- 1Department of Geological Sciences, Jackson School of Geosciences, The University of Texas at Austin, Austin, TX, United States
- 2Insitute for Geophysics, Jackson School of Geosciences, The University of Texas at Austin, Austin, TX, United States
Correlations of rock-physics model inputs are important to know to help design informative prior models within integrated reservoir-characterization workflows. A Bayesian framework is optimal to determine such correlations. Within that framework, we use velocity and porosity measurements on unconsolidated, dry, and clean sands. Three pressure- and three porosity-dependent rock-physics models are applied to the data to examine relationships among the inputs. As with any Bayesian formulation, we define a prior model and calculate the likelihood in order to evaluate the posterior. With relatively few inputs to consider for each rock-physics model, we found that sampling the posterior exhaustively to be convenient. The results of the Bayesian analyses are multivariate histograms that indicate most likely values of the input parameters given the data to which the rock-physics model was fit. When the Bayesian procedure is repeated many times for the same data, but with different prior models, correlations emerged among the input parameters in a rock-physics model. These correlations were not known previously. Implications, for the pressure- and porosity-dependent models examined here, are that these correlations should be utilized when applying these models to other relevant data sets. Furthermore, additional rock-physics models should be examined similarly to determine any potential correlations in their inputs. These correlations can then be taken advantage of in forward and inverse problems posed in reservoir characterization.
Introduction
Correlations of input parameters in a rock-physics model is always of concern, particularly when a model contains multiple inputs. Analysis of those correlations, however, is often performed in trial-and-error fashion when visually fitting a model to data. In doing so, any quantitative links among the input parameters could be overlooked inadvertently. The purpose of this work is to identify significant correlations among model parameters. This process is done for established rock-physics models. Three are pressure-dependent models, and three are porosity-dependent models, each used in conjunction with well characterized and controlled laboratory data. The model parameters are tested within a Bayesian framework where the models drawn from the posterior are used to identify most likely parameters or combinations of parameters when quantitatively fitting the models to data. This aspect is important within the context of reservoir characterization, and the analyses demonstrated here would allow us to define informative prior distributions within relevant workflows.
Many examples consist of a Bayesian formulation or framework to estimate rock properties and/or facies from seismic or other geophysical data. A short list includes Buland and Omre (2003); Spikes et al. (2007); Bosch et al. (2010); Rimstad et al. (2012); Ray et al. (2013); Kemper and Gunning (2014); Jullum and Kolbjørnsen (2016); de Figueiredo et al. (2017); Grana et al. (2017); de Figueiredo et al. (2018); Grana (2018); de Figueiredo et al. (2019); Grana (2020); and Nawaz et al. (2020). In all these efforts, the end goal is to obtain an estimate and the associated uncertainty of some facies and/or rock-property distributions from subsurface data. Moreover, these efforts must address disparate spatial scales and measurement types that must be reconciled to provide the estimate and associated uncertainty of subsurface rock properties (e.g., Ba et al., 2013; Pang et al., 2019; and ; Pang et al., 2021). These methods include the explicit use of a rock-physics model within the formulation or not (e.g., Avseth et al., 2005; Mavko et al., 2020); and Grana et al., 2021). The work we present here is different and unique because it directly examines the fit of rock-physics models to controlled laboratory data with the intention to determine correlations among the input parameters. Prior and posterior models are the underpinning of the approach, but the related information regarding linkages among the parameters is the primary emphasis. The results suggest which model might be easier or better to use than the others in a qualitative sense. Importantly, the results include previously unknown correlations for the three pressure-dependent and three porosity-dependent rock-physics models under consideration. This work is by no means exhaustive in terms of the examination of multiple models or data sets. The results for the rock-physics models studied here should be used with other relevant data. Furthermore, the method should be applied to other models and appropriate data to determine correlations among their inputs.
Conventional inversion techniques based on local optimization methods are prone to being trapped in local minima of the objective function closest to the starting model. In those techniques, uncertainty is estimated using the covariance matrix computed at the optimal (local) model. First, this approach gives incorrect estimates when the optimization becomes trapped in one of the local minima. Second, even if the optimization can find the globally optimal solution, covariance-based uncertainty estimates would be incorrect in that the uncertainties would be underestimated (e.g., Sen and Stoffa, 1996). The approach taken here is a sampling approach in which we carry out exhaustive sampling. Therefore, our approach is not prone to becoming trapped in local minima.
Materials and Methods
Data
We use the published data set from Zimmer (2003) and Zimmer et al. (2007a); Zimmer et al. (2007b), comprised of clean, dry, unconsolidated sands. Laboratory measurements consisted of compressional-and shear-wave velocities and porosity as a function of pressure for 15 different samples. Eight of those samples were reconstituted sands, named Gulf of Mexico, Pomponio, Santa Cruz Dry 1, Santa Cruz Dry 2, Sa Big Dry, SA Small Dry, Galveston Bay, and Merritt. Figure 1A is a plot of all those data sets of P-wave velocity (
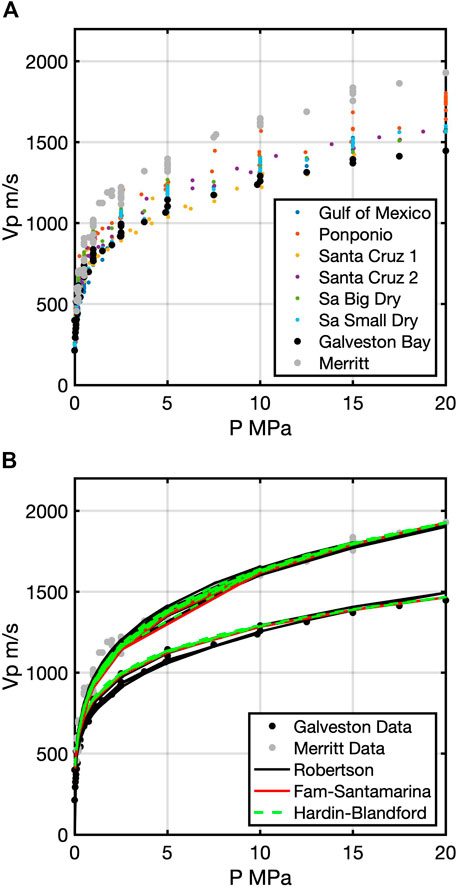
FIGURE 1. (A) Plot of P-wave velocity as a function of pressure for all the reconsolidated sand samples. The two used in the study here are the Galveston Bay data (black points) and the Merritt data (gray) points. In (B) the Galveston Bay and Merritt are replotted and overlain on them are the Robertson model (solid black line), Fam-Santamarina (red), and Hardin-Blandford (green). All three have a similar qualitative fit to the two data sets.
For the porosity-dependent models, we use all data from all reconstituted sands and glass beads. Figure 2A shows the compressional modulus (
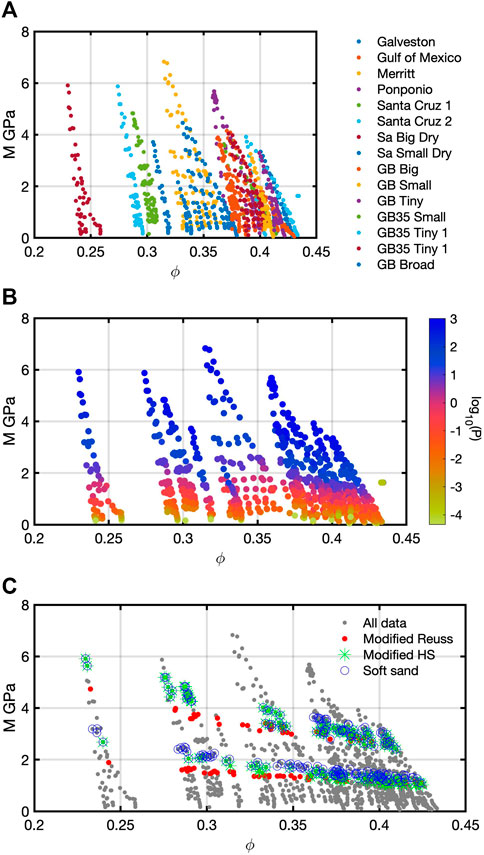
FIGURE 2. (A) Measured data (compressional modulus M) as a function of porosity color-coded by sample name. Sa refers to Santa Cruz, and GB refers to glass bead followed by the size. (B) The same data as in a) except color-coded by the log10(P) for all 15 samples. For the rock-physics models used in the Bayesian analysis, slightly different subsets of the data are necessary to select. An initial model is visually fit, and then data within a 3% of that model are selected to use with that particular model (modified Reuss, modified Hashin-Strikman, and soft sand). We use two subsets (C) that correspond to different pressures and the different models.
Models
Pressure-Dependent Models
Two models are used to represent
In Equation 1,
The Fam and Santamarina (1997) Model is
For this model, the pressures are defined as the same as in Eq. 1. The
The Hardin and Blandford (1989) model (HB) in Eq. 3,
is a function of elastic moduli rather than velocity. These moduli could be the bulk, shear, or compressional moduli. The terms of pressure and
Porosity-Dependent Models
Three rock-physics models were used in the Bayesian analysis. The first two were published in Zimmer et al. (2007b). Those two models are the modified Reuss
and modified Hashin-Strikman (HS) models
In Equation 4,
Equations 5, 6 are
The third model used for porosity and pressure is the soft-sand model (Dvorkin and Nur, 1996), which was not used in the Zimmer et al. (2007b) paper. We introduce it here to demonstrate the effects of additional input parameters, beyond the number in the modified Reuss and Hashin-Strikman models. The soft-sand model combines Hertz-Mindlin (Mindlin, 1949) contact theory with modified Hashin-Strikman formulations to represent unconsolidated sands. The Hertz-Mindlin theory expresses the effective bulk and shear moduli,
Included in these equations are the mineral shear modulus and Poisson’s ratio (
Bayesian Analysis
We reproduced a subset of the models published in Zimmer et al. (2007a). We used the Galveston Bay sand sample and fit Eqs 1–3 using the published values for the fitting parameters (Table 1). Then we applied Bayesian analysis to determine which inputs into the rock physics model have correlations among them. This approach includes a quantitative match of model to data. More specifically (i.e., Ulrych et al., 2001; Tarantola, 2005; Sen, 2006; Sen and Stoffa, 2013) we compute the likelihood (l(d|m)) with a given prior (p(m)), whose product is proportional to the posterior probability distribution (
Where d is the data vector, and m is the model. The likelihood function is
Where

TABLE 1. Published values (Zimmer et al., 2007a) of the model parameters for the three pressure-dependent models for the Galveston Bay sample (GB) and the Merritt sample (M).
In Equation 11, g(m) is the forward modeling operator on the model, and CD is the data covariance matrix (assuming Gaussian noise). The operator g(m) is a rock physics model in this case, where the inputs into that model are contained in m as a vector. The data-model comparison in Eq. 11 is equivalent to a least-squares fit of the model to the data. Equation 12 is the Gaussian prior where mprior is the prior model mean, and CM is the prior model covariance matrix.
The number of model parameters (three) is small for each of the models. Therefore, we find it convenient to sample exhaustively from the PPD rather than Monte Carlo Markov chain sampling. In other words, we draw many samples from the prior and evaluate the PPD for each model using Eq. 9. None of the rock-physics models are linear. Accordingly, the likelihood function becomes non-Gaussian, so an analytical solution for the posterior cannot be computed.
For the pressure-dependent problem, a trivariate Gaussian random distribution is used as the prior pdf. However, a sufficient number of models must be drawn, but the time to run a sufficient number of models must be considered. The number N = 300 was determined via trial and error to be sufficient that did not require significant computation time, and this size was used for the three rock-physics models. In addition to the size of the model, the prior mean model and covariance matrix are user defined. The means were set to the values in Table 1. The covariance matrices, however, had to be tested and determined via trial and error. This process was necessary to ensure that the collection of computed rock-physics models fell reasonably close to the data and without significant scatter among them. The posterior is a trivariate histogram of size 300 × 300 × 300. That size comes from calculating a model for every combination of inputs. We analyze bivariate joint histograms of the prior model and posterior, so any pair of values must be considered together rather than one conditional upon the other.
The number of model parameters for the porosity-dependent rock-physics models is two, three, and four, where each is a relatively small number. We again sampled exhaustively the PPD. The prior model is a multivariate Gaussian random distribution for each rock-physics model. Via trial and error, the number 2 × 2000 was determined sufficient for the modified Reuss model, where the 2 is number of inputs, and 2000 is the length of the prior model. For the modified HS model, the size 3 × 750 was deemed sufficient. Last, for the soft-sand model, 4 × 125 was found to be a sufficient number. Because of the exhaustive sampling, the size of the posterior for the modified Reuss is 2000 × 2000; 750 × 750 × 750 for the modified HS, and 125 × 125 × 125 × 125 for the soft-sand model. For analysis of the results, we examine bivariate joint histograms computed from the posterior alongside bivariates of the prior model.
For the porosity-dependent models, the user most also define the mean of the prior model and its covariance matrix. Table 2 contains the mean values. For the modified Reuss model, the two values come from the highest porosity sample at that pressure, which is the

TABLE 2. Mean values for the multivariate random Gaussian prior models for data set 1 and data 2 for the three porosity-dependent rock-physics models.
Starting Models and Data Selection
For the porosity-dependent models, the selected data in Figure 2C had to be chosen for each of the three rock-physics models. If the data were too far away from the forward models, the objective function becomes large, and the likelihood and posterior values become very small. To select the data, a starting model was computed using the mean values in Table 2. Then data were selected based on 3% around the starting model. The data covariance (CD ) was estimated assuming 5% error in the data. The starting models are displayed in relevant subsequent figures. Table 3 contains the number of selected data points for data sets 1 and 2 for the three rock-physics models.
Results
Pressure-Dependent Models
As aforementioned, the first step is to reproduce the models from Zimmer et al. (2007a). Figure 1B shows the fit of Eqs 1–3 to the Galveston Bay (black points) and Merritt (gray points) data. The solid black lines are the R model fits to the Galveston Bay and Merritt data. Red and green dashed lines are the FS and HB models, respectively, fit to the two data sets. Table 1 contains the fitting coefficients from Zimmer et al. (2007a) for the three models.
We applied the Bayesian formulation for the three models to the Galveston Bay data. The length of the Galveston Bay data vector was 58x1, and CD was estimated assuming 5% error in the data, which is typical of in these types of laboratory measurements. For the R model, the three dimensions of the trivariate normal random distribution corresponded to A, B, and n. First, m was generated, where the means for the three variables are the A, B, and n values in Table 1 and a covariance matrix with diagonal [50 (m/s) 50 (m/s) 0.0002]. Off-diagonal terms were zero. The size of m was 3x300, so 3003 models were evaluated.
Figures 3A,B contain the prior model and posterior bivariate histograms, respectively, for A-B. Figures 3C,D correspond to the bivariate histograms for variables A-n, and 3E and 3F to B-n. In all the bivariate histograms for the prior model, one peak is apparent that is close to the mean. These histograms for the prior model are Gaussian although they might not always appear to be because the full trivariate has been marginalized in one direction. The posterior histogram for A-B shows a clear unimodal distribution peaking at a point different from the prior model mean. The posterior for A-n or B-n, however, is broad over A (or B) but shows a narrow range for n. When these posterior histogram for A and B is displayed in a logarithmic plot, it appears Gaussian, but the linear plot much more clearly identifies the highest peak. For the other two posterior histograms on a logarithmic plot, more than one value of n corresponds to broad ranges of A or B. Again, the linear plot by far indicates the n value with the highest frequency.
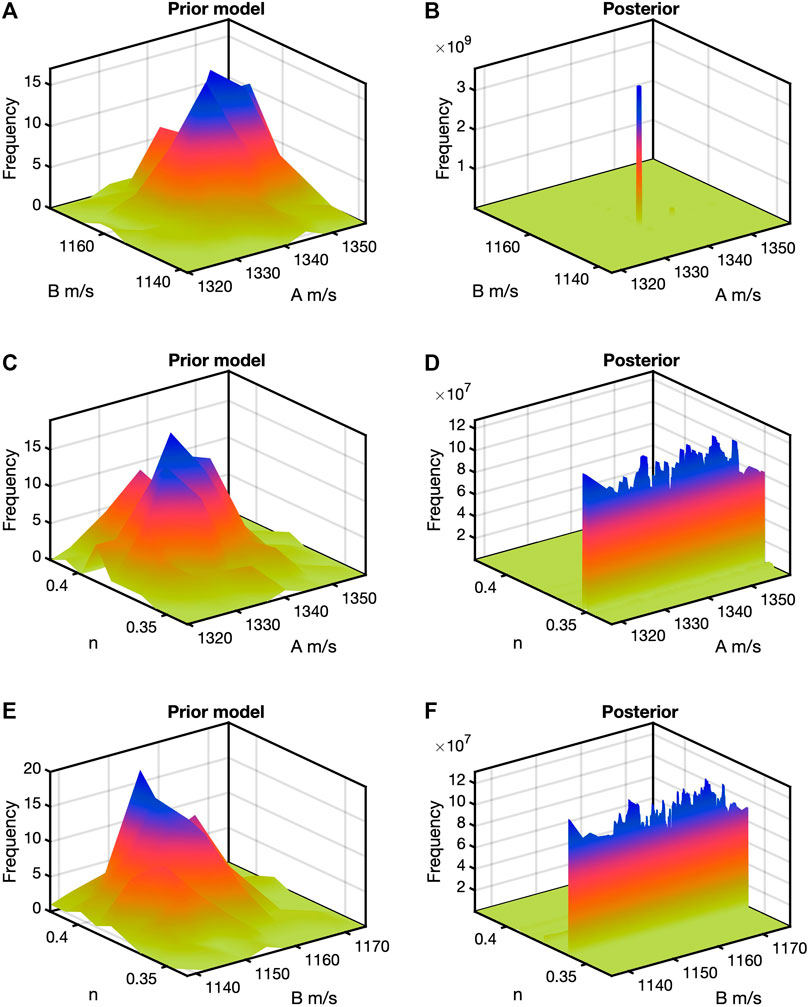
FIGURE 3. Bayesian analysis results for the Robertson model. In (A,B) are the bivariate prior model histogram and posterior histogram, respectively, for the joint A-B pair. The peak of the prior model histogram is at the mean, but the isolated peak in the posterior does not fall at the mean. The model and posterior bivariate distributions for the A-n pair are in (C,D), respectively. The prior model is a normal distribution by design, but the posterior indicates a very narrow range for n with and wide range of A values. The B-n pairs in (E,F) resemble those in (C,D).
The same procedure used with the R model was repeated using the FS and HB models. For both, the size of m was 3x300, and the means for m are in Table 1 for S, n, and k. The diagonal of the covariance matrix for m in the FS model was [43.7 (m/s) 0.0005 0.0011]. For the HB model, the covariance diagonal for m was [145 (MPa) 0.0004 0.0032].
Figure 4 shows the results for the FS model where the layout is the same as Figure 3 except for the different variables. Figures 4A,B are the prior model and posterior for the S-n pair, respectively, 4C and 4D for S-n, and 4E and 4F for n-k. Of the three posterior histograms, the S-n pair shows one clear peak, and the other two show a narrow for k with wider ranges for S or n. Bivariate histogram results for the HB model are in Figure 5. The prior model bivariate histogram for the S-n pair is in Figure 5A and the posterior in Figure 5B. The posterior shows a narrow range for n and a wide range of S (Figure 5B). In Figure 5C (the S-k pair), the central peak is at the mean value, and the corresponding posterior (Figure 5D) shows an isolated S-k pair with high frequency away from the prior model mean. The histogram in Figure 5F shows a narrow range of n for a wide range of k.
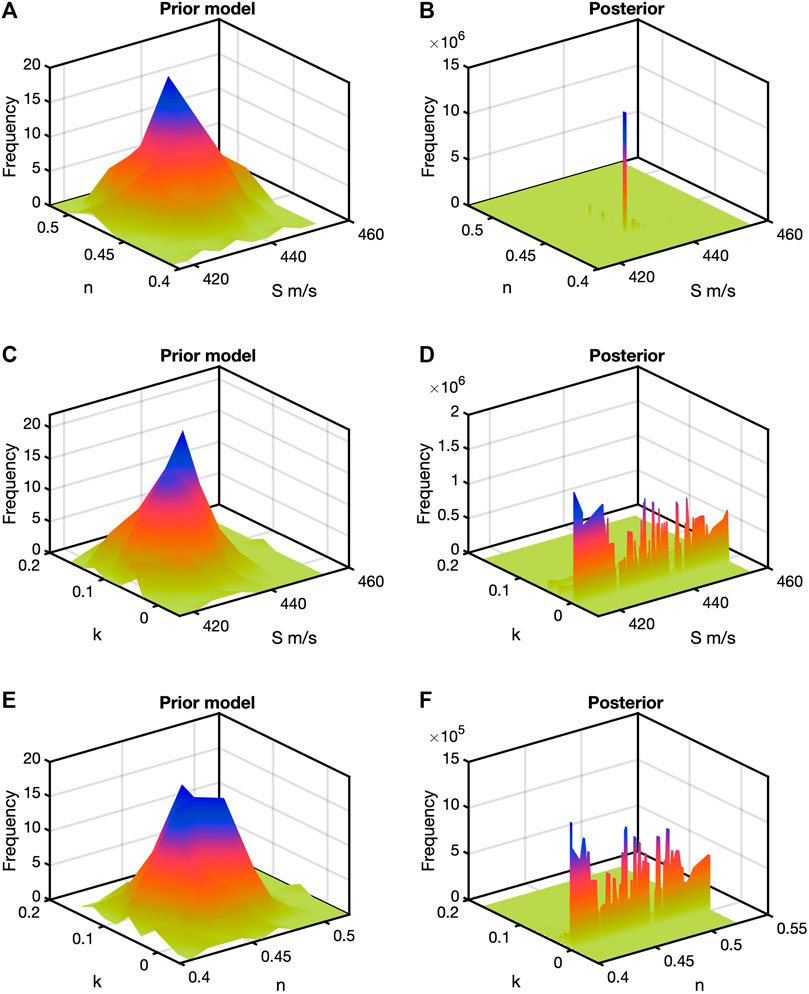
FIGURE 4. Bivariate histograms for the Fam-Santamarina model. (A,B) correspond to the S-n, pair, (C,D) to S-k, and (E,F) to n-k. The posterior histogram for S-n (B) shows an isolated high-frequency peak away from the mean in (A). In the other two posterior plots, a narrow range of k shows large frequencies for a wide range of S (E) and n (F).
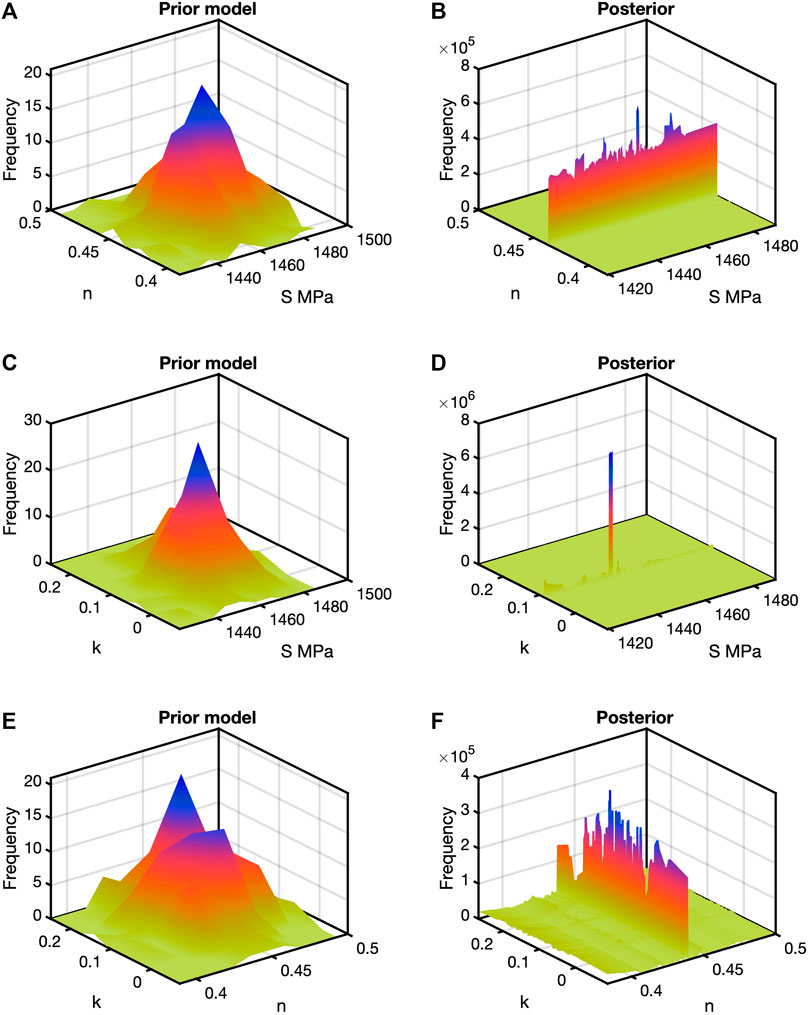
FIGURE 5. Model and posterior histograms for the Hardin-Blandford results. The juxtaposition is the same as in Figure 5. Different from the Fam-Santamarina model, the posterior in (A) shows a narrow range of n and wide range of S. The posterior in (D) shows an isolated peak for the S-k pair. Last the posterior in (F) show a narrow range of n for a wide range of k.
Next, we ran the three rock physics models for 100 trials with the Galveston Bay data where m was different for each trial. The mean and covariance matrices for each m remained the same, but the values in the random trivariate normal distribution differed from trial to trial. In all cases, the model size was 3x300. For each trial for the R model, the A-B pair corresponding to the highest frequency was selected using the information in the A-B posterior histogram in Figure 3B. To obtain n, the A-n posterior histogram maximum value was used (Figure 3D). Figure 6A exhibits these results, which indicate correlation among the three variables. Figure 6B displays the data, a selection of prior models (blue), and in gray the 100 maximum a posterior (MAP) results from the R model computed from the points in Figure 6A. The number of prior models is a small fraction of 1% of the number of computed models. Figures 6C,D show the corresponding plots for the FS model. The highest-frequency inputs for Figure 6C came from Figure 4B for S and n and 5F for k. Figures 6E,F show the same type of results for the HB model. Inputs for Figure 6E came from the values of S and k in Figure 5D and n from Figure 5F. Of the three, the MAP models in Figure 6B overall cluster more closely to the data than do the models in Figures 6D,F.
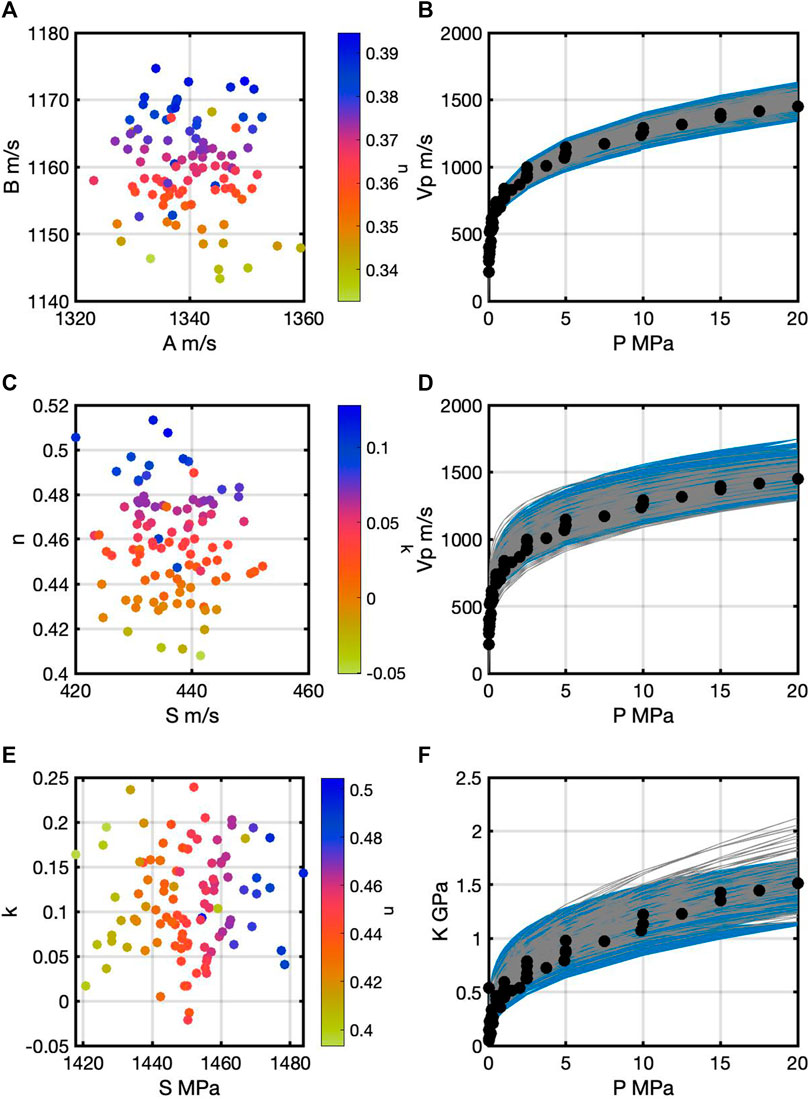
FIGURE 6. (A) Most likely values of A and B color coded by n for 100 trials of the Robertson model on the Galveston data. Correlation is evident among the three parameters. (B) contains the Galveston Bay data (points), a selection of prior models (gray), and MAP models (gray) from the 100 trials for each most likely value of A, B, and n from (A). The plots in (C) contains most likely values for the Fam-Santamarina model; in (D) are a selection of prior models (blue), and the 100 MAP models (gray). Last, the corresponding plots for the Hardin-Blandford model are in (E,F).
We then applied the Bayesian formulation for the three pressure-dependent rock-physics models to the Merritt data (Figure 1). The length of the Merritt data vector was 112 × 1, and CD was estimated based on 5% data error. For the R model, the means for the three variables are the A, B, and n values in Table 1 and a covariance matrix with diagonal [50 (m/s) 50 (m/s) 0.0008]. Off-diagonal terms were zero. The size of m was 3 × 300, so 3003 models were evaluated. The means for m are in Table 1 for S, n, and k for the FS and HB models. The diagonal of the covariance matrix for m in the FS model was [59.9 (m/s) 0.0009 0.0001]. For the HB model, the covariance diagonal for m was [352 (MPa) 2x10−6 9.6−8].
Results for the three rock-physics models applied to the Merritt data resemble those from the Galveston Bay data. Posterior bivariate histograms show overall similar features except for different values for the inputs. For the sake of the space, individual prior and posterior histograms are not displayed. Figure 7 contains plots akin to those in Figure 6 where most likely data pairs are selected for each model from the 100 trials; the corresponding MAP models and selections of prior models are also plotted with the Merritt data. Correlations among the R model inputs are similar for the Merritt (Figure 7A) and Galveston (Figure 6A) data. The MAP models in Figure 7B come from those points in Figure 7A. The corresponding plots from the FS model are in Figures 7C,D; for the HB model, the plots are Figures 7E,F.
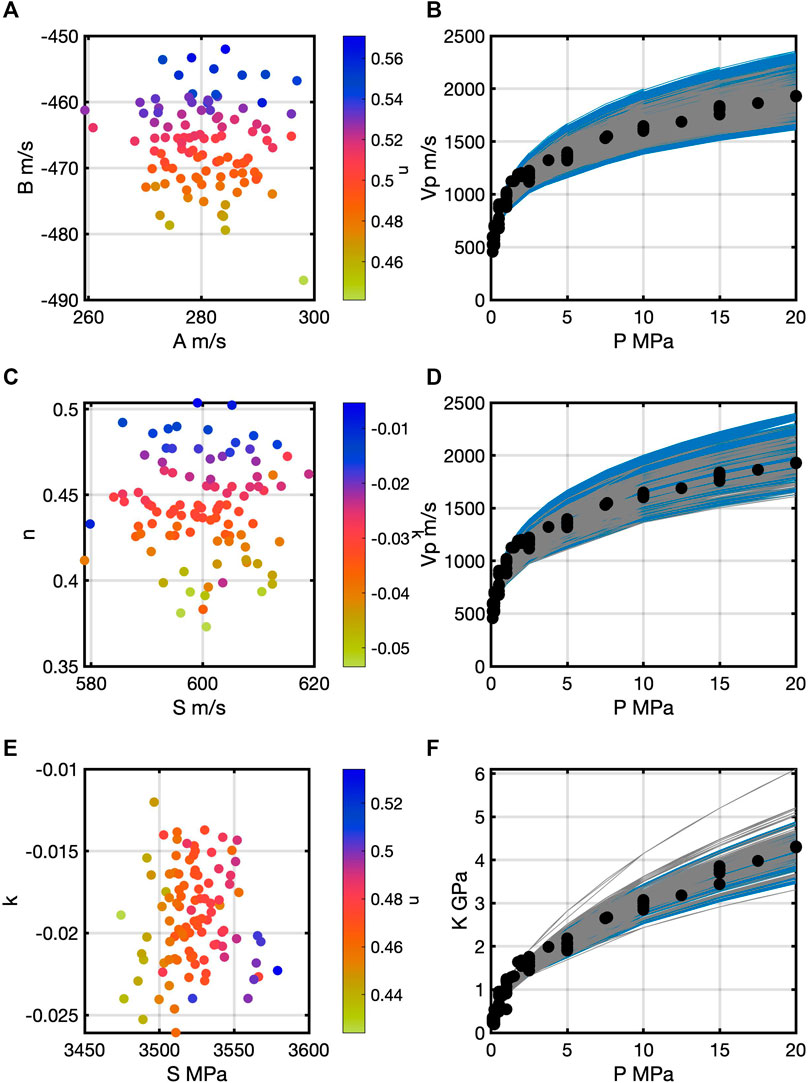
FIGURE 7. (A) Scatter plot of most likely values of A and B color coded by n for 100 trials of the Robertson model on the Merritt data. Correlation among the three parameters is present much like Figure 6A. In (B) are the Merritt data, a selection of prior models (blue), and the MAP models (gray) from the 100 trials for each most likely value of A, B, and n. In (C,D) are corresponding plots for the Fam-Santamarina model and the Hardin-Blandford model in (E,F).
Porosity-Dependent Models
We applied the Bayesian analysis first for the modified Reuss model to data set 1 (Figure 2C). The mean of m is in Table 2 along with a covariance matrix with diagonal [3.818x10−4 0.010 (GPa)]. Off-diagonal terms were zero. Table 3 shows the number of data points. Figure 8A is the prior model bivariate histogram, and Figure 8B is the corresponding posterior bivariate histogram for the modified Reuss model applied to data set 1. The prior histogram is a random 2D Gaussian, and the posterior shows a narrow range of
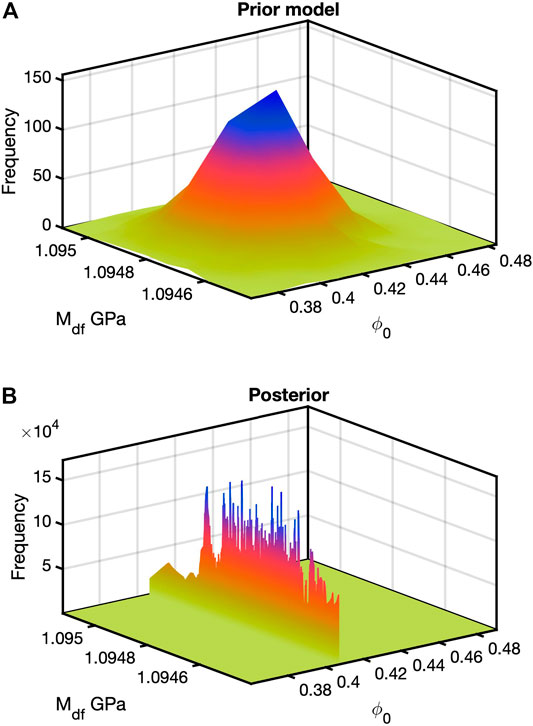
FIGURE 8. Bivariate histograms for the prior model (A) and posterior (B) for data set 1 and the modified Reuss model. In this case, the prior model and posterior are two dimensional. The joint bivariate in b) contains a very narrow range of
Next for data set 1, the Bayesian analysis was run using the modified HS model. Table 2 contains the mean of m. The diagonal of the covariance matrix was [2.121 × 10−4 0.017 (GPa) 0.008 (GPa)] with off-diagonal terms set to zero. Figure 9 contains six bivariate histograms, for three prior models and three posteriors. With the number of input terms at three, the prior model and the full posterior are trivariate. Thus, we marginalize the prior and posterior in one direction to obtain the bivariates for display. Figures 9A,B are the prior model and posterior, respectfully, for
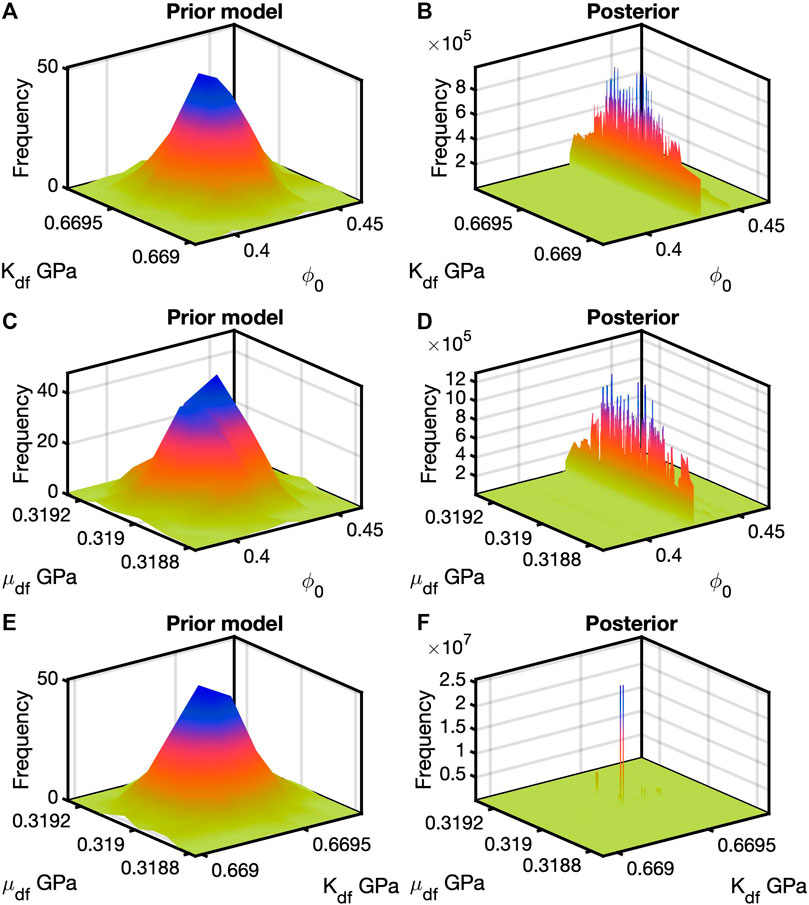
FIGURE 9. Example results from the modified HS model applied to data set 1. Bivariates for
The third porosity-dependent model applied to data set 1 was the soft-sand model. The diagonal of the covariance matrix was [0.006 (MPa) 0.002 0.030 0.015] with off-diagonal terms set to zero. Table 2 contains the mean values for the four variables. Figure 10 exhibits 12 bivariate histograms, half prior models and half posteriors. This number comes from computing each pair from the 4D full posterior. Figures 10A–C are prior model histograms for the pairs of P with
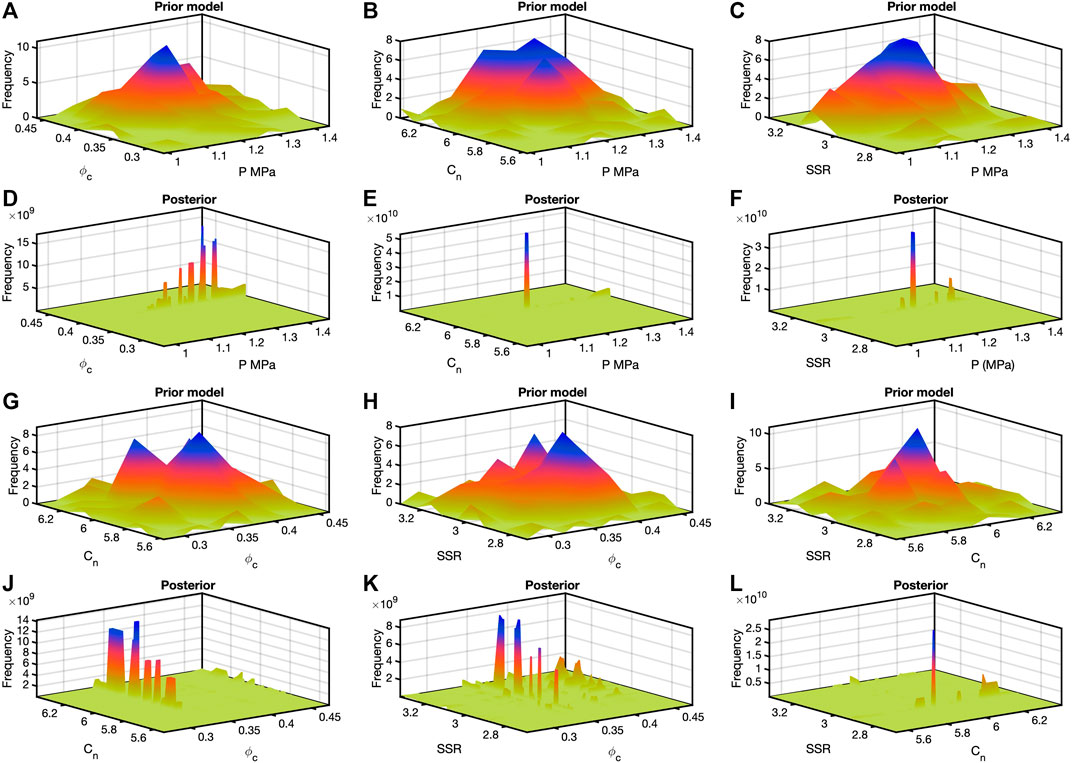
FIGURE 10. Soft-sand model and data set 1 results. Plots (A–C) are the bivariate prior model histograms for P versus
An additional application for data set 1 was to run 100 trials of each rock-physics model. Within each trial, a different m was generated. The mean and covariance matrix were the same each time, but the randomly generated values for each trial were different. The results from each trial are the sets of bivariate histograms such as those in Figures 8–10. Values for inputs at maximum frequencies were selected from the appropriate bivariate posterior for each trial. For the modified Reuss, the cross plot in Figure 11A shows little to no correlation between
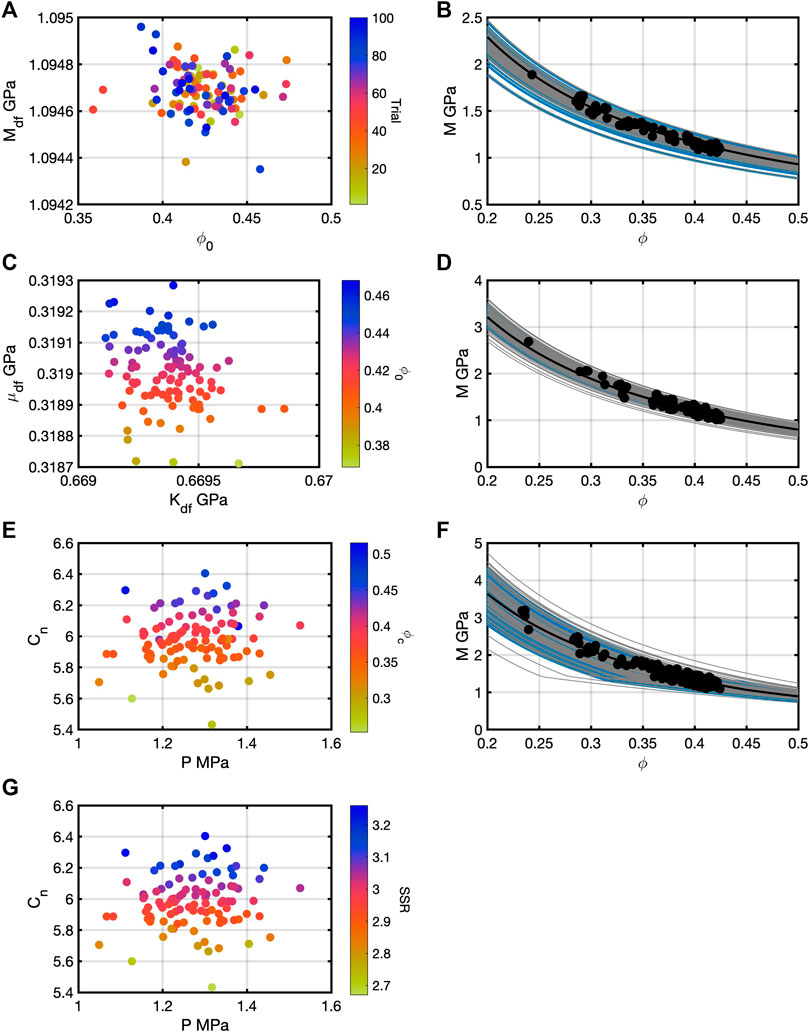
FIGURE 11. The pair of
Similarly for the modified HS model, Figure 11C shows the scatter plot of
We applied the Bayesian framework to data set 2 using each of the rock-physics models. The sizes of m stay the same as they were for applications to data set 1. The general appearance of the results for data set 2 is much like the posterior bivariate histogram for data set 1. Thus, we do not display them here.
Finally, each rock-physics model was applied to data set 2 in 100 independent trials. Each trial introduced a different random m but with the same mean and covariance matrix. Values of the inputs were selected from the posterior histograms as described for the MAP models in 11. Figure 12 contains the scatter plots of the inputs and the 100 posterior models. Figure 12A shows the lack of correlation between
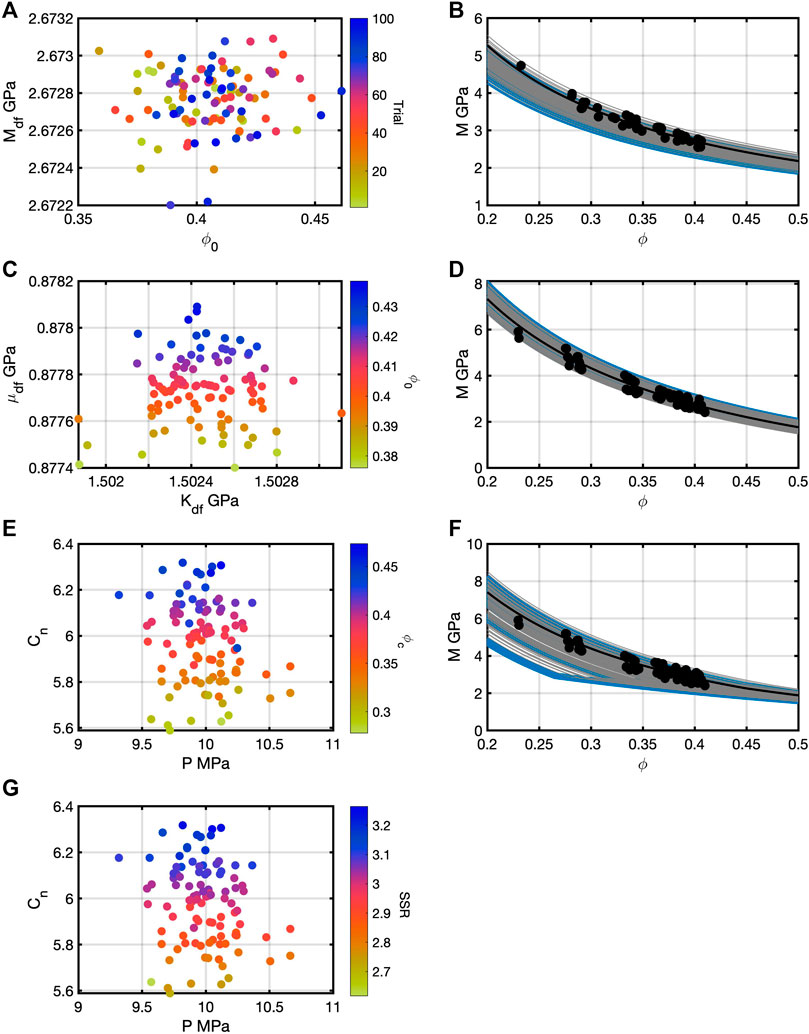
FIGURE 12. After 100 trials for the three models for data set 2, the highest frequency pairs were extracted as in Figure 11 for data set 1. (A) corresponds to the extracted values; (B) contains the starting model (black), a subset of prior models (blue), and MAP models (gray) for the modified Reuss. (C,D) contain those for the modified HS model. (E–G) contain those for the soft-sand model. Correlations are easily identifiable in the modified HS and soft-sand model parameters.
Discussion
The results of the Bayesian framework for the all the rock-physics models indicate correlations among their inputs that were not known previously. Specific to the R model, all three terms are correlated (Figures 6A, 7A). These correlations are not apparent when looking at the equation of the model, the sensitivities, or by looking at one set of bivariate histograms (e.g., Figure 3). After multiple trials each with a different m, the correlation pattern emerges. These correlations show up with both data sets but of course with different values for the three terms. Similar arguments can be made for the FS and HB models. Any given set of posterior histograms provides for an optimally fit model. Only after conducting many trials do the correlations among the inputs become evident. For these three models, all could be recast as linear in the log-log domain. The data could be plotted in the that domain as well, and the Bayesian analysis could have been performed in terms of fitting a line in the log-log domain. We did not do this because the velocity sensitivity as a function of pressure cannot be captured fully in the log-log domain.
When the covariance matrix of m was tested by trial and error for the three models, the R model was the easiest among the three models to find suitable values for the matrix. This situation likely occurred because A and B are coefficients, and n is an exponent. In the other two models, S is a coefficient, and n and k are exponents. The MAP models in Figures 6, 7 indicate this pattern. In Figure 6 especially, the posterior models for the R model clearly are better matches to the data than for the FS and HB models. In Figure 7, arguably all three sets of MAP models show about the same amount of scatter at higher pressures. The Merritt data set had nearly twice the number of data points as the Galveston Bay data set, and many more points are present at lower pressures, so a slight bias in fitting exists for the lower pressure data points.
Specific to the modified Reuss model, the two inputs are not clearly correlated (Figures 11A, 12A). After 100 trials each with a different m, no correlation pattern becomes clear with both data sets although different values for the two terms change. Correlations among the three inputs of the modified HS model emerged after the 100 trials. Within each trial, the appearances of the posterior histograms were similar with a lone high-frequency peak in the
When using these porosity-dependent rock-physics models with two, three, and four parameters, obviously providing two inputs versus three or four is easier. These models, used in a porosity-dependent manner, help to estimate porosities from velocity not observed in a data set. Of the three, one question that could arise would be which one is the most reliable? A potential answer could be inferred from the MAP models in Figures 11, 12. In both figures for the two data sets, the modified HS posterior models show the least scatter among themselves. Extrapolation to lower porosities might be more reliable from this model relative to the modified Reuss and soft-sand models. That said, if these models were used in studies that dealt with variable compositions and fluid saturations, they would need to be examined in the Bayesian framework with an appropriate data set.
This study identifies correlations among input parameters of pressure- and porosity-dependent rock-physics models. The Bayesian framework provided the means to establish these correlations. In a seismic-inversion or reservoir-characterization scheme, these correlations could be used to construct informative prior distributions. A user of any of the models examined here should be aware of these correlations. Exact values of the inputs will vary depending on the particular data set, but at least some simple templates could be defined to guide the setting of the values. Additional pressure- and porosity-dependent models should be examined using the Bayesian framework because the results obtained here might not be exactly relevant for other models. Furthermore, other types of rock-physics models, such as inclusion-based or excess-compliance models, would have to be examined in a similar fashion using appropriate data sets.
Data Availability Statement
Publicly available datasets were analyzed in this study. This data can be found here: doi:10.1190/1.2399459 and doi:10.1190/1.2364849.
Author Contributions
KS wrote the codes, the article, and generated the figures MS provided initial motivation for the work, edited the articles, and critiqued the results.
Conflict of Interest
The authors declare that the research was conducted in the absence of any commercial or financial relationships that could be construed as a potential conflict of interest.
Publisher’s Note
All claims expressed in this article are solely those of the authors and do not necessarily represent those of their affiliated organizations, or those of the publisher, the editors, and the reviewers. Any product that may be evaluated in this article, or claim that may be made by its manufacturer, is not guaranteed or endorsed by the publisher.
References
Avseth, P., Mukerji, T., and Mavko, G. (2005). Quantitative Seismic Interpretation: Applying Rock Physics Tools to Reduce Interpretation Risk. Cambridge, England: Cambridge University Press, 359.
Ba, J., Cao, H., Carcione, J. M., Tang, G., Yan, X.-F., Sun, W.-t., et al. (2013). Multiscale Rock-Physics Templates for Gas Detection in Carbonate Reservoirs. J. Appl. Geophys. 93, 77–82. doi:10.1016/j.jappgeo.2013.03.011
Bosch, M., Mukerji, T., and Gonzalez, E. F. (2010). Seismic Inversion for Reservoir Properties Combining Statistical Rock Physics and Geostatistics: A Review. Geophysics 75 (5), 75A165–75A176. doi:10.1190/1.3478209
Buland, A., and Omre, H. (2003). Bayesian Linearized AVO Inversion. Geophysics 68 (1), 185–198. doi:10.1190/1.1543206
de Figueiredo, L. P., Grana, D., Bordignon, F. L., Santos, M., Roisenberg, M., and Rodrigues, B. B. (2018). Joint Bayesian Inversion Based on Rock-Physics Prior Modeling for the Estimation of Spatially Correlated Reservoir Properties. Geophysics 83, M49–M61. doi:10.1190/geo2017-0463.1
de Figueiredo, L. P., Grana, D., Roisenberg, M., and Rodrigues, B. B. (2019). Multimodal Markov Chain Monte Carlo Method for Nonlinear Petrophysical Seismic Inversion. Geophysics 84, M1–M13. doi:10.1190/geo2018-0839.1
de Figueiredo, L. P., Grana, D., Santos, M., Figueiredo, W., Roisenberg, M., and Schwedersky Neto, G. (2017). Bayesian Seismic Inversion Based on Rock-Physics Prior Modeling for the Joint Estimation of Acoustic Impedance, Porosity and Lithofacies. J. Comput. Phys. 336, 128–142. doi:10.1016/j.jcp.2017.02.013
Dvorkin, J., and Nur, A. (1996). Elasticity of High‐porosity Sandstones: Theory for Two North Sea Data Sets. Geophysics 61 (5), 1363–1370. doi:10.1190/1.1444059
Fam, M., and Santhamarian, J. C. (1997). A Study of Consolidation Using Mechanical and Electromagnetic Waves. Géotechnique 47, 203–219. doi:10.1680/geot.1997.47.2.203
Grana, D. (2020). Bayesian Petroelastic Inversion with Multiple Prior Models. Geophysics 85 (5), M57–M71. doi:10.1190/GEO2019-0625.1
Grana, D., Fjeldstad, T., and Omre, H. (2017). Bayesian Gaussian Mixture Linear Inversion for Geophysical Inverse Problems. Math. Geosci. 49, 493–515. doi:10.1007/s11004-016-9671-9
Grana, D. (2018). Joint Facies and Reservoir Properties Inversion. Geophysics 83, M15–M24. doi:10.1190/geo2017-0670.1
Grana, D., Mukerji, T., and Doyen, P. (2021). Seismic Reservoir Modeling: Theory, Examples, and Algorithms. Hoboken, New Jersey: Wiley-Blackwell, 272.
Hardin, B. O., and Blandford, G. E. (1989). Elasticity of Particulate Materials. J. Geotechnical Eng. 115, 788–805. doi:10.1061/(ASCE)0733-941010.1061/(asce)0733-9410(1989)115:6(788)
Jullum, M., and Kolbjørnsen, O. (2016). A Gaussian-Based Framework for Local Bayesian Inversion of Geophysical Data to Rock Properties. Geophysics 81, R75–R87. doi:10.1190/GEO2015-0314.1
Kemper, M., and Gunning, J. (2014). Joint Impedance and Facies Inversion - Seismic Inversion Redefined. First Break 32 (9), 89–95. doi:10.3997/1365-2397.32.9.77968
Mavko, G., Mukerji, T., and Dvorkin, J. (2020). The Rock Physics Handbook. Third edition. Cambridge, England: Cambridge University Press, 727.
Mindlin, R. D. (1949). Compliance of Elastic Bodies in Contact. Trans. ASME 71, A–259. doi:10.1115/1.4009973
Nawaz, M. A., Curtis, a., Shahraeeni, M. S., and Gerea, C. (2020). Variational Bayesian Inversion of Seismic Attributes Jointly for Geologic Facies and Petrophysical Rock Properties. Geophysics 85 (4), MR213–MR233. doi:10.1190/GEO2019-0163.1
Pang, M., Ba, J., Carcione, J. M., Picotti, S., Zhou, J., and Jiang, R. (2019). Estimation of Porosity and Fluid Saturation in Carbonates from Rock-Physics Templates Based on Seismic Q. Geophysics 84, M25–M36. doi:10.1190/GEO2019-0031.1
Pang, M., Ba, J., Carcione, J. M., Zhang, L., Ma, R., and Wei, Y. (2021). Seismic Identification of Tight-Oil Reservoirs by Using 3D Rock-Physics Templates. J. Pet. Sci. Eng. 201, 108476. doi:10.1016/j.petrol.2021.108476
Ray, A., Alumbaugh, D. L., Hoversten, G. M., and Key, K. (2013). Robust and Accelerated Bayesian Inversion of marine Controlled-Source Electromagnetic Data Using Parallel Tempering. Geophysics 78 (6), E271–E280. doi:10.1190/geo2013-0128.1
Rimstad, K., Avseth, P., and Omre, H. (2012). Hierarchical Bayesian Lithology/fluid Prediction: A North Sea Case Study. Geophysics 77, B69–B85. doi:10.1190/geo2011-0202.1
Robertson, P. K., Sasitharan, S., Cunning, J. C., and Sego, D. C. (1995). Shear-Wave Velocity to Evaluate In-Situ State of Ottawa Sand. J. Geotechnical Eng. 121, 262–273. doi:10.1061/(ASCE)0733-941010.1061/(asce)0733-9410(1995)121:3(262)
Sen, M. K., and Stoffa, P., L. (2013). Global Optimization Methods in Geophysical Inversion. Second edition. Cambridge, England: Cambridge University Press, 289.
Sen, M. K., and Stoffa, P. L. (1996). Bayesian Inference, Gibbs' Sampler and Uncertainty Estimation in Geophysical Inversion1. Geophys. Prospect 44, 313–350. doi:10.1111/j.1365-2478.1996.tb00152.x
Spikes, K., Mukerji, T., Dvorkin, J., and Mavko, G. (2007). Probabilistic Seismic Inversion Based on Rock-Physics Models. Geophysics 72 (5), R87–R97. doi:10.1190/1.2760162
Tarantola, A. (2005). Inverse Problem Theory and Methods for Model Parameter Estimation. Paris, France: Society for Industrial and Applied Mathematics, 352.
Ulrych, T. J., Sacchi, M. D., and Woodbury, A. (2001). A Bayes Tour of Inversion: A Tutorial. Geophysics 66 (1), 55–69. doi:10.1190/1.1444923
Zimmer, M. A., Prasad, M., Mavko, G., and Nur, A. (2007a). Seismic Velocities of Unconsolidated Sands: Part 1 - Pressure Trends from 0.1 to 20 MPa. Geophysics 72, E1–E13. doi:10.1190/1.2399459
Zimmer, M. A., Prasad, M., Mavko, G., and Nur, A. (2007b). Seismic Velocities of Unconsolidated Sands: Part 2 - Influence of Sorting- and Compaction-Induced Porosity Variation. Geophysics 72, E15–E25. doi:10.1190/1.2364849
Keywords: rock physics, Bayesian analysis, correlations, informed distributions, templates
Citation: Spikes KT and Sen MK (2022) Correlations of Rock-Physics Model Parameters From Bayesian Analysis: Pressure- and Porosity-Dependent Models Applied to Unconsolidated Sands. Front. Earth Sci. 9:805742. doi: 10.3389/feart.2021.805742
Received: 30 October 2021; Accepted: 22 November 2021;
Published: 14 January 2022.
Edited by:
Giovanni Martinelli, National Institute of Geophysics and Volcanology, ItalyReviewed by:
Dario Grana, University of Wyoming, United StatesJing Ba, Hohai University, China
Hemin Yuan, China University of Geosciences, China
Copyright © 2022 Spikes and Sen. This is an open-access article distributed under the terms of the Creative Commons Attribution License (CC BY). The use, distribution or reproduction in other forums is permitted, provided the original author(s) and the copyright owner(s) are credited and that the original publication in this journal is cited, in accordance with accepted academic practice. No use, distribution or reproduction is permitted which does not comply with these terms.
*Correspondence: Kyle T. Spikes, a3lsZS5zcGlrZXNAanNnLnV0ZXhhcy5lZHU=