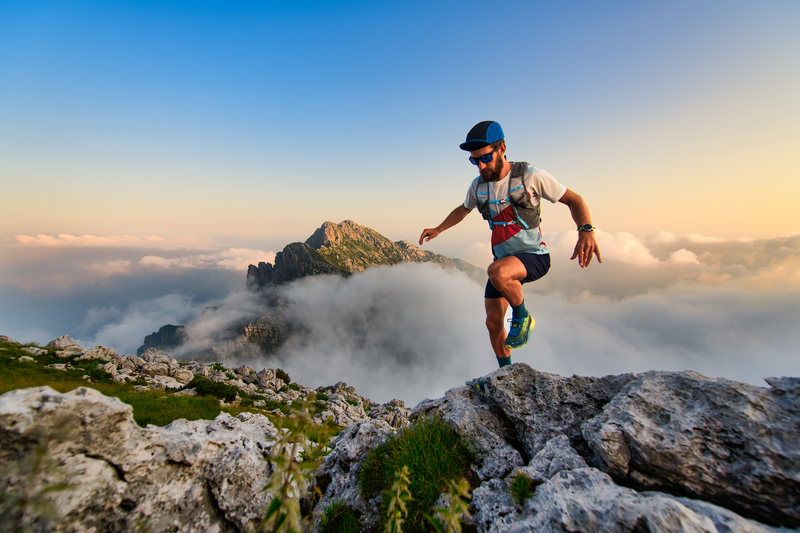
94% of researchers rate our articles as excellent or good
Learn more about the work of our research integrity team to safeguard the quality of each article we publish.
Find out more
ORIGINAL RESEARCH article
Front. Earth Sci. , 24 January 2022
Sec. Hydrosphere
Volume 9 - 2021 | https://doi.org/10.3389/feart.2021.813287
This article is part of the Research Topic Wetlands and Water Resources: Ecological Solutions for the Environment View all 13 articles
Compared to the eutrophication of lakes and reservoirs, the mechanism of river algal blooms in a flowing water body are more complicated, and often lead to serious consequence in catchment scale. Due to the simultaneous impact of a variety of environmental pressures, the water ecosystem integrity state often shows a response characteristic of accumulation, complexity and time lag, therefore it is difficult to use conventional hydrodynamic and water quality models to scientifically characterize and analyze. The lower Hanjiang River (HR) is an important influence area of the middle route of the South-to-North Water Diversion Project (SNWDP) in China, continuous river blooms issue has become a major national concern. In this study, a time-lag analysis approach was developed to identify the causes of algal blooms formation and the time-lag response law in the lower HR, including principal component analysis (PCA), grey relation analysis (GRA), and Almon Distributed Lag Model. Results found that, the hydrological regime (ΔH) contributes the most to the river bloom, especially due to the flow hindrance of the Yangtze River (YR) and the water project upstream. It is also found that the algal bloom outbreak in the lower HR is not an immediate response to the driving factors. It has a time lag of about 1 period (10 days) in the response with antecedent driving factors. Finally, we discussed the influence and its responding mechanism of ΔH on the growth of phytoplankton. The research can provide early warning for the prevention and control of algal blooms in the large river system.
Algal blooms as an extreme form of water eutrophication (Whitehead et al., 2015), usually occur in relatively static water bodies such as lakes and reservoirs (Cheng et al., 2019). However, the anthropogenic regulation of rivers (i.e., dam construction, flood protection, and water extraction) can greatly change the hydrological conditions in the rivers, causing severe environmental loss of river connectivity (Zhou et al., 2013; Maavara et al., 2015). Therefore, algal blooms have become one of the serious environmental issue at catchment scale that are greatly disturbed by human activities (Jeong et al., 2007; Mitrovic et al., 2007). Algal blooms prevent sunlight from penetrating the water surface, causing the death of aquatic life (Bhat et al., 2006). On the other hand, many phytoplankton species can produce potent toxins that can cause direct or indirect harm to various lives through the food chain and food web (Hallegraef, 1993). The causal analysis and driving mechanism of algal blooms in large hydrological-regulated rivers have become a major concern, as the algal blooms in these rivers not only lead to deteriorating water quality and aquatic ecosystems but also restrict socioeconomic developments and threaten public health with a greater sphere of influence.
Because of the nonlinearity and time-lag of aquatic ecological process, river blooms are not only caused by a single driving factor but also resulting of a combination of multiple driving factors under antecedent environmental conditions (Xia et al., 2020). There is a general perspective that river blooms are mainly caused by sufficient nutrients, suitable climatic conditions, and slow hydrological conditions (Mitrovic et al., 2007; Jung et al., 2009; Jung et al., 2011; Yang et al., 2011; Liu et al., 2016; Ji et al., 2017). In the most algal bloom rivers, nutrients often meet the basic conditions for algae growth, so they are not the main limiting factor for algal blooms (Mischke et al., 2011; Yang et al., 2012; Oliver et al., 2014). However, increased nutrient loads could lead to harmful algal blooms increase (Zhou et al., 2001; Wang, 2006; Li et al., 2014). With higher water temperature and longer water retention time at low flow rates, which can promote the growth of algae in the Hunter River, Australia (Mitrovic et al., 2007). Even though physical and chemical conditions are conducive to the growth of algae, the hydrodynamic conditions also determine the occurrence of algal blooms in the tributaries of the Three Gorges Reservoir, China (Ji et al., 2017; Chuo et al., 2019). Experimental studies have shown that hydrodynamic conditions (i.e., fluctuations and agitation of water flow) usually have a significant impact on the migration, diffusion, growth, and accumulation of algae (Chung et al., 2008; Lucas et al., 2009; Whitehead et al., 2015). Fluctuations in hydrological conditions can change the migration and transformation of nutrients, the transmission of solar radiation, and the migration of algae (McKiver and Neufeld, 2009; Istvánovics and Honti, 2012). In addition, the gentle hydrological situation will reduce the turbidity of the water body, which help the growth and reproduction of algae (Wang, 1974). All of the above studies show that the hydrologic regime is an important driving factor for river-type algal blooms (Mitrovic et al., 2007; Cheng et al., 2019; Xia et al., 2020). However, few studies have explored the time-lag response of algal blooms to driving factors.
Time-lag response refers to a response relationship with a certain time lag between the dependent variable and the independent variable. The majority of research on river algal blooms focuses on phytoplankton and driving the short-term “snapshot effect” between factors (Jeong et al., 2007). However, the time-lag effect not only has an important explanatory effect on the field observation results of microalgae communities under natural conditions but also has an important effect on the simulation, prediction, and early warning of water bloom outbreaks (Harris, 1983). Studies have shown that there is a lag of at least 2 years between the population dynamics of Microcystis aeruginosa and Stephanodiscus hantzschii and hydrological environment (i.e., the quantity of dam storage and discharge) in the lower Nakdong River, South Korea (Jeong et al., 2007). Other studies on the Nakdong River have also proved this conclusion. In a normal year, the response period of the Chla concentration in the Nam River dam to rainfall is 1 month later than that of other dams, while in a wet year, the lag response period is 2 months (Kim et al., 2009). In fact, in previous studies of algal blooms in the HR, scholars proposed the importance of the time-lag effect (Yang et al., 2012; Xia et al., 2020). According to the study of algal blooms in HR, some scholars have pointed out the importance of the time-lag effect. Yang et al. (2011) proposed that the time lag effect is a frontier issue in the study of river-type algal blooms. Furthermore, through the gradient boosting machine (GBM), it is proved that the use of the environmental factors (especially the hydrological situation) in the first 10 days can better predict the algal blooms of the HR, China (Xia et al., 2020). The above research shows that different rivers and water bodies have different time lags. However, few studies have quantitatively revealed the length of the lag response time of algal blooms to various driving factors. It is necessary to carry out a quantitative analysis of the time-lag effect between river algal blooms and its multiple driving factors, in order to reveal the mechanism of river water ecological degradation.
Plenty of algal blooms occurred in the lower HR, and it is essential to analyze them from multiple angles. Therefore, this study aims to reveal the response mechanism of river algal blooms based on the analysis of interactions of those influencing factors, by quantitatively determining the time-lag response of algal bloom to multiple driving factors. We conduct following studies including 1) determining the key driving factors regulating the algal blooms in the lower HR. 2) evaluating the time-lag effect of influencing factors by analyzing data collected from field surveys with principal component analysis (PCA), Almon Distributed Lag Model and curve fitting. PCA was carried out to classify the environmental factors. Almon Distributed Lag Model was applied to establish the optimal lag time of every variable. Curve fitting was applied to quantitatively describe the impact of hydrological regimes on algal blooms. Finally, we expect to propose a method for predicting river water ecological degradation in advance.
The HR is the largest tributary of the YR of China (Figure 1). It flows from the northwest to the southeast and joins the YR in Wuhan City (Li et al., 2009). The annual discharge from the HR to the YR is 33.2 billion m3 (Xin et al., 2020). The average annual temperature is 16°C. The average annual precipitation amounts to 700–1,000 mm, with >80% occurring between May to September (Chen et al., 2007). Danjiangkou Reservoir, as the water source for the middle route of the South-to-North Water Diversion Project, is located in the middle reaches of the HR (Xia et al., 2016). Flowing through the most economically-developed region in Hubei Province, the middle and lower reaches of the HR are the most important source of drinking water for coastal cities (Xia et al., 2012; Cheng et al., 2019). However, the wastewater influent to the HR with nutrients and organic matters has increased in the past 30–40 years as a consequence of rapid urbanization, economic growth, and intensification of agricultural productivity (Xie et al., 2004). In addition, the opening of the middle route of the SNWDP has also changed the hydrologic situation of the lower reaches of the HR (Cao et al., 2020). The middle and lower reaches of the HR have been plagued by algal blooms. There have been lots of algal blooms in this area since 1992 (Li et al., 2020). The driving factors for the algal blooms in the lower reaches of the HR are complex. It is not only related to the water environmental factors of the HR but also to the hydrological situation in the estuary confluence area (backwater area) (Xia et al., 2020) (Figure 1). As the impact of algal blooms on the lower reaches of the HR becomes more and more serious, it has become one of the urgent problems to be solved (Xie et al., 2006; Li, 2007).
FIGURE 1. Overview of the study area and sample sites. Water levels were measured at the Xiantao Station for the HR and at the Hankou Station for the YR. Water quality and Chla were measured at three sample sites: Baihezui (BHZ), Qinduandou (QDK), and Zongguan (ZG).
Unlike lakes or other rivers, the causes of algal blooms in the lower HR are extremely complex. Because the lower HR is located in the area affected by the middle route of the SNWDP and also in the hydrological change area where the HR merges into the YR. In this study, we used the algal blooms data (Chlorophyll-a concentration, Chla) at Baihezui (BHZ), Qinduankou (QDK), and Zongguan (ZG) sample sites located in the lower HR every 10 days from February to April in 2004–2014. We collected the hydrological data of the Xiantao (XT) and Hankou (HK) hydrologic stations during the same period from the YR Water Conservancy Commission Hydrological Bureau. The hydrological data include daily flow (QH), flow velocities (vH), and water level (WLH) in the Xiantao Station; daily flow (QY), flow velocities (vY), and water level (WLY) in the Hankou Station. Furthermore, we calculated the water level difference (ΔH) between the two hydrological stations. In addition, 10 days water quality data (i.e., water temperature (WT), total nitrogen concentration (TN), and total phosphorus concentration (TP)) at the BHZ, QDK, and ZG stations were provided by the YR Basin Ecological and Environmental Supervision Authority. 1 L of water sample was filtered in situ and taken back to the laboratory for the analysis of nutrients, including TP and TN, using ammonium molybdate spectrophotometric method and alkaline potassium digestion UV spectrophotometric method. WT was determined using a HACH Hydrolab MI-parameter Meter. The above datasets were used for analysis.
PCA (Zhou et al., 2017) is often used to classify the dominant environmental factors and define the effects of the hidden variables (major components) on the biomass of phytoplankton in the study area. The standardized environmental factor data, including hydrological data and water quality data, were used for PCA.
where
Almon Distributed Lag Model (Almon, 1965) was used to analyze the time-lag effect of algal blooms on each driving factor quantitatively.
The Almon Lag Model as
where
To eliminate the multicollinearity between variables in different lag periods, the polynomial distributed lag method is used:
where, m is the order number of a polynomial, and generally, m < k.
By substituting Eq. 3 into Eq. 2, we get:
It is transformed into the regression of Y on a reconstructed variable Z.
By using Eq. 4, estimated values
The correlation coefficient between the estimated Yt and the measured Yt was used to determine an optimal lag time. If the correlation coefficient no longer increases with the increase of k, a value of k at this time is considered to be the optimal lag time (Liu, 2019; Özbay and Toker, 2021).
A variety of driving factors were used to determine the driving effect on algal blooms. According to their availability, we divide them into algal bloom characterization data, water quality data, and hydrological data. The statistics of the different driving factors, including the mean, minimum (min), maximum (max), standard deviation (SD), and coefficient of variation (CV), are listed in Table 1. The maximum of Chla during the three sites is 67.8 μg/L (ZG). The variability of Chla at ZG is also the largest (0.20–67.8 μg/L). Statistics of TP are similar at three sites including BHZ (mean 0.12 mg/L; min 0.05 mg/L; max 0.35 mg/L; SD 0.04; CV 0.35), QDK (mean 0.12 mg/L; min 0.05 mg/L; max 0.35 mg/L; SD 0.04; CV 0.34), and ZG (mean 0.12 mg/L; min 0.04 mg/L; max 0.30 mg/L; SD 0.04; CV 0.36). The situations of TN and WT are similar to that of TP. The differences among the three sites are not significant. Regarding hydrological data, the flow and velocity of the HR are less than those of the YR. Moreover, the ΔH is in a state of volatility.
TABLE 1. Mean, range (min, max), standard deviation (SD), and coefficient of variation (CV) of chlorophyll-a and the associated environmental factors during 2004–2014.
According to the level of Chla, the biomass in the three sections were in a state of flux. The average value of Chla was high in other years except for the years 2005 and 2007 (Figures 2A–C). For TP and TN, a similar trend was exhibited. TN was in a state of rising volatility from 2004 to 2014, but TP had relatively large fluctuations during the year, relative to TN (Figures 2D–I). In addition, the WT showed a unique trend, and the change had a certain periodicity, about 6–7 years (Figures 2J–L).
We used the hydrological data of hydrological situation in Xiantao (located in HR) and Hankou (located in YR) to represent the hydrological conditions of the HR and the YR, respectively. The results are shown in Figure 3. We can see that the hydrological situation of the YR had changed significantly, compared to that of the HR. The two low values of QY appeared in 2006 and 2011 and the WLY was also at a low peak. As shown in Figure 3B, ΔH had been showing a downward trend since 2011 and the direct causes are the increase of WLY and the decrease of WLH Figure 4.
The normalized data met the analysis requirements of PCA. The results of PCA at three sites (BHZ, QDK, and ZG) are as follows (Figure 4). At the BHZ sample site, three principal components have been selected and their total contribution rate reached 81.1%. The first two components together represented 70.5% of the total variation. The first principal component explained 47.4% of the total variation and the second principal component explained 23.1% of the total variation. Moreover, the third principal component explained 10.6% of the total variation. The Radar chart of the score for each variable at the BHZ site indicated that WT, QH, vH, QY, vY, WLH, and WLY had high capacities in the first principal component, while ΔH had high capacity in the second principal component. In addition, TP and TN were dominant in the third principal component. More specific PCA results are shown in Supplementary Appendix A.
The results of PCA at the QDK and ZG sites are similar to that at the BHZ site. It is worth noting that the second principal component only contains the variable ΔH, which contributes 23.1, 23.3, and 23.3% of interpretation at the BHZ, QDK, and ZG sites respectively. Therefore, the ΔH is considered as an important factor influencing the algal blooming in the lower HR.
The above analysis shows that there is not much difference between each variable among the three sites, so we will average the variable values of the three sites. GRA was used to determine the correlation between each environmental driving factor and Chla during 2004–2014. ΔH achieves the highest degree of relevance, which is 0.8960. WT, TN, TP are then in turn. The results are shown in Supplementary Appendix B.
Furthermore, we analyzed the annual change trends of Chla and ΔH during the four consecutive years of algal blooms from 2008 to 2011. The results are shown in Supplementary Appendix C. During the algal blooming period, the ΔH between Xiantao and Hankou was always at a low level. The relatively gentle hydrological regime was suitable for the growth of phytoplankton. We also found that the ΔH was already at a low level during the pre-order time when the Chla appeared high. Therefore, the growth of phytoplankton was not an “instantaneous” response to hydrological driving factors but a response lagged a certain amount of time. The response time of the algal blooms in 2008–2011 has a relatively significant lag effect, which means that the change of the water level usually occurs before the algal bloom occurs.
To analyze the time-lag response of algal blooms to various driving factors, we identified four main variables based on the previous analysis, including ΔH, WT, TP, and TN. Furthermore, the Almon Distributed Lag Model was used to determine the effect of algal bloom on various driving factors. The length of the lag time of the driving factors and the average value of the variable are continued to be used. The analysis results are as follows (Figures 5, 6). For the driving factor ΔH, when we used the data from the same period as Chla, the correlation coefficient (r) of the measured and simulated values of Chla was 0.240 (p > 0.05). When we used the ΔH data of the current period and the 1 period ahead (10 days) at the same time, it was 0.297 (p < 0.05). Furthermore, when we used the ΔH data of the current period, 1 period ahead (10 days), and two periods ahead (20 days), the result became 0.298 (p < 0.05). In addition, the results for the driving factor WT are 0.040 (p > 0.05), 0.285 (p < 0.05), and 0.288 (p < 0.05). For the driving factor TN, the results are 0.071 (p > 0.05), 0.209 (p > 0.05), and 0.210 (p > 0.05). Regarding the driving factor TP, the results are 0.475 (p < 0.05), 0.493 (p < 0.01), and 0.492 (p < 0.01).
Furthermore, from Figure 6 we can infer that when the lag time was set to two periods ahead (20 days), the r did not continue to increase or the increase was very small. For the increased range in r, WT achieved the largest value of 0.248 and TP had the smallest value of 0.017. Therefore, we set 1 period ahead (10 days) as the optimal time-lag length, which is the most significant impact on the algal blooms in the lower HR are the driving factors in the current period and the 1 period ahead (10 days).
Based on the results of PCA and GRA, the roles of the different environmental factors were evaluated during the river-type algal blooms. TP and TN exhibited no significant effects on Chla during the diatom algal blooms. Although they are critical for the growth of diatoms, their concentrations were high enough to support the diatom bloom (Zhang et al., 2008). Previous studies have confirmed that nutrients are not a necessary limiting factor for most river algal bloom events, but nutrients are a prerequisite for the occurrence of algal blooms (Mitrovic et al., 2007; Yang et al., 2017). Our results show that the GRA scores of the concentration of Chla and the concentrations of TP and TN are ranked behind those of the ΔH and WT. Similar to our research, Zeng et al. (2006) believed that there is no significant positive correlation between the concentration of phytoplankton and Chla and the content of nutrients in the Three Gorges reservoir area. Besides, during the outbreak of algal blooms in the lower HR, the concentrations of TN and TP have far met the needs of phytoplankton growth, and there is a continuous supply without restriction. Moreover, water temperature is essential for the growth of phytoplankton (Carey et al., 2012). Does low temperature constrain the growth rates of heterotrophic protists become a question, and Rose and Caron (2007) gave a negative answer. Any aquatic organism has its optimal growth temperature range and the suitable water temperatures for the growths of blue algae, green algae, and blue-green algae are approximately 30–35°C, 20–25°C, and 15–20°C, respectively (Cheng et al., 2019). However, diatom algae as the dominant species in the spring algal blooms of HR, have an optimal growth temperature range of 5–15°C (Ha et al., 2002; Kim et al., 2007). Some experimental results in the laboratory showed that the growth rate of S.hantzschii decreased when the temperature exceeded 20°C (Jung et al., 2011). Therefore, the temperature recovery period in late winter and early spring is a window period for the growth of diatoms and also a period when water blooms are prone to outbreaks in the lower reaches of the HR.
Our analysis also show that, ΔH is closely related to the algal blooms in the lower HR as a variable that comprehensively expresses the hydrological situation of the HR and the YR. Many researchers have also proposed that the hydrological regime is the most important driving factor for the outbreak of river algal blooms (Yang et al., 2011; Kim et al., 2019; Xia et al., 2019; Xia et al., 2020). A lower water level difference indicates a smaller flow rate. It decreases the loss of phytoplankton caused by flow flushing and provides phytoplankton more retention time to enlarge their biovolumes (Fisher, 1996). In addition, sometimes gentle hydrological conditions can lead to thermal stratification in some regulated rivers, which promotes the growth of diatoms (Bormans and Webster, 1998). The lack of underwater sunlight will reduce the biomass of underwater phytoplankton in turbulent rivers (Reynolds and Descy, 1996). The lower flow rate will reduce the disturbance of the water body, which reduces the turbidity and increases the transparency. Therefore, ΔH was found to provide new insights for the prevention and control of river algal blooms.
Time-lag response widely exists in the biological world due to the complexity of aquatic ecological processes. Dependent on the algal species and the magnitude of the nutrient pulse, the cell division has different states (Collos, 1986). For different phytoplanktons, their growth can be divided into two different strategies. One is that the body of certain phytoplanktons does not accumulate nutrients. The growth of these phytoplanktons and the absorption of nutrients are closely integrated. Therefore, these phytoplanktons have a rapid stress growth response to the stimulation of nutrients. In contrast, other kinds of phytoplanktons have the ability to accumulate a large amount of nutrients in the body and carry out complex transformation and absorption processes. The time lags in cell division are usually more than 24 h (Collos, 1986). Our studies show that the length of the time lag in response of the algal blooms to the driving factors is 1 period (10 days) in HR. It is consistent with previous studies. For example, Xia et al. (2020) have proposed river algal blooms are well predicted by antecedent environmental conditions, especially hydrological factors.
However, it is hard to quantify the time-lag effect of algal bloom outbreaks to various driving factors. Although we were not able to conduct a more detailed analysis since the time interval of our data is 10 days, results are similar to the conclusions of many other studies. Based on the artificial neural network model, it was indicate that the time lag in response to environmental variables is often between 7 and 14 days (Lee et al., 2003). Moreover, some studies show that the length of time lag between the algal bloom outbreak and the driving factors is 2–4 days in lakes (Recknagel et al., 2013). In addition, the length of time lag is related to the distance between the driving element monitoring point and the algal bloom monitoring point, which includes the time involved in environmental variable migration and exercise (Jeong et al., 2007). Our research area is located at the confluence of estuaries where the HR merges into the YR, it is also the sensitive impact area of mega water project. Therefore, the intrusion of the YR water also affects the driving factors in the study area, and it makes the causes of blooms are more complex. By utilizing the time-lag response of algal bloom outbreaks to the driving factors, hydrological regulations that are feasible and efficient to implement can be developed to reduce the potential risk of river algal blooms (Mitrovic et al., 2007; Xin et al., 2020). As demonstrated above, hydrological data are easy to observe and effective for early warning of algal bloom outbreaks. Based on the hydrological sensitivity of algal blooms, we can adjust the interactive hydrological situation of the YR and HR to alleviate algal blooms.
We have also found that the ΔH affects the ecological processes and flow patterns of the river channel in several ways. When ΔH becomes smaller, the YR’s supporting effect on the HR is obvious and its inflow become hindered. This promotes the formation of a stable hydrological environment similar to a lake reservoir in the estuary confluence area. It provides a site and environmental stimulation for the growth and reproduction of phytoplankton. From Figure 3, we can see that the water level fluctuation of the YR is more intense than that of the HR. The change of the water level of the YR contributes the most to the change of ΔH. We infer that the water level change was highly affected by the impoundment of the Danjiangkou Reservoir upstream, which also decreases the ΔH. The drop of ΔH forms a backwater area in the estuary area (Qu et al., 2014), which lengthens the residence time of the water body and slows down the changes in hydrological situation (Yin et al., 2012). The nutrients, such as nitrogen and phosphorus, in the YR are significantly overloaded (Li et al., 2007; Liu et al., 2018). The backflow of the YR causes nutrients to enter the Han River, which promotes the growth of phytoplankton and increases the probability of algal blooms.
This study shows that there is a significant correlation between Chla and ΔH, and the ΔH contributed most to the algal blooms (Appendix B). Therefore, it can be concluded that the hydrological condition of the YR has greatly contributed to the occurrence of algal blooms in the lower HR. To further analyze the relationship between phytoplankton growth (G(h)) and ΔH, we assume a specific environment. At this time, the water quality and climate conditions are kept in a relatively stable state. Furthermore, we performed a curve fitting analysis on the phytoplankton change rate and the two-periods ΔH data. The results (Figure 7) show that a cubic equation can fit the correlation best, compared to a quadratic equation, logarithmic equation, and linear equation. The fitting equation is as follows.
Some experiments have been conducted to analyze the mechanism underlying the correlation between hydrological regimes and algal blooms by estimating the suitable hydrological conditions (Zheng et al., 2009; Yin et al., 2012). Our results imply that the growth rate of Chla first decreases with the increase of water level then increases. After that, it keeps decreasing. This fluctuation is related to the growth habit of diatoms. As the ΔH increases, phytoplankton is washed away to reduce (Yang et al., 2012). Diatoms are suitable for growth in water bodies with a certain flow rate (Zheng et al., 2009). At the same time, the proper flow rate also reduces the settlement of phytoplankton (Xia et al., 2019). During the treatment of algae blooms in the lower reaches of the HR in 2018, the discharge flow rate of Danjiangkou Reservoir was increased to 800 m3/s but the elimination of algal blooms did not achieve the expected effect. When the discharge flow increased to 1,300 m3/s, the algal bloom outbreak was further curbed (Li et al., 2020). Therefore, we can make early decisions and warnings for the prevention and control of algal blooms in the lower HR by discovering the key driving role of ΔH based on the time-lag effect of the algal bloom outbreak.
In this study, we used a variety of statistical analysis methods and the Almon Time Lag Model to investigate the algal blooms during a decade (2003–2014) in the lower HR, which is the largest tributary of the YR of China. It is found that all hydrological conditions, nutrients, and water temperature contributed to the outbreak of river algal blooms. In particular, hydrological conditions contributed the most to the outbreak of algal blooms in the rivers. Hydrological conditions contained only the principal component of a variable ΔH, contributing more than 23% of the explanatory power. Moreover, the gray correlation between Chla and ΔH was the highest, which was 0.896. In addition, there was a time lag of about 1 period (10 days) in response to the main driving factors of algal bloom outbreaks. These findings provide new discovery for the simulation and early warning of algal blooms, as well as provide time to take emergency measures for the upcoming algal bloom in advance. Therefore, the water level relationship between the YR and the HR should be harmonized to destroy the hydrological regime that is conducive to the growth of phytoplankton. Traditionally, the elimination of algal blooms in the lower HR mainly relied on the flushing of upstream water, which sometimes resulted in the waste of many high-quality freshwater resources. This study is limited by the scale of the data interval and the analysis of the length of the time lag can be further improved. With the rapid expansion of human activities and the intensification of climate change, many rivers will suffer from algae blooms in the future. Therefore, our research provides a baseline for the prevention and control of algae blooms in other rivers.
The original contributions presented in the study are included in the article/Supplementary Material, further inquiries can be directed to the corresponding author.
CL and YC contributed to the conceptualization, ideas, methodology, original paper draft and visualization. LZ, BC and TH contributed to study area definition, methods selection and results discussion. All authors contributed to article revision, read, and approved the submitted version.
This work was supported by National Key R&D Program of China (grant No. 2021YFC3201003), The National Natural Science Foundation of China (grant No. 51879252), The Fundamental Research Funds for the Central Public-interest Scientific Institution (grant No. 2021KSKY-04), The Special Fund for Basic Scientific Research of Central Public Research Institutes (2020YSKY-003).This work is supported and funded by the Chinese Research Academy of Environmental Sciences.
The authors declare that the research was conducted in the absence of any commercial or financial relationships that could be construed as a potential conflict of interest.
All claims expressed in this article are solely those of the authors and do not necessarily represent those of their affiliated organizations, or those of the publisher, the editors, and the reviewers. Any product that may be evaluated in this article, or claim that may be made by its manufacturer, is not guaranteed or endorsed by the publisher.
The Supplementary Material for this article can be found online at: https://www.frontiersin.org/articles/10.3389/feart.2021.813287/full#supplementary-material
Almon, S. (1965). The Distributed Lag between Capital Appropriations and Expenditures. Econometrica 33, 178–196. doi:10.2307/1911894
Bhat, S. R., Devi, P., D'Souza, L., Verlecar, X. N., and Naik, C. G. (2006). Harmful Algal Blooms. India: TERI Press.
Bormans, M., and Webster, I. T. (1998). Dynamics of Temperature Stratification in lowland Rivers. J. Hydraulic Eng. 124, 1059–1063. doi:10.1061/(asce)0733-9429(1998)124:10(1059)
Cao, S., Xia, R., Zhang, Y., Li, Z., Ren, Y., and Ta, L. (2020). Characteristics and Response of Ecological Environment in Downstream of Hanjiang River before and after Running of Middle Route of South-To-North Water Diversion Project. Res. Environ. Sci. 33, 1431–1439. doi:10.13198/j.issn.1001-6929.2019.07.30
Carey, C. C., Ibelings, B. W., Hoffmann, E. P., Hamilton, D. P., and Brookes, J. D. (2012). Eco-physiological Adaptations that Favour Freshwater Cyanobacteria in a Changing Climate. Water Res. 46, 1394–1407. doi:10.1016/j.watres.2011.12.016
Chen, H., Guo, S., Xu, C.-y., and Singh, V. P. (2007). Historical Temporal Trends of Hydro-Climatic Variables and Runoff Response to Climate Variability and Their Relevance in Water Resource Management in the Hanjiang basin. J. Hydrol. 344, 171–184. doi:10.1016/j.jhydrol.2007.06.034
Cheng, B., Xia, R., Zhang, Y., Yang, Z., Hu, S., Guo, F., et al. (2019). Characterization and Causes Analysis for Algae Blooms in Large River System. Sustain. Cities Soc. 51, 101707. doi:10.1016/j.scs.2019.101707
Chung, S.-W., Lee, H., and Jung, Y. (2008). The Effect of Hydrodynamic Flow Regimes on the Algal Bloom in a Monomictic Reservoir. Water Sci. Technol. 58, 1291–1298. doi:10.2166/wst.2008.482
Chuo, M., Ma, J., Liu, D., and Yang, Z. (2019). Effects of the Impounding Process during the Flood Season on Algal Blooms in Xiangxi Bay in the Three Gorges Reservoir, China. Ecol. Model. 392, 236–249. doi:10.1016/j.ecolmodel.2018.11.017
Collos, Y. (1986). Time-lag Algal Growth Dynamics: Biological Constraints on Primary Production in Aquatic Environments. Mar. Ecol. Prog. Ser. 33, 193–206. doi:10.3354/meps033193
Fisher, W. (1996). Stream Ecology: Structure and Function of Running Waters. Utaf 125, 154–158. doi:10.1577/1548-8659-125.1.154
Ha, K., Jang, M.-H., and Joo, G.-J. (2002). Spatial and Temporal Dynamics of Phytoplankton Communities along a Regulated River System, the Nakdong River, Korea. Hydrobiologia 470, 235–245. doi:10.1023/a:1015610900467
Hallegraeff, G. M. (1993). A Review of Harmful Algal Blooms and Their Apparent Global Increase. Phycologia 32, 79–99. doi:10.2216/i0031-8884-32-2-79.1
Harris, G. P. (1983). Mixed Layer Physics and Phytoplankton Populations: Studies in Equilibrium and Non-equilibrium Ecology. Prog. Phycological Res. 2, 1–15.
Istvánovics, V., and Honti, M. (2012). Efficiency of Nutrient Management in Controlling Eutrophication of Running Waters in the Middle Danube Basin. Hydrobiologia 686, 55–71. doi:10.1007/s10750-012-0999-y
Jeong, K.-S., Kim, D.-K., and Joo, G.-J. (2007). Delayed Influence of Dam Storage and Discharge on the Determination of Seasonal Proliferations of Microcystis Aeruginosa and Stephanodiscus Hantzschii in a Regulated River System of the Lower Nakdong River (South Korea). Water Res. 41, 1269–1279. doi:10.1016/j.watres.2006.11.054
Ji, D., Wells, S. A., Yang, Z., Liu, D., Huang, Y., Ma, J., et al. (2017). Impacts of Water Level Rise on Algal Bloom Prevention in the Tributary of Three Gorges Reservoir, China. Ecol. Eng. 98, 70–81. doi:10.1016/j.ecoleng.2016.10.019
Jung, S. W., Kwon, O. Y., Lee, J. H., and Han, M.-S. (2009). Effects of Water Temperature and Silicate on the Winter Blooming DiatomStephanodiscus hantzschii(Bacillariophyceae) Growing in Eutrophic Conditions in the Lower Han River, South Korea. J. Freshw. Ecol. 24, 219–226. doi:10.1080/02705060.2009.9664286
Jung, S. W., Min Joo, H., Kim, Y.-O., Hwan Lee, J., and Han, M.-S. (2011). Effects of Temperature and Nutrient Depletion and Reintroduction on Growth ofStephanodiscus hantzschii(Bacillariophyceae): Implications for the Blooming Mechanism. J. Freshw. Ecol. 26, 115–121. doi:10.1080/02705060.2011.553927
Kim, D.-K., Jeong, K.-S., Whigham, P. A., and Joo, G.-J. (2007). Winter Diatom Blooms in a Regulated River in South Korea: Explanations Based on Evolutionary Computation. Freshw. Biol 52, 2021–2041. doi:10.1111/j.1365-2427.2007.01804.x
Kim, H. G., Hong, S., Jeong, K.-S., Kim, D.-K., and Joo, G.-J. (2019). Determination of Sensitive Variables Regardless of Hydrological Alteration in Artificial Neural Network Model of Chlorophyll a: Case Study of Nakdong River. Ecol. Model. 398, 67–76. doi:10.1016/j.ecolmodel.2019.02.003
Kim, M.-C., Jeong, K.-S., Kang, D.-K., Kim, D.-K., Shin, H.-S., and Joo, G.-J. (2009). Time Lags between Hydrological Variables and Phytoplankton Biomass Responses in a Regulated River (The Nakdong River). J. Ecol. Environ. 32, 221–227. doi:10.5141/jefb.2009.32.4.221
Lee, J. H. W., Huang, Y., Dickman, M., and Jayawardena, A. W. (2003). Neural Network Modelling of Coastal Algal Blooms. Ecol. Model. 159, 179–201. doi:10.1016/s0304-3800(02)00281-8
Lee, M., Kim, B., Kwon, Y., and Kim, J. (2009). Characteristics of the marine Environment and Algal Blooms in Gamak Bay. Fish. Sci. 75, 401–411. doi:10.1007/s12562-009-0056-6
Li, C. (2007). Analysis and Research on Influencing Factors of Algae Bloom in Hanjiang River. Kunming: Kunming University of Science and Technology.
Li, H.-M., Tang, H.-J., Shi, X.-Y., Zhang, C.-S., and Wang, X.-L. (2014). Increased Nutrient Loads from the Changjiang (Yangtze) River Have Led to Increased Harmful Algal Blooms. Harmful Algae 39, 92–101. doi:10.1016/j.hal.2014.07.002
Li, J., Yin, W., Jia, H., and Xin, X. (2020). Reserch Progress on Diatom Blooms in the Middle and Lower Hanjiang River: Review and Advances. J. Hydroecology 41, 136–144. doi:10.15928/j.1674-3075.2020.05.016
Li, M., Xu, K., Watanabe, M., and Chen, Z. (2007). Long-term Variations in Dissolved Silicate, Nitrogen, and Phosphorus Flux from the Yangtze River into the East China Sea and Impacts on Estuarine Ecosystem. Estuarine, Coastal Shelf Sci. 71, 3–12. doi:10.1016/j.ecss.2006.08.013
Li, S., Cheng, X., Xu, Z., Han, H., and Zhang, Q. (2009). Spatial and Temporal Patterns of the Water Quality in the Danjiangkou Reservoir, China. Hydrological Sci. J. 54, 124–134. doi:10.1623/hysj.54.1.124
Liu, D., Yang, Z., Ji, D., Ma, J., Cui, Y., and Song, L. (2016). A Review on the Mechanism and its Controlling Methods of the Algal Blooms in the Tributaries of Three Gorges Reservoir. J. Hydraulic Eng. 47, 443–454. doi:10.13243/j.cnki.slxb.20151304
Liu, X., Beusen, A. H. W., Van Beek, L. P. H., Mogollón, J. M., Ran, X., and Bouwman, A. F. (2018). Exploring Spatiotemporal Changes of the Yangtze River (Changjiang) Nitrogen and Phosphorus Sources, Retention and export to the East China Sea and Yellow Sea. Water Res. 142, 246–255. doi:10.1016/j.watres.2018.06.006
Liu, X. (2019). Study on Time Lag Effect and Spatial-Temporal Distribution Model of Agricultural Drought — A Case Study of the Plain Area of South Hebei ProvinceSchool of Water Conservancy and Hydroelectric Power. Handan City, China: Hebei University of Engineering.
Lucas, L. V., Thompson, J. K., and Brown, L. R. (2009). Why Are Diverse Relationships Observed between Phytoplankton Biomass and Transport Time? Limnol. Oceanogr. 54, 381–390. doi:10.4319/lo.2009.54.1.0381
Maavara, T., Parsons, C. T., Ridenour, C., Stojanovic, S., Dürr, H. H., Powley, H. R., et al. (2015). Global Phosphorus Retention by River Damming. Proc. Natl. Acad. Sci. USA 112, 15603–15608. doi:10.1073/pnas.1511797112
McKiver, W. J., and Neufeld, Z. (2009). Influence of Turbulent Advection on a Phytoplankton Ecosystem with Nonuniform Carrying Capacity. Phys. Rev. E 79, 061902. doi:10.1103/PhysRevE.79.061902
Mischke, U., Venohr, M., and Behrendt, H. (2011). Using Phytoplankton to Assess the Trophic Status of German Rivers. Int. Rev. Hydrobiology 96, 578–598. doi:10.1002/iroh.201111304
Mitrovic, S. M., Chessman, B. C., Davie, A., Avery, E. L., and Ryan, N. (2007). Development of Blooms of Cyclotella Meneghiniana and Nitzschia Spp. (Bacillariophyceae) in a Shallow River and Estimation of Effective Suppression Flows. Hydrobiologia 596, 173–185. doi:10.1007/s10750-007-9094-1
Oliver, A. A., Dahlgren, R. A., and Deas, M. L. (2014). The Upside-Down River: Reservoirs, Algal Blooms, and Tributaries Affect Temporal and Spatial Patterns in Nitrogen and Phosphorus in the Klamath River, USA. J. Hydrol. 519, 164–176. doi:10.1016/j.jhydrol.2014.06.025
Özbay, N., and Toker, S. (2021). Prediction Framework in a Distributed Lag Model with a Target Function: an Application to Global Warming Data. Environ. Ecol. Stat. 28, 87–134. doi:10.1007/s10651-020-00477-x
Qu, Y., Huang, Y., He, Z., and Wu, G. (2014). The Influence of the Backwater Support of the Yangtze River on the Water-Sediment Process from the Xinglong of the Hanjiang River to the Hanchuan River. China Water Transport 11, 62–64. doi:10.13646/j.cnki.42-1395/u.2014.11.027
Recknagel, F., Ostrovsky, I., Cao, H., Zohary, T., and Zhang, X. (2013). Ecological Relationships, Thresholds and Time-Lags Determining Phytoplankton Community Dynamics of Lake Kinneret, Israel Elucidated by Evolutionary Computation and Wavelets. Ecol. Model. 255, 70–86. doi:10.1016/j.ecolmodel.2013.02.006
Reynolds, C. S., and Descy, J.-P. (1996). The Production, Biomass and Structure of Phytoplankton in Large Rivers. rs 10, 161–187. doi:10.1127/lr/10/1996/161
Rose, J. M., and Caron, D. A. (2007). Does Low Temperature Constrain the Growth Rates of Heterotrophic Protists? Evidence and Implications for Algal Blooms in Cold Waters. Limnol. Oceanogr. 52, 886–895. doi:10.4319/lo.2007.52.2.0886
Rui, X., Zhi, C., and Yun, Z. (2012). Impact Assessment of Climate Change on Algal Blooms by a Parametric Modeling Study in Han River. J. Resour. Ecol. 3, 209–219. doi:10.5814/j.issn.1674-764x.2012.03.003
Wang, B. (2006). Cultural Eutrophication in the Changjiang (Yangtze River) Plume: History and Perspective. Estuarine, Coastal Shelf Sci. 69, 471–477. doi:10.1016/j.ecss.2006.05.010
Whitehead, P. G., Bussi, G., Bowes, M. J., Read, D. S., Hutchins, M. G., Elliott, J. A., et al. (2015). Dynamic Modelling of Multiple Phytoplankton Groups in Rivers with an Application to the Thames River System in the UK. Environ. Model. Softw. 74, 75–91. doi:10.1016/j.envsoft.2015.09.010
Xia, R., Wang, G., Zhang, Y., Yang, P., Yang, Z., Ding, S., et al. (2020). River Algal Blooms Are Well Predicted by Antecedent Environmental Conditions. Water Res. 185, 116221. doi:10.1016/j.watres.2020.116221
Xia, R., Zhang, Y., Critto, A., Wu, J., Fan, J., Zheng, Z., et al. (2016). The Potential Impacts of Climate Change Factors on Freshwater Eutrophication: Implications for Research and Countermeasures of Water Management in China. Sustainability 8, 229. doi:10.3390/su8030229
Xia, R., Zhang, Y., Wang, G., Zhang, Y., Dou, M., Hou, X., et al. (2019). Multi-factor Identification and Modelling Analyses for Managing Large River Algal Blooms. Environ. Pollut. 254, 113056. doi:10.1016/j.envpol.2019.113056
Xie, M., Wang, X., Guan, G., and Hu, M. (2006). Analysis of Causes of "Water Bloom" in the Middle and Lower Reaches of Hanjiang River and its Countermeasures. Yangtze River 37 (8), 43–46. doi:10.16232/j.cnki.1001-4179.2006.08.017
Xie, P., Xia, J., Dou, M., and Zhang, W. (2004). Research into the Effects of the Middle Route of China's South-To-north Water Transfer Project on Water Bloom in the Middle-Down Stream of Hanjiang River and its Countermeasures Part Ⅰ- an Analysis of the Key Factors Generating Water Bloom in Hanjiang River. J. Nat. Resour. 19, 418–423.
Xin, X., Zhang, H., Lei, P., Tang, W., Yin, W., Li, J., et al. (2020). Algal Blooms in the Middle and Lower Han River: Characteristics, Early Warning and Prevention. Sci. Total Environ. 706, 135293. doi:10.1016/j.scitotenv.2019.135293
Yang, J. R., Lv, H., Isabwe, A., Liu, L., Yu, X., Chen, H., et al. (2017). Disturbance-induced Phytoplankton Regime Shifts and Recovery of Cyanobacteria Dominance in Two Subtropical Reservoirs. Water Res. 120, 52–63. doi:10.1016/j.watres.2017.04.062
Yang, Q., Xie, P., Shen, H., Xu, J., Wang, P., and Zhang, B. (2012). A Novel flushing Strategy for Diatom Bloom Prevention in the Lower-Middle Hanjiang River. Water Res. 46, 2525–2534. doi:10.1016/j.watres.2012.01.051
Yang, Q., Xie, P., Xu, J., Shen, H., Zhang, M., Wang, S., et al. (2011). Research Advances of Diatom Blooms in Rivers. Resour. Environ. Yangtze Basin 20, 159–165.
Yin, D., Huang, W., Wu, X., and Song, L. (2012). Preliminary Study on Biological Characteristics of Spring Diatom Bloom in the Hanjiang River. J. Yangtze River Scientific Res. Inst. 29, 6–10.
Zeng, H., Song, L., Yu, Z., and Chen, H. (2006). Distribution of Phytoplankton in the Three-Gorge Reservoir during Rainy and Dry Seasons. Sci. Total Environ. 367, 999–1009. doi:10.1016/j.scitotenv.2006.03.001
Zhang, C., Wang, J., Zhu, D., Shi, X., and Wang, X. (2008). The Preliminary Analysis of Nutrients in Harmful Algal Blooms in the East China Sea in the spring and Summer of 2005. Acta Oceanologica Sinica 30, 153–159.
Zheng, L.-L., Song, L.-R., Wu, X.-H., and Zhuang, H.-R. (2009). Analysis of Morphology and 18s Rdna Gene from the Causative Specie Related Diatom Bloom in Hanjiang River. Acta Hydr Sin 33, 562–565. doi:10.3724/sp.j.1035.2009.00562
Zhou, J., Zhang, M., and Lu, P. (2013). The Effect of Dams on Phosphorus in the Middle and Lower Yangtze River. Water Resour. Res. 49, 3659–3669. doi:10.1002/wrcr.20283
Zhou, M., Zhu, M., and Zhang, J. (2001). Status of Harmful Algal Blooms and Related Research Activities in China. Chin. Bull. Life Sci. 13, 54–59.
Keywords: river algal blooms, time-lag effect, drive factors, river ecosystem, Hanjiang River
Citation: Liu C, Chen Y, Zou L, Cheng B and Huang T (2022) Time-Lag Effect: River Algal Blooms on Multiple Driving Factors. Front. Earth Sci. 9:813287. doi: 10.3389/feart.2021.813287
Received: 11 November 2021; Accepted: 06 December 2021;
Published: 24 January 2022.
Edited by:
Guobin Fu, CSIRO Land and Water, AustraliaCopyright © 2022 Liu, Chen, Zou, Cheng and Huang. This is an open-access article distributed under the terms of the Creative Commons Attribution License (CC BY). The use, distribution or reproduction in other forums is permitted, provided the original author(s) and the copyright owner(s) are credited and that the original publication in this journal is cited, in accordance with accepted academic practice. No use, distribution or reproduction is permitted which does not comply with these terms.
*Correspondence: Chengjian Liu, Y2hlbmdqaWFubGl1QHN0dW1haWwubnd1LmVkdS5jbg==
Disclaimer: All claims expressed in this article are solely those of the authors and do not necessarily represent those of their affiliated organizations, or those of the publisher, the editors and the reviewers. Any product that may be evaluated in this article or claim that may be made by its manufacturer is not guaranteed or endorsed by the publisher.
Research integrity at Frontiers
Learn more about the work of our research integrity team to safeguard the quality of each article we publish.