilistic framework for regional volcanic risk assessment including hazards interacting at multiple temporal and spatial scales, granted by the Swiss National Science Foundation (SNSF). AE is funded by the ANID 21192242 National PhD Grant.
We thank John Santibañez and Francisco Armijo for their insights about some aspects of the research. We thank Dr. Gabriel Ureta for providing access to the TanDEM–X digital elevation model through the DEM_GEOL2956 proposal and the German Aerospace Center (Deutsches Zentrum für Luft- und Raumfahrt, DLR) for the development of the TanDEM–X project. We thank reviewers Sam Poppe and Marta Della Setta who helped to improve the manuscript with their constructive revisions and feedback. Finally, we thank editor Karoly Nemeth for the editorial handling of the manuscript.
The authors declare that the research was conducted in the absence of any commercial or financial relationships that could be construed as a potential conflict of interest.
All claims expressed in this article are solely those of the authors and do not necessarily represent those of their affiliated organizations, or those of the publisher, the editors and the reviewers. Any product that may be evaluated in this article, or claim that may be made by its manufacturer, is not guaranteed or endorsed by the publisher.
The Supplementary Material for this article can be found online at: https://www.frontiersin.org/articles/10.3389/feart.2022.897315/full#supplementary-material
1https://rnvv.sernageomin.cl/volcan-san-pedro/
2https://github.com/geoscience-community-codes/tephra2
3https://github.com/e5k/TephraProb
5https://www.laharflow.bristol.ac.uk
6https://we.vub.ac.be/en/q-lavha
7https://github.com/drewstev/arcgridwrite
Aguilera, F., Layana, S., Rojas, F., Arratia, P., Wilkes, T. C., González, C., et al. (2020). First measurements of gas flux with a low-cost smartphone sensor-based UV camera on the volcanoes of northern Chile. Remote Sens. 12 (13), 2122. doi:10.3390/rs12132122
Alcorn, R., Panter, K. S., and Gorsevski, P. V. (2013). A GIS-based volcanic hazard and risk assessment of eruptions sourced within Valles Caldera, New Mexico. J. Volcanol. Geotherm. Res. 267, 1–14. doi:10.1016/j.jvolgeores.2013.09.005
Amigo, A. (2012). Volcano monitoring and hazard assessments in Chile. Volcanica 4 (S1), 1–20. doi:10.30909/vol.04.S1.0120
Amigo, Á., Bertin, D., and Orozco, G. (2012). Peligros volcánicos de la zona norte de Chile, Regiones de Arica y Parinacota, Tarapacá, Antofagasta y Atacama. Carta Geológica de Chile, Serie Geología Ambiental, 17. Santiago: Servicio Nacional de Geología y Minería SERNAGEOMIN.
Aravena, D., Villalón, I., and Sánchez, P. (2015). Igneous related geothermal resource in the Chilean Andes. Proc. World Geotherm. Congr. 2015, 401. doi:10.1016/0375-6505(88)90068-5
Barcaza, G., Nussbaumer, S. U., Tapia, G., Valdés, J., García, J.-L., Videla, Y., et al. (2017). Glacier inventory and recent glacier variations in the Andes of Chile, South America. Ann. Glaciol. 58 (75pt2), 166–180. doi:10.1017/aog.2017.28
Becerril, L., Bartolini, S., Sobradelo, R., Martí, J., Morales, J. M., Galindo, I., et al. (2014). Long-term volcanic hazard assessment on El Hierro (Canary Islands). Nat. Hazards Earth Syst. Sci. 14 (7), 1853–1870. doi:10.5194/nhess-14-1853-2014
Becerril, L., Martí, J., Bartolini, S., and Geyer, A. (2017). Assessing qualitative long-term volcanic hazards at Lanzarote Island (Canary Islands). Nat. Hazards Earth Syst. Sci. 17 (7), 1145–1157. doi:10.5194/nhess-17-1145-2017
Bertin, D. (2017). 3-D ballistic transport of ellipsoidal volcanic projectiles considering horizontal wind field and variable shape-dependent drag coefficients. J. Geophys. Res. Solid Earth 122 (2), 1126–1151. doi:10.1002/2016JB013320
Bertin, D., and Amigo, Á. (2019). Geología del Volcán San Pedro, Región de Antofagasta. Carta Geológica de Chile, Serie Geología Básica, 201. Santiago: Servicio Nacional de Geología y Minería SERNAGEOMIN.
Bertin, D., and Amigo, Á. (2015). Peligros del Volcán San Pedro, Región de Antofagasta. Carta Geológica de Chile, Serie Geología Ambiental, 25. Santiago: Servicio Nacional de Geología y Minería SERNAGEOMIN.
Biass, S., and Bonadonna, C. (2013). A fast GIS-based risk assessment for tephra fallout: The example of Cotopaxi volcano, Ecuador, Part I: Probabilistic hazard assessment. Nat. Hazards (Dordr). 65 (1), 477–495. doi:10.1007/s11069-012-0378-z
Biass, S., Bonadonna, C., Connor, L., and Connor, C. (2016b). TephraProb: A Matlab package for probabilistic hazard assessments of tephra fallout. J. Appl. Volcanol. 5 (1), 10. doi:10.1186/s13617-016-0050-5
Biass, S., Bonadonna, C., di Traglia, F., Pistolesi, M., Rosi, M., Lestuzzi, P., et al. (2016a). Probabilistic evaluation of the physical impact of future tephra fallout events for the Island of Vulcano, Italy. Bull. Volcanol. 78 (5), 37. doi:10.1007/s00445-016-1028-1
Biass, S., Falcone, J. L., Bonadonna, C., Di Traglia, F., Pistolesi, M., Rosi, M., et al. (2016c). Great balls of fire: A probabilistic approach to quantify the hazard related to ballistics—a case study at La Fossa volcano, Vulcano Island, Italy. J. Volcanol. Geotherm. Res. 325, 1–14. doi:10.1016/j.jvolgeores.2016.06.006
Biass, S., Scaini, C., Bonadonna, C., Folch, A., Smith, K., Höskuldsson, A., et al. (2014). A multi-scale risk assessment for tephra fallout and airborne concentration from multiple Icelandic volcanoes – Part 1: Hazard assessment. Nat. Hazards Earth Syst. Sci. 14 (8), 2265–2287. doi:10.5194/nhess-14-2265-2014
Biass, S., Todde, A., Cioni, R., Pistolesi, M., Geshi, N., Bonadonna, C., et al. (2017). Potential impacts of tephra fallout from a large-scale explosive eruption at Sakurajima volcano, Japan. Bull. Volcanol. 79 (10), 73. doi:10.1007/s00445-017-1153-5
Blong, R. (2000). “Volcanic hazard and risk management,” in The encyclopedia of volcanoes. Editor H. Sigurdsson. 1st ed. (Academic Press), 1215–1227.
Bonadonna, C., Connor, C. B., Houghton, B. F., Connor, L., Byrne, M., Laing, A., et al. (2005). Probabilistic modeling of tephra dispersal: Hazard assessment of a multiphase rhyolitic eruption at Tarawera, New Zealand. J. Geophys. Res. 110 (B3), B03203. doi:10.1029/2003JB002896
Bonadonna, C., and Houghton, B. F. (2005). Total grain-size distribution and volume of tephra-fall deposits. Bull. Volcanol. 67 (5), 441–456. doi:10.1007/s00445-004-0386-2
Bonasia, R., Scaini, C., Capra, L., Nathenson, M., Siebe, C., Arana-Salinas, L., et al. (2014). Long-range hazard assessment of volcanic ash dispersal for a Plinian eruptive scenario at Popocatépetl volcano (Mexico): Implications for civil aviation safety. Bull. Volcanol. 76 (1), 789. doi:10.1007/s00445-013-0789-z
Bonne, K., Kervyn, M., Cascone, L., Njome, S., Van Ranst, E., Suh, E., et al. (2008). A new approach to assess long‐term lava flow hazard and risk using GIS and low‐cost remote sensing: The case of Mount Cameroon, West Africa. Int. J. Remote Sens. 29 (22), 6539–6564. doi:10.1080/01431160802167873
Brewer, C. A. (2003). A transition in improving maps: The ColorBrewer example. Cartogr. Geogr. Inf. Sci. 30 (2), 159–162. doi:10.1559/152304003100011126
Calder, E., Wagner, K., and Ogburn, S. E. (2015). “Volcanic hazard maps,” in Global volcanic hazards and risk. Editors S. C. Loughlin, S. Sparks, S. K. Brown, S. F. Jenkins, and C. Vye-Brown. 1st ed. (Cambridge: Cambridge University Press), 335–342. doi:10.1017/CBO9781316276273.022
Capra, L., Norini, G., Groppelli, G., Macías, J. L., and Arce, J. L. (2008). Volcanic hazard zonation of the Nevado de Toluca volcano, México. J. Volcanol. Geotherm. Res. 176 (4), 469–484. doi:10.1016/j.jvolgeores.2008.04.016
Carvalho, L. M. V. (2020). Assessing precipitation trends in the Americas with historical data: A review. WIREs Clim. Change 11 (2). doi:10.1002/wcc.627
Casertano, L. (1963). Catalogue of the active volcanoes and solfatara fields of the Chilean continent. Catalogue of the active volcanoes of the world, including solfatara fields, 15. Rome: International Volcanological Association.
Cavalcanti, I. F. A. (2012). Large scale and synoptic features associated with extreme precipitation over South America: A review and case studies for the first decade of the 21st century. Atmos. Res. 118, 27–40. doi:10.1016/j.atmosres.2012.06.012
Charbonnier, S. J., Germa, A., Connor, C. B., Gertisser, R., Preece, K., Komorowski, J.-C., et al. (2013). Evaluation of the impact of the 2010 pyroclastic density currents at Merapi volcano from high-resolution satellite imagery, field investigations and numerical simulations. J. Volcanol. Geotherm. Res. 261, 295–315. doi:10.1016/j.jvolgeores.2012.12.021
Charbonnier, S. J., and Gertisser, R. (2012). Evaluation of geophysical mass flow models using the 2006 block-and-ash flows of Merapi Volcano, Java, Indonesia: Towards a short-term hazard assessment tool. J. Volcanol. Geotherm. Res. 231, 87–108. doi:10.1016/j.jvolgeores.2012.02.015
Charbonnier, S. J., Thouret, J.-C., Gueugneau, V., and Constantinescu, R. (2020). New insights into the 2070 cal yr BP pyroclastic currents at El Misti volcano (Peru) from field investigations, satellite imagery and probabilistic modeling. Front. Earth Sci. (Lausanne). 8, 557788. doi:10.3389/feart.2020.557788
Clarke, B., Tierz, P., Calder, E., and Yirgu, G. (2020). Probabilistic volcanic hazard assessment for pyroclastic density currents from pumice cone eruptions at Aluto Volcano, Ethiopia. Front. Earth Sci. (Lausanne). 8, 348. doi:10.3389/feart.2020.00348
Decker, R. W. (1986). Forecasting volcanic eruptions. Annu. Rev. Earth Planet. Sci. 14, 267–291. doi:10.1146/annurev.ea.14.050186.001411
Delunel, R., Blard, P.-H., Martin, L. C. P., Nomade, S., and Schlunegger, F. (2016). Long term low latitude and high elevation cosmogenic 3He production rate inferred from a 107 ka-old lava flow in northern Chile: 22°S–3400 m a.s.l. Geochimica Cosmochimica Acta 184, 71–87. doi:10.1016/j.gca.2016.04.023
Doyle, E. E. H., McClure, J., Johnston, D. M., and Paton, D. (2014). Communicating likelihoods and probabilities in forecasts of volcanic eruptions. J. Volcanol. Geotherm. Res. 272, 1–15. doi:10.1016/j.jvolgeores.2013.12.006
Espín Bedón, P. A., Mothes, P. A., Hall, M. L., Valverde Arcos, V., and Keen, H. (2019). The “Mera” lahar deposit in the upper Amazon basin: Transformation of a late Pleistocene collapse at Huisla volcano, central Ecuador. J. Volcanol. Geotherm. Res. 385, 103–119. doi:10.1016/j.jvolgeores.2018.10.008
Esposti Ongaro, T., Komorowski, J.-C., Legendre, Y., and Neri, A. (2020). Modelling pyroclastic density currents from a subplinian eruption at La Soufrière de Guadeloupe (West Indies, France). Bull. Volcanol. 82 (12), 76. doi:10.1007/s00445-020-01411-6
Farr, T. G., Rosen, P. A., Caro, E., Crippen, R., Duren, R., Hensley, S., et al. (2007). The shuttle radar topography mission. Rev. Geophys. 45 (2), RG2004. doi:10.1029/2005rg000183
Felpeto, A., Araña, V., Ortiz, R., Astiz, M., and García, A. (2001). Assessment and modelling of lava flow hazard on Lanzarote (Canary Islands). Nat. hazards 23 (2), 247–257. doi:10.1023/a:1011112330766
Felpeto, A., Martí, J., and Ortiz, R. (2007). Automatic GIS-based system for volcanic hazard assessment. J. Volcanol. Geotherm. Res. 166 (2), 106–116. doi:10.1016/j.jvolgeores.2007.07.008
Flynn, I. T. W., and Ramsey, M. S. (2020). Pyroclastic density current hazard assessment and modeling uncertainties for Fuego volcano, Guatemala. Remote Sens. 12 (17), 2790. doi:10.3390/rs12172790
Folch, A. (2012). A review of tephra transport and dispersal models: Evolution, current status, and future perspectives. J. Volcanol. Geotherm. Res. 235–236, 96–115. doi:10.1016/j.jvolgeores.2012.05.020
Francis, P. W., Roobol, M. J., Walker, G. P. L., Cobbold, P. R., and Coward, M. (1974). The San Pedro and San Pablo volcanoes of northern Chile and their hot avalanche deposits. Geol. Rundsch. 63 (1), 357–388. doi:10.1007/BF01820994
Francis, P. W., and Wells, G. L. (1988). Landsat thematic mapper observations of debris avalanche deposits in the Central Andes. Bull. Volcanol. 50 (4), 258–278. doi:10.1007/BF01047488
Gardeweg, M., and Amigo, Á. (2015). Peligros del volcán Láscar, Región de Antofagasta. Carta Geológica de Chile, Serie Geología Ambiental, 22. Santiago: Servicio Nacional de Geología y Minería SERNAGEOMIN.
Garreaud, R. D. (2009). The Andes climate and weather. Adv. Geosci. 22, 3–11. doi:10.5194/adgeo-22-3-2009
Gjerløw, E., Höskuldsson, Á., Bartolini, S., Biass, S., Mossoux, S., Gilbert, J., et al. (2022). The volcanic hazards of Jan Mayen Island (North-Atlantic). Front. Earth Sci. (Lausanne). 10, 730734. doi:10.3389/feart.2022.730734
Godoy, B., Wörner, G., Kojima, S., Aguilera, F., Simon, K., Hartmann, G., et al. (2014). Low-pressure evolution of arc magmas in thickened crust: The San Pedro–Linzor volcanic chain, Central Andes, Northern Chile. J. S. Am. Earth Sci. 52, 24–42. doi:10.1016/j.jsames.2014.02.004
Godoy, B., Wörner, G., Le Roux, P., de Silva, S., Parada, M. Á., Kojima, S., et al. (2017). Sr- and Nd-isotope variations along the Pleistocene San Pedro–Linzor volcanic chain, N. Chile: Tracking the influence of the upper crustal Altiplano-Puna Magma Body. J. Volcanol. Geotherm. Res. 341, 172–186. doi:10.1016/j.jvolgeores.2017.05.030
González-Maurel, O., Deegan, F. M., le Roux, P., Harris, C., Troll, V. R., Godoy, B., et al. (2020). Constraining the sub-arc, parental magma composition for the giant Altiplano-Puna Volcanic Complex, northern Chile. Sci. Rep. 10 (1), 6864. doi:10.1038/s41598-020-63454-1
González-Maurel, O., Godoy, B., le Roux, P., Rodríguez, I., Marín, C., Menzies, A., et al. (2019a). Magmatic differentiation at La Poruña scoria cone, Central Andes, northern Chile: Evidence for assimilation during turbulent ascent processes, and genetic links with mafic eruptions at adjacent San Pedro volcano. Lithos 338–339, 128–140. doi:10.1016/j.lithos.2019.03.033
González-Maurel, O., le Roux, P., Godoy, B., Troll, V. R., Deegan, F. M., Menzies, A., et al. (2019b). The great escape: Petrogenesis of low-silica volcanism of Pliocene to Quaternary age associated with the Altiplano-Puna Volcanic Complex of northern Chile (21°10′-22°50′S). Lithos 346–347, 105162. doi:10.1016/j.lithos.2019.105162
Grosse, P., Euillades, P. A., Euillades, L. D., and van Wyk de Vries, B. (2014). A global database of composite volcano morphometry. Bull. Volcanol. 76 (1), 784. doi:10.1007/s00445-013-0784-4
Gueugneau, V., Kelfoun, K., Charbonnier, S., Germa, A., and Carazzo, G. (2020). Dynamics and impacts of the May 8th, 1902 pyroclastic current at Mount Pelée (Martinique): New insights from numerical modeling. Front. Earth Sci. (Lausanne). 8, 279. doi:10.3389/feart.2020.00279
Gueugneau, V., Kelfoun, K., and Druitt, T. (2019). Investigation of surge-derived pyroclastic flow formation by numerical modelling of the 25 June 1997 dome collapse at Soufrière Hills Volcano, Montserrat. Bull. Volcanol. 81 (4), 25. doi:10.1007/s00445-019-1284-y
Guimarães, L. F., Nieto-Torres, A., Bonadonna, C., and Frischknecht, C. (2021). A new inclusive volcanic risk ranking, Part 2: Application to Latin America. Front. Earth Sci. (Lausanne). 9, 757742. doi:10.3389/feart.2021.757742
GVP (2013b). “Lascar (355100) [data set],” in Volcanoes of the world, v. 4.10.4 (09 December 2021). Editor E. Venzke (Washington: Smithsonian Institution, Global Volcanism Program). Available at: https://volcano.si.edu/gvp_votw.cfm?vn=355100.
GVP (2013a). “San Pedro–San Pablo (355070) [data set],” in Volcanoes of the world, v. 4.10.4 (09 December 2021). Editor E. Venzke (Washington: Smithsonian Institution, Global Volcanism Program). Available at: https://volcano.si.edu/volcano.cfm?vn=355070.
GVP (2013c). Volcanoes of the world version 4.10.4 (09 December 2021). Editor E. Venzke (Washington: Smithsonian Institution, Global Volcanism Program).
Harris, A. J., and Rowland, S. (2001). FLOWGO: A kinematic thermo-rheological model for lava flowing in a channel. Bull. Volcanol. 63 (1), 20–44. doi:10.1007/s004450000120
Hayes, J. L., Wilson, T. M., Deligne, N. I., Lindsay, J. M., Leonard, G. S., Tsang, S. W. R., et al. (2020). Developing a suite of multi-hazard volcanic eruption scenarios using an interdisciplinary approach. J. Volcanol. Geotherm. Res. 392, 106763. doi:10.1016/j.jvolgeores.2019.106763
Jay, J. A., Welch, M., Pritchard, M. E., Mares, P. J., Mnich, M. E., Melkonian, A. K., et al. (2013). Volcanic hotspots of the central and southern Andes as seen from space by ASTER and MODVOLC between the years 2000 and 2010. Geol. Soc. Lond. Spec. Publ. 380 (1), 161–185. doi:10.1144/SP380.1
Jiménez, D., Becerril, L., Bartolini, S., Escobar, D., and Martí, J. (2020). Making a qualitative volcanic-hazards map by combining simulated scenarios: An example for San Miguel Volcano (El Salvador). J. Volcanol. Geotherm. Res. 395, 106837. doi:10.1016/j.jvolgeores.2020.106837
Jorquera, C., Rodriguez, I., Bertin, L. J., and Flores, F. (2019). Peligros del volcán Guallatiri, Región de Arica y Parinacota. Carta Geológica de Chile, Serie Geología Ambiental, 35. Santiago: Servicio Nacional de Geología y Minería SERNAGEOMIN.
Kelfoun, K. (2017). A two-layer depth-averaged model for both the dilute and the concentrated parts of pyroclastic currents: A model for pyroclastic currents. J. Geophys. Res. Solid Earth 122 (6), 4293–4311. doi:10.1002/2017JB014013
Kelfoun, K., Gueugneau, V., Komorowski, J.-C., Aisyah, N., Cholik, N., Merciecca, C., et al. (2017). Simulation of block-and-ash flows and ash-cloud surges of the 2010 eruption of Merapi volcano with a two-layer model. J. Geophys. Res. Solid Earth 122 (6), 4277–4292. doi:10.1002/2017JB013981
Kelfoun, K., Samaniego, P., Palacios, P., and Barba, D. (2009). Testing the suitability of frictional behaviour for pyroclastic flow simulation by comparison with a well-constrained eruption at Tungurahua volcano (Ecuador). Bull. Volcanol. 71 (9), 1057–1075. doi:10.1007/s00445-009-0286-6
Lara, L., Orozco, G., Amigo, Á., and Silva, C. (2011). Peligros volcánicos de Chile. Carta Geológica de Chile, Serie Geología Ambiental, 13. Santiago: Servicio Nacional de Geología y Minería SERNAGEOMIN.
Lindsay, J. M., and Robertson, R. E. A. (2018). Integrating volcanic hazard data in a systematic approach to develop volcanic hazard maps in the Lesser Antilles. Front. Earth Sci. (Lausanne). 6, 42. doi:10.3389/feart.2018.00042
Loughlin, S. C., Sparks, S., Brown, S. K., Jenkins, S. F., and Vye-Brown, C. (Editors) (2015). Global volcanic hazards and risk. (Cambridge: Cambridge University Press). doi:10.1017/CBO9781316276273
Lube, G., Breard, E. C. P., Esposti-Ongaro, T., Dufek, J., and Brand, B. (2020). Multiphase flow behaviour and hazard prediction of pyroclastic density currents. Nat. Rev. Earth Environ. 1 (7), 348–365. doi:10.1038/s43017-020-0064-8
Maeno, F., Nakada, S., Nagai, M., and Kozono, T. (2013). Ballistic ejecta and eruption condition of the vulcanian explosion of Shinmoedake volcano, Kyushu, Japan on 1 February, 2011. Earth Planets Space 65 (6), 609–621. doi:10.5047/eps.2013.03.004
Malin, M. C., and Sheridan, M. F. (1982). Computer-assisted mapping of pyroclastic surges. Science 217 (4560), 637–640. doi:10.1126/science.217.4560.637
Mamani, M., Tassara, A., and Wörner, G. (2008). Composition and structural control of crustal domains in the Central Andes. Geochem. Geophys. Geosyst. 9, Q03006. doi:10.1029/2007gc001925
Marrero, J. M., García, A., Berrocoso, M., Llinares, Á., Rodríguez-Losada, A., Ortiz, R., et al. (2019). Strategies for the development of volcanic hazard maps in monogenetic volcanic fields: The example of La Palma (Canary Islands). J. Appl. Volcanol. 8 (1), 6. doi:10.1186/s13617-019-0085-5
Martí, J. (2017). Assessing volcanic hazard, 1. Oxford University Press. doi:10.1093/oxfordhb/9780190699420.013.32
Marzocchi, W., and Bebbington, M. S. (2012). Probabilistic eruption forecasting at short and long-time scales. Bull. Volcanol. 74 (8), 1777–1805. doi:10.1007/s00445-012-0633-x
Marzocchi, W., Sandri, L., Gasparini, P., Newhall, C., and Boschi, E. (2004). Quantifying probabilities of volcanic events: The example of volcanic hazard at Mount Vesuvius: Quantifying volcanic hazard. J. Geophys. Res. 109, B11201. doi:10.1029/2004JB003155
Marzocchi, W., Selva, J., and Jordan, T. H. (2021). A unified probabilistic framework for volcanic hazard and eruption forecasting. Nat. Hazards Earth Syst. Sci. 21 (11), 3509–3517. doi:10.5194/nhess-21-3509-2021
Mastin, L. G. (2001). A simple calculator of ballistic trajectories for blocks ejected during volcanic eruptions. Open-File Report 2001–0045. Reston, VA: U.S. Geological Survey. doi:10.3133/ofr0145
Mastin, L. G., Guffanti, M., Servranckx, R., Webley, P., Barsotti, S., Dean, K., et al. (2009). A multidisciplinary effort to assign realistic source parameters to models of volcanic ash-cloud transport and dispersion during eruptions. J. Volcanol. Geotherm. Res. 186 (1–2), 10–21. doi:10.1016/j.jvolgeores.2009.01.008
Mastrandrea, M. D., Field, C. B., Stocker, T. F., Edenhofer, O., Ebi, K. L., Frame, D. J., and Zwiers, F. W. (2010). Guidance note for lead authors of the IPCC fifth assessment report on consistent treatment of uncertainties. Available at: https://www.ipcc.ch/site/assets/uploads/2018/05/uncertainty-guidance-note.pdf.
Mead, S., Procter, J., Bebbington, M., and Rodriguez-Gomez, C. (2022). Probabilistic volcanic hazard assessment for national park infrastructure proximal to Taranaki Volcano (New Zealand). Front. Earth Sci. (Lausanne). 10, 832531. doi:10.3389/feart.2022.832531
Michaud-Dubuy, A., Carazzo, G., and Kaminski, E. (2021). Volcanic hazard assessment for tephra fallout in Martinique. J. Appl. Volcanol. 10 (1), 8. doi:10.1186/s13617-021-00106-7
MINVU and IDE (2017). Áreas urbanas consolidadas [Data set]. Ministerio de Vivienda y Urbanismo (MINVU) and Infraestructura de Datos Geoespaciales (IDE). Gobierno de Chile. Available at: https://www.ide.cl/descargas/capas/minvu/Area_Urbana_Consolidada.
Mossoux, S., Saey, M., Bartolini, S., Poppe, S., Canters, F., Kervyn, M., et al. (2016). Q-LavHA: A flexible GIS plugin to simulate lava flows. Comput. Geosciences 97, 98–109. doi:10.1016/j.cageo.2016.09.003
Newhall, C. G., and Hoblitt, R. (2002). Constructing event trees for volcanic crises. Bull. Volcanol. 64 (1), 3–20. doi:10.1007/s004450100173
Newhall, C. G., and Self, S. (1982). The Volcanic Explosivity Index (VEI) an estimate of explosive magnitude for historical volcanism. J. Geophys. Res., 87 (C2), 1231–1238. doi:10.1029/JC087iC02p01231
Nieto-Torres, A., Guimarães, L. F., Bonadonna, C., and Frischknecht, C. (2021). A new inclusive volcanic risk ranking, Part 1: Methodology. Front. Earth Sci. (Lausanne). 9, 697451. doi:10.3389/feart.2021.697451
O’Callaghan, L. J., and Francis, P. W. (1986). Volcanological and petrological evolution of San Pedro volcano, provincia EI Loa, north Chile. J. Geol. Soc. 143 (2), 275–286. doi:10.1144/gsjgs.143.2.0275
Ogburn, S. E., and Calder, E. S. (2017). The relative effectiveness of empirical and physical models for simulating the dense undercurrent of pyroclastic flows under different emplacement conditions. Front. Earth Sci. (Lausanne). 5, 83. doi:10.3389/feart.2017.00083
ONEMI (2019). Plan específico de emergencia por variable de riesgo: Erupciones volcánicas, Región de Antofagasta. Santiago: Oficina Nacional de Emergencia del Ministerio del Interior y Seguridad Pública (ONEMI). Gobierno de Chile.
OVDAS (2022). Reporte de actividad volcánica, enero 2022, Región de Antofagasta (No. 1). Observatorio Volcanológico de los Andes del Sur OVDAS. Santiago: Servicio Nacional de Geología y Minería SERNAGEOMIN.
Patra, A. K., Bauer, A. C., Nichita, C. C., Pitman, E. B., Sheridan, M. F., Bursik, M., et al. (2005). Parallel adaptive numerical simulation of dry avalanches over natural terrain. J. Volcanol. Geotherm. Res. 139 (1–2), 1–21. doi:10.1016/j.jvolgeores.2004.06.014
Payne, D. (1998). Climatic implications of rock glaciers in the arid western cordillera of the Central Andes. Glacial Geol. Geomorphol. rp03/1998.
Petit-Breuilh, M. (2004). La historia eruptiva de los volcanes hispanoamericanos (siglos XVI al XX): El modelo Chileno. Lanzarote: Servicio de Publicaciones, Cabildo Insular de Lanzarote.
Pierson, T. C., Janda, R. J., Thouret, J.-C., and Borrero, C. A. (1990). Perturbation and melting of snow and ice by the 13 November 1985 eruption of Nevado del Ruiz, Colombia, and consequent mobilization, flow and deposition of lahars. J. Volcanol. Geotherm. Res. 41 (1–4), 17–66. doi:10.1016/0377-0273(90)90082-Q
Poland, M. P., and Anderson, K. R. (2020). Partly cloudy with a chance of lava flows: Forecasting volcanic eruptions in the Twenty‐First Century. J. Geophys. Res. Solid Earth 125, e2018JB016974. doi:10.1029/2018JB016974
Pritchard, M. E., Henderson, S. T., Jay, J. A., Soler, V., Krzesni, D. A., Button, N. E., et al. (2014). Reconnaissance earthquake studies at nine volcanic areas of the Central Andes with coincident satellite thermal and InSAR observations. J. Volcanol. Geotherm. Res. 280, 90–103. doi:10.1016/j.jvolgeores.2014.05.004
Pritchard, M. E., and Simons, M. (2004). An InSAR-based survey of volcanic deformation in the Central Andes. Geochem. Geophys. Geosyst. 5, Q02002. doi:10.1029/2003GC000610
Pyle, D. (2015). “Sizes of volcanic eruptions,” in The encyclopedia of volcanoes. Editor H. Sigurdsson. 2nd ed. (Elsevier), 257–264. doi:10.1016/B978-0-12-385938-9.00013-4
Reyes-Hardy, M.-P., Aguilera, F., Sepúlveda, J. P., Esquivel, A., and Inostroza, M. (2021). GIS-based volcanic hazards, vulnerability and risks assessment of the Guallatiri Volcano, Arica y Parinacota Region, Chile. J. S. Am. Earth Sci. 109, 103262. doi:10.1016/j.jsames.2021.103262
Rodriguez-Gonzalez, A., Aulinas, M., Mossoux, S., Perez-Torrado, F. J., Fernandez-Turiel, J. L., Cabrera, M., et al. (2021). Comparison of real and simulated lava flows in the Holocene volcanism of Gran Canaria (Canary Islands, Spain) with Q-LavHA: Contribution to volcanic hazard management. Nat. Hazards (Dordr). 107 (2), 1785–1819. doi:10.1007/s11069-021-04660-6
Sandri, L., Costa, A., Selva, J., Tonini, R., Macedonio, G., Folch, A., et al. (2016). Beyond eruptive scenarios: Assessing tephra fallout hazard from Neapolitan volcanoes. Sci. Rep. 6 (1), 24271. doi:10.1038/srep24271
Sandri, L., Thouret, J.-C., Constantinescu, R., Biass, S., and Tonini, R. (2014). Long-term multi-hazard assessment for El Misti volcano (Peru). Bull. Volcanol. 76 (2), 771. doi:10.1007/s00445-013-0771-9
Sandri, L., Tierz, P., Costa, A., and Marzocchi, W. (2018). Probabilistic hazard from pyroclastic density currents in the Neapolitan area (Southern Italy). J. Geophys. Res. Solid Earth 123 (5), 3474–3500. doi:10.1002/2017JB014890
Scaini, C., Folch, A., and Navarro, M. (2012). Tephra hazard assessment at Concepción volcano, Nicaragua. J. Volcanol. Geotherm. Res. 219–220, 41–51. doi:10.1016/j.jvolgeores.2012.01.007
Schilling, S. P. (2014). Laharz_py: GIS tools for automated mapping of lahar inundation hazard zones. Open-File report No. 2014–1073. Reston, VA: U.S. Geological Survey. doi:10.3133/ofr20141073
Scollo, S., Coltelli, M., Bonadonna, C., and Del Carlo, P. (2013). Tephra hazard assessment at Mt. Etna (Italy). Nat. Hazards Earth Syst. Sci. 13 (12), 3221–3233. doi:10.5194/nhess-13-3221-2013
Sellés, D., and Gardeweg, M. (2017). Geología del área Ascotán–Cerro Inacaliri, Región de Antofagasta. Carta Geológica de Chile, Serie Geología Básica, 190. Santiago: Servicio Nacional de Geología y Minería SERNAGEOMIN.
Selva, J., Costa, A., De Natale, G., Di Vito, M. A., Isaia, R., Macedonio, G., et al. (2018). Sensitivity test and ensemble hazard assessment for tephra fallout at Campi Flegrei, Italy. J. Volcanol. Geotherm. Res. 351, 1–28. doi:10.1016/j.jvolgeores.2017.11.024
SERNAGEOMIN (2020). Ranking de riesgo específico de volcanes activos de Chile 2019. Red Nacional de Vigilancia Volcánica (RNVV). Santiago: Servicio Nacional de Geología y Minería SERNAGEOMIN.
Siebert, L., Simkin, T., and Kimberly, P. (2010). Volcanoes of the world. 3rd ed. Washington, DC: Smithsonian Institution.
Spiller, E. T., Wolpert, R. L., Ogburn, S. E., Calder, E. S., Berger, J. O., Patra, A. K., et al. (2020). Volcanic hazard assessment for an eruption hiatus, or post-eruption unrest context: Modeling continued dome collapse hazards for Soufrière Hills volcano. Front. Earth Sci. (Lausanne). 8, 535567. doi:10.3389/feart.2020.535567
Stern, C. R. (2004). Active Andean volcanism: Its geologic and tectonic setting. Rev. Geol. Chile 31 (2), 161–206. doi:10.4067/s0716-02082004000200001
Sulpizio, R. (2005). Three empirical methods for the calculation of distal volume of tephra-fall deposits. J. Volcanol. Geotherm. Res. 145 (3–4), 315–336. doi:10.1016/j.jvolgeores.2005.03.001
Tadini, A., Azzaoui, N., Roche, O., Samaniego, P., Bernard, B., Bevilacqua, A., et al. (2022). Tephra fallout probabilistic hazard maps for Cotopaxi and Guagua Pichincha volcanoes (Ecuador) with uncertainty quantification. J. Geophys. Res. Solid Earth 127, e2021JB022780. doi:10.1029/2021JB022780
Taddeucci, J., Alatorre-Ibargüengoitia, M. A, Cruz-Vázquez, O., Del Bello, E., Scarlato, P., and Ricci, T. (2017). In-flight dynamics of volcanic ballistic projectiles. Rev. Geophys. 55, 675–718. doi:10.1002/2017RG000564
Tennant, E., Jenkins, S. F., Winson, A., Widiwijayanti, C., Gunawan, H., Haerani, N., et al. (2021). Reconstructing eruptions at a data limited volcano: A case study at Gede (West Java). J. Volcanol. Geotherm. Res. 418, 107325. doi:10.1016/j.jvolgeores.2021.107325
Thompson, M. A., Lindsay, J. M., and Gaillard, J. (2015). The influence of probabilistic volcanic hazard map properties on hazard communication. J. Appl. Volcanol. 4 (1), 6. doi:10.1186/s13617-015-0023-0
Thompson, M. A., Lindsay, J. M., Leonard, G. S., Lutteroth, C., Bostrom, A., Corballis, P., et al. (2021). Volcanic hazard map visualisation affects cognition and crisis decision-making. Int. J. Disaster Risk Reduct. 55, 102102. doi:10.1016/j.ijdrr.2021.102102
Thouret, J.-C., Antoine, S., Magill, C., and Ollier, C. (2020). Lahars and debris flows: Characteristics and impacts. Earth-Science Rev. 201, 103003. doi:10.1016/j.earscirev.2019.103003
Tierz, P., Loughlin, S. C., and Calder, E. S. (2019). VOLCANS: an objective, structured and reproducible method for identifying sets of analogue volcanoes. Bull. Volcanol. 81, 76. doi:10.1007/s00445-019-1336-3
Tierz, P., Stefanescu, E. R., Sandri, L., Sulpizio, R., Valentine, G. A., Marzocchi, W., et al. (2018). Towards quantitative volcanic risk of pyroclastic density currents: Probabilistic hazard curves and maps around Somma-Vesuvius (Italy). J. Geophys. Res. Solid Earth 123, 6299. doi:10.1029/2017JB015383
Tierz, P., Woodhouse, M. J., Phillips, J. C., Sandri, L., Selva, J., Marzocchi, W., et al. (2017). A framework for probabilistic multi-hazard assessment of rain-triggered lahars using Bayesian belief networks. Front. Earth Sci. (Lausanne). 5, 73. doi:10.3389/feart.2017.00073
Tilling, R. I. (2009). Volcanism and associated hazards: The Andean perspective. Adv. Geosci. 22, 125–137. doi:10.5194/adgeo-22-125-2009
Titos, M., Martínez Montesinos, B., Barsotti, S., Sandri, L., Folch, A., Mingari, L., et al. (2022). Long-term hazard assessment of explosive eruptions at Jan Mayen (Norway) and implications for air traffic in the North Atlantic. Nat. Hazards Earth Syst. Sci. 22 (1), 139–163. doi:10.5194/nhess-22-139-2022
Vázquez, R., Bonasia, R., Folch, A., Arce, J. L., and Macías, J. L. (2019). Tephra fallout hazard assessment at Tacaná volcano (Mexico). J. S. Am. Earth Sci. 91, 253–259. doi:10.1016/j.jsames.2019.02.013
Warwick, R., Williams-Jones, G., Kelman, M., and Witter, J. (2022). A scenario-based volcanic hazard assessment for the Mount Meager volcanic complex, British Columbia. J. Appl. Volcanol. 11 (1), 5. doi:10.1186/s13617-022-00114-1
Keywords: Northern Chile, Central Andes, hazard modeling, hazard simulation, volcanic hazard assessment
Citation: Alcozer-Vargas N, Reyes-Hardy M-P, Esquivel A and Aguilera F (2022) A GIS-based multi-hazard assessment at the San Pedro volcano, Central Andes, northern Chile. Front. Earth Sci. 10:897315. doi: 10.3389/feart.2022.897315
Received: 16 March 2022; Accepted: 28 June 2022;
Published: 19 August 2022.
Edited by:
Karoly Nemeth, Massey University, New ZealandReviewed by:
Sam Poppe, Vrije University Brussel, BelgiumCopyright © 2022 Alcozer-Vargas, Reyes-Hardy, Esquivel and Aguilera. This is an open-access article distributed under the terms of the Creative Commons Attribution License (CC BY). The use, distribution or reproduction in other forums is permitted, provided the original author(s) and the copyright owner(s) are credited and that the original publication in this journal is cited, in accordance with accepted academic practice. No use, distribution or reproduction is permitted which does not comply with these terms.
*Correspondence: Nicolás Alcozer-Vargas, bmljb2xhcy5hbGNvemVyQGFsdW1ub3MudWNuLmNs
Disclaimer: All claims expressed in this article are solely those of the authors and do not necessarily represent those of their affiliated organizations, or those of the publisher, the editors and the reviewers. Any product that may be evaluated in this article or claim that may be made by its manufacturer is not guaranteed or endorsed by the publisher.
Research integrity at Frontiers
Learn more about the work of our research integrity team to safeguard the quality of each article we publish.