- 1Department of Geological Sciences, Roma Tre University, Roma, Italy
- 2C.U.G.RI., InterUniversity Research Center for Prevision and Prevention of Great Risks, University of Salerno, Fisciano University Campus, Fisciano, Italy
- 3DICIV_UNISA, Department of Civil Engineering, University of Salerno, Fisciano University Campus, Fisciano, Italy
The assessment and mitigation of landslide risk affecting hillslopes in highly urbanized and infrastructured environments are often problematic due to the inadequacy of the traditional approach based on landslide inventories and the absence of a shared language between the different scientific-technical operators (geologists, engineers, architects, environmentalists, economists, jurists) and recurrent understanding problems with policymakers, stakeholders, and property owners. Therefore, innovative technologies and working procedures are required to address these problems. In this context, the European INSPIRE Directive and the Italian national Catalog of Territorial Data with the related Geo-Topographic DB provide positive responses in terms of data standardization and transdisciplinary interoperability. On the other hand, the application of the object-oriented geomorphological mapping of landslides and, even more, the recently proposed Landslide Object-Oriented Model (LOOM) make it possible to develop a more thorough approach to assess the spatial and temporal relationships between landslides and affected slopes. Following the above perspective, the InterUniversity Research Center for Prevision and Prevention of Great Risks (C.U.G.RI.) produced the LOOM-based “eventory” of landslides over a sector of the Tyrrhenian coastal belt, northwest of Salerno city, in the framework of a multi-disciplinary investigation project launched by the Campania Regional Administration to assess the landslide risk. The quantitative assessment of the geomorphological expert-judgment procedures has been carried out exploiting morphometric indexes: the Topographic Position Index (TPI) for automatic slope features recognition, and the Slope-Area plots for surficial process domains. Furthermore, the application of the INSPIRE, and related Italian National Geo-Topographic DB standards allowed transdisciplinary interaction between scientists, technicians, and managers. Such proposal can support the risk management procedure, adding in the Value Judgement and Risk Tolerance Criteria simplicity and effective interoperability in trans-disciplinary frameworks.
1 Introduction
Since the early 1980s, quantitative methods and procedures have been increasingly applied to assessing landslide risk of single slopes within broad areas in the perspective of land planning and management (Varnes and IAEG, 1984; Whitman, 1984; Einstein, 1988; Fell, 1994; Cruden and Fell, 1997; Australian Geomechanics Society, 2000; Hartford and Baecher, 2004; Lee and Jones, 2004; Fell et al., 2005; Hungr et al., 2005; Canuti and Sassa, 2008; Corominas et al., 2014).
According to Fell et al. (2005), Fell et al. (2008), a quantitative assessment of landslide risk includes several outcomes such as 1) systematic analysis of the landslides characteristics, including their travel distance and velocity, frequency of reactivation phases, temporal-spatial distribution, vulnerability, and value of elements at risk; 2) applications to situations that are not amenable to conventional deterministic; 3) criteria used to determine the areas where the building is suitable; 4) prioritization of remedial works, and potentially setting of risk-based standards for appropriate designs; 5) regional governmental planning considering landslide risk management principles, in terms they can relate to other hazards. Figure 1 summarizes the framework for landslide risk management taken from Fell et al. (2005).
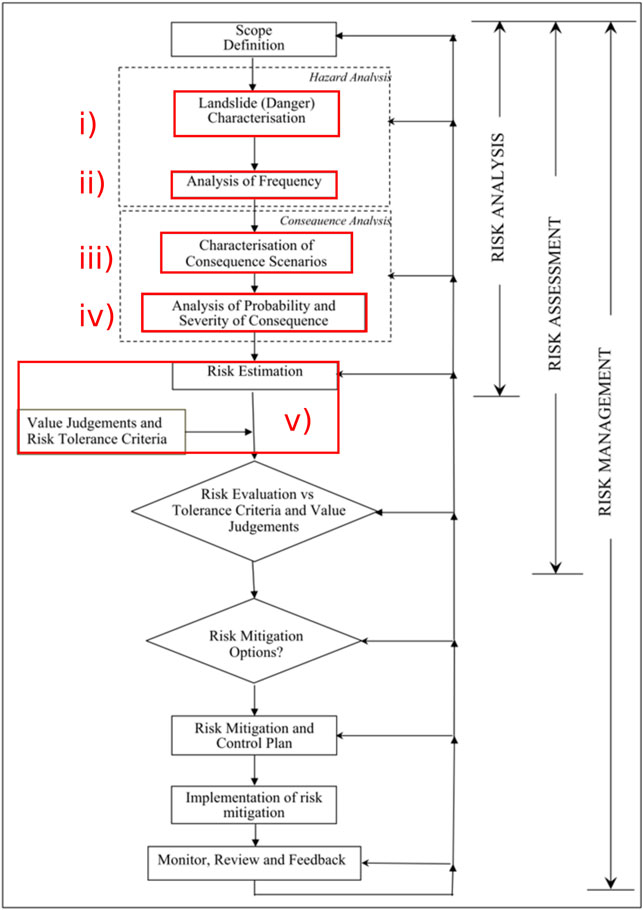
FIGURE 1. Flow chart of landslide risk management, modified from Hungr et al. (2005) and Fell et al. (2008), red boxes highlight the “weak points”.
In any case, a reliable quantitative approach to landslide risk assessment requires appropriate working procedures and technologies that may allow easier interaction among different disciplines and interoperability between different geographic information systems. In this perspective, the object-oriented inventory mapping of landslides, represented as spatial entities (objects) with a precise identity and persistence character (Egenhofer and Frank, 1987; Worboys et al., 1990; Worboys, 1994; Bian, 2007; Anders et al., 2009; Lahousse et al., 2011; Verhangen and Drăguţ, 2011; Blaschke et al., 2014; Guida et al., 2016), appears particularly suitable to develop a more exhaustive establishment of the relationships between landslides and affected slopes, as compared with traditional, symbol-based landslide inventories and maps (Campobasso et al., 2018a; Campobasso et al., 2018b).
Also, the quantitative assessment of landslide risk focuses on the liabilities and responsibilities of the involved parties, providing a reliable framework to put the uncertainties of engineering-geomorphological expert judgments into a more robust decisional system. It foresees an open and transparent cognitive process of the critical factors of landsliding and a productive discussion with public administrators, allowing systematic consideration of risk mitigation options and cost-benefit ratios, consistent with the As Low As Reasonably Practical (ALARP) principles (Melchers, 2001).
The above-cited literature declares numerous potential sources of error in the landslide hazard and risk zoning process. A few of these include the following weak points from a geomorphological perspective:
1) Limits in the landslide inventory map on which the susceptibility map and the hazard map are based,
2) Limits in the stationarity of the time series,
3) Limits in the available level of detail of the topographic, geological, and geomorphological map, of rainfall data, and other input data,
4) Uncertainties of the model, thus meaning the limits of the methods used to relate the inventory, topography, geology, geomorphology, and triggering causes in the analysis of susceptibility, hazard, and risk from landslide,
5) The inexperience of the people called to conduct zoning.
Figure 1 integrates the framework for landslide risk management taken from Fell et al. (2005), highlighting the weak points above described.
In addition, the absence of a shared language between the different scientific-technical operators (geologists, engineers, architects, environmentalists, economists, jurists) and understanding problems with policymakers, stakeholders, and property owners make the risk assessment and mitigation in highly urbanized and infrastructured environments often very problematic.
A noteworthy contribution to the interaction among different scientific, technical, and managing partners in European countries is provided by the INSPIRE (Infrastructure for Spatial Information in Europe) Directive. It concerns dictionaries and related hierarchical and multiscalar data coding (Craglia and Annoni, 2007; Masser, 2007), on which the Italian Catalog of Territorial Data Specifications for Geo-Topographic Databases are based.
The Data Specifications for Geology and Natural risk zones are reported on the INSPIRE website (TWG-GE, 2013; TWG-NZ, 2013). Concerning the Geology Theme, the Data Specifications indicate 3 Application schemas: 1) Geology; 2) Hydrogeology; and 3) Geophysics. For the basic Geomorphologic Features, Landform is defined as: “An abstract spatial object type describing the shape and nature of the Earth's land surface”. The framework for slope and gravitational features is reported in Table 1.
In order to overcome these limits, uncertainties, and unshared languages, a wide integrated multidisciplinary project for assessing and mitigating the landslide risk of hillslopes in a broad area was performed in the Campania region (southern Italy). The author’s contribution in such a project was based on large-scale object-oriented inventory mapping of landslides and advanced working procedures, including standardization (Bernstein, 2015) and exchange-interoperative aspects. The overall project was focused on the evaluation of the landslide risk affecting infrastructures along the coastal stretch between Salerno and Cava dei Tirreni. In the following sections, after an overview of the project, the application of an original object-oriented data model for landslides (LOOM, Valiante et al., 2021a) will be discussed, along with its comparison with geomorphometric techniques for landslide-related features recognition.
2 The Object-Based Landslide Inventory Mapping System
Landslides may interact with inhabited areas, infrastructures (such as roads, tunnels, bridges, viaducts, railways), land properties, or sites of cultural interest, often causing heavy damages and loss of lives (Petley, 2012). Assessing the risks associated with landslide impact on human communities requires accurate landslide inventories and large-scale maps highlighting the landslide details, the litho-technical characteristics of the affected slopes, the relationships with other surface and near-surface conditions and gravity-driven processes that may favor landslide triggering or reactivation, and the anthropogenic features that are likely to be involved.
Landslide inventories and landslide susceptibility maps aimed to assess landslide hazard and risk, at different scales, have become increasingly widespread since the past mid-century (Guzzetti et al., 1999; Corominas et al., 2014; Reichenbach et al., 2018). However, only large-scale detailed approaches can be considered reliable to assess the landslide risk in densely urbanized areas (Coltorti et al., 1986; Parise, 2003; Morelli et al., 2018).
Moreover, considering that the destructive impact of landsides is often due to complex overlaps and interaction of different type of slope movements rather than a single one (Cascini et al., 2008; Valiante et al., 2016). Considering that the standard inventory archiving and mapping formats are unable to handle this complexity, the currently used methods are generally unable for assessing landslide risk at site level.
A new object-oriented approach, LOOM (Landslide Object-Oriented Model), has been recently proposed by Valiante et al. (2021a). This model examines the spatiotemporal relationships of landslides within a functional landslide association, including their horizontal and vertical topological relationships, defined as representative of their “under-over” and “old-young” spatial and temporal arrangements. Its basic assumption is that the single objects (single landslides) are represented entirely, including overlapping parts with other objects, in such a way as to preserve their spatial relations and, indirectly, also the temporal ones. The above-below sorting is then carried out in the display phase on a temporal basis, both absolute and relative. In particular, LOOM defines a specific ontology to describe landslide associations and their space-time relationships by implementing them in a database structure capable of storing both spatial and temporal information in a single dataset, avoiding the fragmentation of the data and the related logical-topological inconsistencies (Figure 2).
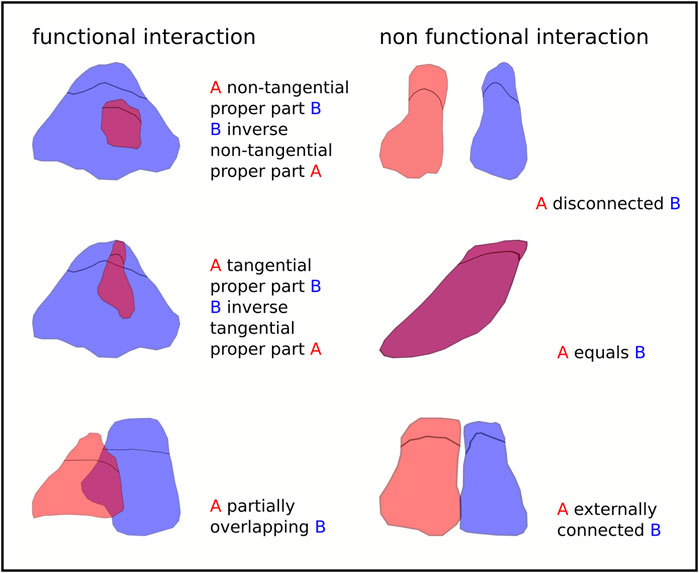
FIGURE 2. Topological relations between landslide sets reinterpreted from their non functional/functional interactions (after Valiante et al., 2020).
This structure allows to quickly derive information about the number of interacting landslides, their relative occurrence (hence the evolution of a slope), the spatial relationships among them, and those with the surrounding non-gravitational landforms or anthropogenic features.
LOOM is based on the hierarchical, object-oriented classification model (Figure 3A) introduced in Valiante et al. (2020). It partially follows Campobasso et al., 2018a, Campobasso et al., 2018b, the new Italian inter-institutional project for a full-coverage and GIS-supported geomorphological mapping guidelines, and it stands as a reference for their future developments addressed to land planning activities at national, regional, and local levels.
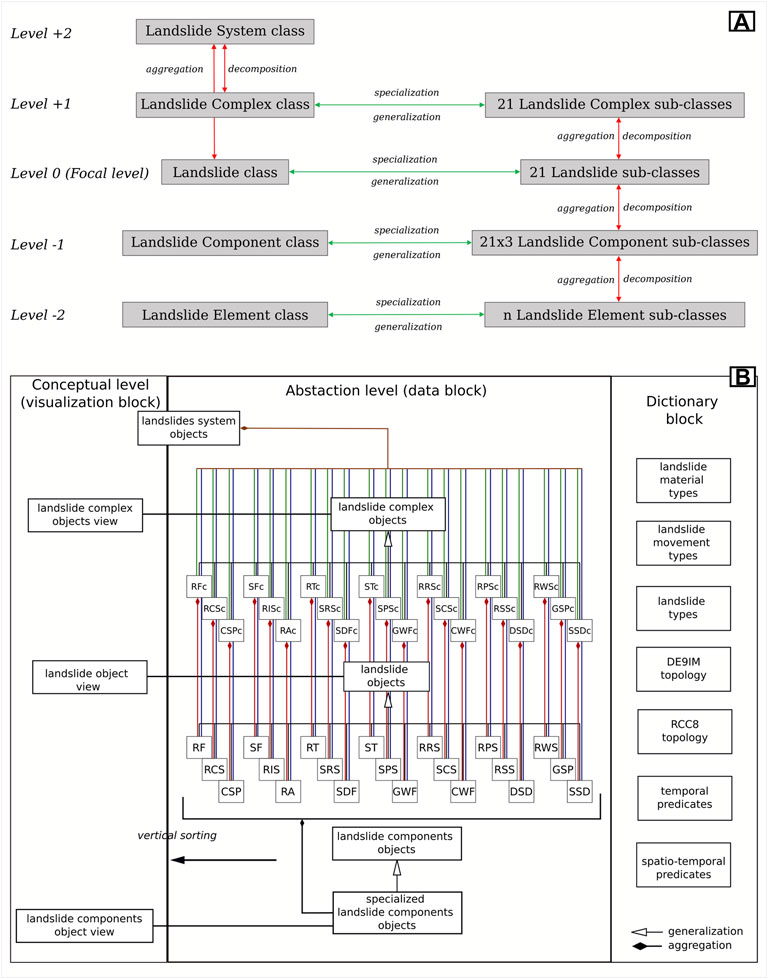
FIGURE 3. (A) Hierarchical relations among Landslide Classes, subclasses and superclasses; (B) Database main structure, each box is a table or a materialized view; table names abbreviations: RF, rock fall; SF, soil fall; RT, rock topple; ST, soil topple; RRS, rock rotational slide; RPS, rock planar slide; RWS, rock wedge slide; RCS, rock compound slide; RIS, rock irregular slide; SRS, soil rotational slide; SPS, soil planar slide; SCS, soil compound slide; RSS, rock slope spread; GSP, granular soil spread; CSP, cohesive soil spread; RA, rock avalanche; SDF, soil dry flow; GWF, granular soil wet flow; CWF, cohesive soil wet flow; DSD, deep-seated slope deformations; SSD, shallow slope deformations, “c” stands for “complex” modified from Valiante (2020).
The LOOM hierarchy defines a multi-level parent-child relationship system where each level can be composed with others into super-level or be broken down into sub-levels until no further decomposition is possible (Simon, 1962; Tsichritzis and Lochovsky, 1976; Singh et al., 1997; Odum and Barrett, 2005; Wu, 2013). Hierarchical levels depend on the purpose to which they are applied: the center of the hierarchy (focal level) can be any single landslide object of interest, parents define its generalization or aggregation levels, and children define its levels of specialization or decomposition (Wu, 1999; Wu, 2013). Two levels of aggregation describe sets of landslides: 1) landslide complexes, resulting from the aggregation of spatially connected landslides of the same type (level +1), and 2) landslide systems, defined as spatially connected sets of landslides of different types (level +2). Two other levels describe the landslide structure: 3) landslide components (level -1), and 4) landslide component elements, not further decomposable (level -2). The hierarchy’s focal level (level 0) is defined by the landslide class, comprising 21 subclasses based on the Hungr et al. (2014) landslide classification. In Table 2 LOOM levels and classes are related to proposed slope and gravitationl features sub-types within the Natural Geomorphologic Feature spatial object type.
The database management system used for LOOM is PostgreSQL © 11.0 with PostGIS © 2.5 extension (versions at compiling time). Database maintenance and manipulation are achieved using PgAdimn4 and the “pgcli” command-line tool, while QGIS 3.4 LTR © is used for data query and visualization. Such infrastructure is completely open source, and it can be easily implemented even on not so sophisticated systems favoring a wide access to the procedure (Valiante, 2020).
Based on the conceptual model described above, the database has been structured into three main blocks: a dictionary, data, and visualization. In the dictionary block, tables containing reference terminology are included. The main purpose of this data is to prevent errors during the process of data entry, therefore, to maintain data integrity. These tables are accessed through foreign keys by the tables contained in the data block and by some of the dictionary tables themselves. The terminology contained in this block is about landslides (Hungr et al., 2014) and spatio-temporal topology semantics (Allen, 1983; Egenhofer and Herring, 1990; Randell et al., 1992). The defined tables are listed in Figure 3B. The data block is structured to contain the instances of the object-oriented model. Here, a table is defined for every class declared in the model, meaning that the final result will be having 21 tables for landslide objects, 21 tables for landslide complex objects, and one table for landslide systems. Tables for landslide components and landslide component element are implemented based on the needs. Landslide complex tables are materialized views derived from the aggregation of the landslide subclasses tables where the functional interaction is satisfied. These objects do not have an exact temporal characterization as they can contain different landslides that occurred at several times, but their vertical sorting is achieved by computing the “mean event” from the aggregated landslides events so that the most active complexes are ensured to be on top. Similarly to the previous tables, the landslide systems table is a materialized view built upon the aggregation of the previous data. As for landslide complexes, systems do not have an exact temporal characterization. Moreover, considering how they are built, the vertical arrangement of landslide systems is invariant as for their definition they cannot overlap: absurdly, if two or more landslide systems overlap, the functional relation is verified; thus, they will be aggregated again into a single landslide system. In the visualization block, objects from the data block are re-arranged for the correct visualization. The vertical sorting is applied using the temporal characterization of landslides. This process only applies to components, landslides, and complexes as systems do not overlap (Valiante, 2020; Valiante et al., 2021a).
A relevant aspect of LOOM concerns its ability to describe the topological relationships between the considered landslide objects whose cartographic representation includes overlapping parts with other objects in such a way as to preserve their spatial relations and, indirectly, also the temporal ones.
Traditional geomorphological mapping represents landforms as polygons juxtaposed with each other with shared edges according to a pattern that could be defined as “tile” or “mosaic”. This procedure defines an information level that “cloaks” the area of interest without overlapping the entities belonging to the same table. Although this model may be helpful in numerous applications, such as cadastral data and administrative boundaries, it is entirely insufficient for a correct cartographic representation of geomorphological data. The mosaic of landforms creates a conceptual inconsistency between the mapped data and the reality, where landforms are often spatially superposed, functionally interacting, and structurally interconnected where topological relations are the only reliable conceptual approach.
In this context, it is indispensable a data structure capable of maintaining those topological relationships that best approximate the actual in-field situation. In particular, it is crucial to maintain the spatial superposition ratios according to a 2.5D or even 3D model.
This approach could be also extended to the relations existing between natural geomorphological features and anthorpogenic features (infrastructures, pipelines, buildings, industries, etc.). An emblematic example regards the spatial relationships between a watercourse and a bridge crossing it: in a 2D mosaic representation, there will be a spatial intersection between the watercourse and the infrastructure, with all the relative consequences in the contexts of danger and hydrogeological risk; if, on the other hand, the two objects are analyzed in a 3D scenario or in one that still takes into account the superimposition relationships, it could even result that the two objects do not come into contact at all, that is, they are disjoint from a topological point of view (Figure 4).
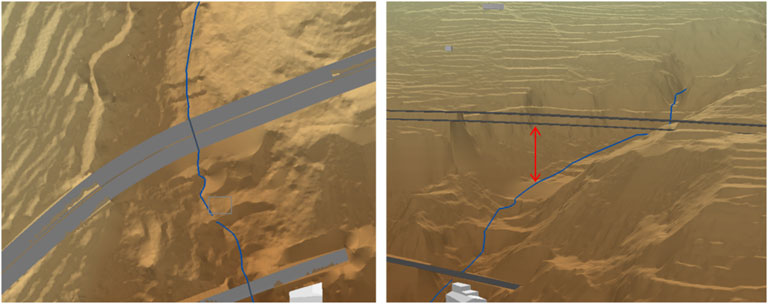
FIGURE 4. - On the left, apparent 2D intersection between the river and the bridge; on the right, the same scene in a 3D view.
3 The CIS_2020 Regional Pilot Project
On July 29th, 2019, the Campania Regional Administration, in agreement with the seven regional universities (C.U.R. - Regional University Committee), launched the multidisciplinary project entitled “Methodologies for the Punctual Assessment of Hydrogeological Risk in Densed Anthropized Areas and Tools for Regional Development Strategies”.
The project has been granted in the perspective of promoting advanced, interdisciplinary, and shared models for assessing, managing, and monitoring landslide risk in critical areas. Such critical areas are characterized by strategic infrastructures concentrated in an urban environment of high socio-economic value. The project aimed at improving the technical-administrative capacities and multi-level governance of public offices in the perspective of new standardization supports.
The selected study area is the Salerno - Cava dei Tirreni Strategic Infrastructural Corridor, a 5 km2 wide strip of the Southern Apennine Tyrrhenian borderland of highest national and regional socio-economic value, between Salerno town to the East, and Vietri Sul Mare, a very popular touristic center (“the Eastern Door” of the Amalfi Coast) to the West. Key transport infrastructures cross this area among which: a section of the A3 Naples-Salerno Highway; the Salerno-Cava dei Tirreni railway; the SS18 “Tirrena Inferiore” state road; the regional roads to the Amalfi Coast; and the connecting roads to the commercial and touristic Salerno Harbor System (Figure 5).
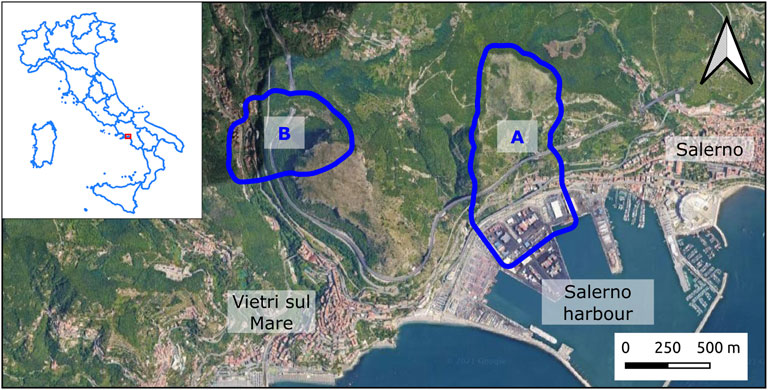
FIGURE 5. Location of the investigated area, the in-depth study areas are outlined in blue: (A) Olivieri catchment; (B) Mt. San Liberatore north-western slope (image from Google repositories).
The geological structure of the project area is characterized by a Mesozoic carbonatic sedimentary sequence belonging to the Apennine Platform Complex thrusting over the Lagonegrese-Molise Basin terrains and locally affected by NW-SE and SW-NE trending low-angle/high angle normal faults (Vitale and Ciarcia, 2013). The near-surface units include alluvial deposits consisting of gravels in a sandy matrix, cemented slope debris, and Pleistocene to recent volcano-clastic and pyroclastic deposits, erupted by the Mt. Somma-Vesuvius volcanic complex (Cascini et al., 2008).
The geomorphological features of the study area are those typical of the Amalfi Coastland, with high relief, coastal carbonate hillslopes incised by deep ravines and gullies, and large headwaters with zero-order basin complexes (Cascini et al., 2008) filled by residual pyroclastic covers. In the nearshore, coastal cliff complexes are alternating with narrow pocket beach complexes. To the Western and Eastern borders are located the coastal plain of the Bonea Torrent and Irno River, respectively. To the NW border of the study area, the cuesta-like morpho-structure of Mt. San Liberatore is the more prominent landscape in the area. It is surrounded by litho-structural cliffs (Figure 5).
Landslides are widespread and frequent: mostly rockfalls and debris avalanches from limestone escarpments and earth-mud flows from pyroclastic hillslope covers in the headwaters, have repeatedly affected the area in the past, even with catastrophic effects (Cascini et al., 2008; Fiorillo et al., 2019) (Figure 6). A critical strategic aspect is that any landslide-related interruption in the Infrastructure System could induce relevant economic damage at the national and regional levels.
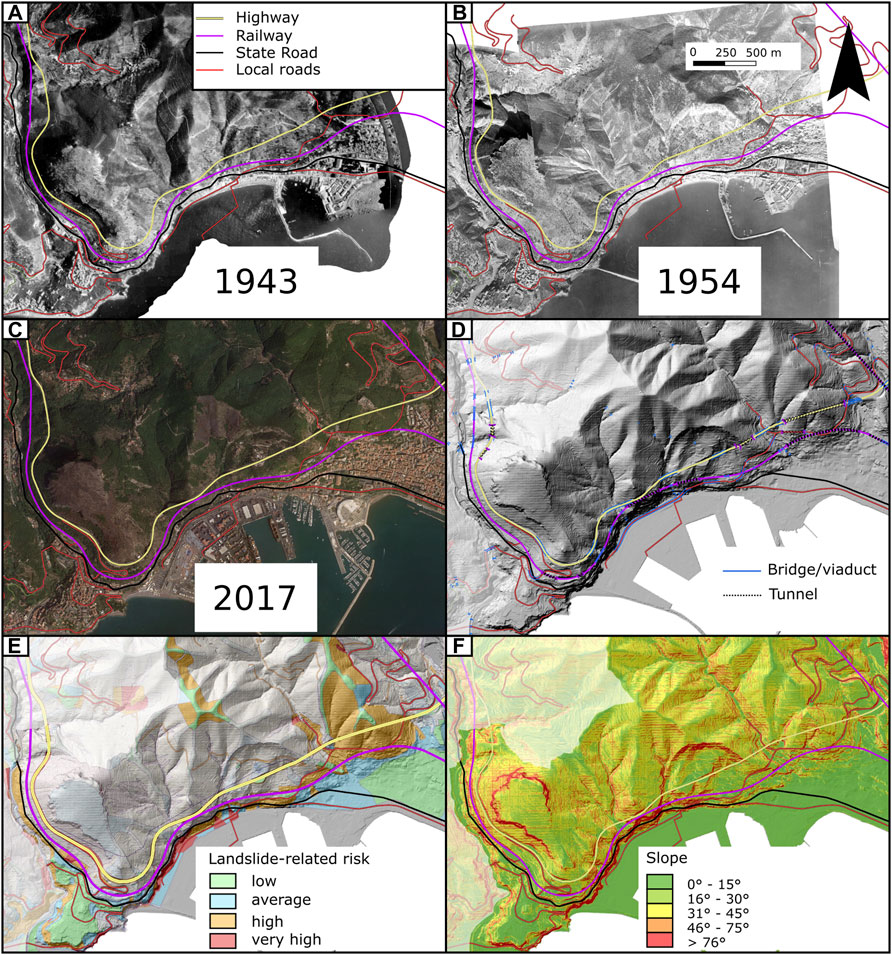
FIGURE 6. The Strategic Infrastructural Corridor Salerno-Vietri sul Mare study area (CIS2020): (A) superposition of the present-day Transport Infrastructure System on the aerial photo taken in 1943 by Royal Air Force—evident surficial scars related to 1909–1910 disaster events; (B) superposition of the same CIS2020 on the aerial photo taken by Italian Military Geographic Institute—evident channelled surficial scars and invasion areas by pyroclastic wet material related to the 1954 October 24 disaster event which strongly affected the settlements of Salerno-Amalfi coastland; (C) superposition of the present-day CIS2020 on the ortho-photo by Campania Region Archives, taken after the major wildfire event occurred during the summer 2017 in the area from decadal times—evident surficial erosion, scars and incision; (D) CIS2020 infrastructures highlighting tunnels, viaducts and bridges over LiDAR data from the National GeoData Repository; (E) superposition of the CIS2020 on currently in force Landslide Hazard and Risk of the District basin Authority “Southern Apennines”; (F) the CIS2020 on the slope map highlighting scarps, cliffs, and channelized landforms.
4 The Landslide Object-Oriented Model Application of the CIS_2020 Project
The specific project targets were improving the technical-scientific methods for quantitative assessment of landslide risk and proposing an advanced territory management system in the perspective of new scientific and interoperation supports. At this end, an updating of the available geo-environmental and infrastructure database of the investigated area was performed to overcome the weak points mentioned before.
Within the CIS_2020 Project, DICIV_UNISA and C.U.G.RI., (University of Salerno) carried out the inventory mapping of landslides over the entire project area, systematically applying the LOOM at the 1:5.000 scale with more detailed scales (1:2000) in sectors of particular interest. This activity provided the basis for the other interventions (engineering, environmental, economic, and legal) aimed at preparing guidelines for the assessment and mitigation of landslide risk and the required resilience measures to be shared with regional, provincial, and municipal offices.
The morphological features of the natural and built-up environment have been surveyed over time by comparing high-resolution DEMs (Digital Elevation Models) and remote-sensing images, including a very high-resolution satellite (Pléiades) stereo-pair covering the entire investigated area. For the sectors of major detail, ultra-high-resolution drone images and scans from Terrestrial Laser Scanner, capable of detecting the ground beneath the vegetation, were used (courtesy of LabM, DICIV_UNISA).
The spatial inventory has been constructed following Dramis et al. (2011), with further integrations contained in Valiante et al. (2021a) transforming the spatial inventory in a spatiotemporal “eventory” (sensu Valiante et al., 2021b). For the initial spatiotemporal mapping of landslides, detailed field surveys were performed, integrating the analysis of remote sensing images, given the need for lithostructural, stratigraphic, sedimentological, and hydrogeological data to avoid geomorphological convergence. After the initial expert-based mapping, data are arranged in the object-oriented data structure (LOOM). Lastly, a multiscale validation has been carried out, exploiting both grid-based techniques and other object-oriented techniques such as OBIA (Object-Based Image Analysis) (Blaschke et al., 2014; Hölbling et al., 2015) (Figure 7).
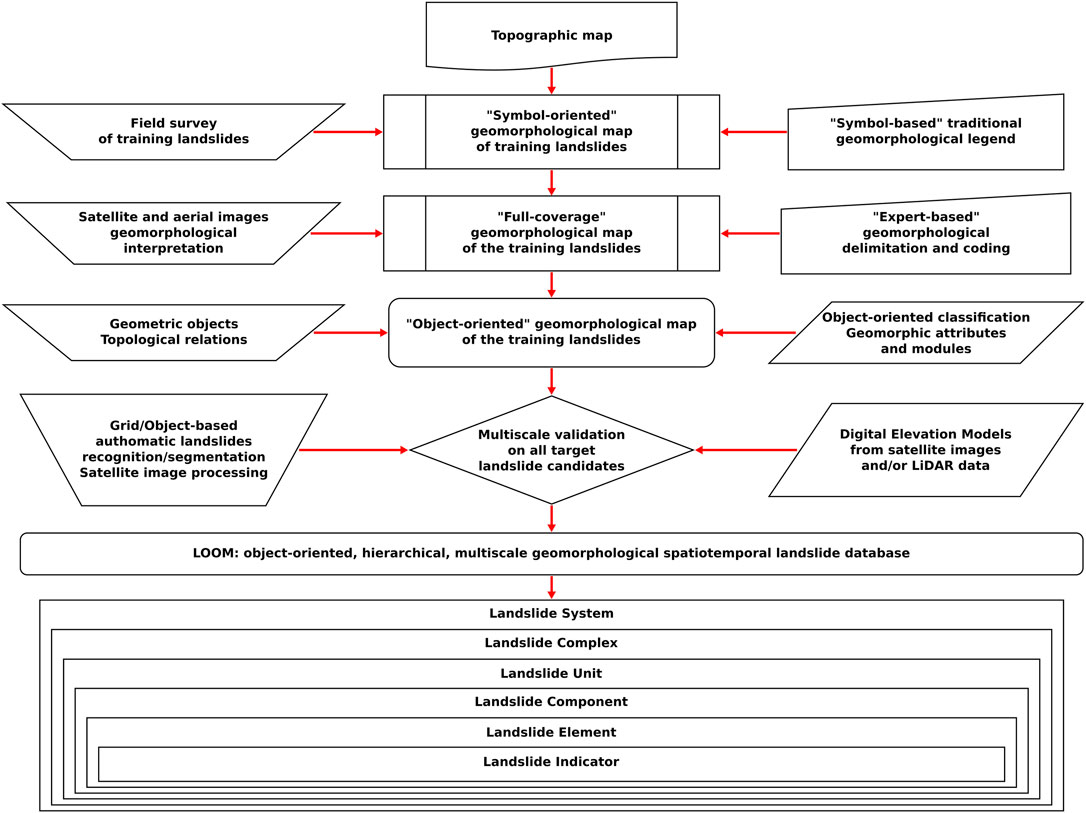
FIGURE 7. LOOM mapping procedure (modified after Dramis et al. (2011)).
Examples of the LOOM inventory mapping of landslides in two in-depth study areas, the Vallone Olivieri catchment and the Mt. San Liberatore north-western slope (box A nd box B in Figure 5 respectively), both interesting for the recurrent occurrence of landslides capable of interrupting road and railway lines, are presented below.
In the previous dataset available from the currently enforced Landslide Inventory Map (Campania Sud Regional Basin Authority, 2012; Fiorillo et al., 2019), the October 26, 1954 landslides (Fiorillo et al., 2019) were represented by drawing their outlines or grouping them into comprehensive polygons, classified as extremely rapid mudflows. Data contained in the PAI dataset was based only on the highest magnitude event in the area, that is, the flow events of 1954. Other minor events, such as small magnitude rock falls, were not taken into account mostly because 1954 flows obliterated them. Successive rock falls, and other small events were also not mapped because the scale of analysis was not supported by field surveys but was performed at the basin scale for planning purposes. In our work, instead, we conducted detailed field surveys also based on high-resolution base maps, such as sub-meter lidar data.
The first in-depth study area comprises the North-South-oriented sector of the Olivieri catchment, immediately north of the Salerno harbour. This area is widely affected by flow-like movements, such as debris flowslides and debris flows (sensu Hungr et al., 2014), which fall within the granular soil wet flow class in the LOOM data structure. Besides flows, the area has also recorded a few debris slides (sensu Hungr et al., 2014) stored as soil planar slide objects, previously classified as extremely rapid earth flows and debris avalanches (Fiorillo et al., 2019).
The LOOM data structure allowed more information from the surveyed data than was possible from the previously adopted investigation methods (Figure 8). From a multitemporal perspective, it is now possible to classify the entire landslide dataset based on every object's time or period of occurrence. From archive records and local testimonies, flows older than those that occurred during the October 25–26, 1954 event, which are predominant in the area, could be recognized and distinguished. After 1954, a few smaller flows and shallow debris slides, mainly related to wildfires that occurred in 2007 (Calcaterra et al., 2007) and during the 2017 summer (Esposito et al., 2017), have been inventoried. Moreover, the LOOM hierarchical aggregation procedure allowed handling landslides' spatial and temporal overlap.
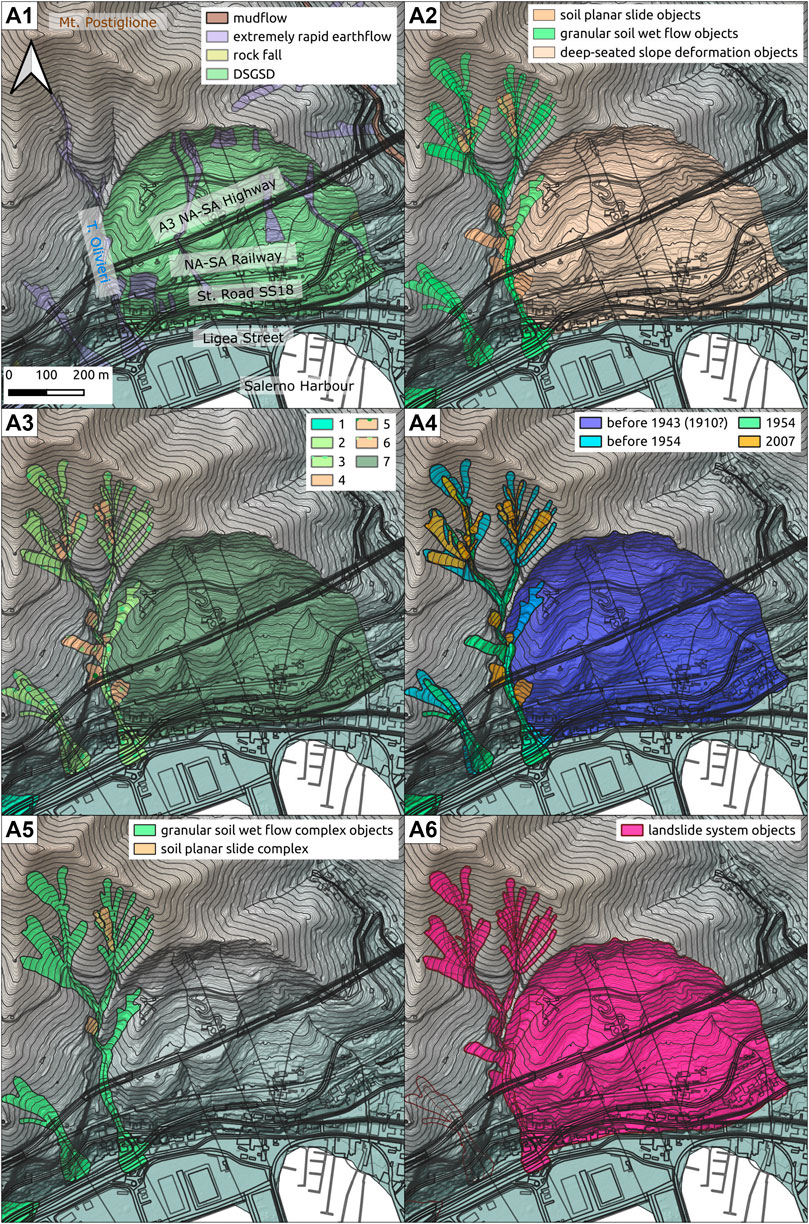
FIGURE 8. Vallone Olivieri Catchment case study: (A1) pre-existing dataset; (A2) LOOM focal classes; (A3) LOOM landslide types, 1. Debris avalanche, 2. Debris flood, 3. Debris flowslide, 4. Debris flowslide—debris avalanche, 5. Debris flowslide—debris flow, 6. Debris slide, 7. Debris slide—debris dry flow, 8. Debris slide—debris flowslide; (A4) LOOM temporal classification; (A5) LOOM +1 level; (A6) LOOM +2 level.
All the granular soil wet flow objects were aggregated into one granular soil wet flow complex object because of their functional interaction relationships (filling-discharging-refilling in Guida, 2003). For the same reason, a cluster of soil planar slide objects affecting the north-eastern area could be aggregated into a soil planar slide complex object (retrogressive and successive sliding). Other objects of the same class could not be aggregated because they were disjoint. With the second aggregation steps, the set of all the inventoried landslide objects was aggregated into a single landslide system object, which exemplifies the evolution of the basin characterized by recurrent flow-like movements along the main valley trunk, which are feed, in turn, by shallow debris slides from the valley flanks (Figure 8).
The second in-depth investigated area, Mt. San Liberatore’s north-western slope, is recurrently affected by rockfalls and debris avalanches from the upper part of the slope, characterized by calcareous rocky cliffs, and debris flows involving the pyroclastic covers (Figure 9). The previous inventory reported for this area a single landslide feature classified as “extremely rapid mudflow” from pyroclastic soils (Fiorillo et al., 2019). With the LOOM approach, these phenomena have been classified respectively as rockfall objects and granular soil wet flow objects.
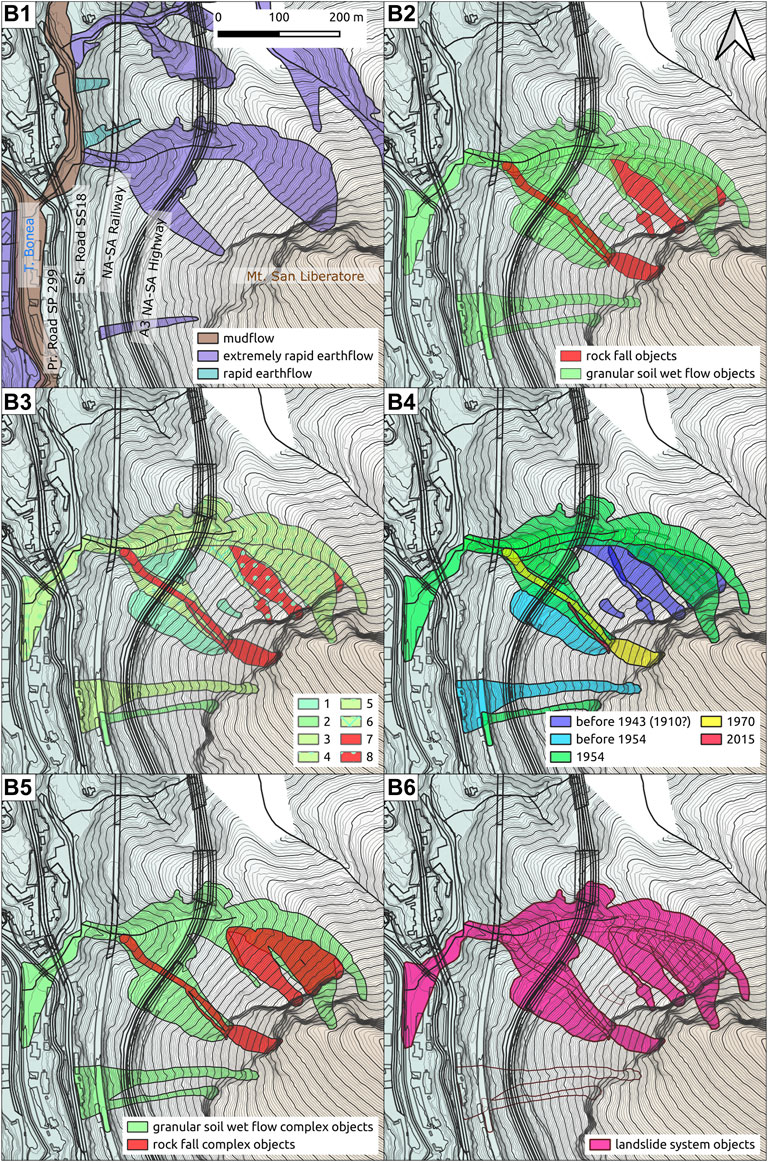
FIGURE 9. Mt. San Liberatore case study: (B1) pre-existing dataset; (B2) LOOM focal classes; (B3) LOOM landslide types, 1. Debris avalanche, 2. Debris flow, 3. Debris flowslide, 4. Debris flowslide—debris avalanche, 5. Debris flowslide—debris flow, 6. Debris flowslide – debris flow—debris flood, 7. Rock fall, 8. Rock fall—debris avalanche; (B4) LOOM temporal classification; (B5) LOOM +1 level; (B6) LOOM +2 level.
Applying the LOOM data structure, the chronology of the landslide events has been registered not only as visual overlap of entities but also as functional interaction of events, thus their recurrence. As for the Vallone Olivieri area, the most extensive flow features occurred during the October 25–26, 1954 event, preceded by a few older ones. Besides flows, the inventoried rock fall objects from local testimonies and archive data date back to 1970 (the larger one) and 2015. The first step of the aggregation procedure allowed the definition of a granular soil wet flow complex object and a rockfall complex object, highlighting the repetitiveness of the events. The second aggregation step defined a single landslide system object resulting from the spatiotemporal succession of debris flows, debris avalanches, and rockfalls (Figure 9).
5 Quantitative Assessment of Gravity-Driven Reference Hillslopes and Process Domain
In order to quantify the expert-judgment procedures morphometric indexes have been exploited. Such indexes are the Topographic Position Index (TPI) proposed by Weiss (2001), for the semi-quantitative delineation of main landforms, and the Slope-Area plots, for the estimation of the slope processes typology, including the gravity-driven ones (Vergari et al., 2019). The analyses were based on Digital Elevation Models with different resolutions for the wide area (2 m resolution) and the more detailed excerpts for the in-depth study areas (0.2 m resolution).
The Topographic Position Index (TPI) proposed by Weiss (2001), defined as the difference in elevation between a point and the average of its neighborhood, was applied to design the topographic basis on which to superimpose the surveyed geomorphological features (Figure 10). The TPI values were classified using three progressive hierarchical levels: Basic Topographic Units (UTB - Campobasso et al., 2018a; Campobasso et al., 2018b); Slope Positions (Weiss, 2001), and Relief Shapes (the landforms defined within this project). The starting point for this classification was the definition of the Slope Positions based on the classification of the TPI values (Table 3); the UTBs were derived by aggregation of the Slope Positions, and the Relief Shapes were obtained from a further decomposition of the Slope Position with the addition of their slope values, according to the scheme shown in Table 3.
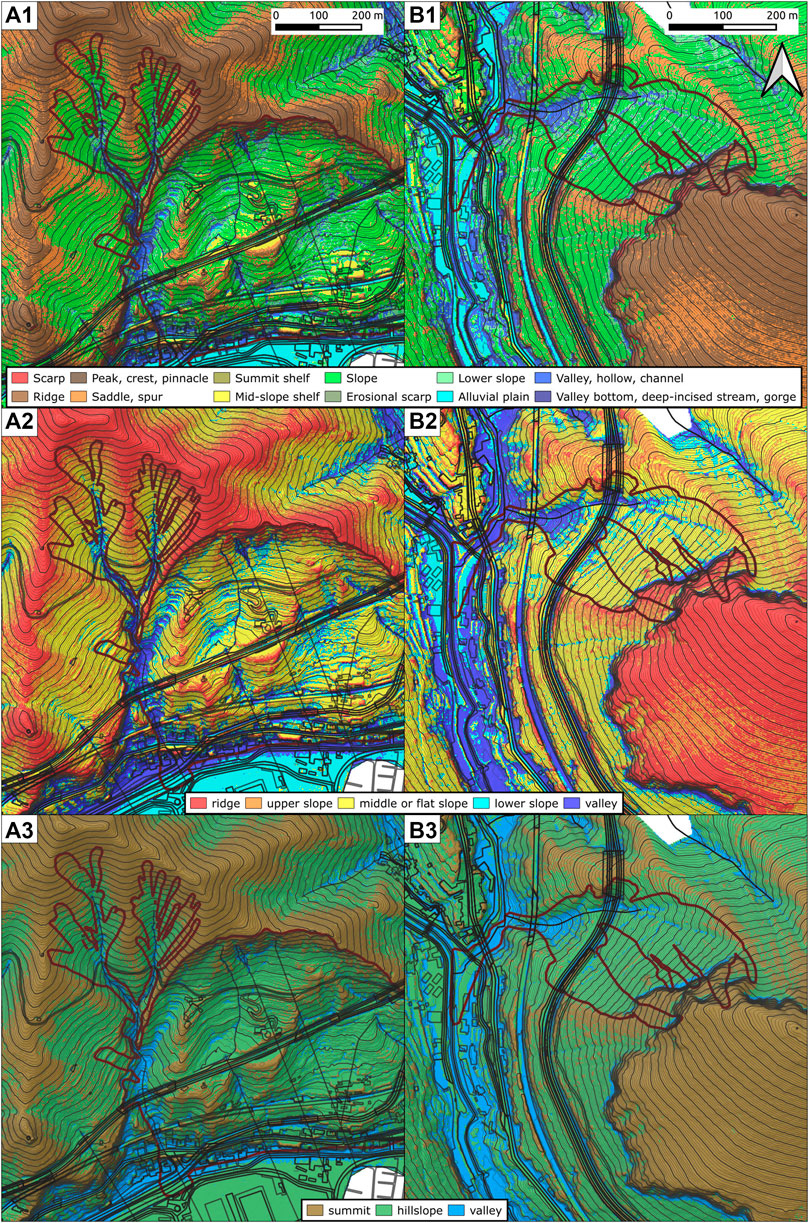
FIGURE 10. TPI elaborations for the selected case studies: (A1) landforms, (A2) slope positions, (A3) Basic Topographic Units for the Olivieri case study; (B1) landforms, (B2) slope positions, (B3) Basi Topographic Units for the Mt. San Liberatore case study. Landslide system objects are outlined in dark red.
Slope - Area Plots (Booth et al., 2013; Tseng et al., 2015) have been exploited to estimate the denudational process types acting on the slopes, including landslide phenomena. The purpose of this analysis is to detect the hypothetical landslide-related channels as a validation tool for the detected flow-like landslides.
The graphical plot of the topographic steepness versus the drainage area can be subdivided into four main regions or segments, each one representing a dominant geomorphic process: I) hillslopes; II) hillslope-to-valley transition; III) debris flow dominated channels or landslides driven channels; IV) alluvial channels. Slope and contributing area values have been plotted for each case study, and domain thresholds have been defined, analyzing the slope derivative values (Vergari et al., 2019).
Generally, the threshold for the I – II boundary has been set at the first zero value of the slope first-order derivative, corresponding to the maximum value of the Slope – Area plot; the threshold between II and III domains has been set at the next zero of the first-order derivative function which reflects an interruption in the steady decreasing trend of the II domain; the last threshold is marked by the last zero of the first-order derivative values corresponding to the transition from fluctuating values to a steady decreasing trend in the slope plot. For this study, instead, classes I and III have been further subdivided to better exploit the high resolution of the grid dataset, also analyzing the second-order derivative of the plot (Figure 11A):
Ia) Areal diffusive processes on ridges and spurs: this is the first segment of the slope-area plot characterized by an initial rising of the slope along with the drainage area up to the peak point of the plot;
Ib) Areal diffusive processes on shoulders: after the peak point of the plot, the previous trend is reverted, and the slope starts to decrease as the drainage area increases;
II) Concentrated erosional processes: the second threshold is identified at the first curvature center of the slope-area plot, corresponding at the first max point or min point of the second-order derivative of the slope-area plot;
IIIa) Transitional erosional processes: the third threshold is identified at the first inflection point of the slope-area plot, corresponding to the first zero value of the second-order derivative of the plot;
IIIb) Channeled depositional-erosional processes: the fourth threshold is identified at the first slope inversion in the slope-area plot, corresponding to a zero value of the first-order derivative of the plot;
IV) the last threshold is identified at the last segment of the plot, which is characterized by a steady decrease of the slope; it corresponds to the last inflection point of the plot.
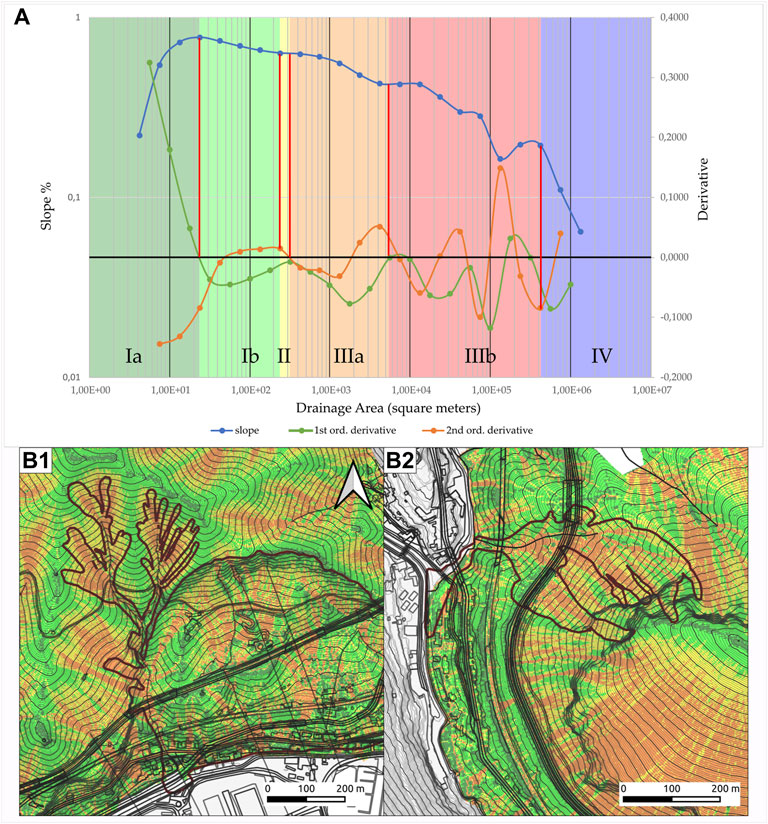
FIGURE 11. (A) Slope-Area plot for the investigated area; (B) Process Domains maps for the selected case studies: (B1) Olivieri and (B2) Mt. San Liberatore. See insert (A) for color legend, landslide system objects are outlined in dark red.
Figure 11B is the spatial expression of the thresholds identified in Figure 11A, mapped on a 2 m resolution DTM derived from LiDAR data available in the Italian National GeoData DB. From comparing LOOM data and the resulting surficial processes grid, we demonstrated not only a good visual correlation, but also a preliminary quantitative correlation between debris avalanches, debris flows and the IIIa region of the slope-area plot. For simplicity, the histogram of Figure 12 shows such correlation.
5 Discussion
With the Union Civil Protection Mechanism (UCPM) Decision No 1313/2013/EU EU, Member States and UCPM participating states are requested to report to the Commission on their disaster risk management activities to support formulating an EU risk management policy that would complete and enhance the national ones. In this framework, the new “Reporting Guidelines on Disaster Risk Management (2019/C 428/07), referring to the Art. 6(1) d of the above Decision,” is aimed to support the use of the Guidelines by relevant national authorities (Poljanšek et al., 2019).
The European Commission, using the Joint Research Centre (JRC), joins national, regional, and global efforts to acquire a better risk governance structure through 1) evidences, 2) science, and 3) knowledge management. Risk governance facilitates the policy cycle to implement integrated disaster risk management. In order to promote an effective and coherent approach to the prevention of and preparedness for disasters by sharing non-sensitive information and promoting the exchange of best practices within the Union Mechanism, Member States shall: 1) further develop risk assessments at national or appropriate sub-national level; 2) further develop the assessment of risk management capability at national or appropriate sub-national level; 3) further develop and refine disaster risk management planning at the national or appropriate sub-national level.
In addition, INFORM partners (Thow et al., 2021) believe that the availability of shared analysis of crises and disasters can lead to better coordination of actors and better outcomes for at-risk and affected people. Specifically, INFORM creates a space and a process for shared analysis to support joint strategy development, planning, and action to prevent, prepare for, respond to and recover from crises. This approach can bring together development, humanitarian and other actors to manage risk and respond better when crises occur. The report sets out by INFORM’s vision for a suite of products to support decision-making easy to use and open to everyone. This vision involves bringing scientific rigor to the process of analyzing crises and pooling expertise to develop shared methodologies. By working together, we can reduce the investments required by individual organizations, assure the quality of our analysis and make it available for the common good.
In order to pursue the EU recommendations concerning landslide risk assessment, the geomorphological working group pilot supported the project above illustrated followed two approaches: expeditious (regarding inspections and classification) at the whole territory level and punctual quantitative landslide hazard assessment focused on individual built-up structures. In particular, the project results indicate that, while the risk associated with rockfalls in the entire area is rather low apart from some limited sectors, that related to debris flows mobilizing pyroclastic deposits above the carbonate slopes is much higher and more widespread.
The common application of the European INSPIRE Directive and the Italian National Catalog of Territorial Data with the related Geo-Topographic DB allowed data transferring and transdisciplinary interoperability between universities and public authorities involving academic researchers and regional technicians and officers, responsible of Civil Protection, and technical-administrative managers of the infrastructural network.
In this perspective, such data structures should also be used for the information concerning the analysis and the evaluation of environmental hazards and risks. The LOOM data structure provides an application for landslide data. The hierarchical classification of landslide sets based on objective topological operators results in a better understanding of landslide relations and provides a standard semantic approach in storing and retrieving such information. Moreover, the very concepts of landslide complex and landslide system are proxies for other characterizations such as repetitiveness and evolutionary trend, as stated in Valiante et al. (2021b). The temporal classification, instead, adds frequency information to repetitive events. Both the hierarchical and temporal classifications aim to better define the landslide hazard, contributing to the landslide characterization and the frequency assessment, namely weak points 1) and 2) of the risk management procedure mentioned in the previous sections.
The interoperability of object-oriented data structures, such as LOOM and Geo-Topographic databases, allows the analysis of the interaction between landslides and vulnerable features, such as infrastructures, in a 2,5D or even 3D framework, surpassing a simple 2D intersection, for a better understanding of consequence scenarios, thus providing for the weak point 3) of the risk analysis procedure. Moreover, a more robust definition of the topological relations between hazardous and vulnerable entities, coupled with the concept of repetitiveness contained in landslide complex definition, and the “domino effect” concept proper of the landslide system construct, can also help in the analysis of probability and severity of consequences (weak point iv).
The analysis of the Slope-Area plot provides a correlation between process domains and landslide features, at least regarding flow-like movements, being the majority of the inventoried phenomena, thus the most representative. As shown in the maps of Figure 11B and the histogram of Figure 12, about 75% of the landslide area falls within the erosional process domains. In detail, the average value of 30% is represented by concentrated erosional processes, and the average value of 45% is represented by transitional erosional processes. Looking at how these domains are distributed within landslide objects, areas characterized by the process domain II could be related to detachment areas, which are contained mostly in the Hillslope UTB but also in the Summit UTB as defined through the TPI analysis; while areas characterized by the process domain IIIa could be related with transit zones or flow channels, which are contained both in the Hillslope and the Valley UTBs.
6 Conclusion
In this paper, the authors develop a proposal to better manage the key issues concerning the following questions posed by Reporting Guidelines on Disaster Risk Management (Poljanšek et al., 2019), addressing new insights on weak points on landslide risk assessments, landslide risk management capability, and priority prevention and preparedness measures:
• advancing shared languages for landslide risk assessment process, using the GeoUML international standards and the LOOM semantics;
• landslide mapping using object-oriented EU INSPIRE interoperable data management, starting from a landslide inventory built with the LOOM data structure;
• communicating risk assessment results using trans-disciplinary consultations with relevant authorities and stakeholders, using the Italian National Data Structure of Geo-Topographic DataBases coherent with EU-INSPIRE;
• all the previous points favor landslide risk information and communication through public events to raise public awareness.
The application of the object-oriented and quantitative geomorphological mapping of landslides and related main landforms demonstrated that the approach to assessing the spatial and temporal relationships within landslides and affected slopes is very effective. This is true in the framework of interdisciplinary academic support to increase the governance capacity of institutions dedicated to disaster management and in the trans-disciplinary perspective of increasing public awareness and involvement of the non-academic institutions in strategic areas. Such areas are characterized by a high landslide hazard connected with strategic and spatially superposed infrastructures within the same narrow hillslope system (Strategic Infrastructure Corridor) at national and community level.
In conclusion, based on the discussed results, the combined analysis of TPI-derived features, process domains, and LOOM-based “eventories” could be an alternative method for assessing the landslide hazard, at least in similar landscapes. Such proposal is coherent with weak point v) of the risk management procedure, inserting in the Value Judgement and Risk Tolerance Criteria simplicity and effective interoperability in trans-disciplinary frameworks.
Data Availability Statement
The raw data supporting the conclusions of this article will be made available by the authors, without undue reservation.
Author Contributions
All the Authors contributed to the conceptualization and the drafting of the manuscript in equal proportion.
Conflict of Interest
The remaining authors declare that the research was conducted in the absence of any commercial or financial relationships that could be construed as a potential conflict of interest.
The reviewer PDV declared a shared consortium with one of the authors DG at time of review.
Publisher’s Note
All claims expressed in this article are solely those of the authors and do not necessarily represent those of their affiliated organizations, or those of the publisher, the editors and the reviewers. Any product that may be evaluated in this article, or claim that may be made by its manufacturer, is not guaranteed or endorsed by the publisher.
Acknowledgments
Authors wish to thank Campania Region for the financial support and the C.U.G.RI. and Department of Civil Engineering of the University of Salerno for logistic and scientific support. Thanks to Prof. Margherita Fiani and Prof. Salvatore Barba (LabM, Department of Civil Engineering, Salerno University) for providing Digital Elevation data used in this work. This research has been conducted along with the activities of the MIUR PON R&I 2014-2020 Program (project MITIGO, ARS01_00964).
References
Allen, J. F. (1983). Maintaining Knowledge about Temporal Intervals. Commun. ACM 26, 832–843. doi:10.1145/182.358434
Anders, N. S., Seijmonsbergen, A. C., and Bouten, W. (2009). “Multi-scale and Object-Oriented Image Analysis of High-Res LiDAR Data for Geomorphological Mapping in Alpine Mountains,” in Proceedings of Geomorphometry (Zurich, Switzerland: Department of Geography, University of Zurich, CH), Vol. 31, 61–63.
Australian Geomechanics Society (2000). Landslide Risk Management Concepts and Guidelines. AGS Subcommittee Landslide Risk Manag. Aust. Geomechanics 35 (1), 1–44.
Bernstein, J. H. (2015). Transdisciplinarity: a Review of its Origins, Development, and Current Issues. J. Res. Pract. 11 (1), 1–20.
Bian, L. (2007). Object-oriented Representation of Environmental Phenomena: Is Everything Best Represented as an Object. Ann. Assoc. Am. Geogr. 97 (2), 267–281. doi:10.1111/j.1467-8306.2007.00535.x
Blaschke, T., Feizizadeh, B., and Holbling, D. (2014). Object-based Image Analysis and Digital Terrain Analysis for Locating Landslides in the Urmia Lake basin, Iran. IEEE J. Sel. Top. Appl. Earth Observations Remote Sensing 7, 4806–4817. doi:10.1109/jstars.2014.2350036
Booth, A. M., Roering, J. J., and Rempel, A. W. (2013). Topographic Signatures and a General Transport Law for Deep-Seated Landslides in a Landscape Evolution Model. J. Geophys. Res. Earth Surf. 118 (2), 603–624. doi:10.1002/jgrf.20051
Calcaterra, D., Parise, M., Strumia, S., and Mazzella, E. (2007). “Relations between Fire, Vegetation and Landslides in the Heavily Populated Metropolitan Area of Naples, Italy,” in Proceedings 1st North American Landslides Conference, June 3-8, 2007 (Denver, Colorado: AEG Special Publication), 1448–1461.
Campania Sud Regional Basin Authority (2012). Landslide Risk Plan (PAI). Naples, Italy: Campania Sud Regional Basin Authority and Sele Inter-Regional Basin Authority.
Campobasso, C., Carton, A., Chelli, A., D’Orefice, M., Dramis, F., Graciotti, R., et al. (2018b). “Aggiornamento e integrazioni delle linee guida della Carta Geomorfologica d’Italia alla scala 1:50.000,” in Quaderni Istituto Superiore per La Protezione e La Ricerca Ambientale Ser., 13 (1), 95. https://www.isprambiente.gov.it/it/pubblicazioni/periodici-tecnici/i-quaderni-serie-iii-del-sgi/quad13settembre2020.pdf.
Campobasso, C., Carton, A., Chelli, A., D’Orefice, M., Dramis, F., Graciotti, R., et al. (2018a). Revisione e aggiornamento delle "Linee Guida al Rilevamento della Carta Geomorfologica d'Italia alla scala 1:50.000" e proposta di un modello di cartografia geomorfologica "a oggetti. Geologia Tecnica & Ambientale 2018, 15–27.
Canuti, P., and Sassa, K. (2008). Landslides - Disaster Risk Reduction. Berlin, Heidelberg: Springer.
Cascini, L., Cuomo, S., and Guida, D. (2008). Typical Source Areas of May 1998 Flow-like Mass Movements in the Campania Region, Southern Italy. Eng. Geology. 96, 107–125. doi:10.1016/j.enggeo.2007.10.003
Coltorti, M., Dramis, F., Gentili, B., Pambianchi, G., and Sorriso-Valvo, M. (1986). Aspetti geomorfologici della frana di Ancona. Studi Geologici Camerti Speciale, 29–39.
Corominas, J., van Westen, C., Frattini, P., Cascini, L., Malet, J. P., Fotopoulou, S., et al. (2014). Recommendations for the Quantitative Analysis of Landslide Risk. Bull. Eng. Geol. Environ. 73, 209–263. doi:10.1007/s10064-013-0538-8
Craglia, M., and Annoni, A. (2007). “INSPIRE: An Innovative Approach to the Development of Spatial Data Infrastructures in Europe,” in Research and Theory in Advancing Spatial Data, Infrastructure Concepts. Editor A. Onsrud (Redlands, CA: ESRI Press), 93–105.
Dramis, F., Guida, D., and Cestari, A. (2011). “Nature and Aims of Geomorphological Mapping,” in Geomorphological Mapping: Methods and Applications. Editors M. J. Smith, P. Paron, and J. S. Griffiths (Oxford: Elsevier), 39–73. doi:10.1016/b978-0-444-53446-0.00003-3
Egenhofer, M. J., and Frank, A. U. (1987). “Object-oriented Databases: Database Requirements for GIS,” in The Research Agenda. Proceedings International Geographic Information Systems (IGIS) Symposium, 15-18 November 1987 (Arlington, Virginia: U.S. Government Research Office), 189–211.
Egenhofer, M. J., and Herring, J. R. (1990). “A Mathematical Framework for the Definition of Topological Relationship,” in Proceedings of Fourth International Symposium on Spatial Data Handling, Zurich, Switzerland. Editors K. Brassel, and H. Kishimoto (International Geographical Union IGU, Commission on Geographic Information Systems, Department of Geography, The Ohio State University), 803–813.
Einstein, H. H. (1988). “Landslide Risk Assessment Procedure. Keynote Lecture,” in Proceedings of the Fifth International Symposium on Landslides, Lausanne, Switzerland, 10-15 July 1988. Editor C. Bonnard (Rotterdam: Balkema), 1075–1090.2
Esposito, G., Parodi, A., Lagasio, M., Masi, R., Nanni, G., Russo, F., et al. (2017). Characterizing Consecutive Flooding Events after the 2017 Mt. Salto Wildfires (Southern Italy): hazard and Emergency Management Implications. Water 11 (12), 2663. doi:10.3390/w11122663
Fell, R., Corominas, J., Bonnard, C., Cascini, L., Leroi, E., and Savage, W. Z. (2008). Guidelines for Landslide Susceptibility, hazard and Risk Zoning for Land Use Planning. Eng. Geology. 102, 85–98. doi:10.1016/j.enggeo.2008.03.022
Fell, R., Ho, K. K. S., Lacasse, S., and Leroi, E. (2005). “A Framework for Landslide Risk Assessment and Management,” in Landslide Risk Management. Editors O. Hungr, R. Fell, R. Couture, and E. Eberhardt (London: Taylor & Francis), 3–26.
Fell, R. (1994). Landslide Risk Assessment and Acceptable Risk. Can. Geotech. J. 31, 261–272. doi:10.1139/t94-031
Fiorillo, F., Guerriero, L., Capobianco, L., Pagnozzi, M., Revellino, P., Russo, F., et al. (2019). Inventory of Vietri-Maiori Landslides Induced by the Storm of October 1954 (Southern Italy). J. Maps 15 (2), 530–537. doi:10.1080/17445647.2019.1626777
Guida, D., Cuomo, A., and Palmieri, V. (2016). Using Object-Based Geomorphometry for Hydro-Geomorphological Analysis in a Mediterranean Research Catchment. Hydrol. Earth Syst. Sci. 20, 3493–3509. doi:10.5194/hess-20-3493-2016
Guida, D. (2003). “The Role of the Zero-Order basin in Flowslide-Debris Flow Occurrence and Recurrence in Campania (Italy),” in Proceeding of the International Conference on Fast Slope Movements - Prediction and Prevention for Risk Mitigation, 11-13 May 2003. Editor L. Picarelli (Italy: Sorrento and Naples).
Guzzetti, F., Carrara, A., Cardinali, M., and Reichenbach, P. (1999). Landslide hazard Evaluation: a Review of Current Techniques and Their Application in a Multi-Scale Study, Central Italy. Geomorphology 31, 181–216. doi:10.1016/s0169-555x(99)00078-1
Hartford, D. N. D., and Baecher, G. B. (2004). Risk and Uncertainty in Dam Safety. London: Thomas Telford Ltd.
Hölbling, D., Friedl, B., and Eisank, C. (2015). An Object-Based Approach for Semi-automated Landslide Change Detection and Attribution of Changes to Landslide Classes in Northern Taiwan. Earth Sci. Inf. 8, 327–335. doi:10.1007/s12145-015-0217-3
Hungr, O., Leroueil, S., and Picarelli, L. (2014). The Varnes Classification of Landslide Types, an Update. Landslides 11, 167–194. doi:10.1007/s10346-013-0436-y
Lahousse, T., Chang, K. T., and Lin, Y. H. (2011). Landslide Mapping with Multi-Scale Object-Based Image Analysis - a Case Study in the Baichi Watershed, Taiwan. Nat. Hazards Earth Syst. Sci. 11, 2715–2726. doi:10.5194/nhess-11-2715-2011
Melchers, R. E. (2001). On the ALARP Approach to Risk Management. Reliability Eng. Syst. Saf. 71, 201–208. doi:10.1016/s0951-8320(00)00096-x
Morelli, S., Pazzi, V., Frodella, W., and Fanti, R. (2018). Kinematic Reconstruction of a Deep-Seated Gravitational Slope Deformation by Geomorphic Analyses. Geosciences 8, 26. doi:10.3390/geosciences8010026
Odum, E. P., and Barrett, G. W. (2005). Fundamentals of Ecology. fifth ed. Pacific Grove Ca: Thomson, Brooks/Cole.
O. Hungr, R. Fell, R. Couture, and E. Eberhardt (Editors) (2005). Landslide Risk Management (London: CRC Press), 786.
Parise, M. (2003). Observation of Surface Features on an Active Landslide, and Implications for Understanding its History of Movement. Nat. Hazards Earth Syst. Sci. 3, 569–580. doi:10.5194/nhess-3-569-2003
Petley, D. (2012). Global Patterns of Loss of Life from Landslides. Geology 40 (10), 927–930. doi:10.1130/g33217.1
Poljanšek, K., Casajus Valles, A., Marin Ferrer, M., De Jager, A., Dottori, F., Galbusera, L., et al. (2019). Recommendations for National Risk Assessment for Disaster Risk Management in EU, EUR 29557 EN. Luxembourg: Publications Office of the European Union, JRC114650. ISBN 978-92-79-98366-5. doi:10.2760/084707
Randell, D. A., Cui, Z., and Cohn, A. G. (1992). “A Spatial Logic based on Regions and Connection,” in 3rd International Conference on Knowledge Representation and Reasoning. Editors B. Nebel, C. Rich, and W. R. Swartout (Morgan Kaufmann Publisher Inc.), 165–176.
Reichenbach, P., Rossi, M., Malamud, B. D., Mihir, M., and Guzzetti, F. (2018). A Review of Statistically-Based Landslide Susceptibility Models. Earth-Science Rev. 180, 60–91. doi:10.1016/j.earscirev.2018.03.001
Singh, L., Scheuermann, P., and Chen, B. (1997). “Generating Association Rules from Semi-structured Documents Using an Extended Concept Hierarchy,” in Proceedings of the 6th International Conference on Information and Knowledge Management, Las Vegas, NV, November 10–14, 1997 (ACM Press), 193–200. doi:10.1145/266714.266895
Thow, A., Vernaccini, L., Nika, A., Poljansek, K., Galimberti, L., and Dalla Valle, D. (2021). INFORM REPORT 2021; Shared Evidence for Managing Crises and Disasters. Luxembourg: Publications Office of the European Union, JRC125620. ISBN 978-92-76-39355-9 (online).
Tseng, C.-M., Lin, C.-W., Dalla Fontana, G., and Tarolli, P. (2015). The Topographic Signature of a Major Typhoon. Earth Surf. Process. Landforms 40 (8), 1129–1136. doi:10.1002/esp.3708
Tsichritzis, D. C., and Lochovsky, F. H. (1976). Hierarchical Data-Base Management: a Survey. ACM Comput. Surv. 8, 105–123. doi:10.1145/356662.356667
TWG-GE (INSPIRE Thematic Working Group on Geology) (2013). Data Specification on Geology – Technical Guidelines. Available at: https://inspire.ec.europa.eu/id/document/tg/ge (Accessed on 01 15, 2022).
TWG-NZ (INSPIRE Thematic Working Group on Natural risk zones) (2013). Data Specifications on Natural Risk Zones – Technical Guidelines. Available at: https://inspire.ec.europa.eu/id/document/tg/nz (Accessed on 01 15, 2022).
Valiante, M., Bozzano, F., and Guida, D. (2016). The Sant'Andrea-Molinello Landslide System (Mt. Pruno, Roscigno, Italy). Rol 41, 214–217. doi:10.3301/rol.2016.132
Valiante, M., Bozzano, F., Della Seta, M., and Guida, D. (2020). “A hierarchical model for the Rocca di Sciara northeastern slope instabilities (Sicily, Italy),” in Applied Geology - Approaches to Future Resource Management. Editors M. De Maio, and T. A. Kumar (New York: Springer International Publishing), 121–144. doi:10.1007/978-3-030-43953-8_8
Valiante, M., Guida, D., Della Seta, M., and Bozzano, F. (2021a). A Spatiotemporal Object-Oriented Data Model for Landslides (LOOM). Landslides 18, 1231–1244. doi:10.1007/s10346-020-01591-4
Valiante, M., Guida, D., Della Seta, M., and Bozzano, F. (2021b). Spatiotemporal data management of recurrent debris flow events using object-oriented data modelling. Italian J Eng Geol Environ, 239–248. doi:10.4408/IJEGE.2021-01.S-22
Valiante, M. (2020). Integration of Object-Oriented Modelling and Geomorphometric Methodologies for the Analysis of Landslide Systems (Rome, Italy: Sapienza University of Rome). PhD Thesis. Available at: https://iris.uniroma1.it/handle/11573/1361628#.YeMLHuiZNhE (Accessed 01 15, 2022).
Varnes, D. J.IAEG (1984). Landslide Hazard Zonation: A Review of Principles and Practice. Paris: The UNESCO Press.
Vergari, F., Troiani, F., Faulkner, H., Del Monte, M., Della Seta, M., Ciccacci, S., et al. (2019). The Use of the Slope-Area Function to Analyse Process Domains in Complex Badland Landscapes. Earth Surf. Process. Landforms 44, 273–286. doi:10.1002/esp.4496
Verhagen, P., and Drăguţ, L. (2011). Object-based Landform Delineation and Classification from DEMs for Archaeological Predictive Mapping. J. Archaeological Sci. 39 (3), 698–703. doi:10.1016/j.jas.2011.11.001
Vitale, S., and Ciarcia, S. (2013). Tectono-stratigraphic and Kinematic Evolution of the Southern Apennines/Calabria-Peloritani Terrane System (Italy). Tectonophysics 583, 164–182. doi:10.1016/j.tecto.2012.11.004
Weiss, A. (2001). Topographic Position and Landforms Analysis, 200. San Diego, CA: Poster presentation, ESRI user Conference. http://www.jennessent.com/downloads/tpi-postertnc_18x22.pdf.
Whitman, R. V. (1984). Evaluating Calculated Risk in Geotechnical Engineering. J. Geot. Eng. ASCE 110 (2), 145–188. doi:10.1061/(asce)0733-9410(1984)110:2(143)
Worboys, M. F., Hearnshaw, H. M., and Maguire, D. J. (1990). Object-oriented Data Modelling for Spatial Databases. Int. J. geographical Inf. Syst. 4, 369–383. doi:10.1080/02693799008941553
Worboys, M. F. (1994). Object-oriented Approaches to Geo-Referenced Information. Int. J. geographical Inf. Syst. 8, 385–399. doi:10.1080/02693799408902008
Wu, J. (1999). Hierarchy and Scaling: Extrapolating Information along a Scaling Ladder. Can. J. Remote Sensing 25, 367–380. doi:10.1080/07038992.1999.10874736
Keywords: inventory map, object-oriented mapping model, transdisciplinary interoperability, european inspire directive, geo-topographic DB, landslide hazard assessment, eventory, loom
Citation: Dramis F, Guida D and Valiante M (2022) Object-Oriented Mapping as a Tool for the Assessment of Landslide Hazard in Higly Urbanized Areas. Front. Earth Sci. 10:834078. doi: 10.3389/feart.2022.834078
Received: 13 December 2021; Accepted: 25 January 2022;
Published: 24 February 2022.
Edited by:
Irasema Alcántara-Ayala, National Autonomous University of Mexico, MexicoReviewed by:
Dario Gioia, Istituto di Scienze del Patrimonio Culturale (CNR), ItalyPantaleone De Vita, Environmental and Resources Sciences—University of Naples Federico Ii, Italy
José Luís Zêzere, University of Lisbon, Portugal
Copyright © 2022 Dramis, Guida and Valiante. This is an open-access article distributed under the terms of the Creative Commons Attribution License (CC BY). The use, distribution or reproduction in other forums is permitted, provided the original author(s) and the copyright owner(s) are credited and that the original publication in this journal is cited, in accordance with accepted academic practice. No use, distribution or reproduction is permitted which does not comply with these terms.
*Correspondence: Francesco Dramis, ZnJhbmNlc2NvLmRyYW1pc0B1bmlyb21hMy5pdA==