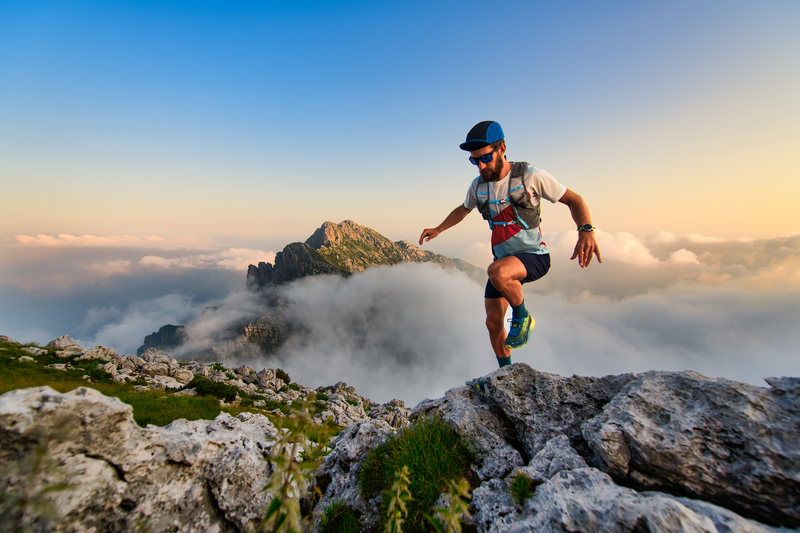
94% of researchers rate our articles as excellent or good
Learn more about the work of our research integrity team to safeguard the quality of each article we publish.
Find out more
EDITORIAL article
Front. Earth Sci. , 06 January 2023
Sec. Solid Earth Geophysics
Volume 10 - 2022 | https://doi.org/10.3389/feart.2022.1126105
This article is part of the Research Topic Advances and Applications of Distributed Optical Fiber Sensing (DOFS) in Multi-scales Geoscience Problems View all 13 articles
Editorial on the Research Topic
Advances and applications of distributed optical fiber sensing (DOFS) in multi-scales geoscience problems
Distributed optical fiber sensing (DOFS) has drawn a lot of attention in the geoscience community, taking advantage of easy-to-deploy, dense-spacing, and multi-physical measurements (temperature and strain), In contrast with traditional sensors, the optical fiber itself is used as both a sensing element and a means of data transfer. Sensing fibers can be easily deployed in harsh environments such as high-pressure and high-temperature downhole settings.
Previous studies have demonstrated the capability of DOFS in multi-scale geoscience tasks related to resource exploration and environmental hazard monitoring. While DOFS shows promise to enhance our capabilities of tackling geoscience problems, there are still challenges and problems associated with its development and applications. This Research Topic reviews many of the recent advances and applications of DOFS and shows how the technology can be improved. We hope this Research Topic may motivate more DOFS developments regarding solving geoscience problems.
a) Distributed acoustic sensing (DAS) demonstrates the robustness of subsurface imaging across different scales. Shao et al. utilize DAS to record traffic-generated seismic data in urban cities. The authors obtain the near-surface shear-wave velocity profile below the DAS cable through surface wave dispersion inversion using a Bayesian Markov Chain Monte Carlo method, which demonstrates the effectiveness of using DAS-recorded urban traffic noise for near-surface characterization. Song et al. investigate the performance of the three-station interferometry method for improving ambient-noise cross-correlation functions of a linear DAS array. The authors enhance the surface-wave signals while suppressing the non-casual signals retrieved from the data recorded by an urban internet cable, and provide high-resolution images of shallow structures in the built-up areas. Yang et al. present a case study of two survey lines with armored optical fiber cables in the Yigong Lake area of the Tibetan Plateau. Ambient noise interferometry and multichannel analysis of surface waves are performed to obtain the 2D shear-wave velocity profiles below the installed fibers. The results validate the robustness of temporary DAS monitoring in areas with extreme environments.
b) Machine learning proves its effectiveness for large-scale DAS data processing. Cong et al. propose an attention-guided denoising convolutional neural network (ADNet) to denoise seismic images and retrieve weak signals. The network adapts different kinds of convolution layers to enlarge the receptive field size and extract the global feature of the input signal. Meanwhile, the attention mechanism is introduced to extract the hidden noise from the complex background. Wang et al. propose a multi-scale interactive convolutional neural network (MSI-Net) to denoise DAS data. The proposed MSI-Net incorporates both coarse-scale and fine-scale features to advance inherent serial convolution to multi-scale parallel convolution. As demonstrated by field examples, the MSI-Net can effectively attenuate a variety of unique DAS background noise and also effectively recover weak signals. Dong et al. develop a novel multi-scale dense-connection denoising network (MDD-Net) to achieve high-accuracy processing of complex DAS-VSP background noise. MDD-Net utilizes a widened convolution block to capture the multi-scale features of the analyzed data. Both synthetic and field DAS-VSP records are processed to verify the effectiveness of MDD-Net, even for the weak upgoing signals. Tang et al. establish a rank reduction U-net (RRU-net), an integration of an encoding structure for down-sampling and a decoding structure for up-sampling, to reconstruct and denoise the DAS-VSP seismic data simultaneously. Zheng et al. propose a deep-learning approach for DAS signal extraction from the borehole-flow noise during hydraulic fracturing. The authors utilize a combination of Convolutional Neural Networks (CNNs) and Bidirectional Long Short-Term Memory Networks (BiLSTM) to extract the spatial and temporal features from the DAS data. The field data results prove its robustness for intelligent monitoring and real-time evaluation for hydraulic fracturing. Ye et al. develop a deep-learning method based on the YOLOv5 object-detection algorithm to estimate traffic flow and vehicle speed from the DAS signals acquired via a 500 m fiber segment in suburban Beijing. The authors evaluate the temporal traffic trend of the road section and explore vehicle classification via the fast-beamforming technique.
c) The Research Topic also includes a number of DAS case studies. Staněk et al. focus on reflected S-waves and use microseismic events as sources to image induced hydraulic fractures, which act as reflectors close to events and monitoring fiber. The proposed algorithm is capable of real-time fracture mapping and tracking fracture evolution in space and time. Nakajima et al. present a case study of a walk-away DAS-VSP survey applied to monitor geological CO2 storage at a pilot site in Japan. The authors obtained DAS data using a single-mode fiber via a casing installation. The authors apply conventional VSP processing workflows to the acquired DAS data and obtain promising images. Zhang et al. develop optimized algorithms for accurate tracking of the train position, speed, and number of trains traversing the position of the DAS system. The authors transform a 6-day set of continuous DAS data sensed by a 2-km cable into the time-velocity domain using beamforming and automatically extract train position and velocity from the time-beampower curves. It serves a supplementary role to conventional temporally and spatially discrete monitoring systems in railway traffic monitoring.
YW, XC, GJ, BW, XZ, GZ, and YZ edited the Research Topic “Advances and Applications of Distributed Optical Fiber Sensing (DOFS) in Multi-scales Geoscience Problems.”
We thank all the authors for their valuable contributions to this Research Topic, the reviewers who shared their valuable time to improve the manuscripts and the members of the Frontiers Team and the Editor in Chief for their help at different stages of this Research Topic preparation.
Author GZ was employed by the company TGS.
The remaining authors declare that the research was conducted in the absence of any commercial or financial relationships that could be construed as a potential conflict of interest.
All claims expressed in this article are solely those of the authors and do not necessarily represent those of their affiliated organizations, or those of the publisher, the editors and the reviewers. Any product that may be evaluated in this article, or claim that may be made by its manufacturer, is not guaranteed or endorsed by the publisher.
Keywords: editorial, distributed optical fiber sensing (DOFS), multi-scales, geoscience, advances and applications, distributed acoustic sensing (DAS)
Citation: Wang Y, Zhao Y, Jin G, Chen X, Zhan G, Wang B and Zeng X (2023) Editorial: Advances and applications of distributed optical fiber sensing (DOFS) in multi-scales geoscience problems. Front. Earth Sci. 10:1126105. doi: 10.3389/feart.2022.1126105
Received: 17 December 2022; Accepted: 21 December 2022;
Published: 06 January 2023.
Edited and reviewed by:
Derek Keir, University of Southampton, United KingdomCopyright © 2023 Wang, Zhao, Jin, Chen, Zhan, Wang and Zeng. This is an open-access article distributed under the terms of the Creative Commons Attribution License (CC BY). The use, distribution or reproduction in other forums is permitted, provided the original author(s) and the copyright owner(s) are credited and that the original publication in this journal is cited, in accordance with accepted academic practice. No use, distribution or reproduction is permitted which does not comply with these terms.
*Correspondence: Yibo Wang, d2FuZ3lpYm9AbWFpbC5pZ2djYXMuYWMuY24=
Disclaimer: All claims expressed in this article are solely those of the authors and do not necessarily represent those of their affiliated organizations, or those of the publisher, the editors and the reviewers. Any product that may be evaluated in this article or claim that may be made by its manufacturer is not guaranteed or endorsed by the publisher.
Research integrity at Frontiers
Learn more about the work of our research integrity team to safeguard the quality of each article we publish.