- 1Department of the Aviation Manufacturing, Shanghai Civil Aviation College, Shanghai, China
- 2College of Oceanography, Hohai University, Nanjing, China
- 3State Key Laboratory of Simulation and Regulation of Water Cycle in River Basin, China Institute of Water Resources and Hydropower Research, Beijing, China
- 4School of Civil and Hydraulic Engineering, Ningxia University, Yinchuan, China
For the optimal performance of climate simulation, it is critical to localize physical parameterization schemes of climate models, especially in regions with unique geographic characteristics. To study the performance of different physical parameterization schemes for climate simulation in the upper reaches of the Yangtze River Basin (UYRB), we conducted short-term simulations with a resolution of 50 km from 1990 to 1993 using RegCM4 driven by ERA-Interim. Simulations with 72 different scheme combinations were conducted and analyzed to identify the optimal cumulus convection schemes (CSs) and the land surface process schemes in the RegCM4 model. Using the multi-standard scoring method, we evaluated the model performance of precipitation and temperature over the UYRB with different physical parameterization schemes. The results show that precipitation is more sensitive to the CSs than the LSPs. Among the selected CSs, the Kain-Fritsch scheme can better reproduce precipitation characteristics of the UYRB, with a wet bias of only 0.2 mm/day for the multi-year average precipitation. Compared to the community land model (CLM 3.5 and CLM4.5), the Biosphere-Atmosphere Transfer Scheme (BATS) performs better in reproducing temperature characteristics of the UYRB, with an average cold bias of only 0.2°C for all BATS schemes. The soil moisture, evapotranspiration and precipitation are lower and sensible heat flux is higher in CLM simulations, which can account for CLMs simulating warmer temperatures than BATS. The results provide a baseline for the localization of the RegCM4’s parameterization schemes in the UYRB and other regions of China.
Introduction
During the last decades, regional climate models (RCMs) have been increasingly used to produce climate information at scales finer than those of global climate models (GCMs) over regions around the world (Giorgi and Gutowski, 2015). The advantages and disadvantages of RCMs have been discussed in many papers (Centella-Artola et al., 2015; Giorgi and Gutowski, 2015; Martínez-Castro et al., 2018). The performance of regional climate models (RCMs) varies with the study region and season, making it difficult to find a physical parameterization scheme that is universally applicable. Furthermore, because the parameterization of regional climate models is often developed for specific climate conditions and resolutions, identical schemes perform very differently in different simulation regions (Giorgi and Marinucci, 1996). Therefore, it is critical to identify the appropriate parameterization schemes for a given study area to optimize model performance. In East Asia (Singh et al., 2006; Kang and Hong, 2008; Bao, 2013; Huang et al., 2013; Kang et al., 2014), Southeast Asia (Juneng et al., 2016; Ngo-Duc et al., 2017), South Africa (Kalognomou et al., 2013; Llopart et al., 2017), and South America (Reboita et al., 2014), numerous sensitivity tests have been conducted on physical parameters to identify the optimal schemes, and some research has improved the simulation performance of the RegCM by introducing or modifying the parameters of the current physical parameterization schemes (Chow et al., 2006; Gianotti and Eltahir, 2014; Zou et al., 2014).
Many studies have shown that the cumulus convection parameterization scheme has a great impact than other schemes on the simulation performance of the RegCM (Liu and Ding, 2001; Dash et al., 2006; Cao et al., 2007; Zou and Zhou, 2011). Different cumulus convection parameterization schemes have varying effects on the performance of the Chinese monsoon area (Jinsong et al., 2002; Liu et al., 2005; Li and Wang, 2008). Gao et al. (2016) and Gao et al. (2017) compared the simulation performance of several cumulus convection schemes (CSs) in major river basins in China and found that the Emanuel scheme performs better. Although the cumulus convection scheme significantly impacts precipitation, the effect of LSPs on precipitation is also important. In East Asia, Kang et al. (2014) and Li et al. (2016) compared simulations with BATS (Dickinson et al., 1993) and CLM3 (Oleson et al., 2004; Steiner et al., 2009) using the Emanuel convection scheme in RegCM and discovered that BATS’ simulations tend to produce more summer precipitation than those of CLM.
Of the RCMs that are applied to East Asia (e.g., RIMES (Fu and Yuan, 2001), IPRCRegCM (Wang et al., 2003), PRECIS (Xu et al., 2006), MM5 (Qian and Leung, 2007), and WRF (Yu et al., 2015)), the RegCM system is among the most commonly used. There have been many case studies in China on the RegCM physical parameterization scheme. However, previous studies typically focused on East Asia or China as a whole and paid less attention to sub-regions. The upper reaches of the Yangtze River Basin (UYRB) have strong spatiotemporal heterogeneity in temperature and precipitation in the transition zone between the subtropical and temperate zones. Under the influence of the East Asian and South Asian monsoon systems, extreme weather and climate events frequently occur in the basin. Due to the influences of two monsoon systems at various time scales and the region’s highly heterogeneous topographical and geographical features, climate simulation in UYRB is still a considerable challenge, especially for precipitation. Previous studies have not yet clarified the most suitable physical parameterization scheme for the UYRB. Therefore, if climate simulation is to improve in the region, it is critical to evaluate the simulation performance of various RegCM physical parameterization schemes.
The main purpose of the study is to evaluate the simulation performance of precipitation and temperature over the UYRB using the selected CSs and land surface process schemes (LSPs) and to analyze the causes of the differences in performance of the various schemes. Sections 3, Sections 4 present comprehensive evaluation results and discussion of precipitation and temperature for all schemes. The results provide a scientific basis for selecting physical parameterization schemes for regional climate simulation using RegCM4 in the UYRB.
Experiments and methodology
Model description
RegCM4 is an RCM developed by the Abdus Salam International Center for Theoretical Physics (Giorgi and Anyah, 2012; Giorgi et al., 2012) and has been widely used in multi-decadal climate change simulations in East Asia (Gao et al., 2008; Zhang et al., 2015; Gao and Giorgi, 2017). RegCM4 provides five CSs and three LSPs for users to choose and can run different CSs over land and ocean, referred to as “mixed convection.” More detailed information about the RegCM4 can be found in Giorgi et al. (2012).
Experimental design and data
The parameterization set of RegCM4 includes planetary boundary layer scheme, sea surface flux scheme, cumulus scheme, radiation scheme, and land surface scheme. The study used the planetary boundary layer scheme and sea surface flux scheme developed by Holtslag et al. (1990) and Zeng et al. (1998), respectively. The computational domain of RegCM4 is shown in Figure 1. ERA-Interim reanalysis data provided the experiments’ initial and lateral boundary conditions (Uppala et al., 2008). The meteorological observation data used for evaluation in the UYRB were extracted based on CN05 with a resolution of 0.5° developed by Wu and Gao (2013). The inverse distance weighted method was used to interpolate CN05 data into the computational grid center of the RegCM4 model. Details of the model parameter configuration are presented in Table 1.
In this study, RegCM4 with 72 sets of physical parameterization schemes was used to simulate the coordinated regional climate downscaling experiment East Asia phase II domain, and only the UYRB was selected for analysis. As shown in Table 2, among the 72 sets of physical parameterization schemes, the LSP schemes used by Nos. 1–24, Nos. 25–48, and Nos. 49–72 were BATS, CLM3.5, and CLM4.5, respectively. Further details of both BATS and CLM schemes are presented in Llopart, Steiner and Dickinson (Dickinson et al., 1993; Steiner et al., 2005; Steiner et al., 2009; Llopart et al., 2017). The mixed CSs were used overland (LCS) and ocean (OCS), and the convection scheme was selected from Kuo, Grell, Emanuel, Tiedtke, and Kain-Fritsch (Anthes, 1977; Emanuel, 1991; Grell, 1993; Kain, 2004).
Methods
This study uses a multi-standard scoring method to evaluate the performances of different physical parameterization schemes in RegCM4. The criteria include mean annual precipitation (or temperature), standard deviation, annual climate cycle, normalized root mean square error (NRMSE), spatial distribution, empirical orthogonal function (EOF), and probability density function (PDF). Table 3 shows the criteria used in the evaluation and the corresponding weights. The rank score (RS) from 0 to 9, which is used to assess each assessment criterion, is written as follows:
where xi is the relative error (RE) or other relevant statistics between the observation and the ith RegCM result. When xi represents RE, the larger the xi, the higher the RS of the ith RegCM result in the assessment criterion. The performance score of each sensitivity test is obtained by the weighted summation of RS for all assessment criteria. RE is used to evaluate the annual mean and standard deviation of the climate variables simulated by RegCM4. NRMSE (Hanna and Heinold, 1985) is used to evaluate RegCM4’s ability to simulate climate variables.
Where XGi and XOi are the values of the simulated and observed climate variables in RegCM4, respectively, during the simulation period i, n is the length of the RegCM4 simulation period, and
The annual cycle and spatial distribution of climate variables simulated by RegCM4 are evaluated using correlation coefficients. Since the empirical orthogonal function (EOF) can qualitatively and quantitatively analyze the relevant characteristics of the spatial field of climate variables (Mu et al., 2004), we used this method to compare the temporal and spatial changes in the climate variables simulated by RegCM4 and in observation on a monthly scale (Harvey and Wigley, 2003). The majority of the variance in EOF can be represented by the first two eigen vectors, so this study only analyses these first two.
Two indicators, the BS and the significance score (Sscore), are used to evaluate the probability density distribution of climate variables on a monthly scale for the RegCM4 simulation results. The BS is the mean square error of the probability prediction (Brier, 1950; Fraedrich and Leslie, 1987), and Sscore is the smallest cumulative probability of the observed and simulated distribution in each equal sequence of values.
In the formula, Pmi and Poi are the probabilities that the climate variables simulated and observed by RegCM4 appear in the ith data segment, respectively, and n is the sequence length divided equally according to the original sequence values of the climate variables. The smaller the BS or the larger the Sscore, the better the simulation ability of the RegCM for the probability density distribution in the region.
Results
Comprehensive evaluation of the physical parameterization scheme
Figure 2 and Table 4 show the evaluation results of the simulated temperature and precipitation from 72 sets of parameterization schemes in the UYRB. As shown in Figure 2, the BATS group (Nos.1–24) has the best performance for temperature, followed by the CLM3.5 group (Nos.25–48), while the CLM4.5 group (Nos.49–72) has the worst performance for temperature. For precipitation, the BATS group performs the worst, followed by the CLM3.5 and CLM4.5 groups. In general, temperature is more sensitive to the change of the LSPs, while precipitation is more sensitive to the CSs.
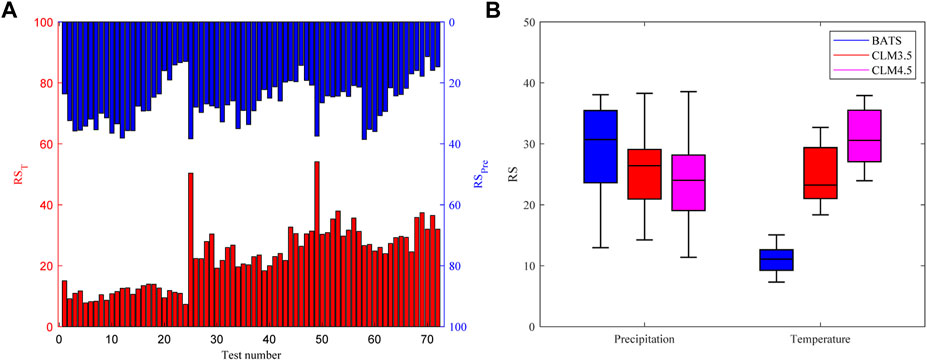
FIGURE 2. Histogram and box plot of the precipitation and temperature ranking score for the 72 sets of physical parameterization schemes. (A) Blue histogram represents precipitation scores, and red histogram represents temperature scores; (B) Blue, red and purple boxes represent BATS, CLM3.5 and CLM4.5 scenarios, respectively.
According to the RS score, the Kain-Fritsch groups perform best among the 72 schemes. All tests using Kain-Fritsch scheme (e.g., Nos.20–24, Nos.44–48 and Nos.68–72) have better total RS value for precipitation and temperature than other schemes (Table 2; Figure 2A). Therefore, the following nine schemes (three groups) are selected to further analyze the performance differences between different CSs and LSPs in the YRB (Table 4): 1) the LCS and OCS are Emanuel (Nos.12, 36, and 60); 2) The Kain-Fritsch is used for LCS, and Tiedtke-Kain is used for OCS (Nos.19, 43, and 67); 3) Both LCS and OCS are Kain-Fritsch (Nos.24, 48, and 72). For each group of three simulations according to CSs, the LSPs for the first, second, and third members are BATS, CLM3.5, and CLM4.5, respectively.
Performance evaluation for climatological of precipitation and temperature
Figures 3A–C shows the Taylor diagrams of the simulated annual, summer, and winter precipitation in the UYRB. As shown in Figure 4 and Supplementary Figure S1, the selected nine schemes can capture the spatial pattern of precipitation in the UYRB, and the simulation performance in winter is better than that in summer, but there are large wet biases as a whole. The spatial correlation coefficient between the observed and simulated summer precipitation over the UYRB by the Emanuel, Kain-Fritsch, and Tiedtke-Kain schemes is about 0.2, but it can reach more than 0.6 in winter. As for the precipitation pattern, the precipitation simulated by the selected nine schemes shows a wet bias in the southwestern mountainous area of the Sichuan Basin, while the precipitation is low in the eastern part of the Sichuan Basin (Figure 4). In addition, when the spatial patterns of precipitation in summer and winter are compared, the wet biases of precipitation in winter are smaller than in summer. The precipitation patterns simulated by different CSs (e.g., Emanuel, Kain-Fritsch, and Tiedtke-Kain) in BATS, CLM3.5, and CLM4.5 experiments are significantly different. The Emanuel, Kain-Fritsch, and Tiedtke-Kain schemes overestimate the annual precipitation by 2.7 mm/day, 1.6 mm/day, and 0.2 mm/day, respectively. The wet biases of precipitation simulated by Emanuel are significantly higher than those of other CSs related to Emanuel’s parameterization setting (Fraedrich and Leslie, 1987; Im et al., 2008). Moreover, except in the east of the Sichuan Basin, the precipitation in the UYRB simulated by the selected scheme generally has a large wet bias, which is related to the incomplete characterization of mesoscale weather activities by the RegCM4. The precipitation biases between the simulations and observations may be due to the imperfection of the convective parameterization scheme (Wang and Seaman, 1997) and the resolution of the downscaling experiment (Shi et al., 2018), resulting in strong wet biases. The same phenomenon has also been found in other regions (Oh and Suh, 2018). In addition, previous studies have shown that the precipitation simulation by RegCM exhibits a significant systematic bias (Oh and Suh, 2018), especially in mountainous areas (Gao et al., 2017; Huang et al., 2020).
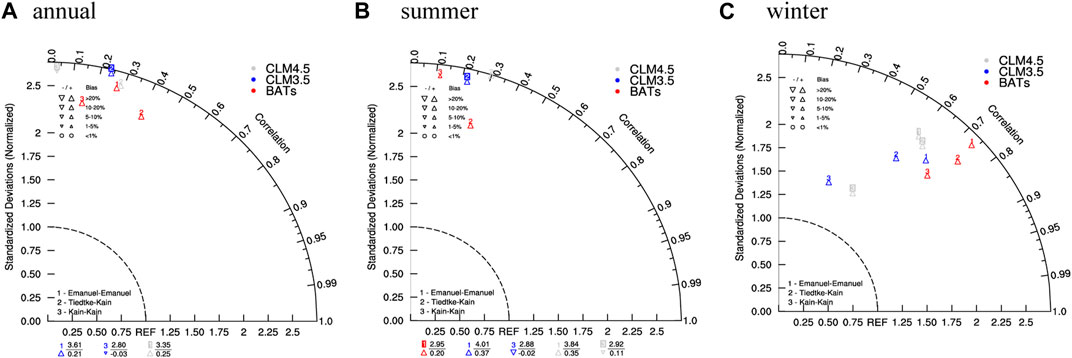
FIGURE 3. Taylor diagrams of annual, summer and winter precipitation in the UYRB. (Values at the bottom are statistics that exceed the threshold of Taylor diagrams.)
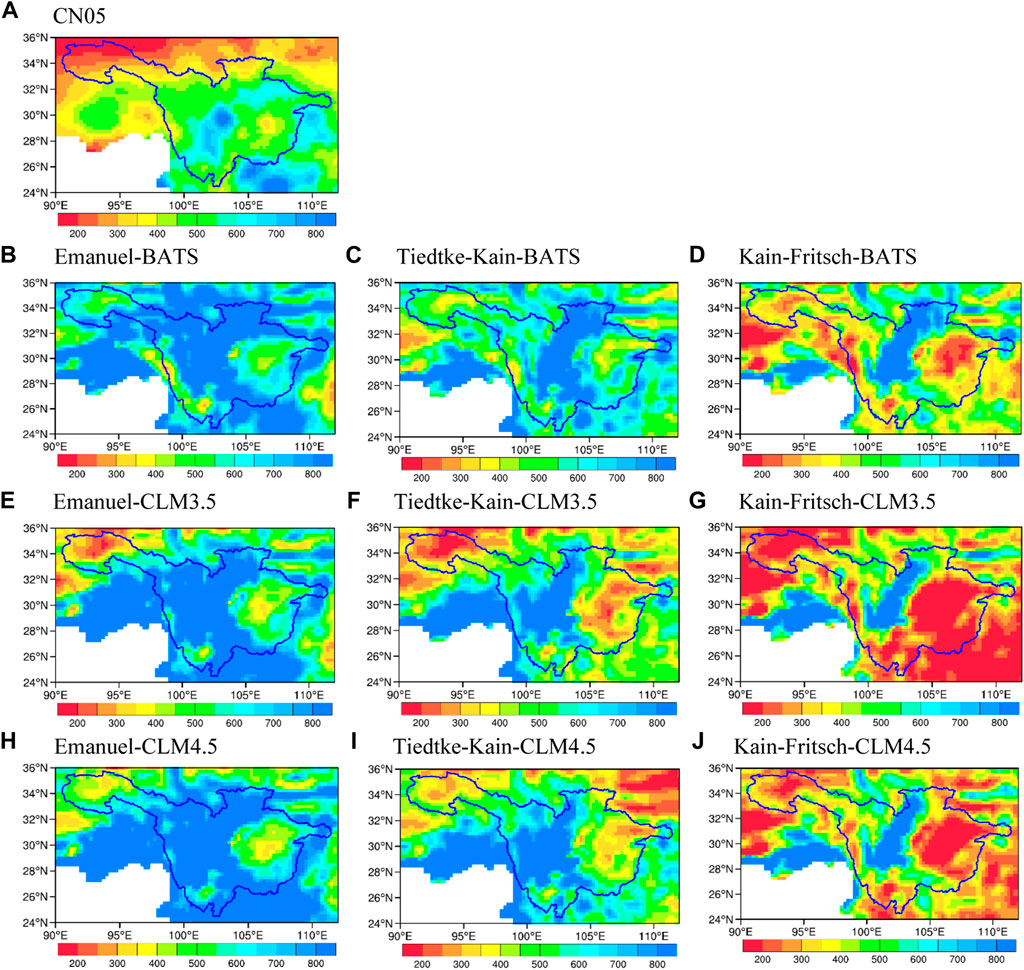
FIGURE 4. Spatial distribution of observed and simulated multi-year average summer total precipitation (unit: mm) for the current climate (1991–1993) over the UYRB. [(A) CN05, (B) simulation of the combination scheme of Emanuel and BATS, (C) simulation of the combination scheme of Tiedtke-Kain and BATS, (D) simulation of the combination scheme of Kain-Fritsch and BATS, (E–G) same as (B–D) but for CLM3.5, and (H–J) same as (B–D) but for CLM4.5].
As shown in Figures 5 and Supplementary Figure S2, the spatial pattern of multi-year average summer and winter temperature simulated by the selected schemes is very close to the observation, and the temperature gradient from northwest to southeast in the UYRB can be well captured. The spatial correlation coefficients of both simulated summer and winter temperatures are above 0.95, but there is a small bias (Figure 6). As for the spatial pattern of temperature, there is no apparent difference between the Emanuel, Kain-Fritsch, and Tiedtke-Kain schemes. In summer, there is a large warm bias in the Sichuan Basin, while in winter, there is a large cold bias in the northwest Qinghai-Tibet Plateau. In general, the annual mean biases of temperature simulated by the Emanuel, Tiedtke-Kain, and Kain-Fritsch schemes (average of the three LSPs) are 1.01°C, 1.16°C, and 2.07°C, respectively.
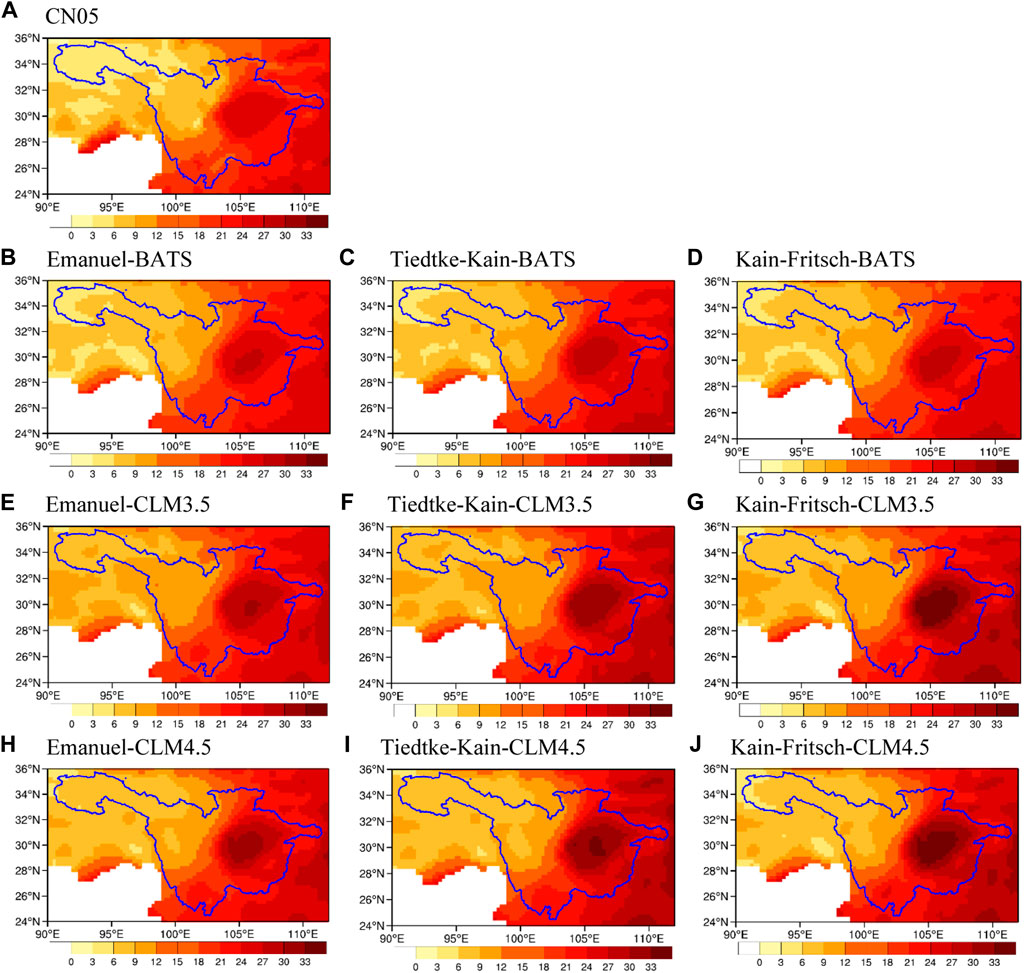
FIGURE 5. Spatial distribution of observed and simulated multi-year summer average temperature (unit: °C) for the current climate (1991–1993) over the UYRB. [(A) CN05, (B) simulation of the combination scheme of Emanuel and BATS, (C) simulation of the combination scheme of Tiedtke-Kain and BATS, (D) simulation of the combination scheme of Kain-Fritsch and BATS, (E–G) same as (B–D) but for CLM3.5, and (H–J) same as (B–D) but for CLM4.5].
Performance evaluation for annual cycle and probability density
As shown in Figures 7A–C, compared with the Tiedtke-Kain and Kain-Fritsch schemes, the Emanuel scheme has a larger overestimation of annual precipitation, especially in summer. In the Kain-Fritsch scheme, the annual precipitation cycle obtained using three different LSPs (BATS, CLM3.5, and CLM4.5) is consistent with the observation. For air temperature, the Emanuel, Tiedtke-Kain, and Kain-Fritsch schemes can simulate the annual cycle well (Figure 7D-F). Overall, the Emanuel scheme simulation results for the annual air temperature cycle are slightly better than those of Tiedtke-Kain and Kain-Fritsch.
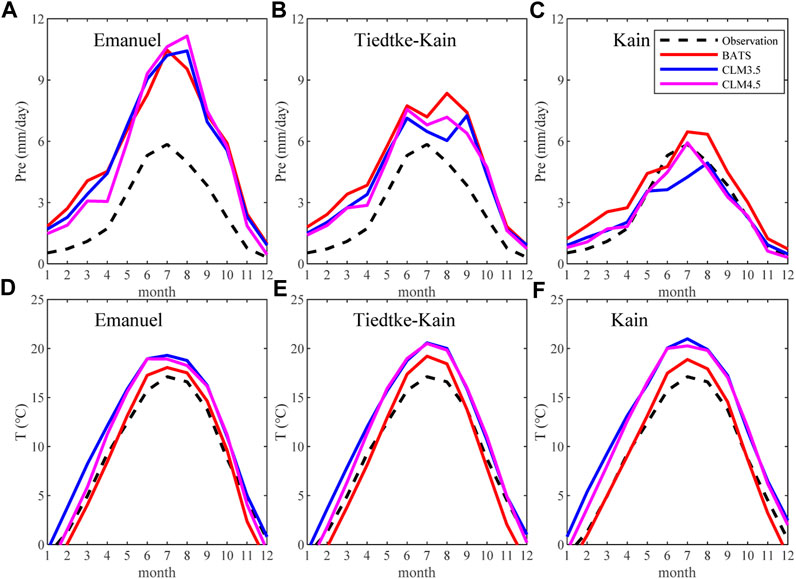
FIGURE 7. Observed and simulated annual cycles of precipitation (mm/day) and temperature (°C) for the UYRB. [(A) precipitation simulated by Emanuel, (B) precipitation simulated by Tiedtke-Kain, (C) precipitation simulated by Kain-Fritsch, and (D–F) same as (A–C) but for temperature].
In general, in the precipitation simulation of the UYRB, the parameterization combination of CLMs and Kain-Fritsch in RegCM4 performs better than other scheme combinations. As shown in Figures 8A–C, different intensities of precipitation simulated by Emanual and Tiedtke-Kain are generally higher than those observed. Among the selected CSs, the Kain-Fritsch scheme simulates precipitation intensity more closely to the observation. However, different LSPs may lead to slight differences between simulated and observed precipitation intensities. For example, precipitation intensities simulated by BATS (blue line) are higher than the observation, while those simulated by CLM3.5 (black line) and CLM4.5 (red line) are lower.
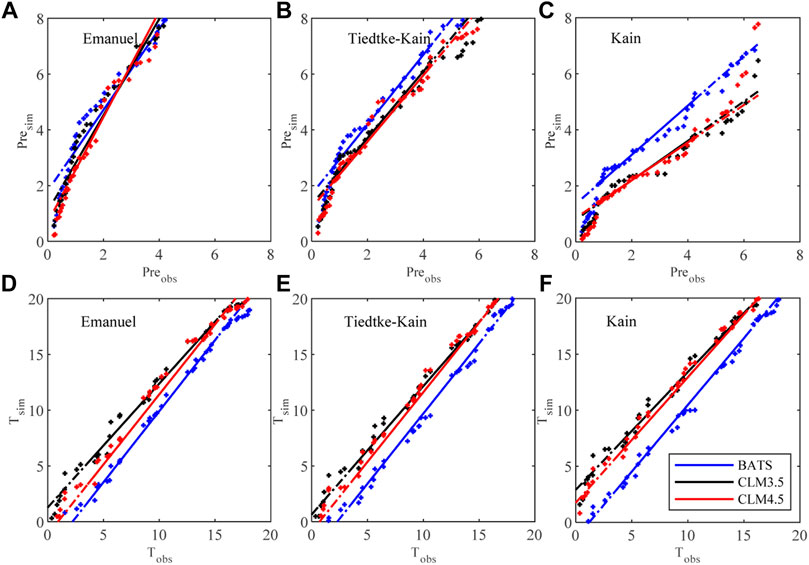
FIGURE 8. The Q-Q plot of precipitation (A–C) and temperature (D–F) in the UYRB between the simulation and observation. [(A) precipitation simulated by Emanuel, (B) precipitation simulated by Tiedtke-Kain, (C) precipitation simulated by Kain-Fritsch, and (D–F) same as (A–C) but for the temperature].
For air temperature, the air temperature Q-Q plots obtained by Emanual, Tiedtke-Kain, and Kain-Fritsch schemes show little difference, and the probability density distributions of air temperature simulated by the three groups of CSs are close to the observed distribution (Figure 8D-F). Among the three LSPs, the quantile distribution simulated by BATS is closer to the observed values, followed by CLM4.5.
Discussion
From the above analysis, the different CSs and LSPs have different simulation performances on precipitation and temperature, and the scheme with a better simulation effect on temperature may have a worse simulation effect on precipitation, and vice versa. Therefore, in practical applications, appropriate schemes need to be selected according to the research objectives. In this study, the Kain-Fritsch scheme, combined with three LSPs, is selected to further analyze the impact of different LSPs on the simulation performance of temperature and precipitation. The statistical differences of climatic factors in different months between CLMs (CLM3.5 and CLM4.5) and BATS are presented in Table 5. As seen in the table, the total precipitation and evaporation simulated by CLMs is less than that of BATS, and the surface soil moisture (SM) simulated by CLMs is significantly drier than that of BATS. The difference between CLMs and BATS in domain-averaged precipitation and evaporation over the UYRB is −0.8–0.9 mm/day and −0.9–1.0 mm/day, respectively. For soil moisture, CLM3.5 is 30% drier than BATS and reaches 13.5% in CLM4.5, consistent with previous studies (Diro et al., 2012). Compared with CLM, BATS can produce higher soil moisture, resulting in higher latent heat and moist static energy (Im et al., 2014), which in turn enhances convective activity and results in larger convective precipitation (Alfieri et al., 2006; Myoung and Nielsen-Gammon, 2010; Hauck et al., 2011). The annual average sensible heat flux of CLM3.5 and CLM4.5 is 10.6W/m2 and 5.3W/m2 higher than that of BATS respectively, indicating that soil moisture influences the surface energy budget by regulating the relative distribution of latent and sensible heat fluxes. In addition, there is a strong connection between the convective precipitation scheme and large-scale precipitation scheme in RegCM4, and the bias of convective precipitation also affects large-scale precipitation (Gianotti et al., 2011).
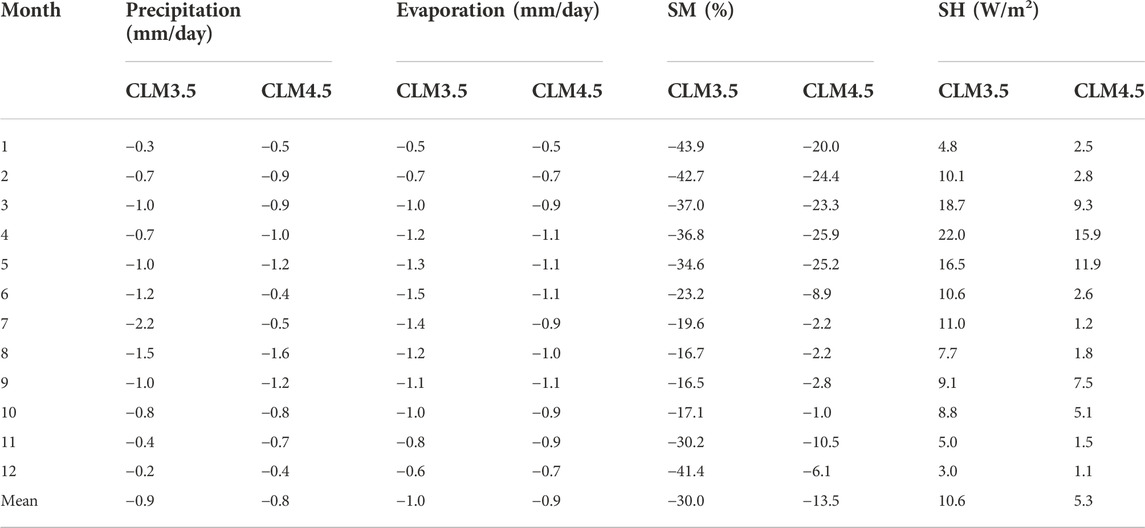
TABLE 5. Differences in hydrometeorological elements in the UYRB between the CLMs (CLM3.5 and CLM4.5) and BATS schemes.
The warm-dry difference between the BATS scheme and CLMs (CLM3.5 and CLM4.5) further affects the water vapor transport and atmospheric circulation in the region, impacting the simulated precipitation. As shown in Figure 9 and Supplementary Figure S3, the temperature difference between the CLMs scheme and the BATS scheme gradually decreases with the increase of altitude, as the land surface schemes mainly affect surface fluxes. For example, the winter temperatures at 850 hPa in the eastern part of the study area simulated by the CLMs scheme are more than 5°C higher than those of BATS. With altitudes up to 500 hPa, the warm bias in the CLMs is reduced to -1-1°C.
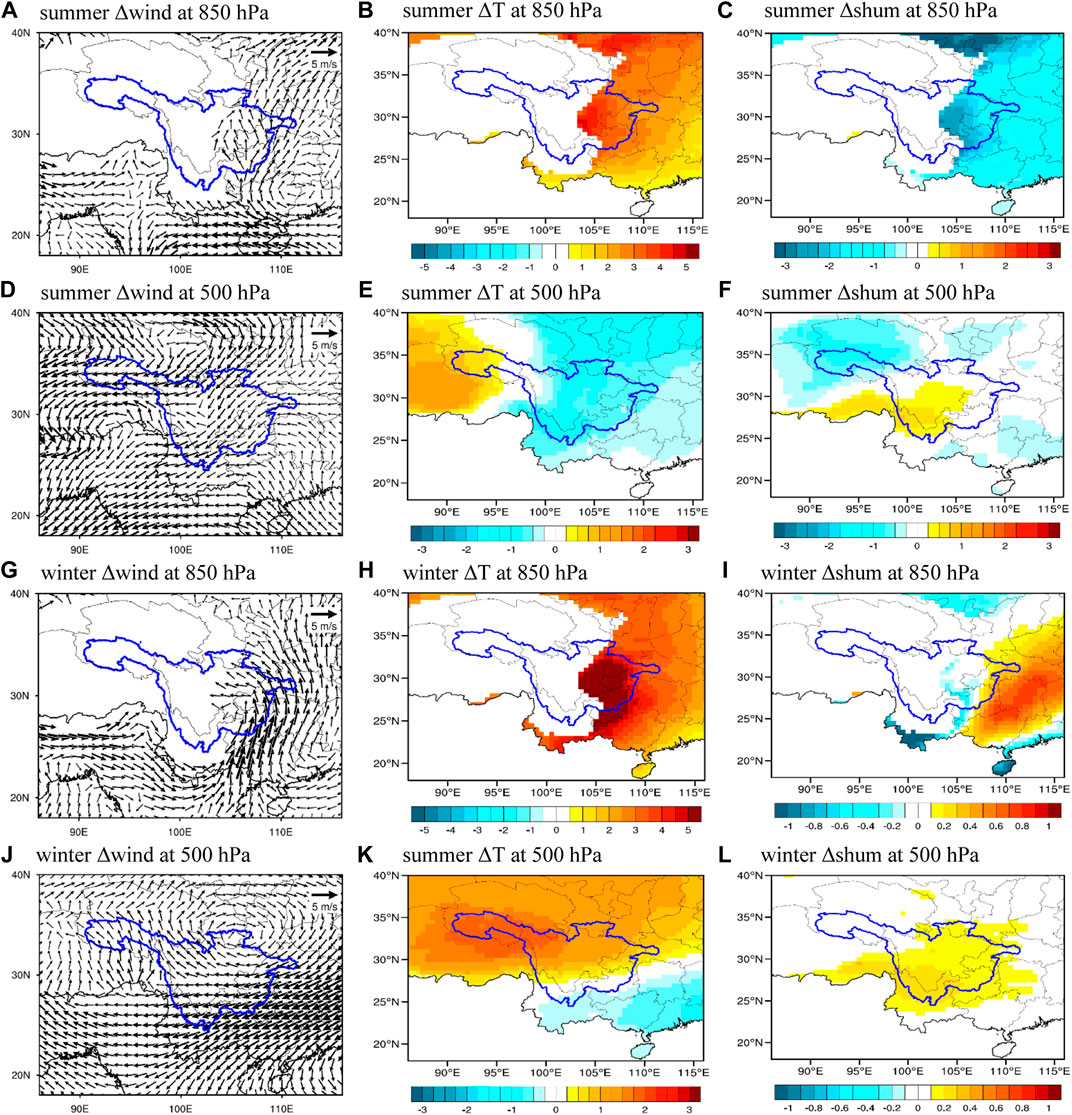
FIGURE 9. Difference in the wind field (m/s), temperature (°C), and specific humidity (g/kg) between CLM3.5 and BATS (defined as CLM3.5-BATS). [(A) difference in summer winds at 850 hPa, (D) difference in summer winds at 500 hPa, (G) difference in winter winds at 850 hPa, (J) difference in winter winds at 850 hPa; (B,E,H,K) same as (A,D,G,J) but for temperature; and (C,F,I,L) same as (A,D,G,J) but for specific humidity].
Similarly, the wind field between CLMs and BATs also shows great differences. As shown in Figure 9 and Supplementary Figure S3, the summer wind field simulated by CLMs is weaker than that of BATS. The wind field difference at 850 hPa in the UYRB is mainly an easterly wind, which may hinder the transport of low-level water vapor from the Bay of Bengal to the basin. In winter, the wind field difference between CLMs and BATS is larger than that in summer, and the differential wind field at 850 hPa is southerly, indicating that the intensity of dry cold air from the north simulated using CLMs is weakened. In addition, the summer specific humidity in the UYRB simulated by CLMs is generally smaller than that of BATS, especially at 850 hPa. The dry bias makes CLMs simulate less summer precipitable water than BATS, which tends to reduce the production of summer precipitation. Therefore, the differences in the atmospheric circulation field and water vapor flux jointly affect the precipitation in the UYRB simulated by CLMs and BATS.
Conclusion
In this study, the ERA-Interim reanalysis data were used as the initial and lateral boundary conditions for the RegCM4 to conduct 4-year simulations of 72 parameterization schemes. Additionally, the multi-standard scoring method was used to evaluate the performance of the selected schemes for the UYRB. Finally, the influence of different CSs and LSPs on the precipitation and temperature simulation performance over the UYRB was analyzed. The main findings are as follows:
The RegCM4 simulated temperature performed better than the simulated precipitation. RegCM4 captured the main spatial distribution characteristics of temperature and precipitation, annual cycle processes, and probability density. In simulating temperature, RegCM4 was particularly successful at capturing the main spatial pattern of observation and showed a high performance. The simulated total precipitation overestimated the observations in the study area and deviated from the observed spatial distribution in summer.
Precipitation was highly sensitive to the cumulus convective parameterization scheme. The commonly used Emanuel scheme overestimated the annual average precipitation by approximately 2.7 mm/day. Among the tested CSs, the Kain-Fritsch scheme had the best comprehensive simulation performance for precipitation in the UYRB, only overestimating the simulated annual average precipitation by 0.2 mm/day. Among the LSPs examined, the BATS group (Nos. 1–24) had the best comprehensive simulation performance for temperature in the UYRB, with an average cold bias of only 0.2°C, while the CLMs group (Nos. 25–72) had a warm bias of approximately 2.5°C.
Under the best performing CS Kain-Fritsch, the soil moisture in the UYRB simulated by CLMs was 13.5%–30% drier than that of BATS. Compared with BATS, the warmer temperature and weaker evapotranspiration in CLMs resulted in weaker horizontal and vertical water vapor transport capacity in the UYRB and less atmospheric precipitable water, leading to less precipitation in simulations using CLMs. In addition, the drier atmosphere simulated by CLMs increased the net radiation flux to the surface and changed the surface energy budget, resulting in a difference in air temperature between CLMs and BATS.
It is important to note that all the selected schemes possess common deficiencies in their precipitation simulations for the UYRB, characterized by a wet bias in mountainous areas. Substantial effort is needed in the future, including more extensive analysis of simulations, implementation, and testing of new physical processes in models, and more numerical experiments, to better understand and further improve the RegCM.
Data availability statement
The data analyzed in this study is subject to the following licenses/restrictions: The authors greatly appreciate the data availability and service provided by the RegCM4 science team. Requests to access these datasets should be directed to http://clima-dods.ictp.it/Data/RegCM_Data/.
Author contributions
BL designed the study, completed the original draft. YH provided some software for the study. LD provided financial support for the research. DW revised the original draft. All authors commented on the manuscript and agreed to the published version of the manuscript.
Funding
8This study was jointly funded by the Open Research Fund of State Key Laboratory of Simulation and Regulation of Water Cycle in River Basin, China Institute of Water Resources and Hydropower Research (IWHR-SKL-KF202204), the Jiangsu Funding Program for Excellent Postdoctoral Talent (2022ZB147), the Natural Science Foundation of China (51909275), and TianHe Qingsuo Project special fund project in the field of climate, meteorology and ocean. In addition, high-performance computing resources were provided by the national supercomputer center in Tianjin, China.
Acknowledgments
The authors greatly appreciate the data availability and service provided by the RegCM4 science team.
Conflict of interest
The authors declare that the research was conducted in the absence of any commercial or financial relationships that could be construed as a potential conflict of interest.
Publisher’s note
All claims expressed in this article are solely those of the authors and do not necessarily represent those of their affiliated organizations, or those of the publisher, the editors and the reviewers. Any product that may be evaluated in this article, or claim that may be made by its manufacturer, is not guaranteed or endorsed by the publisher.
Supplementary material
The Supplementary Material for this article can be found online at: https://www.frontiersin.org/articles/10.3389/feart.2022.1092368/full#supplementary-material
References
Alfieri, L., Claps, P., D Odorico, P., Laio, F., and Over, T.-M. (2006). An analysis of the soil moisture feedback on convective and stratiform precipitation. J. Hydrometeorol. 2, 280–291. doi:10.1175/2007jhm863.1
Anthes, R.-A. (1977). A cumulus parameterization scheme utilizing a one-dimensional cloud model. Mon. Weather Rev. 3, 270–286. doi:10.1175/1520-0493(1977)105<0270:acpsua>2.0.co;2
Bao, Y. (2013). Simulations of summer monsoon climate over East Asia with a regional climate model (RegCM) using Tiedtke convective parameterization scheme (CPS). Atmos. Res. 134, 35–44. doi:10.1016/j.atmosres.2013.06.009
Brier, G.-W. (1950). Verification of forecasts expressed in terms of probability. Mon. Weather Rev. 1, 1–3. doi:10.1175/1520-0493(1950)078<0001:vofeit>2.0.co;2
Cao, J., Zhang, X.-N., You, Y.-L., and Yang, R.-W. (2007). Applicability of cumulus convective parameter schemes in RegCM3 to the rainfall over the Longitudinal Range-Gorge Region. Chin. Sci. Bull. 2, 115–121. doi:10.1007/s11434-007-7015-3
Centella-Artola, A., Taylor, M.-A., Bezanilla-Morlot, A., Martinez-Castro, D., Campbell, J.-D., Stephenson, T.-S., et al. (2015). Assessing the effect of domain size over the Caribbean region using the PRECIS regional climate model. Clim. Dyn. 7, 1901–1918. doi:10.1007/s00382-014-2272-8
Chow, K.-C., Chan, J.-C.-L., Pal, J.-S., and Giorgi, F. (2006). Convection suppression criteria applied to the MIT cumulus parameterization scheme for simulating the Asian summer monsoon. Geophys. Res. Lett. 24, 247099–L25199. doi:10.1029/2006gl028026
Dash, S.-K., Shekhar, M.-S., and Singh, G.-P. (2006). Simulation of Indian summer monsoon circulation and rainfall using RegCM3. Theor. Appl. Climatol. 1, 161–172. doi:10.1007/s00704-006-0204-1
Dickinson, R.-E., Henderson-Sellers, A., and Kennedy, J. (1993). Biosphere atmosphere transfer scheme (BATS) version 1e as coupled to the NCAR community climate modelNCAR Technical Note NCAR/TN-387+STR. Boulder, Colorado: National Center for Atmospheric Research.
Diro, G.-T., Rauscher, S.-A., Giorgi, F., and Tompkins, A.-M. (2012). Sensitivity of seasonal climate and diurnal precipitation over Central America to land and sea surface schemes in RegCM4. Clim. Res. 52, 31–48. doi:10.3354/cr01049
Emanuel, K.-A. (1991). A scheme for representing cumulus convection in large-scale models. J. Atmos. Sci. 21, 2313–2329. doi:10.1175/1520-0469(1991)048<2313:asfrcc>2.0.co;2
Fraedrich, K., and Leslie, L.-M. (1987). Evaluation of techniques for the operational, single station, short-term forecasting of rainfall at a midlatitude station (Melbourne). Mon. Wea. Rev. 115, 1645–1654. doi:10.1175/1520-0493(1987)115<1645:eotfto>2.0.co;2
Fu, C.-B., and Yuan, H.-L. (2001). An virtual numerical experiment to understand the impacts of recovering natural vegetation on the summer climate and environmental conditions in East Asia. Chin. Sci. Bull. 14, 1199–1203. doi:10.1007/bf02900602
Gao, X.-J., and Giorgi, F. (2017). Use of the RegCM system over East Asia: Review and perspectives. Engineering 5, 766–772. doi:10.1016/j.eng.2017.05.019
Gao, X.-J., Shi, Y., Han, Z.-Y., Wang, M.-L., Wu, J., Zhang, D.-F., et al. (2017). Performance of RegCM4 over major river basins in China. Adv. Atmos. Sci. 4, 441–455. doi:10.1007/s00376-016-6179-7
Gao, X.-J., Shi, Y., Song, R., Giorgi, F., Wang, Y., and Zhang, D.-F. (2008). Reduction of future monsoon precipitation over China: Comparison between a high resolution RCM simulation and the driving GCM. Meteorol. Atmos. Phys. 1-4, 73–86. doi:10.1007/s00703-008-0296-5
Gao, X.-J., Ying, S., and Giorgi, F. (2016). Comparison of convective parameterizations in RegCM4 experiments over China with CLM as the land surface model. Atmos. Ocean. Sci. Lett. 4, 246–254. doi:10.1080/16742834.2016.1172938
Gianotti, R.-L., and Eltahir, E.-A.-B. (2014). Regional climate modeling over the maritime continent. Part II: New parameterization for autoconversion of convective rainfall. J. Clim. 4, 1504–1523. doi:10.1175/jcli-d-13-00171.1
Gianotti, R.-L., Zhang, D., and Eltahir, E.-A.-B. (2011). Assessment of the regional climate model version 3 over the maritime continent using different cumulus parameterization and land surface schemes. J. Clim. 2, 638–656. doi:10.1175/jcli-d-11-00025.1
Giorgi, F., and Anyah, R.-O. (2012). INTRODUCTION the road towards RegCM4 F. Giorgi1, *, R. O. Anyah2. Clim. Res. 52, 3–6. doi:10.3354/cr01089
Giorgi, F., Coppola, E., Solmon, F., Mariotti, L., Sylla, M.-B., Bi, X., et al. (2012). RegCM4: Model description and preliminary tests over multiple CORDEX domains. Clim. Res. 52, 7–29. doi:10.3354/cr01018
Giorgi, F., and Gutowski, W.-J. (2015). Regional dynamical downscaling and the CORDEX initiative. Annu. Rev. Environ. Resour. 40, 467–490. doi:10.1146/annurev-environ-102014-021217
Giorgi, F., and Marinucci, M.-R. (1996). A investigation of the sensitivity of simulated precipitation to model resolution and its implications for climate studies. Mon. Weather Rev. 1, 148–166. doi:10.1175/1520-0493(1996)124<0148:aiotso>2.0.co;2
Grell, G.-A. (1993). Prognostic evaluation of assumptions used by cumulus parameterizations. Mon. Weather Rev. 3, 764–787. doi:10.1175/1520-0493(1993)121<0764:peoaub>2.0.co;2
Hanna, S.-R., and Heinold, D.-W. (1985). Development and application of a simple method for evaluating air quality models. American Petroleum Institute.
Harvey, L., and Wigley, T. (2003). Characterizing and comparing control-run variability of eight coupled AOGCMs and of observations. Part 1: Temperature. Clim. Dyn. 7, 619–646. doi:10.1007/s00382-003-0357-x
Hauck, C., Barthlott, C., Krauss, L., and Kalthoff, N. (2011). Soil moisture variability and its influence on convective precipitation over complex terrain. Q. J. R. Meteorol. Soc. S1, 42–56. doi:10.1002/qj.766
Holtslag, A.-A.-M., De Bruijn, E.-I.-F., and Pan, H. (1990). A high resolution air mass transformation model for short-range weather forecasting. Mon. Wea. Rev. 8, 1561–1575. doi:10.1175/1520-0493(1990)118<1561:ahramt>2.0.co;2
Huang, W.-R., Chan, J.-C.-L., and Au-Yeung, A.-Y.-M. (2013). Regional climate simulations of summer diurnal rainfall variations over East Asia and Southeast China. Clim. Dyn. 7-8, 1625–1642. doi:10.1007/s00382-012-1457-2
Huang, Y., Xiao, W., Hou, G., Yi, L., Li, Y., and Zhou, Y. (2020). Changes in seasonal and diurnal precipitation types during summer over the upper reaches of the Yangtze River Basin in the middle twenty-first century (2020–2050) as projected by RegCM4 forced by two CMIP5 global climate models. Theor. Appl. Climatol. 3, 1055–1070. doi:10.1007/s00704-020-03364-4
Im, E.-S., Gutowski, W.-J., and Giorgi, F. (2008). Consistent changes in twenty-first century daily precipitation from regional climate simulations for Korea using two convection parameterizations. Geophys. Res. Lett. 14, L14706. doi:10.1029/2008gl034126
Im, E., Marcella, M.-P., and Eltahir, E.-A.-B. (2014). Impact of potential large-scale irrigation on the west african monsoon and its dependence on location of irrigated area. J. Clim. 3, 994–1009. doi:10.1175/JCLI-D-13-00290.1
Jinsong, P., Guoqing, Z., and Kun, G. (2002). Comparisons of three convection parameterization schemes in regional climate simulations. Chin. J. Atmos. Sci. 2, 220–228. (In Chinese).
Juneng, L., Tangang, F., Chung, J.-X., Ngai, S.-T., Tay, T.-W., Narisma, G., et al. (2016). Sensitivity of Southeast Asia rainfall simulations to cumulus and air-sea flux parameterizations in RegCM4. Clim. Res. 1, 59–77. doi:10.3354/cr01386
Kain, J.-S. (2004). The kain–fritsch convective parameterization: An update. J. Appl. Meteor. 1, 170–181. doi:10.1175/1520-0450(2004)043<0170:tkcpau>2.0.co;2
Kalognomou, E.-A., Lennard, C., Shongwe, M., Pinto, I., Favre, A., Kent, M., et al. (2013). A diagnostic evaluation of precipitation in CORDEX models over southern Africa. J. Clim. 23, 9477–9506. doi:10.1175/jcli-d-12-00703.1
Kang, H.-S., and Hong, S.-Y. (2008). Sensitivity of the simulated East Asian summer monsoon climatology to four convective parameterization schemes. J. Geophys. Res. 113, D15119. doi:10.1029/2007jd009692
Kang, S., Im, E.-S., and Ahn, J.-B. (2014). The impact of two land-surface schemes on the characteristics of summer precipitation over East Asia from the RegCM4 simulations. Int. J. Climatol. 15, 3986–3997. doi:10.1002/joc.3998
Li, J.-Y., and Wang, H.-J. (2008). Influences of modeling the summer heavy rain process in southern China using cumulus convective parameterization schemes. Clim. Environ. Res. 2, 149–160. (In Chinese).
Li, Y.-B., Tam, C.-Y., Huang, W.-R., Cheung, K.-K.-W., and Gao, Z.-Q. (2016). Evaluating the impacts of cumulus, land surface and ocean surface schemes on summertime rainfall simulations over East-to-southeast Asia and the Western north Pacific by RegCM4. Clim. Dyn. 7-8, 2487–2505. doi:10.1007/s00382-015-2714-y
Liu, X.-D., Jiang, Z.-H., and Luo, S.-R. (2005). A simulation of summer precipitation over eastern China with RegCM3. J. Nanjing Inst. Meteorology 3, 351–359. (In Chinese).
Liu, Y.-M., and Ding, Y.-H. (2001). Modified mass flux cumulus parameterization scheme and its simulation experiment partI: Mass flux scheme and its simulation of the flooding in 1991. Acta Meteorol. Sin., 10–22.
Llopart, M., Da Rocha, R.-P., Reboita, M., and Cuadra, S. (2017). Sensitivity of simulated South America climate to the land surface schemes in RegCM4. Clim. Dyn. 11-12, 3975–3987. doi:10.1007/s00382-017-3557-5
Martínez-Castro, D., Vichot-Llano, A., Bezanilla-Morlot, A., Centella-Artola, A., Campbell, J., Giorgi, F., et al. (2018). The performance of RegCM4 over the Central America and Caribbean region using different cumulus parameterizations. Clim. Dyn. 11, 4103–4126. doi:10.1007/s00382-017-3863-y
Mu, Q., Jackson, C.-S., and Stoffa, P.-L. (2004). A multivariate empirical-orthogonal-function-based measure of climate model performance. J. Geophys. Res. D15, D15101. doi:10.1029/2004jd004584
Myoung, B., and Nielsen-Gammon, J.-W. (2010). The convective instability pathway to warm season drought in Texas. Part I: The role of convective inhibition and its modulation by soil moisture. J. Clim. 17, 4461–4473. doi:10.1175/2010jcli2946.1
Ngo-Duc, T., Tangang, F.-T., Santisirisomboon, J., Cruz, F., Trinh-Tuan, L., Nguyen-Xuan, T., et al. (2017). Performance evaluation of RegCM4 in simulating extreme rainfall and temperature indices over the CORDEX-Southeast Asia region. Int. J. Climatol. 3, 1634–1647. doi:10.1002/joc.4803
Oh, S.-G., and Suh, M.-S. (2018). Changes in seasonal and diurnal precipitation types during summer over South Korea in the late twenty-first century (2081–2100) projected by the RegCM4.0 based on four RCP scenarios. Clim. Dyn. 51, 3041–3060. doi:10.1007/s00382-017-4063-5
Oleson, K., Dai, Y., Bonan, G., Mike, B., Dickinson, R., Dirmeyer, P., et al. (2004). Technical description of the community land model (CLM). Boulder, Colorado: National center for atmospheric research, 10–5065.
Qian, Y., and Leung, L.-R. (2007). A long-term regional simulation and observations of the hydroclimate in China. J. Geophys. Res. 112, D14104. doi:10.1029/2006jd008134
Reboita, M., Fernandez, J., Pereira Llopart, M., Porfirio, D.-R.-R., Albertani Pampuch, L., and Cruz, F. (2014). Assessment of RegCM4.3 over the CORDEX South America domain: Sensitivity analysis for physical parameterization schemes. Clim. Res. 3, 215–234. doi:10.3354/cr01239
Shi, Y., Wang, G.-L., and Gao, X.-J. (2018). Role of resolution in regional climate change projections over China. Clim. Dyn. 5-6, 2375–2396. doi:10.1007/s00382-017-4018-x
Singh, G.-P., Oh, J.-H., Kim, J.-Y., and Kim, O.-Y. (2006). Sensitivity of summer monsoon precipitation over East Asia to convective parameterization schemes in RegCM3. SOLA - Sci. Online Lett. Atmos. 9, 29–32. doi:10.2151/sola.2006-008
Steiner, A.-L., Pal, J.-S., Giorgi, F., Dickinson, R.-E., and Chameides, W.-L. (2005). The coupling of the Common Land Model (CLM0) to a regional climate model (RegCM). Theor. Appl. Climatol. 3, 225–243. doi:10.1007/s00704-005-0132-5
Steiner, A.-L., Pal, J.-S., Rauscher, S.-A., Bell, J.-L., Diffenbaugh, N.-S., Boone, A., et al. (2009). Land surface coupling in regional climate simulations of the West African monsoon. Clim. Dyn. 6, 869–892. doi:10.1007/s00382-009-0543-6
Uppala, S.-M., Dee, D., Berrisford, P., and Simmons, A. (2008). Towards a climate data assimilation system: Status update of ERA-Interim. Reading, United Kingdom: ECMWF NewsletterECMWF. 115. doi:10.21957/byinox4wot
Wang, W., and Seaman, N.-L. (1997). A comparison study of convective parameterization schemes in a mesoscale model. Mon. Weather Rev. 2, 252–278. doi:10.1175/1520-0493(1997)125<0252:acsocp>2.0.co;2
Wang, Y.-Q., Sen, O.-L., and Wang, B. (2003). A highly resolved regional climate model (IPRC-RegCM) and its simulation of the 1998 severe precipitation event over China. Part I: Model description and verification of simulation. J. Clim. 11, 1721–1738. doi:10.1175/1520-0442(2003)016<1721:ahrrcm>2.0.co;2
Wu, J., and Gao, X.-J. (2013). A gridded daily observation dataset over China region and comparison with the other datasets. Chin. J. Geophys. 04, 1102–1111. (In Chinese).
Xu, Y.-L., Zhang, Y., Lin, E., Lin, W.-T., Dong, W.-J., Jones, R., et al. (2006). Analyses on the climate change responses over China under SRES B2 scenario using PRECIS. Chin. Sci. Bull. 18, 2260–2267. doi:10.1007/s11434-006-2099-8
Yu, E.-T., Sun, J.-Q., Chen, H.-P., and Xiang, W.-L. (2015). Evaluation of a high-resolution historical simulation over China: Climatology and extremes. Clim. Dyn. 7, 2013–2031. doi:10.1007/s00382-014-2452-6
Zeng, X.-B., Zhao, M., and Dickinson, R.-E. (1998). Intercomparison of bulk aerodynamic algorithms for the computation of sea surface fluxes using TOGA COARE and TAO data. J. Clim. 10, 2628–2644. doi:10.1175/1520-0442(1998)011<2628:iobaaf>2.0.co;2
Zhang, S.-B., Lü, S.-H., Bao, Y., and Ma, D. (2015). Sensitivity of precipitation over China to different cumulus parameterization schemes in RegCM4. J. Meteorol. Res. 1, 119–131. doi:10.1007/s13351-014-4042-2
Zou, L.-W., Qian, Y., Zhou, T.-J., and Yang, B. (2014). Parameter tuning and calibration of RegCM3 with MIT–emanuel cumulus parameterization scheme over CORDEX East Asia domain. J. Clim. 20, 7687–7701. doi:10.1175/jcli-d-14-00229.1
Keywords: RegCM4, physical parameterization scheme, precipitation, temperature, Yangtze River Basin
Citation: Li B, Huang Y, Du L and Wang D (2023) Sensitivity experiments of RegCM4 using different cumulus and land surface schemes over the upper reaches of the Yangtze river. Front. Earth Sci. 10:1092368. doi: 10.3389/feart.2022.1092368
Received: 08 November 2022; Accepted: 21 November 2022;
Published: 20 January 2023.
Edited by:
Jianhua Sun, Institute of Atmospheric Physics (CAS), ChinaReviewed by:
Zhenhua Li, University of Saskatchewan, CanadaDanqiong Dai, Institute of Atmospheric Physics (CAS), China
Copyright © 2023 Li, Huang, Du and Wang. This is an open-access article distributed under the terms of the Creative Commons Attribution License (CC BY). The use, distribution or reproduction in other forums is permitted, provided the original author(s) and the copyright owner(s) are credited and that the original publication in this journal is cited, in accordance with accepted academic practice. No use, distribution or reproduction is permitted which does not comply with these terms.
*Correspondence: Ya Huang, hygccw@hhu.edu.cn