- 1Henan Key Laboratory of Yellow Basin Ecological Protection and Restoration, Yellow River Institute of Hydraulic Research, Zhengzhou, China
- 2State Key Laboratory of Simulation and Regulation of Water Cycle in River Basin, China Institute of Water Resources and Hydropower Research, Beijing, China
Climate changes and underlying surface of the watershed have affected the evolution of streamflow to a different degree. It is of great significance to quantitatively evaluate main drivers of streamflow change for development, utilization, and planning management of water resources. In this study, the Huangshui River basin, a typical basin of the Qinghai-Tibetan Plateau, China, was chosen as the research area. Huangshui is the largest first-class tributary in the upstream of the Yellow River. Based on the Budyko hypothesis, streamflow and meteorological datasets from 1958 to 2017 were used to quantitatively assess the relative contributions of changes in climate and watershed characteristics to streamflow change in research area. The results show that the streamflow of Huangshui River basin shows an insignificant decreasing trend; the sensitivity coefficients of streamflow to precipitation, potential evapotranspiration, and watershed characteristic parameter are 0.5502, −0.1055, and −183.2007, respectively. That is, 1 unit increase in precipitation would induce an increase of 0.5502 units in streamflow, and 1 unit increase in potential evapotranspiration would induce a decrease of 0.1055 units in streamflow, and an increase of 1 unit in the watershed characteristic coefficient would induce a decrease of 183.2007 units in streamflow. The streamflow decreased by 20.48 mm (13.59%) during the change period (1994–2017) compared with that during the reference period (1958–1993), which can be attributed to watershed characteristic changes (accounting for 73.64%) and climate change (accounting for 24.48%). Watershed characteristic changes exert a dominant influence upon the reduction of streamflow in the Huangshui River basin.
Introduction
As a significant part of the hydrological process, streamflow in many basins of the world is experiencing a decreasing trend, which is particularly evident in the Haihe River, Huaihe River, Songhuajiang River, Liaohe River, and Hanjiang River of China, and streamflow variability is generally influenced by climate change and anthropogenic activities (Wang et al., 2013; Zhang et al., 2015; Kong et al., 2016). Climate changes, such as precipitation and potential evaporation changes caused by increasing temperature, may have a crucial impact on streamflow (Huo et al., 2008). Human activities, including irrigation, land use changes, reservoir, and other hydraulic engineering, can result in significant hydrological alterations. Previous studies showed human activities are the primary driving factor of streamflow variability (Wang et al., 2012; Zhan et al., 2013). Due to the increases in human activities caused by rapid growth of economy and population in lots of places, the demand for water resources has been increased sharply over the past decades, and the decreasing trend of streamflow will continue (Velpuri and Senay, 2013). Situations of water shortage recorded have increased rapidly around the world. Recently, extreme hydrological events including floods, drought, and heavy rainfall have been reported more frequently in a changing environment (Thompson, 2012; Zhao et al., 2019). Understanding the evolution of streamflow and quantifying the individual impacts of climate change and human activities on streamflow are crucial to managers and policy-makers for sustainable water resources/basin management. Simultaneously, they are essential for the sustainable use of water resources and development of regional economics.
Researchers initially analyze components, annual and inter-annual changes of streamflow, and the correlations with climatic factors (Li and Tang, 1981; You, 1995). With the strengthening of human activities, researchers gradually consider the impact of human activities to streamflow, but it is limited to qualitative explanation (Tang, 1995; Xu, 1995; Wang et al., 2021). The sensitivity analysis of climate on streamflow is reported more frequently recently, and generally the sensitivity coefficient of streamflow to precipitation is greater than potential evaporation in many regions (Wang and Yu, 2015). Latest studies focus on quantitative assessments of runoff changes, including climate change and human activities/land use changes, and indicate that human activities are the dominant factor for runoff variability in most of the world’s rivers (Wang et al., 2012; Wang et al., 2013; Lv et al., 2019; Hu et al., 2020; Liu, 2020). Vegetation condition is the most representative factor of human activities in some areas, such as the Loess Plateau of China, the effect of vegetation was deeply conducted (Zhao et al., 2010; Li et al., 2019; Sun et al., 2020). However, the sensitivity analyses of streamflow to climatic and no-climatic factors are still rare in present studies.
In order to assess the hydrological response in a changing environment, over the past years, two primary methods have been applied to separate the effects of climate change and human activities on streamflow variability: hydrologic model and statistical methods. Hydrological models, such as SWAT model (Zhang et al., 2012), Xinanjiang model (Jiang et al., 2007), and GBHM model (Xu et al., 2013), are very useful to investigate streamflow evolution, and the models require numbers of high-quality recorded datasets as the input in order to ensure accuracy. Simultaneously, the modeling results have some uncertainties caused by the shortcomings from model structure, parameters, and scale problem. By contrast, statistical methods, including the cumulative anomaly curve method (Ran et al., 2010) and time trend method (Wei and Zhang, 2010), are comparatively simple, but lack a clear physical mechanism and so provide generalized relationships. In addition, catchment experiments also are powerful tools for assessing the impact of vegetation on the water balance (Costa et al., 2003); nevertheless, they are applied to small catchments due to the limiting conditions.
Numerous methods have been proposed to study the hydrological cycle process, and among them, hydrological modeling method has been widely used in different regions of the world. However, as these process-based models are complex combinations of scientific hypotheses, their results are highly dependent on the embedded hypotheses and often vary greatly among models (Beer et al., 2010; Yang et al., 2015). Additionally, they also require major efforts on model calibration and have uncertainties in the structure and parameters of the model (Wang and Yu, 2015). The advantage of using the Budyko framework lies in its ability to analytically separate the impacts of mean climate conditions and catchment properties on hydrological partitioning (Roderick & Farquhar, 2011; Wang & Hejazi, 2011; Liu and McVicar, 2012; Xu et al., 2014; Liang et al., 2015).
The Budyko framework demonstrates the long-term physical distribution between precipitation, evaporation, and streamflow. Due to the efforts and contributions of subsequent researchers, the original Budyko equation (Budyko, 1974) has developed into many forms, such as Fu (1981), Mezentsev–Choudhury–Yang (Mezentsev, 1955; Choudhury, 1999; Zhang et al., 2001; Yang et al., 2008; Wang and Tang, 2014), which all have one parameter (ω) related to the characteristics of watershed (e.g., soils, topography, vegetation, and land use types). Among the above equations, Fu equation is considered to be the most widely concerned and applied equation in the world. The parameter (ω) can be calculated according to the method in literature (Lv et al., 2019). Additionally, based on the Budyko framework, the climate sensitivity method initially proposed by Schaake (1990), which is very similar to other sensitivity (Milly and Dunne, 2002), has been also adopted to estimate the effects of climate change with continuous extension and improvement (Fu et al., 2007). Researchers believe that the sensitivity method is more credible than other statistical methods because of including basic physical processes. Over the last studies, the climate elasticity approach has been widely used to investigate climate- and human-induced streamflow variability and furthermore analyze the major driving factor of change (Ma et al., 2010).
The Huangshui River basin is the important water-producing area of the upper catchment of the Yellow River, and streamflow in this region has showed a reducing trend recently (Huo et al., 2008). However, quantitatively assessing the sensitivities and relative contributions of streamflow to different factors (climatic and no-climatic factors) for the whole basin have not been reported. The major purposes of this study are to 1) explore the temporal trends and change point of annual streamflow; 2) obtain the sensitivity coefficients of streamflow to precipitation, potential evaporation, and watershed characteristic; and 3) quantitatively separate the impacts of climate change and watershed characteristic change to streamflow changes in the Huangshui River basin.
Study Area
The Huangshui River is the largest first-level tributary on the left bank of the upper Yellow River (Figure 1), which primarily includes the main stream and the tributary Datong River. Its main river length is 374 km, and the drainage area of the catchments is 32863 km2 (36°02′–38°20′N, 98°54′–103°24′E). The Huangshui River basin is located in the transitional zone between the Qinghai-Tibetan Plateau and the Loess Plateau. The whole basin is shaped like leaves, narrow in the east and wide in the west. The topography of the basin is high in the northwest and low in the southeast. The basin is at an altitude of 1500–5200 m and belongs to a continental climate; alpine and drought are the climatic characteristics of this area, and the basin is one of the regions which is frequently influenced by East Asian Monsoon, South Asian Monsoon, and Plateau Monsoon. The average mean temperature is 0.6–7.9°C, the average maximum temperature is 34.7°C, the average minimum temperature is −32.6°C, the average mean evaporation is 800–1500 mm, and the average mean precipitation is 300–500 mm. The precipitation shows obvious season variation, and more than 70% occurs between June and September due to the southeast monsoon from the Bay of Bengal. The annual runoff is about 44.5 billion m3, which mainly comes from the mixed supplies of snowmelt and precipitation. The soil and vegetation are significantly different in different regions of the basin due to the comprehensive influence of terrain, altitude, climate, and soil parent material. The main soil-forming parent materials are the tertiary red soil and the quaternary loess.
Research Methods
The Budyko Hypothesis
Budyko (1974) believed that on a long time, the actual evapotranspiration of the basin was determined by a balance between the land surface water supply conditions (precipitation) and atmospheric evaporation requirements (net radiation or potential evapotranspiration) and put forward the general form of a balanced relationship:
where E is the actual evapotranspiration (mm); P is the mean annual precipitation (mm); E0 is the mean annual potential evapotranspiration (mm).
Following the Budyko hypothesis, according to the physical meaning of watershed hydrometeorology, Baopu Fu (1981) analyzed and deduced the form of Budyko hypothesis curve and provided another form of analytical expression:
where ω is a non-dimensional parameter of the watershed underlying characteristic, which is related to the land use, soil, vegetation, topography, and other characteristics.
Therefore, taking the underlying surface factors of the watershed, Budyko hypothesis was developed into a new form of expression:
Sensitivity Analysis of Influencing Factors of Water Watershed Runoff
Based on the water balance equation (P = E + Q) for a long period, combined with Eq. 2, there is
Based on the definition of the sensitivity coefficient, combined with Eq. 4, the calculation formulas of sensitivity coefficients of Q to P, E0, ω can be obtained as follows:
where ω is calculated using the least squares method.
Estimating the Contribution of Climate and Watershed Characteristic Variabilities to Streamflow
Perturbations in precipitation, potential evaporation, and watershed characteristic will result in changes of water balances. Therefore, it can be suggested that the mean annual streamflow change was caused by climate and watershed characteristic variabilities, and this can be expressed as the following:
where ∆P, ∆E0, and ∆ω denote changes in streamflow, potential evaporation, and watershed characteristic, respectively;
Therefore, the contribution rates of climate and watershed characteristic variabilities can be determined in the following expression:
The Mann–Kendall Test
1) The Mann–Kendall trend test
The Mann–Kendall trend test, recommended by the World Meteorological Organization and widely used to detect varying trends in hydro-climatic time series (Wang and Yu, 2015), is adopted in this study. The test statistics is as follows:
where
where xi and xj denote two simple variables from a time series data (x1, x2, x3 … , xn); n is the data size; Z and S stand for a test statistic, respectively.
Under the significance level of α, the null hypothesis of no trend is rejected if |Z| > Z(1-α/2), where Z(1-α/2) denotes the critical value obtained from the standard normal distribution tables. A positive Z value indicates an upward trend and vice versa. In this study, α = 0.05.
2) The Mann–Kendall change-point analysis
The test statistic Sk is calculated as follows:
Then, the statistics index UFk is calculated as follows:
where
Another statistics index UBk is obtained using the same procedure shown above but with an adverse time series. The two lines of UFk and UBk may make an intersection point at a certain significance level, and then the point will be identified as the abrupt point.
Precipitation-Streamflow Double Cumulative Curve
Double cumulative curve (Huo et al., 2008) is the most intuitive and widely used method in time series analysis, and it is adopted to determine the time and degree when watershed characteristic begins to significantly influence streamflow in this study. When it is primarily affected by precipitation, the double cumulative curve is a straight line; when other factors such as the underlying surface of the watershed are significantly affected, the curve will shift and have a break point. The degree of curve deviation indicates the impact intensity of the underlying surface of the watershed. The precipitation-streamflow double cumulative curve can be defined by
where ∑Q, ∑P, ∑Qdcc, and ∆Qdcc denote cumulative streamflow, cumulative precipitation, simulated cumulative streamflow, and cumulative streamflow by watershed characteristic, respectively; a and b denote fixed parameters.
Data
This study utilized annual runoff data from China Hydrology Yearbook. The observed series cover the period from 1958 to 2017. Meteorological data from eight weather stations within and around the research area were provided by the National Climate Center of China Meteorological Administration (http: //www.data.cma.cn/) (Table 1). All the meteorological data series with daily temperature, relative humidity, wind speed, sunshine duration, and precipitation were also from 1958 to 2017, and the potential evapotranspiration was calculated by Penman–Monteith formula (Allen et al., 1998). The NDVI dataset used in this study is NASA Global Inventory Monitoring and Modeling Systems Normalized Difference Vegetation Index third dataset (GIMMS NDVI 3 g) and it is the longest NDVI time series so far, with a period of from 1982 to 2015, a spatial resolution of 1/12°, and a temporal interval of 15 days (Ni et al., 2020), and it is confirmed that the dataset can provide high-quality data for places from mid to high latitudes. Annual NDVI values are obtained by using maximum value composite method (Zhang et al., 2013).
Results
Characteristics of Streamflow Variability in the Huangshui River Basin
The Mann–Kendall trend test of annual streamflow for Huangshui River basin was summarized in Figure 2. The results showed that the statistical test value (Z) was −1.59, and its absolute value (|Z|) was less than 1.96, indicating that the annual streamflow of the Huangshui River basin exhibited an insignificant downward trend at the 0.05 significance level.
The Mann–Kendall mutation test was performed on the streamflow data, as is showed in Figure 2. It can be noted that, before 1987, although the UF and UB lines had several intersections, however, there was no obvious abrupt point. After 1987, lines of UF and UB crossed in 1993, and the intersection point was within the critical lines of
It was clearly seen that compared with the reference period, the mean annual streamflow in the change period decreased from 150.71 to 130.23 mm (Figure 3). It is concluded that the annual streamflow reduced by 20.48 mm (13.59%).
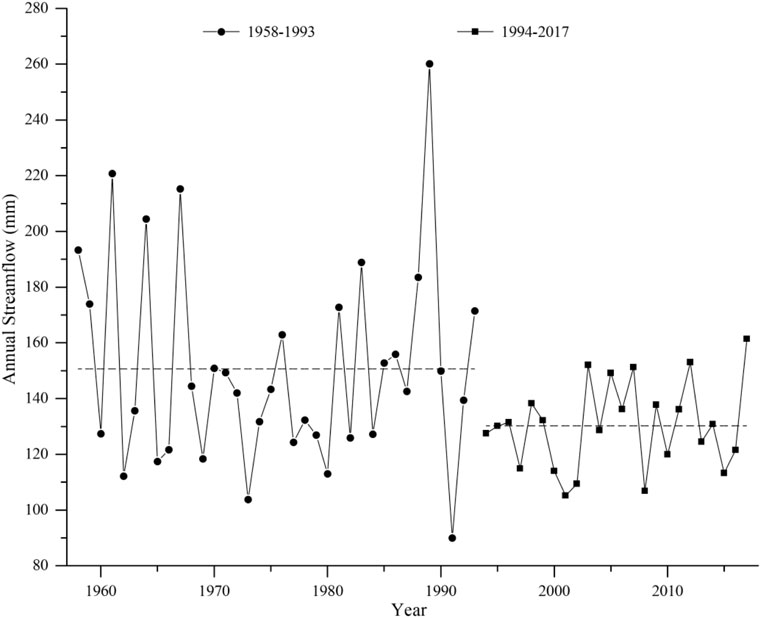
FIGURE 3. Annual streamflow comparison of reference period and change period in the Huangshui River basin.
Sensitivity of Watershed Streamflow to Climatic Factors and Watershed Characteristic
Based on the annual data series of 1958–2017 in the Huangshui River basin, values of the mean annual precipitation, the mean annual streamflow, and the mean potential evapotranspiration were 418.81, 142.52, and 833.28mm, respectively. Further, watershed characteristic parameter (ω) calculated on the basis of Eq. 4 was 1.7306.
These data obtained previously were used in Eq. 5, Eq. 6, and Eq. 7; the sensitivity coefficients of streamflow to precipitation, potential evapotranspiration, and watershed characteristic parameter were ∂Q/∂P = 0.5502, ∂Q/∂E0 = −0.1055, and ∂Q/∂ω = −183.2007, respectively. That means that every unit increase in precipitation, potential evapotranspiration, and watershed characteristic parameter will lead to the streamflow change by 0.5502, −0.1055, and −183.2007 units. Thus, it is suggested that the streamflow change is positively related to precipitation, and it is negatively related to potential evapotranspiration and watershed characteristic. Meanwhile, among climate factors, streamflow becomes more sensitive to change in precipitation than that in potential evapotranspiration. The results also suggest that watershed characteristic changes play a more important role than climate changes on streamflow variability in the Huangshui River basin.
Attribution Analysis of Streamflow Changes in the Huangshui River Basin
Based on the data provided from the Huangshui River basin in period of 1958–2017, the mean annual streamflow, the mean annual precipitation, and the mean annual potential evapotranspiration in the reference period (1958–1993) and the change period (1994–2017) were calculated, respectively. Further, watershed characteristic parameter (ω) can be also calculated on the basis of Eq. 4. The results are shown in Table 2. During two periods, P and ω increase from 411.65 to 429.56 mm and from 1.6412 to 1.8153, respectively, while E0 decreases from 836.46 to 828.51 mm, conversely.
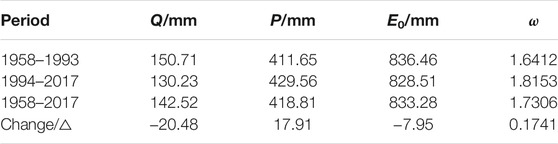
TABLE 2. The changes of streamflow and meteorological and watershed characteristic parameter in two periods.
The effects of climate changes and watershed characteristic changes on the streamflow amount were 10.69 mm and −31.90 mm, respectively, calculated by Eq. 8–12. The impacts of climate and watershed characteristic changes on streamflow are summarized in Table 3. The watershed characteristic changes should be principally responsible for streamflow change, which led to a large streamflow decrease of 31.90 mm and contributes 73.64% of the total streamflow change, while climate-induced streamflow change only accounted for 24.68%.

TABLE 3. Relative contribution rates of climate change and watershed characteristic changes to streamflow variability.
Double Cumulative Curve Verification
As shown in Figure 4, the slope of the curve changed abruptly in 1993, which is consistent with the Mann–Kendall test result and the division of the study period in this study. That means that underlying surface of the watershed has significant impact on the streamflow of the Huangshui River basin after 1993.
Figure 4 also shows that the correlation equation of cumulative streamflow and cumulative precipitation in the period of 1958–1993 was ∑Q = 0.3729, ∑P–14.669, the correlation coefficient R2 was 0.9977, the time series N was 60 years, and the confidence level was 0.001.
The cumulative simulated streamflow in the change period was obtained by using the correlation equation on the basis of cumulative precipitation in the same period. Thus, further, the values of simulated streamflow are calculated during the change period. During the changing period (1994–2017), the observed mean streamflow is 130.23 mm, while the simulated mean streamflow is 160.19 mm (Figure 5). Therefore, the underlying surface of watershed is estimated to have resulted in a 29.96 mm change of the basin.
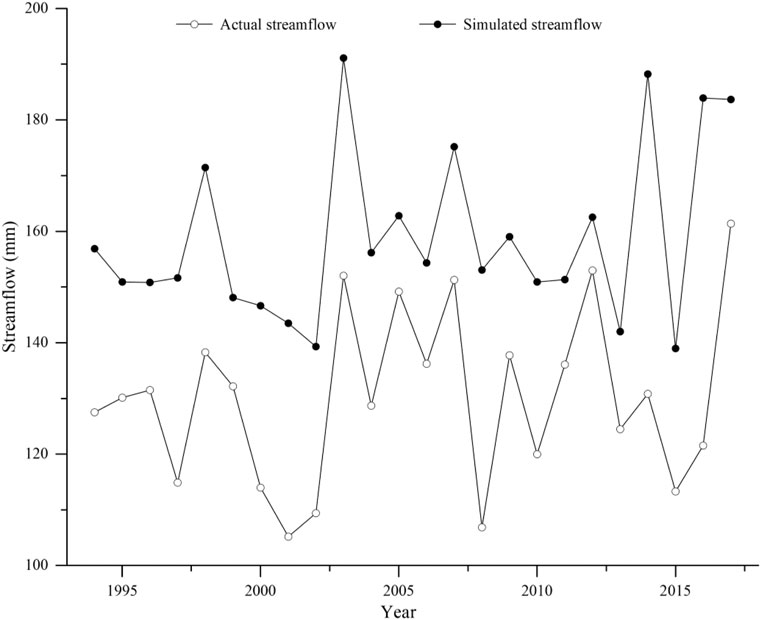
FIGURE 5. Double cumulative curve comparison of simulated values and observed values in the changed period.
Discussion
Attribution Analysis of Streamflow Variability
In spite of slowly upward trend in annual precipitation and slightly downward trend in annual potential evaporation, slightly decreasing trend in annual streamflow can be found for the Huangshui River basin. That infers, streamflow is affected by other factors (primary human activities) in addition to climate change. Huangshui River Basin plays an important role in politics, economy, culture and transportation in Qinghai Province, China. Due to the needs of water supply and development, many water retaining structures (such as reservoirs) have been built in this region, which reduces the streamflow of Huangshui River and its tributaries directly to different degrees and changes spatiotemporal distribution of streamflow. Researchers believe that reservoirs with large storage capacity could lead to the strengthening of evaporation and river channel leakage, which also reduces streamflow to some degree (Feng et al., 2020). In addition, owing to serious soil and water loss in the middle and lower reaches of the basin, soil and water conservations have gradually covered this area since the 1970s, including terraced fields, afforestation and grass-planting, and warping dam. Vegetation is the most important land use type in Huangshui basin, with a proportion of more than 85%, and the proportion of other land use types is small. As seen in Figure 6, the vegetation coverage of this region during 1982–2015 has improved dramatically, and annual mean NDVI has increased gradually at a rate of 0.0008/year (range from 0.6094 in 1982 to 0.6716 in 2015). Therefore, the increase in vegetation coverage could improve the soil structure and result in the enhancing of evaporation and infiltration and more water is trapped in the soil, leading to streamflow decline in the basin outlet through intercepting more precipitation (Xu, 2011; Qiu et al., 2021).
Climate change and human activities are generally considered as the two primary factors of streamflow variation, and the effects of them to streamflow are significantly different in different catchments. Studies have shown that the decrease in precipitation caused by climate change is the main reason for the decrease in the runoff of the Congo River in Africa (Noel and Saiers, 2017); however, for the Colorado River in the United States, the impact of human activities on runoff changes is slightly greater than climate change (Shi et al., 2019). In the mainstream area of the Yangtze basin, the increase in precipitation caused by climate change is the main reason for the streamflow variability in the upper reaches of the basin, and human activities are the main factor in runoff change for the lower reaches of the basin, mainly including the reduction of lake area and the hardening of the ground caused by urbanization (Wang et al., 2013). For the tributaries of the Haihe River, human activities are the main factors for declining streamflow in Luanhe River catchment, Chaohe River catchment, and Zhanghe River catchment, while climate change is the main driver leading to decreasing streamflow in the Huto River catchment, and increasing water demand should be responsible for streamflow variability in the whole basin (Wang et al., 2013). Streamflow of the Yellow River basin shows a significantly downward trend, which is primarily caused by human activities. As shown in Figure 7, compared with 1950s, in the early 21st century, the water consumption of the upper reaches and whole basin of the Yellow River basin has increased by approximately two and three times, respectively. Research indicates that agricultural irrigation accounted for more than 50% of the Yellow River runoff during the same period. In addition, in order to control serious soil and water loss, large-scale soil and water conservation projects have been constructed in the upper and middle reaches of the Yellow River since the 1970s, but it also reduces the runoff generation capacity of the basin.
In order to further understand streamflow variability of the upper Yellow River, this study compares attributions of streamflow in the headwaters of the Yellow River basin (HYRB) (Zheng et al., 2009), the Tao River basin (Sun et al., 2019), the Zuli River basin (Deng, 2001), and the Xiliugou basin (Yao et al., 2015) of the upper Yellow River. The contributions of runoff change in the above studies were quantitatively evaluated by using the double mass curves method, climate elasticity method, RCC-WBM model, and SWAT model, and the results are listed in Figure 8. Research in the HYRB indicates that the contribution rates of climate change and human activities to streamflow variability were 26.20 and 73.80%, respectively, which is extremely similar to the conclusions of this study (climate changes account for 24.68% and watershed characteristic changes account for 73.64%). These results suggest that the sensitivity analysis method based on Budyko theory is used to investigate the river basin streamflow evolution is feasible, and the results are accurate. Besides, these also indicate related human activities have tremendous influences on the slow decline in streamflow for the entire upper Yellow River.
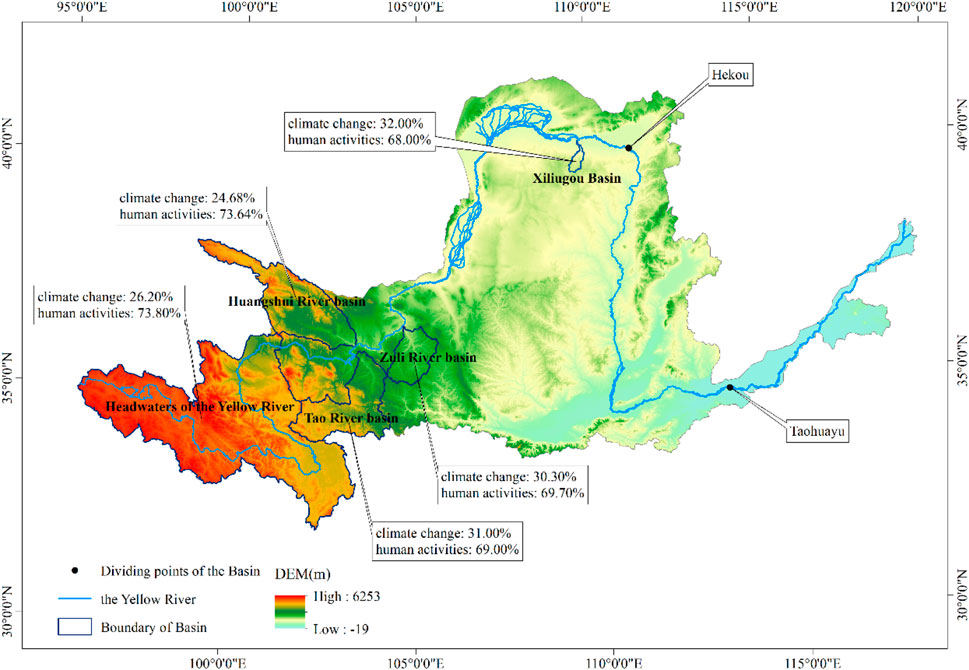
FIGURE 8. The map focusing on observed impacts of the hydrological response in the upper Yellow River.
Cause-Effect of Changing Point
In this study, the streamflow of Huangshui River basin showed a change point in 1993, which was very similar with other researches in the upper reaches of the Yellow River. After studying climate change in northwest China where the Huangshui River basin is located, some researchers believe that the abrupt changes of surface wind speed and mean annual temperature in the past 44 years (1960–2003) are in 1990 and 1994, and the region experiences a period of lower surface wind speed after 1990 and a warmer period after 1994, respectively (Wang et al., 2007), and researchers believe that obvious changes of extreme maximum and minimum temperatures in Hexi Corridor of northwest China are in 1996 and 1993, and the subsequent climate is all warmer (Li et al., 2015).
Uncertainties
It is essential that there are some uncertainties in the assessment of climate change and human activities on basin drainage. This study assumes that climate and watershed characteristic changes are independent of each other. Nevertheless, these two factors are interacting in an exceedingly complicated way, even in the reference period (Wang et al., 2019). In addition, we analyze streamflow variability and estimate climate and watershed-characteristic-induced to streamflow variability, but the values (Table 2 and 3) show only average values during the reference and change period and lack consideration of range or change of the values. Moreover, the change of present permafrost in an alpine basin significantly complicated the hydrological processes due to climate warming, and we do not consider the extent of its impact (Fang et al., 2019). In fact, watershed characteristic parameter (ω) includes both climate-induced and watershed-characteristic-induced variabilities, and attribution analysis in this study overestimates the effect of watershed characteristic on streamflow variability (Xu et al., 2014). The spatiotemporal distribution of meteorological stations is not considered in the Budyko framework, and it is possible to have another type of error in quantitative assessment/analysis (Shahid et al., 2018). In the future research, these uncertainties need be paid more attention.
Conclusion
Based on the Budyko framework, this study assessed relative contributions of climate and watershed characteristic changes on streamflow in the Huangshui River basin. The main conclusions are as follows:
1) From 1958 to 2017, the annual streamflow of Huangshui River basin showed an insignificant downward trend. The streamflow series had a change point in 1993, and thus the streamflow reference period was set to 1958–1993, and the change period was 1994–2017. In the change period, the streamflow decreased by 20.48 mm (13.59%) compared with the reference period.
2) Sensitivity coefficients of streamflow to precipitation, potential evapotranspiration, and watershed characteristic parameter in Huangshui River basin are 0.5502, −0.1055, and −183.2007, respectively. It shows that an increase in precipitation by 1 unit will induce an increase of 0.5502 units in streamflow, and an increase in potential evapotranspiration by 1 unit will induce a decrease of 0.1055 units in streamflow, and an increase in the watershed characteristic parameter by 1 unit will induce a decrease of 183.2007 units in streamflow.
3) During the change period (1994–2017), the streamflow change in the Huangshui River basin is mainly caused by the watershed underlying surface change, and its contribution rate is 77.64%, and the impact of climate changes on runoff is only 24.68%.
Data Availability Statement
The original contributions presented in the study are included in the article/Supplementary Material; further inquiries can be directed to the corresponding author.
Author Contributions
XL wrote the original draft of this manuscript. TQ and SSL designed the manuscript. SPL and YN undertook the analysis of data. QZ revised the manuscript.
Funding
This study was financially supported by the National Key Research and Development Project (No. 2017YFA0605004), State Key Laboratory Fund (IWHR-SKL-KF201903), National Natural Sciences Foundation of China (51979118), and Central Nonprofit Research Institutions Basic Scientific Research Special Fund (HKY-JBYW-2019-01). All experiments were in compliance with the current laws of the country in which they were performed.
Conflict of Interest
The authors declare that the research was conducted in the absence of any commercial or financial relationships that could be construed as a potential conflict of interest.
Publisher’s Note
All claims expressed in this article are solely those of the authors and do not necessarily represent those of their affiliated organizations, or those of the publisher, the editors, and the reviewers. Any product that may be evaluated in this article, or claim that may be made by its manufacturer, is not guaranteed or endorsed by the publisher.
Acknowledgments
The authors thank the data observers for their hard work.
References
Beer, C., Reichstein, M., Tomelleri, E., Ciais, P., Jung, M., Carvalhais, N., et al. (2010). Terrestrial Gross Carbon Dioxide Uptake: Global Distribution and Covariation with Climate. Science 329 (5993), 834–838. doi:10.1126/science.1184984
Choudhury, B. J. (1999). Evaluation of an Empirical Equation for Annual Evaporation Using Field Observations and Results from a Biophysical Model. J. Hydrol. 216 (1-2), 99–110. doi:10.1016/S0022-1694(98)00293-5
Costa, M. H., Botta, A., and Cardille, J. A. (2003). Effects of Large-Scale Changes in Land Cover on the Discharge of the Tocantins River, Southeastern Amazonia. J. Hydrol. 283 (1-4), 206–217. doi:10.1016/S0022-1694(03)00267-1
Deng, J. L. (2001). Trend and Mutation Analysis of Spatial and Temporal Distribution on Runoff and Sediment in Zulihe River Basin. J. China Hydrol. 21 (2), 47–50.
Fang, J., Li, G., Rubinato, M., Ma, G., Zhou, J., Jia, G., et al. (2019). Analysis of Long-Term Water Level Variations in Qinghai Lake in China. Water 11 (10), 2136–2156. doi:10.3390/w11102136
Feng, J., Zhao, G., Mu, X., Tian, P., and Tian, X. (2020). Analysis on Runoff Regime in Middle Yellow River and its Driving Factors. J. Hydroelectric Eng. 39 (8), 90–103.
Fu, B. P. (1981). On the Calculation of the Evaporation from Land Surface. Scientia atmosoherica sinica 5 (1), 25–33.
Fu, G., Charles, S. P., and Chiew, F. H. S. (2007). A Two-Parameter Climate Elasticity of Streamflow index to Assess Climate Change Effects on Annual Streamflow. Water Resour. Res. 43 (11), 2578–2584. doi:10.1029/2007WR005890
Hu, J., Wu, Y., Wang, L., Sun, P., Zhao, F., Jin, Z., et al. (2021). Impacts of Land-Use Conversions on the Water Cycle in a Typical Watershed in the Southern Chinese Loess Plateau. J. Hydrol. 593, 125741. doi:10.1016/j.jhydrol.2020.125741
Huo, Z., Feng, S., Kang, S., Li, W., and Chen, S. (2008). Effect of Climate Changes and Water‐related Human Activities on Annual Stream Flows of the Shiyang River basin in Arid north‐west China. Hydrol. Process. 22, 3155–3167. doi:10.1002/hyp.6900
Jiang, T., Chen, Y. D., Xu, C.-y., Chen, X., Chen, X., and Singh, V. P. (2007). Comparison of Hydrological Impacts of Climate Change Simulated by Six Hydrological Models in the Dongjiang Basin, South China. J. Hydrol. 336, 316–333. doi:10.1016/j.jhydrol.2007.01.010
Kong, D., Miao, C., Wu, J., and Duan, Q. (2016). Impact Assessment of Climate Change and Human Activities on Net Runoff in the Yellow River Basin from 1951 to 2012. Ecol. Eng. 91, 566–573. doi:10.1016/j.ecoleng.2016.02.023
Li, H., Gao, Z., Wang, S., and Wang, H. (2015). Extreme Temperature Variation of Hexi Corridor in Recent 60 Years. Arid Land Geogr. 38 (1), 1–9.
Li, X., and Tang, Q. (1981). An Analysis on Annual Runoff of West Sichuan and north Yunnan. Acta Geographica Sinica 36 (1), 90–100.
Li, Y., Mao, D., Feng, A., and Schillerberg, T. (2019). Will Human-Induced Vegetation Regreening Continually Decrease Runoff in the Loess Plateau of China. Forests 10 (10), 906–925. doi:10.3390/f10100906
Liang, W., Bai, D., Jin, Z., You, Y., Li, J., and Yang, Y. (2015). A Study on the Streamflow Change and its Relationship with Climate Change and Ecological Restoration Measures in a Sediment Concentrated Region in the Loess Plateau, China. Water Resour. Manage. 29 (11), 4045–4060. doi:10.1007/s11269-015-1044-5
Liu, Q., and Mcvicar, T. R. (2012). Assessing Climate Change Induced Modification of Penman Potential Evaporation and Runoff Sensitivity in a Large Water-Limited basin. J. Hydrol. 464-465, 352–362. doi:10.1016/j.jhydrol.2012.07.032
Liu, X. (2020). Quantitative Attribution of Runoff and Sediment Load Change in Huangshui River. Yellow River 42 (1), 6–10.
Lv, X., Zuo, Z., Ni, Y., Sun, J., and Wang, H. (2019). The Effects of Climate and Catchment Characteristic Change on Streamflow in a Typical Tributary of the Yellow River. Sci. Rep. 9, 1–10. doi:10.1038/s41598-019-51115-x
Ma, H., Yang, D., Tan, S. K., Gao, B., and Hu, Q. (2010). Impact of Climate Variability and Human Activity on Streamflow Decrease in the Miyun Reservoir Catchment. J. Hydrol. 389 (3-4), 317–324. doi:10.1016/j.jhydrol.2010.06.010
Mezentsev, V. S. (1955). More on the Calculation of Average Total Evaporation. Meteorol. Gidrol 5, 24–26.
Milly, P. C. D., and Dunne, K. A. (2002). Macroscale Water Fluxes 2. Water and Energy Supply Control of Their Interannual Variability. Water Resour. Res. 38 (10), 24-1–24-9. doi:10.1029/2001WR000760
Ni, Y., Zhou, Y., and Fan, J. (2020). Characterizing Spatiotemporal Pattern of Vegetation Greenness Breakpoints on Tibetan Plateau Using GIMMS NDVI3g Dataset. IEEE Access 8, 56518–56527. doi:10.1109/ACCESS.2020.2982661
Noel, A., and Saiers, J. (2017). Simulated Hydrologic Response to Projected Changes in Precipitation and Temperature in the Congo River basin. Hydrol. Earth Syst. Sci. 21, 4115–4130. doi:10.5194/hess-21-4115-2017
Qiu, L., Wu, Y., Shi, Z., Yu, M., Zhao, F., and Guan, Y. (2021). Quantifying Spatiotemporal Variations in Soil Moisture Driven by Vegetation Restoration on the Loess Plateau of China. J. Hydrol. 600. doi:10.1016/j.jhydrol.2021.126580
Ran, L., Wang, S., and Fan, X. (2010). Channel Change at Toudaoguai Station and its Responses to the Operation of Upstream Reservoirs in the Upper Yellow River. J. Geogr. Sci. 20 (2), 231–247. doi:10.1007/s11442-010-0231-9
Roderick, M. L., and Farquhar, G. D. (2011). A Simple Framework for Relating Variations in Runoff to Variations in Climatic Conditions and Catchment Properties. Water Resour. Res. 47 (12), 667–671. doi:10.1029/2010WR009826
Shahid, M., Cong, Z., and Zhang, D. (2018). Understanding the Impacts of Climate Change and Human Activities on Streamflow: a Case Study of the Soan River basin, Pakistan. Theor. Appl. Climatology 134 (1-2), 205–219. doi:10.1007/s00704-017-2269-4
Shi, X., Qin, T., Nie, H., Weng, B., and He, S. (2019). Changes in Major Global River Discharges Directed into the Ocean. Ijerph 16, 1469. doi:10.3390/ijerph16081469
Sun, L., Wang, Y.-Y., Zhang, J.-Y., Yang, Q.-L., Bao, Z.-X., Guan, X.-X., et al. (2019). Impact of Environmental Change on Runoff in a Transitional basin: Tao River Basin from the Tibetan Plateau to the Loess Plateau, China. Adv. Clim. Change Res. 10 (4), 214–224. doi:10.1016/j.accre.2020.02.002
Sun, P., Wu, Y., Wei, X., Sivakumar, B., Qiu, L., Mu, X., et al. (2020). Quantifying the Contributions of Climate Variation, Land Use Change, and Engineering Measures for Dramatic Reduction in Streamflow and Sediment in a Typical Loess Watershed, China. Ecol. Eng. 142. doi:10.1016/j.ecoleng.2019.105611
Tang, Y. (1995). On Human Action Influence on the Runoff in Luanhe River basin. J. Shaanxi Normal Univ. (Natural Sci. Edition) 23 (2), 125126. doi:10.15983/j.cnki.jsnu.1995.02.035
Thompson, J. R. (2012). Modelling the Impacts of Climate Change on upland Catchments in Southwest Scotland Using MIKE SHE and the UKCP09 Probabilistic Projections. Hydrol. Res. 43 (4), 507–530. doi:10.2166/nh.2012.105
Velpuri, N. M., and Senay, G. B. (2013). Analysis of Long-Term Trends (1950-2009) in Precipitation, Runoff and Runoff Coefficient in Major Urban Watersheds in the United States. Environ. Res. Lett. 8 (2), 024020–024026. doi:10.1088/1748-9326/8/2/024020
Wang, D., and Hejazi, M. (2011). Quantifying the Relative Contribution of the Climate and Direct Human Impacts on Mean Annual Streamflow in the Contiguous United States. Water Resour. Res. 47 (10), 411. doi:10.1029/2010WR010283
Wang, D., and Tang, Y. (2014). A One-Parameter Budyko Model for Water Balance Captures Emergent Behavior in Darwinian Hydrologic Models. Geophys. Res. Lett. 41 (13), 4569–4577. doi:10.1002/2014GL060509
Wang, F., Duan, K., Fu, S., Gou, F., Liang, W., Yan, J., et al. (2019). Partitioning Climate and Human Contributions to Changes in Mean Annual Streamflow Based on the Budyko Complementary Relationship in the Loess Plateau, China. Sci. Total Environ. 665, 579–590. doi:10.1016/j.scitotenv.2019.01.386
Wang, H., Lv, X., and Zhang, M. (2021). Sensitivity and Attribution Analysis Based on the Budyko Hypothesis for Streamflow Change in the Baiyangdian Catchment, China. Ecol. Indicators 121, 107221. doi:10.1016/j.ecolind.2020.107221
Wang, H., and Yu, X. (2015). Sensitivity Analysis of Climate on Streamflow in north China. Theor. Appl. Climatol 119, 391–399. doi:10.1007/s00704-014-1289-6
Wang, H., and Yu, X. (2015). Sensitivity Analysis of Climate on Streamflow in north China. Theor. Appl. Climatol. 119 (1-2), 391–399. doi:10.1007/s00704-014-1289-6
Wang, P., Yang, J., Zhang, Q., He, J., Wang, D., and Lu, D. (2007). Climate Change Characteristic of Northeast China in Recent Half century. Adv. Earth Sci. 22 (6), 649–656.
Wang, S., Yan, M., Yan, Y., Shi, C., and He, L. (2012). Contributions of Climate Change and Human Activities to the Changes in Runoff Increment in Different Sections of the Yellow River. Quat. Int. 282, 66–77. doi:10.1016/j.quaint.2012.07.011
Wang, W., Shao, Q., Yang, T., Peng, S., Xing, W., Sun, F., et al. (2013). Quantitative Assessment of the Impact of Climate Variability and Human Activities on Runoff Changes: a Case Study in Four Catchments of the Haihe River basin, China. Hydrol. Process. 27 (8), 1158–1174. doi:10.1002/hyp.9299
Wang, Y., Ding, Y., Ye, B., Liu, F., Wang, J., and Wang, J. (2013). Contributions of Climate and Human Activities to Changes in Runoff of the Yellow and Yangtze Rivers from 1950 to 2008. Sci. China Earth Sci. 56, 1398–1412. doi:10.1007/s11430-012-4505-1
Wei, X., and Zhang, M. (2010). Quantifying Streamflow Change Caused by forest Disturbance at a Large Spatial Scale: A Single Watershed Study. Water Resour. Res. 46 (12), 439–445. doi:10.1029/2010WR009250
Xu, J. (1995). A Comparative Study of the Zonal Differences in River Runoff and Human Influence in China. Geographical Res. 14 (3), 33–42.
Xu, J. (2011). Variation in Annual Runoff of the Wudinghe River as Influenced by Climate Change and Human Activity. Quat. Int. 244 (2), 230–237. doi:10.1016/j.quaint.2010.09.014
Xu, X., Yang, D., Yang, H., and Lei, H. (2014). Attribution Analysis Based on the Budyko Hypothesis for Detecting the Dominant Cause of Runoff Decline in Haihe basin. J. Hydrol. 510, 530–540. doi:10.1016/j.jhydrol.2013.12.052
Xu, X., Yang, H., Yang, D., and Ma, H. (2013). Assessing the Impacts of Climate Variability and Human Activities on Annual Runoff in the Luan River basin, China. Hydrol. Res. 44 (5), 940–952. doi:10.2166/nh.2013.144
Yang, H., Yang, D., Lei, Z., and Sun, F. (2008). New Analytical Derivation of the Mean Annual Water-Energy Balance Equation. Water Resour. Res. 44 (3), 893–897. doi:10.1029/2007WR006135
Yang, Y., Donohue, R. J., McVicar, T. R., and Roderick, M. L. (2015). An Analytical Model for Relating Global Terrestrial Carbon Assimilation with Climate and Surface Conditions Using a Rate Limitation Framework. Geophys. Res. Lett. 42, 9825–9835. doi:10.1002/2015GL066835
Yao, H., Shi, C., Shao, W., Bai, J., and Yang, H. (2015). Impacts of Climate Change and Human Activities on Runoff and Sediment Load of the Xiliugou basin in the Upper Yellow River. Adv. Meteorology 2015, 1–12. doi:10.1155/2015/481713
You, P. (1995). Surface Water Resources and Runoff Composition in the Tarim River Basin. Arid Land Geogr. 18 (2), 29–35. doi:10.13826/j.cnki.cn65-1103/x.1995.02.005
Zhan, C., Niu, C., Song, X., and Xu, C. (2013). The Impacts of Climate Variability and Human Activities on Streamflow in Bai River basin, Northern China. Hydrol. Res. 44 (5), 875–885. doi:10.2166/nh.2012.146
Zhang, B., Wu, P., Zhao, X., Wang, Y., and Gao, X. (2013). Changes in Vegetation Condition in Areas with Different Gradients (1980-2010) on the Loess Plateau, China. Environ. Earth Sci. 68 (8), 2427–2438. doi:10.1007/s12665-012-1927-1
Zhang, L., Dawes, W. R., and Walker, G. R. (2001). Response of Mean Annual Evapotranspiration to Vegetation Changes at Catchment Scale. Water Resour. Res. 37 (3), 701–708. doi:10.1029/2000WR900325
Zhang, M., Wei, X., Sun, P., and Liu, S. (2012). The Effect of forest Harvesting and Climatic Variability on Runoff in a Large Watershed: The Case Study in the Upper Minjiang River of Yangtze River basin. J. Hydrol. 464-465, 1–11. doi:10.1016/j.jhydrol.2012.05.050
Zhang, S., Yang, D., Yang, H., and Lei, H. (2015). Analysis of the Dominant Causes for Runoff Reduction in Five Major Basins over China during 1960-2010. Adv. Water Sci. 26 (5), 605–613. doi:10.14042/j.cnki.32.1309.2015.05.001
Zhao, F., Zhang, L., Xu, Z., and Scott, D. F. (2010). Evaluation of Methods for Estimating the Effects of Vegetation Change and Climate Variability on Streamflow. Water Resour. Res. 46 (3), 742–750. doi:10.1029/2009WR007702
Zhao, Y., Yang, N., Wei, Y., Hu, B., Cao, Q., Tong, K., et al. (2019). Eight Hundred Years of Drought and Flood Disasters and Precipitation Sequence Reconstruction in Wuzhou City, Southwest China. Water 11 (2), 219. doi:10.3390/w11020219
Keywords: streamflow, Budyko hypothesis, climate changes, underlying surface, Huangshui River basin, climate changes
Citation: Lv X, Liu S, Li S, Ni Y, Qin T and Zhang Q (2021) Quantitative Estimation on Contribution of Climate Changes and Watershed Characteristic Changes to Decreasing Streamflow in a Typical Basin of Yellow River. Front. Earth Sci. 9:752425. doi: 10.3389/feart.2021.752425
Received: 03 August 2021; Accepted: 14 September 2021;
Published: 20 October 2021.
Edited by:
Yulei Xie, Guangdong University of Technology, ChinaReviewed by:
Yiping Wu, Xi’an Jiaotong University, ChinaHenian Wang, Chinese Academy of Forestry, China
Zhe Yuan, Changjiang River Scientific Research Institute (CRSRI), China
Copyright © 2021 Lv, Liu, Li, Ni, Qin and Zhang. This is an open-access article distributed under the terms of the Creative Commons Attribution License (CC BY). The use, distribution or reproduction in other forums is permitted, provided the original author(s) and the copyright owner(s) are credited and that the original publication in this journal is cited, in accordance with accepted academic practice. No use, distribution or reproduction is permitted which does not comply with these terms.
*Correspondence: Tianling Qin, cWludGxAaXdoci5jb20=