- 1State Key Laboratory of Water Environment Simulation, School of Environment, Beijing Normal University, Beijing, China
- 2Key Laboratory for Water and Sediment Sciences, Ministry of Education, School of Environment, Beijing Normal University, Beijing, China
- 3Key Laboratory of City Cluster Environmental Safety and Green Development, Ministry of Education, School of Ecology, Environment and Resources, Guangdong University of Technology, Guangzhou, China
High-strength alterations in the water level due to extreme climate change and increased anthropogenic activities have implications for methane (CH4) and carbon dioxide (CO2) emission variations in shallow lakes. However, the consistency of the carbon emission flux in response to water-level fluctuations and temperature is still unclear. Here, we evaluated the water depth (WD) on the magnitude and variation sensitivity of CH4, CO2, and GHG, and then the temperature dependence of carbon emissions was estimated at different water levels. The water depth threshold indicated a maximum CH4 (97.5 cm) and CO2 (10 cm), resulting in a water depth threshold of GHG at 54.6 cm. Inside the whole WD, the effect of rising water depth on CH4, CO2 and GHG sensitivity shifted from a positive effect to a negative effect at a WD of 97.5 cm. And CH4, CO2 and GHG in 10 cm<WD<97.5 cm show the highest emission flux and sensitivity to varying water depths. Furthermore, a consistency of carbon emission flux responding to water depth and temperature was only found in specific zones of shallow lakes with 10 cm<WD<97.5 cm, indicating that the temperature dependence of CH4 and CO2 are driven by the hydrological regime without water level stress, shifting the GHG emission flux. Ensuring the restoration management goal related to the carbon peak by governing the time of threshold occurrence is essential.
1 Introduction
Hydrological regimes of shallow lake wetlands play a vital role in controlling physiochemical, biological processes and function (Poff et al., 1997; Mitsch and Gosselink, 2015; Hilt et al., 2017; Palmer and Ruhi, 2019), especially in the establishment and maintenance of specific wetland types (Mitsch and Gosselink, 2015; Yang et al., 2020). However, strong alterations in hydrological regimes caused by both extreme climate change and increased anthropogenic activities contribute to the widespread loss and degradation of wetlands globally (Millennium Ecosystem Assessment, 2005; Davidson, 2014; Gardner et al., 2015; Liu et al., 2020a). In the restoration process with water regulation (Jiang et al., 2016; Kong et al., 2017; Moor et al., 2017), the abrupt increase in water level elevation due to ecological water transfer projects and reductions in seasonal water level fluctuations resulting from water level controls inevitably induced changes in biological processes while also influencing carbon C) balances (Martínez-Santos et al., 2008; Kong et al., 2017; Olefeldt et al., 2017).
As indicators of C balances, CH4 and CO2 fluxes are outputs of biological processes related to moisture restriction, and a threshold is proposed while CH4 or CO2 emissions vary with water level according to Shelford’s tolerance law (Shelford, 1913; Shelford, 1931; Odum, 1971; Erofeeva, 2021). Additionally, the threshold is amplified or minimized due to the fluctuating pattern of the water level (e.g., constant non-fluctuation, natural fluctuations driven by normal meteorological factors, and high-strength alterations due to extreme climate change and increased anthropogenic activities) with the same annual or multiyear mean water level.
Shifts in water level lead to threshold variations in CH4 and CO2 and are mainly governed by the transfer between anaerobic methane (CH4) production and aerobic CH4 oxidation processes. Within the optimal threshold range, higher water levels are generally associated with higher net CH4 emissions and less CO2 emissions (Jacinthe, 2015; Li X. et al., 2019; Ye et al., 2019), resulting in uncertainty in total carbon emissions (GHG). The “enzyme latch” theory has been used to clarify wetland C responses to varying water levels (Ise et al., 2008). As shown in a previous study, the reduction of electron acceptor concentrations with water level drawdown alters the accumulation of aromatic solubility and hydrolase activity (Wang et al., 2017), which explains the positive, neutral and negative effects of water level reduction on soil organic carbon (SOC). Additionally, water level fluctuations will alter redox conditions directly, subsequently triggering CH4 and carbon dioxide (CO2) emissions to occur (Granberg et al., 1997; Chimner et al., 2016; Van der Lee et al., 2017) and resulting in relative contribution differences to GHGs.
However, the relative contribution difference of CH4 and CO2 to GHG threshold uncertainty is larger under multiple stressors. Stressor impacts can combine additively or can interact, causing synergistic or antagonistic effects (Simmons et al., 2021). Birk et al. (2020) evaluated the effects in European lakes, and only one of the two stressors had a significant effect of 39%; 28% of the paired-stressor combinations resulted in additive effects, and 33% resulted in interactive effects. Based on metabolic theory, the temperature dependence of carbon emissions is extremely general in wetlands, including shallow lakes. Studies have shown that both CH4 and CO2 show exponential growth within −25°C–35°C (Song et al., 2010; Chen et al., 2021), and temperature dependence is widely found in various wetland types and scales (Yvon-Durocher et al., 2012; Yvon-Durocher et al., 2014; Chen et al., 2021). However, it is unknown whether the GHG threshold uncertainty is amplified or minimized when high-strength water level fluctuations and temperatures act simultaneously.
As the relative contribution variation of CH4 and CO2 depends on the anerobic condition change along with water depth (Van der Lee et al., 2017), the threshold of GHG emissions in shallow lakes is between the thresholds of CH4 and CO2. Additionally, upon Shelford’s tolerance law and metabolic theory, the sensitivity of carbon emissions to varying water depths is related to temperature. Consequently, the objectives of the present study are 1) to explore the response of the characteristics of CH4, CO2 and GHG emissions to water depth; 2) to assess the sensitivity of CH4, CO2 and GHG emissions to varying water depths inside or between WD intervals; and 3) to attribute the effects of water depth changes on the temperature dependence of carbon emissions. To achieve this, we subdivided water level intervals based on turning points found in the response process of CH4 and CO2 to water depth. Additionally, accounting for the ecological response of CO2 and CH4 flux and sensitivity to variations in water depth, we also attempted to extend our results to enrich the general theory as it pertains to shallow lake wetland restoration. In this study, we choose Lake Baiyangdian (BYD) as a case study, using CH4, CO2 and GHG as quantitative indicators of carbon emission magnitude and variation.
2 Materials and methods
2.1 Case study
The BYD (38◦43′to 39◦02′N, 115◦38′to 116◦07′E) is the largest inland freshwater lake-marsh wetland in the North China Plain (Figure 1). It includes 94 km2 of raised fields and greater than 3700 of ditches that subdivide the basin into 140 small shallow lakes, with a surface area of 366 km2 and an average water depth of 2 ± 0.35 m (CCLCAC, 2000). Historically, nine rivers fed the BYD; however, most of these rivers have dried up due to climate changes and increased anthropogenic activities (Liu et al., 2010). Ecosystem processes and functions in the BYD, which is a typical shallow lake for which reeds are the dominant emergent plant, are sensitive to water-level fluctuations, namely, fluctuations related to net primary productivity and organic matter. With a decline in water level, macrophytes, especially reeds, have exhibited a tendency to expand, resulting in terrestrialization in some shallow lakes within the BYD (e.g., Zaozha Lake and Guding Lake) (Cui et al., 2017). To maintain the water ecology and integrity of the BYD, ecological water transfer projects have been implemented since the 1980s to replenish the lake (Wang et al., 2018). Specifically, the planning outline of the Xiong’an New Area, which has jurisdiction over BYD, includes an ordinance for its ecological restoration. Inevitably, under highly intensive anthropogenic activities, hydrological regime alterations have caused aquatic ecosystem changes to occur (Wang et al., 2018; Li Y. L. et al., 2019) and affected the C sequestration capacity of the lake (Li et al., 2009; Chen et al., 2017).
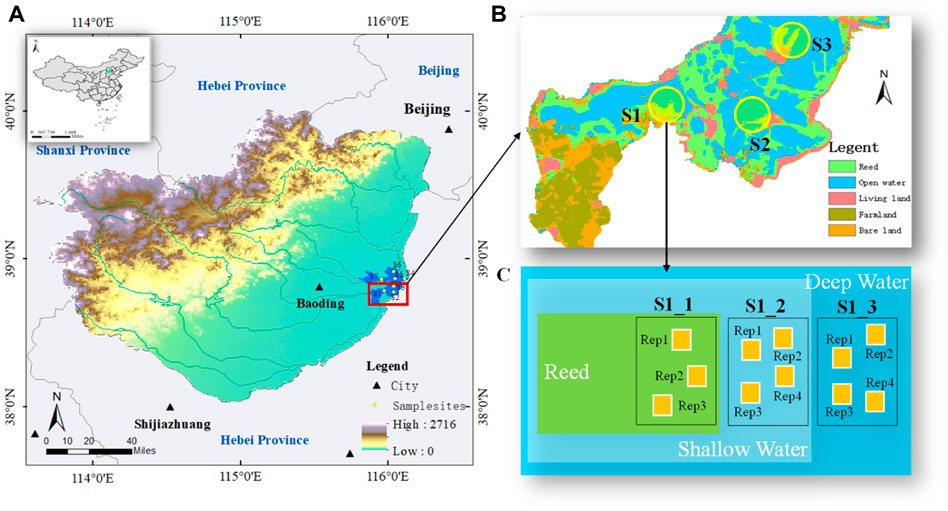
FIGURE 1. Location of Lake Baiyangdian (BYD, Figure 1A), seven sampling strips (Figure 1A; Figure 1B), and repetitions at each sample site (Figure 1C). As shown in Figure 1B, three sample sites were conducted in each sampling strip, where sampling strip S1 is consist of sample sites marked as S1_1, S1_2, and S1_3. And Rep1 to Rep4 showed the repetitions in each sample sites.
Based on our preliminary field investigations, combined with diverse geomorphological, hydrological, and vegetative conditions in the lake, we chose seven sample strips consisting of 21 sample sites (Figure 1A). According to the historical water levels from 1960 to 2019 (Supplementary Figure S1), 21 sample sites were examined considering the annual water depth. As shown in Figures 1B, C, three sample sites were conducted in each sampling strip, where sampling strip S1 consists of sample sites marked as S1_1, S1_2, and S1_3. Triplicates or four repetitions were set for sample sites with water depths of negative and positive, respectively, according to the spatial heterogeneity of terrain and biological factors (Figure 1C). We performed five field surveys: in June, October, and December 2020, and in February and April 2021. We simultaneously tested the water depth (WD) and temperatures of water (T_Water), air (T_Air) and chamber (T_cham) for each field survey. In above, we obtained 105 couples of data for further analysis. And the water depth involved in all sampling sites ranged from -1.10 m to 4.20 m.
2.2 Greenhouse gas and environmental factor measurements
2.2.1 Greenhouse gas collection and measurement
The in situ CH4 and CO2 emissions were measured with the static opaque chamber and gas chromatography technique (Zhang et al., 2020; Gao et al., 2022). The fluxes of CH4 and CO2 were measured simultaneously with the collection of surface water and local ambient air samples. At each site, four floating chambers were deployed from the open water alongside the zone of emergent vegetation. These chambers were of the same size and shape and streamlined with a flexible plastic foil collar to minimize the effects of chamber-induced turbulence when measuring fluxes. Each chamber was also covered with aluminum foil to reflect sunlight and minimize internal heating. Chambers were allowed to drift, and each chamber measurement lasted for 60–80 min. After mixing the contents of the chambers three times, 50 mL of gas was extracted from the chambers and transferred to airtight gas sampling bags at 0, 5, 10, 20, 40, 60, and 80 min. This multi-chamber method and prolonged deployment not only increased the probability of capturing ebullition but also incorporated spatiotemporal variability in both diffusion and ebullition within and among streams and rivers. The concentrations of CH4 and CO2 in the gas samples were determined as described above, and CH4 and CO2 fluxes were calculated based on the closed-chamber technique (Yuan et al., 2021).
2.2.2 Meteorological and soil physiochemical property measurements
The water depth, air temperature, temperature in the chamber, and soil temperature at a depth of 5 cm were simultaneously measured in situ while gas samples were collected. For samples in deep water and shallow water, a portable water quality analyzer (Hach H40 d) was used to simultaneously monitor water temperature, pH, dissolved oxygen (DO), and redox potential (Eh).
2.3 Evaluation of carbon emission flux in response to water depth
2.3.1 Assessment of the water depth threshold by a piecewise regression model
As the carbon emission fluxes showed significant piecewise regression varying with water depth, a piecewise regression model was applied to the carbon emission flux series to detect the turning points for CH4 flux, CO2 flux and GHG varying with water depth (Toms and Lesperanc, 2003; Yang et al., 2017):
where t is the water depth; y is the carbon emission flux; β0, β1 and β2 are regression coefficients; and α is the assumed turning point, which was determined based on carbon emission flux analysis. The range of the α value was set to be the water depth when β1 and β2 were found to be different. Least squares linear regression was used to estimate the three regression coefficients, and a t-test was applied to test if β2 was not equal to zero.
2.3.2 Sensitivity of carbon emission flux to water depth
To evaluate the sensitivity and final effect of water depth on CH4, CO2 and GHG sensitivity, we compare the magnitude of emission sensitivity in all WD intervals with a baseline of average water depth of all sites. The carbon emission flux sensitivity to water depth (ΔC, WD) is equal to the difference between the CH4, CO2 and GHG emission fluxes at specific water depths and the average water depth. ΔC, WD is quantified as follows:
where
2.4 Dependence of carbon emission flux on temperature
To exclude the independence between water depth and temperature, we tested the correlation between each pair of variables. The correlation between temperature and water depth in all WD intervals is identified, and pairs of data with significant correlations are removed. The apparent activation energy was selected as the characterization index of the temperature dependence of carbon emission. The temperature-dependent quantification of CH4 and CO2 emissions is based on the Boltzmann-Arrhenius function of the form (Jaap van der Meer, 2006; Price et al., 2010; Chen et al., 2021):
where lnRi(T) represents the natural logarithm of the CH4 or CO2 emission flux at any location i at the absolute temperature T (K);
2.5 Statistical analysis
The spatiotemporal differences in CH4 and CO2 emission fluxes and their significance were characterized using analysis of variance (ANOVA). All variables were tested for homogeneity of variance and normal distribution. For the variable data satisfying the normal distribution, the Pearson correlation coefficient was used for analysis; otherwise, the Spearman correlation coefficient was used for correlation analysis. SPSS 22.0 was used to construct a linear mixed model and quantify the temperature dependence. Then, the slope and intercept are treated as random variables according to the mean of
3 Results
3.1 Carbon emission flux varying with water depth
The CH4, CO2 and GHG fluxes varied with water depth, and turning points were found at 97.5 cm, 10 cm and 54.6 cm (water depth threshold, WDT), respectively. As shown in Figure 2, CH4 and CO2 fluxes increased when the water depth was less than the WDT, while decreasing trends were shown when the water depth was larger than the WDT. CH4 flux and CO2 flux ranged from −0.07 mg CO2-eq/m2/h to 8.32 mg CO2-eq/m2/h and −4.76 mg CO2/m2/h to 196.52 mg CO2/m2/h, respectively, with a higher absolute value of the regression slope at water depths of -97.5 cm–54.6 cm. The regression slopes of CH4 and CO2 are 0.018 and −0.008 mg CO2-eq/m2/h/cm, respectively, and 0.558 and −0.162 mg CO2-eq/m2/h/cm, respectively, when the water depth is lower and higher than the WDT.
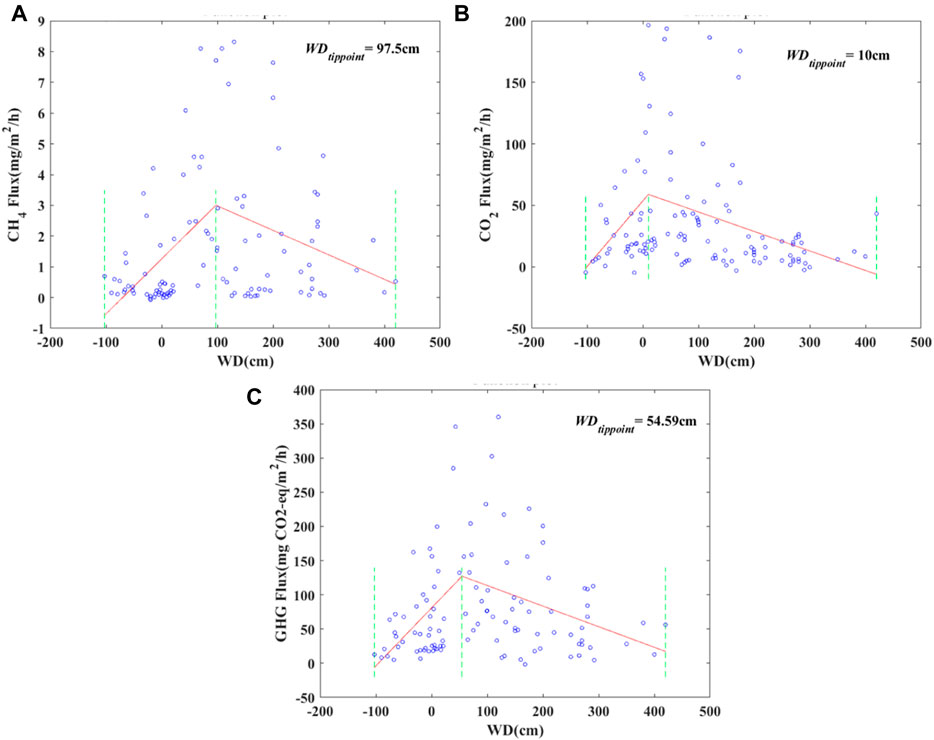
FIGURE 2. Characteristics of carbon fluxes varying with water depth, where CH4, CO2 and GHG are shown in Figure 2A, Figure 2B, and Figure 2C, respectively.
Additionally, The GHG varied between −1.70 mg CO2-eq/m2/h and 360.02 mg CO2-eq/m2/h, showing a similar pattern in the regression slope with CH4 and CO2 fluxes. And the corresponding regression slopes of GHG are 0.848 and −0.301 mg CO2-eq/m2/h/cm, respectively. Moreover, the turning point of GHG in response to water depth is lower than that of CH4 and higher than that of CO2.
3.2 Sensitivity of carbon emission flux varying with water depth
Both CH4 and CO2 show the highest sensitivity in 10 cm<WD<97.5 cm, and the sensitivities are 4.89 mg CO2-eq/m2/h/cm and 4.01 mg CO2/m2/h/cm, respectively (Figure 3A). The medium and lowest sensitivity of CH4 and CO2 occurred when WD<10 cm and WD>97.5 cm, respectively. The sensitivities are 0.52 mg CO2-eq/m2/h/cm and −0.30 mg CO2-eq/m2/h/cm for CH4 emissions varying with water depths of WD<10 cm and WD>97.5 cm, and the sensitivity of CO2 emissions in the corresponding range of water depths is 0.16 mg CO2/m2/h/cm and −0.04 mg CO2/m2/h/cm. Additionally, GHG shows similar results when comparing the magnitude of sensitivity in focused water depth. The sensitivity is 4.51 mg CO2-eq/m2/h/cm, 0.35 mg CO2-eq/m2/h/cm and −0.18 mg CO2-eq/m2/h/cm, where we evaluate the magnitude of the sensitivity on its absolute values.
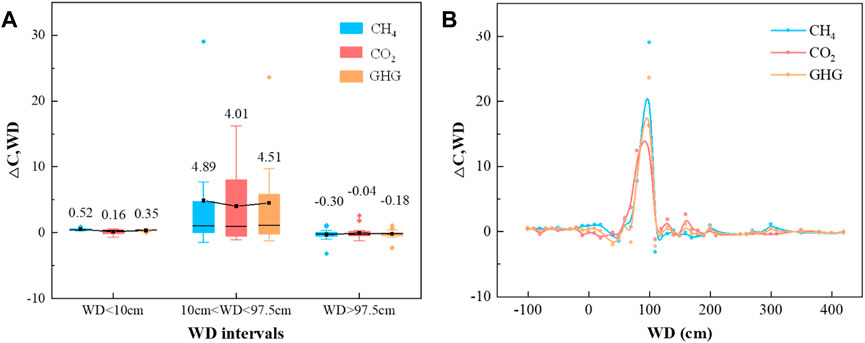
FIGURE 3. Carbon emission sensitivity varying with water depth. Figure 3A shows the average sensitivity of CH4, CO2 and GHG in each focused WD interval, and the corresponding emission sensitivity at each water depth is described in Figure 3B.
For the whole water depth of −110 cm–420 cm, CH4, CO2 and GHG sensitivity shows a consistent trend with water depth elevation (Figure 3B). Flux sensitivity increased when the water depth was less than the WDT of CH4 (97.5 cm), followed by a declining trend when WD>97.5, and sensitivity remained relatively low at WD>200 cm. The elevation of water depth, from WD<10 cm–10 cm<WD<97.5 cm, shows a positive effect on the CH4, CO2 and GHG sensitivities, promoting by 8.48, 23.86 and 11.76 times, respectively. However, CH4, CO2 and GHG sensitivities were reduced by nearly 1-fold (0.94–0.99) when the mean water depth was further elevated to WD>97.5 cm.
3.3 Temperature dependence of CH4 and CO2 varying with water depth
The results of the correlation analysis of carbon emissions with water depth and temperature showed that CH4 and CO2 emission fluxes were correlated with both water depth and temperature (Table 1). Among them, CH4 was significantly positively correlated with water depth (p<0.01, r=0.267), CO2 was significantly negatively correlated with water depth (p<0.01, r=-0.419), CH4 was positively correlated with temperature (p<0.01, 0.427<r<0.565), and the positive correlation between CO2 and temperature did not reach a significant level (0.071<p<0.253, -0126<r<0.157). In addition, there is no correlation between temperature changes and water depth changes during the sampling period (0.341<p<0.928); that is, in the paired analysis data involved in this study, the change law of carbon emission flux with water depth is not affected by temperature.
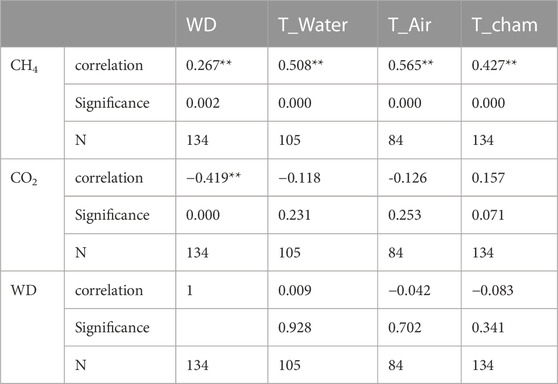
TABLE 1. Correlations of CH4 and CO2 with water depth and temperature, where * and ** showed the significant difficance at p < .05 and p < .01.
The CH4 flux shows a higher temperature dependence than the CO2 flux, where EM and EC for all water depths are 0.69 eV and 0.35 eV (Figure 4), respectively. For the three WD intervals, EM and EC showed similar results in 10 cm<WD<97.5 cm and WD>97.5 cm, and EM was 114.29% and 204.35% higher than EC. As shown in Figure 5, EM and EC in WD<10 cm are 0.42 eV and 0.40 eV, respectively, which are almost the same. The maximum temperature dependence between CH4 and CO2 was found at WD>97.5 cm.
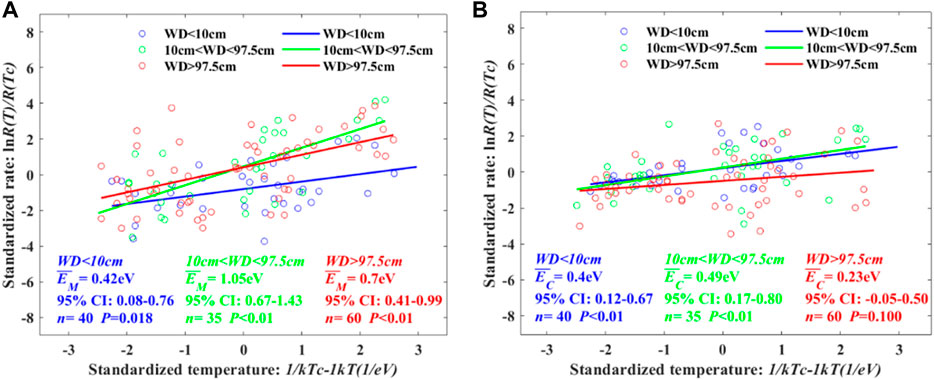
FIGURE 5. Temperature dependence of CH4 and CO2 varying with water depth. We evaluated the average temperature dependence at all water depths and separately WD intervals divided by turning points of CH4 and CO2 varying with water depth. The temperature independence of CH4 in WD<10 cm, 10 cm<WD<97.5 cm, and WD>97.5 cm is shown in Figure 5A. The corresponding value of CO2 is described in Figure 5B.
Both CH4 and CO2 temperature dependence show non-monotonic increasing or decreasing trends with water depth elevation or drawdown. For CH4 temperature dependence in the specific WD intervals, the highest value occurred in 10 cm<WD<97.5 cm, where EM is 1.05 eV (Table 2). The CH4 temperature dependence of WD>97.5 cm is higher than that of WD<10 cm, where EM is 0.70 eV and 0.42 eV, respectively. Additionally, CO2 temperature dependence indicates the highest EC in WD 10 cm<WD<97.5 cm, while the EC is lower in WD>97.5 cm than in WD<10 cm.
4 Discussion
4.1 Fluxes and variation sensitivity of carbon emission flux to varying water depth
Turing points at water depths of 97.5 cm and 10 cm for CH4 and CO2, respectively, indicate a probable threshold, while the carbon emission flux varies with the fluctuating water level. Redox condition alteration resulting in microbial tolerance and enzymatic activity is one of the main causes (Chimner et al., 2016; Van der Lee et al., 2017). The elevation of water depth increases the ratio of anaerobic microorganisms to aerobic microorganisms, while the overall microbial activity decreases, leading to higher CH4 emissions and less CO2 emissions (Jacinthe, 2015; Mader et al., 2017; Li X. et al., 2019; Ye et al., 2019). Additionally, the competition among electron acceptors alters oxidative pathways under oxygen-limited conditions. Recently, Wang et al. (2017) reported that ferrous iron [Fe(II)] has been shown to decrease with a decrease in water levels while also acting as a controlling factor of oxidative phenolic activity. Then, the difference between the relative contributions of CH4 and CO2 resulted in a turning point of final GHG emissions at a water depth of 54.6 cm (Holgerson and Raymond, 2016; Zhang et al., 2017; Chen et al., 2021; Huang et al., 2021).
In the range of 10 cm–97.5 cm, CH4, CO2 and GHG showed the highest emission flux and sensitivity to varying water depths. As previous studies have shown, emergent vegetation, as part of biological progress, plays a vital role in gas transport from sediment to air and dissolved oxygen changes due to and resistance to hydrodynamic disturbances such as wind and waves in shallow lakes (DelSontro et al., 2018; Tang et al., 2019; Ran et al., 2022). Along the inner range, CH4 and CO2 sensitivity increased with the water depth, indicating a positive effect on GHG sensitivity before the water depth reached the tolerance value. As shown in Figures 3A, B positive effect on GHG sensitivity existed in the range of water depths between 10 cm and 97.5 cm, showing that the minimum and maximum tolerance values were out of 10 cm<WD<200 cm. In this study, the sensitivities of CH4, CO2 and GHG remained at a low value when the water depth was >200 cm, which also indicated that with a further increase in water depth, the changes in carbon emissions were small.
Therefore, the zone (Z) with a water depth range varying from 10 cm to 97.5 cm may be a more critical zone for reducing carbon emissions through water level control in shallow lakes. Firstly, the highest emission flux reveled that the maximum emission reduction potential can be obtained by controlling the distribution area of Z. Because the potential carbon emission in Z is larger when the area is the same with other zones, and its contribution to the whole lake is greater with increased distribution of Z. Secondly, higher sensitivity to fluctuating water depths indicated that the emission reduction control efficiency is higher with lower cost in management practice, as that increase or decrease amplitude of carbon emission flux in Z, with per unit water depth variation, is larger than other zones. Finally, regulating carbon emissions in Z through adjusting the micro-topography and vegetation distribution is scientific and operable in manage practice (Tang et al., 2019; Liu et al., 2020b), as the crucial role of emergent vegetation and submerged vegetation of Z in carbon emissions by controlling the physical condition and water use (DelSontro et al., 2018; Tang et al., 2019; Ran et al., 2022).
4.2 Temperature dependence of CH4 and CO2 varying with water depth
Based on Shelford’s tolerance law (Shelford, 1913; Shelford, 1931; Odum, 1971; Erofeeva, 2021) and metabolic theory (Price et al., 2010), which state that the sensitivity of carbon emissions to varying water depths is temperature-dependent (Chen et al., 2021), this study proved it in specific zones of shallow lakes with water depths varying from 10 cm to 97.5 cm. This study revealed a consistency of carbon emission flux in response to water depth and temperature. CH4, CO2 and GHG fluxes show the highest sensitivity to water depth in 10 cm<WD<97.5 cm, and both EM and EC are higher than those in other WD intervals. This may be attributed to water depth shifting carbon emissions by adjusting temperature dependence or to additive and interactive effects between water depth and temperature (Birk et al., 2020; Simmons et al., 2021). However, the assumption was not totally verified in WD<10 cm and WD>97.5 cm.
In shallow lakes, for WD<10 cm, dramatic decreasing or elevating water depth shifts anaerobic conditions, and the anaerobic conditions are even broken due to the surface soil being exposed to air (Van der Lee et al., 2017; Jane et al., 2021). Based on the limiting factor principle and metabolic theory, the limitation of water depth restricts the metabolism of organisms related to CH4 and CO2, resulting in low temperature dependence and higher sensitivity of CH4 to water depth (Price et al., 2010; Yvon-Durocher et al., 2014). As shown in this study, the temperature dependence of CH4 emissions is lowest at WD<10 cm, and EM is 0.42 eV. However, the difference in CH4 and CO2 sensitivity was maximum (108.99%) in this WD interval, and the sensitivity of CH4 (0.52) to varying water depths was lower than 10 cm<WD<97.5 cm. Additionally, the elevated water depth indicating higher CH4 and CO2 sensitivity was additional evidence when WD<10 cm, as shown in Figure 3B.
The pattern of temperature dependence is not totally consistent with the assumption when WD>97.5 cm. Based on this assumption, water depth shifts carbon emissions by adjusting the temperature dependence, EM and EC should be higher accordingly when the sensitivity of CH4, CO2 and GHG of WD>10 cm is higher than that of WD<97.5 cm. However, compared with WD<10 cm, EM is indeed increased by 66.67%, while EC is reduced by -42.50%. Additionally, in WD>97.5 cm, the difference between EC and EM reaches a maximum (204.35%), and EC is lower than the mean value (0.35 eV) at all water depths. Previous studies have shown that CO2 is an important indicator to characterize the overall activity from microorganisms to ecosystem scales (Price et al., 2010; Yvon-Durocher et al., 2012). A higher water depth may result in an overall metabolic level decline, excluding anaerobic microorganisms correlated with CH4 (Vicca et al., 2009; Chen et al., 2020).
Above all, there is a specific water depth scope (Figure 6), such as 10 cm<WD<97.5 cm, ensured by the turning point of CH4 and CO2, where the temperature dependence of CH4 and CO2 is driven by water depth, shifting the GHG emission flux. Additionally, in water-limited conditions and oversaturated conditions with much higher water levels, the impact of temperature on the magnitude and sensitivity of carbon flux is also restricted.
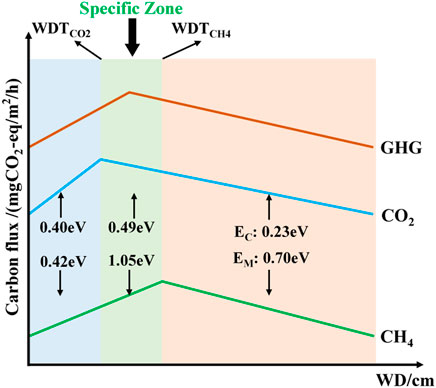
FIGURE 6. Relationship comparison of carbon emissions varying with water depth and carbon emissions responding to temperature.
5 Conclusion
Based on the limiting factor principle of Shelford’s tolerance law and metabolic theory, we explore the threshold while the GHG emission flux varies with water depth in shallow lakes and try to clarify whether the sensitivity of carbon emissions to different water depths is related to temperature. We found that the water depth threshold indicates a maximum CH4 (97.5 cm) and CO2 (10 cm), resulting in a water depth threshold of GHG at 54.6 cm. Inter WD intervals, CH4, CO2 and GHG in 10 cm<WD<97.5 cm showed the highest emission flux and sensitivity to varying water depths. In the inner WD intervals, the effect of increasing water depth on CH4, CO2 and GHG shifted from a positive effect to a negative effect at a WD of 97.5 cm CH4 and CO2 sensitivity increase with water depth elevation when WD<97.5 cm, while CH4 and CO2 sensitivity show a decreasing trend when WD>97.5 cm. Furthermore, a consistency of carbon emission flux responding to water depth and temperature is only found in specific zones of shallow lakes with 10 cm<WD<97.5 cm, indicating that the temperature dependence of CH4 and CO2 are driven by the hydrological regime without water level stress, shifting the GHG emission flux. Linking the result to restoration in shallow lakes, water level control considering seasonal effects on carbon emission reduction is essential. We propose an advice to ensure the goal by governing the time that a turning point occurs and the control costs upon the sensitivity of carbon emissions to unit water depth variation. As we combined samples from multiple dates in this study, it is difficult to clarify short time response and variation of environmental factors, which significantly impacting carbon process. And more sample sites with higher temporal or spatial resolution will improve our understanding of these variations in future research.
Data availability statement
The raw data supporting the conclusions of this article will be made available by the authors. Further inquiries can be directed to the corresponding author.
Author contributions
XY: Methodology, Investigation, Writing–original draft, Writing–review and editing. QL: Conceptualization, Supervision, Funding acquisition, Writing–review. SL: Methodology, Investigation, Writing–original draft. BC: Methodology, Conceptualization. WY: Methodology, Writing–review. TS: Methodology, Conceptualization. XW: Methodology, Investigation. CL: Methodology, Writing–review. YC: Methodology, Conceptualization. ML: Investigation, Visualization. JZ: Methodology, Investigation.
Funding
This study was financially supported by the National Natural Science Foundation of China (No. 42071129, 42271141), and the National Key R&D Program of China (No. 2022YFF1300902).
Conflict of interest
The authors declare that the research was conducted in the absence of any commercial or financial relationships that could be construed as a potential conflict of interest.
Publisher’s note
All claims expressed in this article are solely those of the authors and do not necessarily represent those of their affiliated organizations, or those of the publisher, the editors and the reviewers. Any product that may be evaluated in this article, or claim that may be made by its manufacturer, is not guaranteed or endorsed by the publisher.
Supplementary material
The Supplementary Material for this article can be found online at: https://www.frontiersin.org/articles/10.3389/feart.2022.1086072/full#supplementary-material
Supplementary Figure S1 | Annual mean state of the spatial distribution (Figure S1A) and frequency distribution (Figure S1B) of water depth from 1960 to 2019, and frequency distribution (Figure S1C) of sample sites water depth in field surveys.
References
Birk, S., Chapman, D., Carvalho, L., Spears, B. M., Andersen, H. E., Argillier, C., et al. (2020). Impacts of multiple stressors on freshwater biota across spatial scales and ecosystems. Nat. Ecol. Evol. 4, 1060–1068. doi:10.1038/s41559-020-1216-4
CCLCAC (2000). Compilation committee of Local chronicles of Anxin county. Beijing: Xinhua Publishing HouseAnxin county Annals.
Chen, H., Xu, X., Fang, C., Li, B., and Nie, M. (2021). Differences in the temperature dependence of wetland CO2 and CH4 emissions vary with water table depth. Nat. Clim. Chang. 11, 766–771. doi:10.1038/s41558-021-01108-4
Chen, H., Zhu, T., Li, B., Fang, C., and Nie, M. (2020). The thermal response of soil microbial methanogenesis decreases in magnitude with changing temperature. Nat. Commun. 11, 5733. doi:10.1038/s41467-020-19549-4
Chen, X., Wang, F., Lu, J., Li, H., Zhu, J., and Lv, X. (2017). Simulation of the effect of artificial water transfer on carbon stock of phragmites australis in the baiyangdian wetland, China. Scientifica 2017, 1–11. doi:10.1155/2017/7905710
Chimner, R. A., Pypker, T. G., Hribljan, J. A., Moore, P. A., and Waddington, J. M. (2016). Multi-decadal changes in water table levels alter peatland carbon cycling. Ecosystems 20 (5), 1042–1057. doi:10.1007/s10021-016-0092-x
Cui, B. S., Han, Z., Li, X., Lan, Y., Bai, J. H., Cai, Y. Z., et al. (2017). Driving mechanism and regulating measures for lake terrestrialization: A case of Lake Baiyangdian. China: Science Press. (In Chinese with English abstract).
Davidson, N. C. (2014). How much wetland has the world lost? Long-term and recent trends in global wetland area. Mar. Freshw. Res. 65, 934–941. doi:10.1071/MF14173
DelSontro, T., del Giorgio, P. A., and Prairie, Y. T. (2018). No longer a paradox: The interaction between physical transport and biological processes explains the spatial distribution of surface water methane within and across lakes. Ecosystems 21, 1073–1087. doi:10.1007/s10021-017-0205-1
Erofeeva, E. A. (2021). Plant hormesis and Shelford’s tolerance law curve. J. For. Res. 32, 1789–1802. doi:10.1007/s11676-021-01312-0
Evans, C. D., Peacock, M., Baird, A. J., Artz, R. R. E., Burden, A., Callaghan, N., et al. (2021). Overriding water table control on managed peatland greenhouse gas emissions. Nature 593, 548–552. doi:10.1038/s41586-021-03523-1
Gao, W., Gao, D., Cai, T., and Liang, H. (2022). Driving factors on greenhouse gas emissions in permafrost region of Daxing'an Mountains, northeast China. J. Geophys. Res. Biogeosciences 127, e2021JG006581. doi:10.1029/2021JG006581
Gardner, R. C., Barchiesi, S., Beltrame, C., Finlayson, C. M., Galewski, T., Harrison, I., et al. (2015). State of the world's wetlands and their services to people: A compilation of recent analyses. Brief. Note, 7. doi:10.2139/ssrn.2589447 no.
Granberg, G., Mikkela, C., Sundh, I., Svensson, B. H., and Nilsson, M. (1997). Sources of spatial variation in methane emission from mires in northern Sweden: A mechanistic approach in statistical modeling. Glob. Biogeochem. Cycles 11 (2), 135–150. doi:10.1029/96GB03352
Hilt, S., Brothers, S., Jeppesen, E., Veraart, A. J., and Kosten, S. (2017). Translating regime shifts in shallow lakes into changes in ecosystem functions and services. BioScience 67 (10), 928–936. doi:10.1093/biosci/bix106
Holgerson, M. A., and Raymond, P. A. (2016). Large contribution to inland water CO2 and CH4 emissions from very small ponds. Nat. Geosci. 9 (3), 222–226. doi:10.1038/ngeo2654
Huang, Y., Ciais, P., Luo, Y., Zhu, D., Wang, Y., Qiu, C., et al. (2021). Tradeoff of CO2 and CH4 emissions from global peatlands under water-table drawdown. Nat. Clim. Chang. 11, 618–622. doi:10.1038/s41558-021-01059-w
Ise, T., Dunn, A. L., Wofsy, S. C., and Moorcroft, P. R. (2008). High sensitivity of peat decomposition to climate change through water-table feedback. Nat. Geosci. 1 (11), 763–766. doi:10.1038/ngeo331
Jaap van der Meer, J. (2006). Metabolic theories in ecology. Trends Ecol. Evol. 21 (3), 136–140. doi:10.1016/j.tree.2005.11.004
Jacinthe, P. A. (2015). Carbon dioxide and methane fluxes in variably-flooded riparian forests. Geoderma 241–242, 41–50. doi:10.1016/j.geoderma.2014.10.013
Jane, S. F., Hansen, G. J. A., Kraemer, B. M., Leavitt, P. R., Mincer, J. L., North, R. L., et al. (2021). Widespread deoxygenation of temperate lakes. Nature 594, 66–70. doi:10.1038/s41586-021-03550-y
Jiang, H. B., Wen, Y., Zou, L. F., Wang, Z., He, C., and Zou, C. (2016). The effects of a wetland restoration project on the Siberian crane (Grus leucogeranus) population and stopover habitat in Momoge National Nature Reserve, China. Ecol. Eng. 96, 170–177. doi:10.1016/j.ecoleng.2016.01.016
Kim, D. G., Vargas, R., Bond-Lamberty, B., and Turetsky, M. R. (2012). Effects of soil rewetting and thawing on soil gas fluxes: A review of current literature and suggestions for future research. Biogeosciences 9 (7), 2459–2483. doi:10.5194/bg-9-2459-2012
Kong, X., He, Q., Yang, B., He, W., Xu, F., Janssen, A. B. G., et al. (2017). Hydrological regulation drives regime shifts: Evidence from paleolimnology and ecosystem modeling of a large shallow Chinese lake. Glob. Change Biol. 23 (2), 737–754. doi:10.1111/gcb.13416
Li, B., Liu, C. Q., Wang, J. X., and Zhang, Y. J. (2009). Carbon storage and fixation function by phragmites australis, a typical vegetation in baiyangdian lake. J. Agro-environment Sci. 28 (12), 2603–2607. (in Chinese with an English abstract).
Li, X., Yang, W., Sun, T., and Su, L. (2019a). Framework of multidimensional macrobenthos biodiversity to evaluate ecological restoration in wetlands. Environ. Res. Lett. 14 (5), 054003. doi:10.1088/1748-9326/ab142c
Li, Y. L., Zhang, Q., Cai, Y. J., Tan, Z., Wu, H., Liu, X., et al. (2019b). Hydrodynamic investigation of surface hydrological connectivity and its effects on the water quality of seasonal lakes: Insights from a complex floodplain setting (Poyang Lake, China). Sci. Total Environ. 660, 245–259. doi:10.1016/j.scitotenv.2019.01.015
Liu, F., Liu, J. L., Zhang, T., and Chen, Q. Y. (2010). Land use change and its effects on water quality in Baiyangdian Lake of North China during last 20 years. J. Agro-environment Sci. 10, 1868–1875. (in Chinese with an English abstract).
Liu, Q., Liu, J. L., Liu, H. F., Liang, L., Cai, Y., Wang, X., et al. (2020b). Vegetation dynamics under water-level fluctuations: Implications for wetland restoration. J. Hydrology 581, 124418. doi:10.1016/j.jhydrol.2019.124418
Liu, Q., Ma, X. J., Yan, S. R., Liang, L., Pan, J., and Zhang, J. (2020a). Lag in hydrologic recovery following extreme meteorological drought events: Implications for ecological water requirements. Water 12 (3), 837. doi:10.3390/w12030837
Mader, M., Schmidt, C., van Geldern, R., and Barth, J. A. (2017). Dissolved oxygen in water and its stable isotope effects: A review. Chem. Geol. 473, 10–21. doi:10.1016/j.chemgeo.2017.10.003
Martínez-Santos, P., de Stefano, L., Llamas, M. R., and Martinez-Alfaro, P. E. (2008). Wetland restoration in the mancha Occidental aquifer, Spain: A critical perspective on water, agricultural, and environmental policies. Restor. Ecol. 16 (3), 511–521. doi:10.1111/j.1526-100X.2008.00410.x
Mitsch, W. J., and Gosselink, J. G. (2015). Wetlands. fifth ed. Hoboken, New Jersey: John Wiley & Sons, 747.
Moor, H., Rydin, H., Hylander, K., Nilsson, M. B., Lindborg, R., and Norberg, J. (2017). Towards a trait-based ecology of wetland vegetation. J. Ecol. 105 (6), 1623–1635. doi:10.1111/1365-2745.12734
Olefeldt, D., Euskirchen, E. S., Harden, J., Kane, E., McGuire, A. D., Waldrop, M. P., et al. (2017). A decade of boreal rich fen greenhouse gas fluxes in response to natural and experimental water table variability. Glob. change Biol. 23 (6), 2428–2440. doi:10.1111/gcb.13612
Palmer, M., and Ruhi, A. (2019). Linkages between flow regime, biota, and ecosystem processes: Implications for river restoration. Science 365 (6459), eaaw2087. doi:10.1126/science.aaw2087
Poff, N. L., Allan, J. D., Bain, M. B., Karr, J. R., Prestegaard, K. L., Richter, B. D., et al. (1997). The natural flow regime: A paradigm for river conservation and restoration. Bioscience 47, 769–784. doi:10.2307/1313099
Price, C. A., Gilooly, J. F., Allen, A. P., Weitz, J. S., and Niklas, K. J. (2010). The metabolic theory of ecology: Prospects and challenges for plant biology. New Phytol. 188 (3), 696–710. doi:10.1111/j.1469-8137.2010.03442.x
Ran, L., Wang, X., Li, S., Zhou, Y., Xu, Y., Chan, C. N., et al. (2022). Integrating aquatic and terrestrial carbon fluxes to assess the net landscape carbon balance of a highly erodible semiarid catchment. J. Geophys. Res. Biogeosciences 127, e2021JG006765. doi:10.1029/2021JG006765
Shelford, V. E. (1913). Animal communities in a temperate America. Chicago: University of Chicago Press, 386.
Simmons, B. I., Blyth, P. S. A., Blanchard, J. L., Clegg, T., Delmas, E., Garnier, A., et al. (2021). Refocusing multiple stressor research around the targets and scales of ecological impacts. Nat. Ecol. Evol. 5, 1478–1489. doi:10.1038/s41559-021-01547-4
Song, C., Xu, X. F., Tian, H., and Wang, Y. (2010). Ecosystem–atmosphere exchange of CH4 and N2O and ecosystem respiration in wetlands in the Sanjiang plain, Northeastern China. Glob. Change Biol. 15 (3), 692–705. doi:10.1111/j.1365-2486.2008.01821.x
Tang, C., Lei, J., and Nepf, H. M. (2019). Impact of vegetation-generated turbulence on the critical, near bed, wave-velocity for sediment resuspension. Water Resour. Res. 55 (7), 5904–5917. doi:10.1029/2018WR024335
Toms, J. D., and Lesperanc, M. L. (2003). Piecewise regression: A tool for identifying ecological thresholds. Ecology 84, 2034–2041. doi:10.1890/02-0472
Van der Lee, G. H., Kraak, M. H. S., Verdonschot, R. C. M., Vonk, J. A., and Verdonschot, P. F. M. (2017). Oxygen drives benthic-pelagic decomposition pathways in shallow wetlands. Sci. Rep. 7 (1), 15051. doi:10.1038/s41598-017-15432-3
Vicca, S., Janssens, I. A., Flessa, H., Fiedler, S., and Jungkunst, H. F. (2009). Temperature dependence of greenhouse gas emissions from three hydromorphic soils at different groundwater levels. Geobiology 7, 465–476. doi:10.1111/j.1472-4669.2009.00205.x
Wang, K. L., Li, H. T., Wu, A. M., Li, M. Z., Zhou, Y., and Li, W. P. (2018). An analysis of the evolution of Baiyangdian wetlands in Hebei Province with artificial recharge. Acta Geol. Sin. 2018 (5), 549–558. doi:10.3975/cagsb.2018.071102
Wang, W., Sardans, J., Wang, C., Zeng, C., Tong, C., Bartrons, M., et al. (2018). Shifts in plant and soil C, N and P accumulation and C:N:P stoichiometry associated with flooding intensity in subtropical estuarine wetlands in China. Coast. Shelf Sci. 215, 172–184. doi:10.1016/j.ecss.2018.09.026
Wang, Y., Chen, M. J., Yan, L., Yang, G., Ma, J., and Deng, W. (2019). Quantifying threshold water tables for ecological restoration in arid northwestern China. Groundwater 58 (1), 132–142. doi:10.1111/gwat.12934
Wang, Y., Wang, H., He, J. S., and Feng, X. (2017). Iron-mediated soil carbon response to water-table decline in an alpine wetland. Nat. Commun. 8, 15972. doi:10.1038/ncomms15972
Yang, W., Xu, M. X., Li, R. Q., Zhang, L., and Deng, Q. (2020). Estimating the ecological water levels of shallow lakes: A case study in tangxun lake, China. Sci. Rep. 10, 5637. doi:10.1038/s41598-020-62454-5
Yang, Y., McVicar, T. R., Donohue, R. J., Zhang, Y., Roderick, M. L., Chiew, F. H., et al. (2017). Lags in hydrologic recovery following an extreme drought: Assessing the roles of climate and catchment characteristics. Water Resour. Res. 53, 4821–4837. doi:10.1002/2017WR020683
Ye, X. C., Meng, Y. K., Xu, L. G., and Xu, C. y. (2019). Net primary productivity dynamics and associated hydrological driving factors in the floodplain wetland of China’s largest freshwater lake. Sci. Total Environ. 659, 302–313. doi:10.1016/j.scitotenv.2018.12.331
Yuan, X., Liu, Q., Cui, B. S., Xu, X., Liang, L., Sun, T., et al. (2021). Effect of water-level fluctuations on methane and carbon dioxide dynamics in a shallow lake of Northern China: Implications for wetland restoration. J. Hydrology 597, 126169. doi:10.1016/j.jhydrol.2021.126169
Yvon-Durocher, G., Allen, A., Bastviken, D., Conrad, R., Gudasz, C., St-Pierre, A., et al. (2014). Methane fluxes show consistent temperature dependence across microbial to ecosystem scales. Nature 507, 488–491. doi:10.1038/nature13164
Yvon-Durocher, G., Caffrey, J., Cescatti, A., Dossena, M., Giorgio, P. d., Gasol, J. M., et al. (2012). Reconciling the temperature dependence of respiration across timescales and ecosystem types. Nature 487, 472–476. doi:10.1038/nature11205
Zhang, L., Xia, X., Liu, S., Zhang, S., Li, S., Wang, J., et al. (2020). Significant methane ebullition from alpine permafrost rivers on the East Qinghai–Tibet Plateau. Nat. Geosci. 13, 349–354. doi:10.1038/s41561-020-0571-8
Keywords: fluctuating water-level, carbon flux sensitivity, temperature dependence, shallow lakes, the lake Baiyangdian
Citation: Yuan X, Liu Q, Li S, Cui B, Yang W, Sun T, Wang X, Li C, Cai Y, Li M and Zhou J (2023) Water level fluctuation controls carbon emission fluxes in a shallow lake in China. Front. Earth Sci. 10:1086072. doi: 10.3389/feart.2022.1086072
Received: 19 November 2022; Accepted: 23 December 2022;
Published: 10 January 2023.
Edited by:
Zhenliang Yin, Northwest Institute of Eco-Environment and Resources (CAS), ChinaReviewed by:
Meng Zhu, Northwest Institute of Eco-Environment and Resources (CAS), ChinaXiaoya Deng, China Institute of Water Resources and Hydropower Research, China
Copyright © 2023 Yuan, Liu, Li, Cui, Yang, Sun, Wang, Li, Cai, Li and Zhou. This is an open-access article distributed under the terms of the Creative Commons Attribution License (CC BY). The use, distribution or reproduction in other forums is permitted, provided the original author(s) and the copyright owner(s) are credited and that the original publication in this journal is cited, in accordance with accepted academic practice. No use, distribution or reproduction is permitted which does not comply with these terms.
*Correspondence: Qiang Liu, cWlhbmcubGl1QGJudS5lZHUuY24=