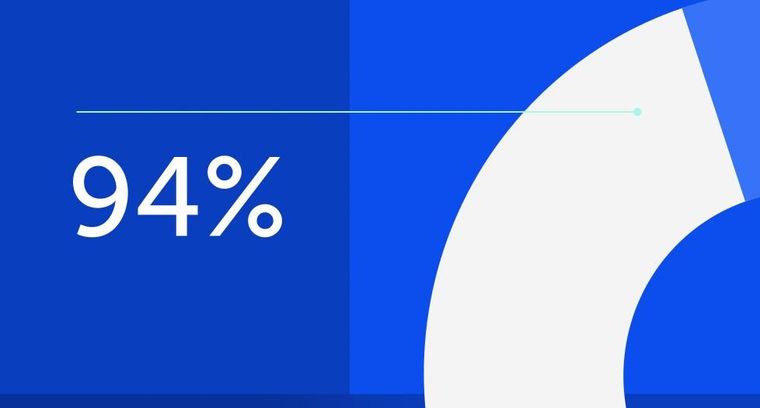
94% of researchers rate our articles as excellent or good
Learn more about the work of our research integrity team to safeguard the quality of each article we publish.
Find out more
ORIGINAL RESEARCH article
Front. Earth Sci., 12 October 2021
Sec. Interdisciplinary Climate Studies
Volume 9 - 2021 | https://doi.org/10.3389/feart.2021.747429
This article is part of the Research TopicFuture Climate Scenarios: Regional Climate Modelling and Data AnalysisView all 23 articles
The South-to-North Water Diversion project (SNWD project) is a mega water project designed to help solve water shortages in North China. The project’s management and operation are highly influenced by runoff change induced by climate change in the water source areas. It is important to understand water availability from the source areas in the context of global warming to optimize the project’s regulation. Based on the projections of nine GCMs, the future runoff in the water source areas of the three diversion routes was simulated by using a grid-based model RCCC-WBM (Water Balance Model developed by Research Center for Climate Change). Results show that temperature will rise by about 1.5°C in the near future (2035, defined as 2026–2045) and 2.0°C in the far future (2050, defined as 2041–2060) relative to the baseline period of 1956–2000. Although GCM projections of precipitation are highly uncertain, the projected precipitation will likely increase for all three water source areas. As a result of climate change, the simulated runoff in the water source areas of the SNWD project will likely increase slightly by less than 3% relative to the baseline period for the near and far future. However, due to the large dispersion and uncertainty of GCM projections, a high degree of attention should be paid to the climate-induced risk of water supply under extreme situations, particularly for the middle route of the SNWD project.
Water is the most direct and vulnerable sector influenced by climate change (Zhang and Wang, 2007; IPCC, 2008, 2013, 2021). China faces shortages in water sources due to a huge population (Liu et al., 2019). Uneven spatiotemporal distribution of water resources further exacerbates water scarcity in many arid regions (Hoekstra, 2014; Cosgrove and Loucks, 2015; Montanari et al., 2015). How much water is available in the context of global warming has been attracting tremendous attention from various arms of the central government, local communities, and river basin managers (Kundzewicz et al., 2018; Lu et al., 2019; Luo et al., 2019).
Studies show that only 10% of the total renewable water resources are currently used by people, and nearly 80% of the world’s population is exposed to high levels of threat to water security (Oki and Kanae, 2006; Vörösmarty et al., 2010). Both climate change and human activities add complexity to the formation, migration, and transformation mechanisms of water resources by altering hydrological cycles, thereby aggravating water scarcity and water conflicts among different socioeconomic sectors (Haddeland et al., 2014; Liu et al., 2017; Tang et al., 2019). Because of the critical importance of water to socioeconomic development, climate change and its impacts on water resources have been investigated in previous studies (Wang et al., 2017; Liu et al., 2018; Bao et al., 2019; Sun et al., 2019). Regional and global hydrologic models combined with global climate model projections have been widely used to assess changes in water resources induced by climate change (Sivakumar, 2011; Schewe et al., 2013; Wang et al., 2012, 2017). The Xin’anjiang model which is based on the saturation excess mechanism has been mostly applied to humid catchments (Yuan et al., 2016; Zhang et al., 2019), while infiltration excess-based watershed models (e.g., GR4J model, SIMHYD model, etc.) have been used for assessing climate change impacts in arid catchments (Jones et al., 2006; Trudel et al., 2017). Land surface models (e.g., VIC model, CAS-LSM model, etc.) are mainly applied to large scale regions or applied at continental scale for hydrological modeling and climate change study (Wang et al., 2012; Wang et al., 2020). Due to the lack of observations, hydrological models with physical interpretation and simple model structure have attracted more interest and been applied in climate change study (Wang et al., 2014; Shahid et al., 2017). Compared with some of the well-known hydrological models (e.g., Xin’anjiang model, Tank model, etc.), simple models (e.g., RCCC-WBM) have advantages of easier understanding, fewer model parameters, more feasible transferability to the poorly gauged areas, etc. (Guan et al., 2019). The projected climate change impacts showed that water cycles have undergone considerable changes in the context of global warming, and such changes have altered water resource distribution in time and space (Bierkens, 2015; Mehran et al., 2017). Available water resources in the eastern monsoon region of China are decreasing and extreme hydrological events are occurring more frequently (Duan and Phillips, 2010; Xia et al., 2017), which increases the vulnerability of water resources and adds extra pressure on the security of water supplies, particularly in arid and semi-arid areas (Wang and Zhang, 2015; Jin et al., 2020).
China suffers from water shortages due to its large population and extremely low per capita water volume, accounting for less than one-third of the world average (CREEI, 2014; Liu et al., 2019). Conditions are particularly severe in the country’s northern regions, where half of the population and two-thirds of the nation’s farmland are located, but where there is only one-fifth of its water resources (Liu and Zheng, 2002; Liu and Xia, 2004). To alleviate water scarcity and maintain socioeconomic development in northern China, the central government has embarked on a strategic and ambitious infrastructure project known as the South-to-North Water Diversion project (SNWD project; Zhang, 2009; Zhao et al., 2017). The project is designed to transfer 44.8 km3 of water per year from the water-abundant Yangtze River to the Huang-Huai-Hai region via its eastern, middle, and western routes, at a total cost of about US$62 billion (Stone and Jia, 2006; Liu et al., 2012; Yan and Chen, 2013; Long et al., 2020). By the end of 2018, the eastern route had brought an accumulated 3.1 billion m3 of water to Shandong and the middle route had brought an accumulated 17.8 billion m3 of water (http://nsbd.mwr.gov.cn; Yin et al., 2020). It has been observed that streamflow into the Danjiangkou Reservoir, the headwater source in the middle route of the SNWD project, has continuously decreased since the 1980s (Liu et al., 2012; Sun et al., 2014; She et al., 2017), negatively affecting the water supply of the middle route of the SNWD project. Using a climate elasticity method, Liu et al. (2012) concluded that the climatic variation (indicated by precipitation and potential evapotranspiration) was responsible for 84.1–90.1% of the stream decline. She et al. (2017) also showed that the sharp decrease in annual runoff from the Danjiangkou Reservoir is mainly influenced by the decrease in annual precipitation. While climate change affects the water availability of the water source area, it also affects the encounter probability of flood and drought between the water source areas and the water receiving areas (Chen and Xie, 2012; Liu et al., 2015; Xia et al., 2017).
Climate change will be one of the major challenges to the management and operation of the SNWD project, as water resources are sensitive to climate change and variability (Wang et al., 2012, 2017). With the expectation that water supplies will only become tighter in the future (Rodell et al., 2018; Pokhrel et al., 2021), it is essential to understand water availability in water source areas under climate change for the efficient and reasonable allocation of water resources by the SNWD project. However, previous studies on the SNWD project mainly focused on the historical variation of stream flow, so there are limited studies on future water availability of water source areas of the SNWD project, particularly for all three source areas together (Su et al., 2016; Yu et al., 2017). The objective of this study is to investigate future climate changes in the three water source areas and the extent to which the stream flow will change in the coming decades relative to the design period (1956–2000) of the SNWD project and finally to support the project operation practices and revisions of the second phase plan.
The SNWD project approved by China’s State Council in 2002 is a national strategic project that transfers water from the Yangtze River to the Huai River, Yellow River, and Hai River to solve water shortages in North China. The project was designed with three water diversion routes among which the eastern route and the middle route have been constructed and in use since 2013 and 2014, respectively, while the western route is still in the planning stages. Based on the project planning, the water source areas of the project consist of the upper Yangtze River for the western route with a drainage area of 299,087 km2, the middle and upper Han River for the mid-route with a drainage area of 94,784 km2, and the area (1,705,383 km2) above Datong hydrometric station for the eastern route, which covers almost the entire Yangtze River basin. The water source areas of the project, major river systems of the Yangtze River, and locations of key hydrometric stations controlling water source areas are shown in Figure 1.
FIGURE 1. Water source areas, river systems, and locations of key hydrometric stations of the South-to-North Water Diversion Project in the Yangtze River basin.
The daily grid meteorological data over the Yangtze River basin with a spatial resolution of 0.25° and 1951–2020 data series were collected from the China Meteorological Administration (CMA). The daily observed discharge data at five hydrometric stations which control drainage source areas of the SNWD project, shown in Figure 1, were collected from the Hydrology Bureau of the Ministry of Water Resources (MWR). These hydro-meteorological data were used to calibrate hydrological models for climate change impact assessment.
The SNWD project was designed by using the 1956–2000 data series. In order to understand the future climate changes relative to those in the design period, we defined two future periods as follows: near future (NF) from 2026 to 2045, and far future (FF) from 2041 to 2060. The future climate scenarios were downloaded from httpps://www.wcrp-climate.org/wgcm-cmip/wgcm-cmip6. As both the high-emission scenarios, e.g., SSP5-8.5, and the low-emission scenarios, e.g., SSP1-2.6, consider extreme emission pathways, the medium-emission scenarios, e.g., SSP2-4.5, will probably occur in the future. We therefore only used climate change projections under the SSP2-4.5 scenario in this study. Based on simulation performance to the past variation of climate variables and consideration of GCM independence (Xin et al., 2020; Zhao et al., 2021), nine GCMs were selected and used in this study (Table 1). The nine GCM projections under the SSP2-4.5 scenario were downscaled to a 0.25° grid by using a LARS-WG statistical downscaling method (Hassan et al., 2014). The data series of the projected climate scenarios are from 1901 to 2099.
In this study, the RCCC-WBM (Water Balance Model developed by the Research Center for Climate Change) was applied to the study areas for climate change impact assessment. The model is a conceptual hydrological model that considers the three runoff components of surface flow, underground flow, and snowmelt flow. The model inputs include monthly precipitation, pan evaporation, and temperature. The model has been applied to hundreds of catchments worldwide (Wang et al., 2014; Guan et al., 2019). The model structure is shown in Figure 2.
Based on the RCCC-WBM, we developed a grid-based model covering the entire Yangtze River basin, which was divided into 1,812 grid cells with a spatial resolution of 0.25°. The RCCC-WBM is employed to calculate runoff yield in each grid cell. For a catchment that covers numerous grid cells, the flow routing scheme in the VIC (Variable Infiltration Capacity) model was referenced in the model flow concentrating from grid cells to catchment outlet (Wang et al., 2012, 2014).
The RCCC-WBM has four parameters that need to be calibrated by comparing the simulated and recorded discharge series. The Nash and Sutcliffe efficiency criterion (NSE) and the relative error of volumetric fit (RE), which describe the fitting performance of the simulated discharge to the recorded discharge, are employed as the objective functions to calibrate the model (Nash and Sutcliffe, 1970; Moriasi et al., 2007; Gupta et al., 2009).
Taking 1956–2000 as a baseline period, changes in temperature in the near and far future relative to the baseline period for all three source areas, i.e., the western route source area (WRSA), middle route source area (MRSA), and eastern route source area (ERSA), of the SNWD project were investigated (Figure 3).
FIGURE 3. Temperature changes in two periods of near future (NF) and far future (FF) relative to baseline of 1956–2000 for all three source areas of the South-to-North Water Diversion Project (WRSA, MRSA, and ERSA denote the western route source area, the middle route source area, and the eastern route source area, respectively; same as Figure 4 and Figure 7).
Figure 3 shows that the nine GCMs all projected that temperatures will continue to rise in the near future and far future although they projected different rise ranges. In the near future of 2026–2045, temperature will rise by 1.64°C [1.27°C, 2.58°C], 1.33°C [0.87°C, 1.71°C], and 1.37°C [1.06°C, 1.89°C] for WRSA, MRSA, and ERSA, respectively. However, temperature will rise higher in the far future of 2041–2060. On average, temperature would rise by 2.09°C, 1.78°C, and 1.82°C, respectively, with ranges of [1.64°C, 3.09°C], [1.02°C, 2.44°C], and [1.19°C, 2.41°C] for the three water source areas.
Temperature is a thermal driver of the hydrological cycle, and temperature rise could reduce runoff yield by increasing catchment evaporation. According to IPCC, there is high confidence that global mean evaporation increases with global warming, with evaporation increasing by 1–3% for every 1°C increase in temperature (IPCC, 2021). Previous studies indicate that a 1°C rise in temperature might lead to an approximately 5% decrease in runoff for humid areas (IPCC, 2008; Wang et al., 2016). Changes in temperature will definitely influence water availability in the water source area of the SNWD project.
Figure 4 shows changes in precipitation during the coming periods of the near future and far future relative to the baseline period. The figure indicates that precipitation projections have a higher uncertainty than that of temperature as a GCM might project decrease in precipitation while another one might project precipitation increase. For the WRSA, all GCMs project that precipitation in near future will increase by 4.9% with a range of [1.43%, 14.1%], and most of the GCM projections show a 6.06% precipitation increase in the far future on average with a range of [−1.68%, 18.77%]. For the MRSA, more than half of the GCMs projected that precipitation will increase by 0.45% [−3.23%, 8.61%] in the near future and 2.54% [−1.77%, 7.5%] in the far future. For the ERSA, most of the GCMs projected that precipitation will increase by 1.63% [−3.62%, 4.84%] in the near future and 3.71% [−3.13%, 7.45%] in the far future.
FIGURE 4. Precipitation changes in two periods of near future (NF) and far future (FF) relative to baseline of 1956–2000 for all three source areas of the South-to-North Water Diversion Project.
According to the definition of uncertainty by the IPCC (IPCC, 2013), precipitation in the WRSA will almost certainly increase in the near future and will very likely increase in the far future, while precipitation in both the MRSA and ERSA is likely to increase in both the near and far future. Increases in precipitation for the source areas could increase runoff yield and will no doubt benefit implementation of the SNWD project.
A suitable hydrological model is essential to quantify the impact of climate change on water resources. Within the source areas of the SNWD project, there are daily discharges available at five hydrometric stations with a data series length of over 30 years. The grid meteorological data were used to drive the grid-based model RCCC-WBM for discharge simulation. Simulation results are given in Table 2. The monthly recorded and simulated discharges at the Yajiang hydrometric station were compared, as shown in Figure 5.
TABLE 2. Discharge simulation results for the five hydrometric stations within source areas of the South-to-North Water Diversion Project.
Table 2 shows that the grid-based model RCCC-WBM performs well in the discharge simulation for all five catchments. The NSEs in both calibration and validation periods are above 0.7, while the REs in the periods are limited in the range of ±2.0%. Figure 5 indicates that the monthly recorded and simulated runoff series at the Yajiang station for 1956–2000 matched well, which is in accordance with the results in Table 2. Table 2 and Figure 5 both sufficiently illustrate that the RCCC-WBM is qualified for simulating runoff under the future climate change scenarios.
By using the downscaled grid climate scenarios of nine GCMs to drive the grid-based model RCCC-WBM, monthly runoff yield series for grid cells were simulated for 1951–2090. The catchment average annual runoff yields of the three source areas of the SNWD project over the period were then calculated based on the areal weighted method. The 9-GCM-based annual runoff simulations for the three source areas and the simulation-based median runoff series over the period of 1951–2090 are shown in Figure 6. Figure 6 shows that the nine simulated annual runoff series all exhibited a natural fluctuation with no significant variation trends. However, the range of runoff variability in the coming decades becomes larger than that in the past.
FIGURE 6. The simulated annual runoff series (gray lines) of the nine GCMs and the simulation-based median runoff series (red line) during 1951–2090 for all three source areas of the South-to-North Water Diversion project ((A–C) denote source areas of the western route, the middle route, and the eastern route, respectively).
Runoff changes in the near future and far future relative to the baseline period of 1956–2000 were investigated based on the simulated runoff over the period of 1951–2090 under the nine GCM scenarios for all three source areas (Figure 7).
FIGURE 7. Runoff changes in two periods of near future (NF) and far future (FF) relative to the baseline period of 1956–2000 for all three source areas of the South-to-North Water Diversion Project.
Figure 7 indicates that for the WRSA, all GCMs project that runoff in the near future will increase by 1.42% with a range of [0.29%, 7.69%], and most of the GCMs project a 1.36% runoff increase in the far future on average with a range of [−5.84%, 11.40%]. The projected runoff in the WRSA will very likely increase in both the near and far future. For the MRSA, over 50% of the GCMs project that runoff will increase by 2.25% [−10.48%, 8.31%] in the near future and 2.35% [−10.27%, 4.60%] in the far future. Although more than half of the GCMs project runoff in the middle route source area will increase in the future, we also find that the projected runoff might decrease by > 10% in extreme conditions. Attention to the risk of runoff reduction induced by climate change should be given in the practical operation of the middle route sub-project. For the ERSA, most of the nine GCMs project runoff will increase in the near future with the exception of the GISS-E2-R which projects runoff will decrease by −7.3%. The GISS-E2-R and GFDL-ESM2G project annual runoff will decrease by −7.8% and −7.0% in far future while the other seven GCMs project annual runoff will increase by [0.1%, 4.2%]. On average, the median GCM project runoff will increase by 0.88% in the near future and 0.7% in the far future. In general, the projected runoff in the eastern route source area will likely increase in the coming decades, which could support operation of the eastern route sub-project.
Both changes in temperature and precipitation could affect regional water resources by altering hydrological cycles. Global land surface temperature rose by 0.85°C during the period 1880–2012 (IPCC, 2013) while temperatures in China rose by 0.9°C in the same period. Temperatures in China have risen particularly fast during recent decades (1956–2012), increasing at a rate of 0.25°C/10a, which is higher than the global average (Qin et al., 2012). The variation of the projected temperature over the three source areas in this study are in accordance with the previous studies, which will continue to rise in the future (Tao et al., 2011). However, the projected increase in temperature in this study is approximately 0.33°C/10a, which is much higher than that in the past (Huang et al., 2014). The projected regional average precipitation over the three source areas will likely increase in the rapid warming situation although several GCMs project a certain decrease in precipitation. Most previous studies support the findings although there is great uncertainty in precipitation projections (Zhang et al., 2010; Guo et al., 2012).
Numerous studies have indicated that the precipitation in the Yangtze River basin will increase in the coming decades, and, as a result, stream flow will probably increase (Bian et al., 2017; Yu et al., 2017; Lu et al., 2018), which is in accordance with the findings in this study. However, Gu et al. (2015) found that annual runoff at the Panzhihua station in the upper Yangtze River basin during 2011–2040 may decrease by 1.2–3.5% compared to that in 1970–1999, which is counter to the conclusions drawn in this study. The discrepancy might result from differences in the baseline period, future periods, GCMs, and the study catchments selected. Although inflow to the Danjiangkou Reservoir decreased in the past (Liu et al., 2012; Sun et al., 2014), the projected runoff will likely increase by 2% in the coming decades. The hydrological regime is shifting to benefit the operation of the SNWD project due to climate change.
In the context of global warming, temperatures in the source areas of the SNWD project will continue to rise. Relative to the baseline period (1956–2000), temperatures will rise by about 1.5°C and 2.0°C in the near future (2026–2045) and the far future (2041–2060). Precipitation will likely increase for all three source areas although GCM projections are quite dispersed and uncertain.
The grid-based model RCCC-WBM performs well for discharges in the study areas. The simulated runoff is associated with GCM projections. According to the nine GCMs, the median runoff will likely increase by less than 3% relative to the baseline for all three source areas of the SNWD project, which could guarantee the security of water supply to some extent. However, attention should be paid to the risk to water supply induced by extreme climate change conditions when the project operates in practice.
The raw data supporting the conclusions of this article will be made available by the authors, without undue reservation.
CQ: conceptualization and methodology. GW and JQ: data curation and model. ZN: formal analysis and visualization. YW and GW: writing and editing the manuscript. QL: discussion and suggestions for data analysis.
This research was financially supported by the National Key Research and Development Programs of China (Grants 2016YFA0601500, 2017YFA0605002, and 2017YFC0404602), the National Natural Science Foundation of China (Grant nos. 41830863, 51879162, 51609242, 51779146, and 41601025), and the State Key Laboratory of Hydrology-Water Resources and Hydraulic Engineering (Grant no. 2019nkzd02).
The authors declare that the research was conducted in the absence of any commercial or financial relationships that could be construed as a potential conflict of interest.
All claims expressed in this article are solely those of the authors and do not necessarily represent those of their affiliated organizations, or those of the publisher, the editors, and the reviewers. Any product that may be evaluated in this article, or claim that may be made by its manufacturer, is not guaranteed or endorsed by the publisher.
Bao, Z., Zhang, J., Wang, G., Chen, Q., Guan, T., Yan, X., et al. (2019). The Impact of Climate Variability and Land Use/Cover Change on the Water Balance in the Middle Yellow River Basin, China. J. Hydrol. 577, 123942. doi:10.1016/j.jhydrol.2019.123942
Bian, H., Lü, H., Sadeghi, A., Zhu, Y., Yu, Z., Ouyang, F., et al. (2017). Assessment on the Effect of Climate Change on Streamflow in the Source Region of the Yangtze River, China. Water. 9, 70. doi:10.3390/w9010070
Bierkens, M. F. P. (2015). Global Hydrology 2015: State, Trends, and Directions. Water Resour. Res. 51, 4923–4947. doi:10.1002/2015WR017173
Chen, F., and Xie, Z. (2012). Effects of Climate Change on Synchronous-Asynchronous Encounter Probability of Rich-Poor Precipitation Between Water Source Area and Water Receiving Areas in the Middle Route of South-To-North Water Transfer Project. Clim. Environ. Res. 17 (2), 139–148. doi:10.3878/j.isn.1006-9585.2011.10097
China Renewable Energy Engineering Institute (CREEI) (2014). Ministry of Water Resources. Water Resources Assessment and its Development & Utilization in China. Beijing: China Hydropower Press.
Cosgrove, W. J., and Loucks, D. P. (2015). Water Management: Current and Future Challenges and Research Directions. Water Resour. Res. 51, 4823–4839. doi:10.1002/2014WR016869
Duan, Q., and Phillips, T. J. (2010). Bayesian Estimation of Local Signal and Noise in Multimodel Simulations of Climate Change. J. Geophys. Res. 115, D18123. doi:10.1029/2009JD013654
Gu, H., Yu, Z., Wang, G., Wang, J., Ju, Q., Yang, C., et al. (2015). Impact of Climate Change on Hydrological Extremes in the Yangtze River Basin, China. Stoch. Environ. Res. Risk Assess. 29, 693–707. doi:10.1007/s00477-014-0957-5
Guan, X., Zhang, J., Elmahdi, A., Li, X., Liu, J., Liu, Y., et al. (2019). The Capacity of the Hydrological Modeling for Water Resource Assessment Under the Changing Environment in Semi-Arid River Basins in China. Water. 11 (7), 1328. doi:10.3390/w11071328
Guo, J., Chen, H., Xu, C.-Y., Guo, S., and Guo, J. (2012). Prediction of Variability of Precipitation in the Yangtze River Basin Under the Climate Change Conditions Based on Automated Statistical Downscaling. Stoch. Environ. Res. Risk Assess. 26 (2), 157–176. doi:10.1007/s00477-011-0464-x
Gupta, H. V., Kling, H., Yilmaz, K. K., and Martinez, G. F. (2009). Decomposition of the Mean Squared Error and NSE Performance Criteria: Implications for Improving Hydrological Modelling. J. Hydrol. 377, 80–91. doi:10.1016/j.jhydrol.2009.08.003
Haddeland, I., Heinke, J., Biemans, H., Eisner, S., Flörke, M., Hanasaki, N., et al. (2014). Global Water Resources Affected by Human Interventions and Climate Change. Proc. Natl. Acad. Sci. USA. 111 (9), 3251–3256. doi:10.1073/pnas.1222475110
Hassan, Z., Shamsudin, S., and Harun, S. (2014). Application of SDSM and LARS-WG for Simulating and Downscaling of Rainfall and Temperature. Theor. Appl. Climatol. 116, 243–257. doi:10.1007/s00704-013-0951-8
Hoekstra, A. Y. (2014). Water Scarcity Challenges to Business. Nat. Clim Change. 4 (5), 318–320. doi:10.1038/nclimate2214
Huang, X., Cai, X., Yang, P., and Zhao, J. (2014). Climate Change Trend Analysis in Western Route of South-To-North Water Diversion Project. South-To-North Water Transf. Water Sci. Technol. 12 (3), 5–9. doi:10.13476/j.cnki.nsbdqk.2014.03.002
IPCC (2013). Climate Change 2013: The Physical Science Basis. Cambridge and New York: Cambridge University Press.
IPCC (2021). Climate Change 2021: The Physical Science Basis. Cambridge and New York: Cambridge University Press.
Jin, J., Wang, G., Zhang, J., Yang, Q., Liu, C., Liu, Y., et al. (2020). Impacts of Climate Change on Hydrology in the Yellow River Source Region, China. J. Water Clim. Chang. 11, 916–930. doi:10.2166/wcc.2018.085
Jones, R. N., Chiew, F. H. S., Boughton, W. C., and Zhang, L. (2006). Estimating the Sensitivity of Mean Annual Runoff to Climate Change Using Selected Hydrological Models. Adv. Water Resour. 29 (10), 1419–1429. doi:10.1016/j.advwatres.2005.11.001
Kundzewicz, Z. W., Krysanova, V., Benestad, R. E., Hov, Ø., Piniewski, M., and Piniewski, I. M. (2018). Uncertainty in Climate Change Impacts on Water Resources. Environ. Sci. Pol. 79, 1–8. doi:10.1016/j.envsci.2017.10.008
Liu, C., and Xia, J. (2004). Water Problems and Hydrological Research in the Yellow River and the Huai and Hai River Basins of China. Hydrol. Process. 18, 2197–2210. doi:10.1002/hyp.5524
Liu, C., and Zheng, H. (2002). South-to-North Water Transfer Schemes for China. Int. J. Water Resour. Development. 18, 453–471. doi:10.1080/0790062022000006934
Liu, J., Bao, Z., Liu, C., Wang, G., Liu, Y., Wang, J., et al. (2019). Change Law and Cause Analysis of Water Resources and Water Consumption in China in Past 20 Years. Hydro-science Eng. 4, 31–41. doi:10.16198/j.cnki.1009-640x.2019.04.005
Liu, J., Yang, H., Gosling, S. N., Kummu, M., Flörke, M., Pfister, S., et al. (2017). Water Scarcity Assessments in the Past, Present, and Future. Earth's Future. 5 (6), 545–559. doi:10.1002/2016ef000518
Liu, X., Liu, C., Luo, Y., Zhang, M., and Xia, J. (2012). Dramatic Decrease in Streamflow from the Headwater Source in the Central Route of China's Water Diversion Project: Climatic Variation or Human Influence? J. Geophys. Res. 117, a–n. doi:10.1029/2011JD016879
Liu, X., Luo, Y., Yang, T., Liang, K., Zhang, M., and Liu, C. (2015). Investigation of the Probability of Concurrent Drought Events Between the Water Source and Destination Regions of China's Water Diversion Project. Geophys. Res. Lett. 42, 8424–8431. doi:10.1002/2015GL065904
Liu, Y., Zhang, J., Wang, G., Wang, G., Jin, J., Liu, C., et al. (2020). How Do Natural Climate Variability, Anthropogenic Climate and Basin Underlying Surface Change Affect Streamflows? A Three-Source Attribution Framework and Application. J. Hydro-environment Res. 28, 57–66. doi:10.1016/j.jher.2018.08.005
Long, D., Yang, W., Scanlon, B. R., Zhao, J., Liu, D., Burek, P., et al. (2020). South-to-North Water Diversion Stabilizing Beijing's Groundwater Levels. Nat. Commun. 11, 3665. doi:10.1038/s41467-020-17428-6
Lu, S., Bai, X., Li, W., and Wang, N. (2019). Impacts of Climate Change on Water Resources and Grain Production. Technol. Forecast. Soc. Change. 143, 76–84. doi:10.1016/j.techfore.2019.01.015
Lu, W., Wang, W., Shao, Q., Yu, Z., Hao, Z., Xing, W., et al. (2018). Hydrological Projections of Future Climate Change Over the Source Region of Yellow River and Yangtze River in the Tibetan Plateau: A Comprehensive Assessment by Coupling RegCM4 and VIC Model. Hydrological Process. 32 (13), 2096–2117. doi:10.1002/hyp.13145
Luo, M., Liu, T., Meng, F., Duan, Y., Bao, A., Xing, W., et al. (2019). Identifying Climate Change Impacts on Water Resources in Xinjiang, China. Sci. Total Environ. 676, 613–626. doi:10.1016/j.scitotenv.2019.04.297
Mehran, A., AghaKouchak, A., Nakhjiri, N., Stewardson, M. J., Peel, M. C., Phillips, T. J., et al. (2017). Compounding Impacts of Human-Induced Water Stress and Climate Change on Water Availability. Sci. Rep. 7, 6282. doi:10.1038/s41598-017-06765-0
Montanari, A., Bahr, J., Blöschl, G., Cai, X., Mackay, D. S., Michalak, A. M., et al. (2015). Fifty Years ofWater Resources Research: Legacy and Perspectives for the Science of Hydrology. Water Resour. Res. 51 (9), 6797–6803. doi:10.1002/2015wr017998
Moriasi, D. N., Arnold, J. G., Van Liew, M. W., Bingner, R. L., Harmel, R. D., and Veith, T. L. (2007). Model Evaluation Guidelines for Systematic Quantification of Accuracy in Watershed Simulations. Trans.ASABE. 50, 885–900. doi:10.13031/2013.23153
Nash, J. E., and Sutcliffe, J. V. (1970). River Flow Forecasting Through Conceptual Models Part I - A Discussion of Principles. J. Hydrol. 10 (3), 282–290. doi:10.1016/0022-1694(70)90255-6
Oki, T., and Kanae, S. (2006). Global Hydrological Cycles and World Water Resources. Science. 313 (5790), 1068–1072. doi:10.1126/science.1128845
Pokhrel, Y., Felfelani, F., Satoh, Y., Boulange, J., Burek, P., Gädeke, A., et al. (2021). Global Terrestrial Water Storage and Drought Severity Under Climate Change. Nat. Clim. Chang. 11, 226–233. doi:10.1038/s41558-020-00972-w
Qin, D., Ding, Y., and Mu, M. (2012). Climate and Environment Evolution: 2012. Beijing: Meteorology Press.
Rodell, M., Famiglietti, J. S., Wiese, D. N., Reager, J. T., Beaudoing, H. K., Landerer, F. W., et al. (2018). Emerging Trends in Global Freshwater Availability. Nature. 557, 651–659. doi:10.1038/s41586-018-0123-1
Schewe, J., Heinke, J., Gerten, D., Haddeland, I., Arnell, N. W., Clark, D. B., et al. (2013). Multimodel Assessment of Water Scarcity Under Climate Change. Proc. Natl. Acad. Sci. USA. 111 (9), 3245–3250. doi:10.1073/pnas.1222460110
Shahid, M., Cong, Z., and Zhang, D. (2017). Understanding the Impacts of Climate Change and Human Activities on Streamflow: a Case Study of the Soan River Basin, pakistan. Theor. Appl. Climatol. 134, 205. doi:10.1007/s00704-017-2269-4
She, D., Xia, J., Shao, Q., Taylor, J. A., Zhang, L., Zhang, X., et al. (2017). Advanced Investigation on the Change in the Streamflow into the Water Source of the Middle Route of China’s Water Diversion Project. J. Geophys. Res. Atmos. 122, 6950–6961. doi:10.1002/2016JD025702
Sivakumar, B. (2011). Global Climate Change and its Impacts on Water Resources Planning and Management: Assessment and Challenges. Stoch. Environ. Res. Risk Assess. 25, 583–600. doi:10.1007/s00477-010-0423-y
Stone, R., and Jia, H. (2006). HYDROENGINEERING: Going Against the Flow. Science. 313, 1034–1037. doi:10.1126/science.313.5790.1034
Su, B., Huang, J., Zeng, X., Gao, C., and Jiang, T. (2016). Impacts of Climate Change on Streamflow in the Upper Yangtze River Basin. Climatic Change. 141 (3), 533–546. doi:10.1007/s10584-016-1852-5
Sun, L., Wang, Y.-Y., Zhang, J.-Y., Yang, Q.-L., Bao, Z.-X., Guan, X.-X., et al. (2019). Impact of Environmental Change on Runoff in a Transitional basin: Tao River Basin from the Tibetan Plateau to the Loess Plateau, China. Adv. Clim. Change Res. 10 (4), 214–224. doi:10.1016/j.accre.2020.02.002
Sun, Y., Tian, F., Yang, L., and Hu, H. (2014). Exploring the Spatial Variability of Contributions From Climate Variation and Change in Catchment Properties to Streamflow Decrease in a Mesoscale Basin by Three Different Methods. J. Hydrol. 508, 170–180. doi:10.1016/j.jhydrol.2013.11.004
Tang, Q., Liu, X., Li, Z., Zhang, X., Yu, Q., et al. (2019). Integrated Water Systems Model for Terrestrial Water Cycle Simulation. Adv. Earth Sci. 34 (2), 115–123. doi:10.11867/j.issn.1001-8166.2019.02.0115
Tao, H., Gemmer, M., Jiang, J., Lai, X., and Zhang, Z. (2011). Assessment of CMIP3 Climate Models and Projected Changes of Precipitation and Temperature in the Yangtze River Basin, China. Climatic Change. 111 (3-4), 737–751. doi:10.1007/s10584-011-0144-3
Trudel, M., Doucet-Généreux, P.-L., and Leconte, R. (2017). Assessing River Low-Flow Uncertainties Related to Hydrological Model Calibration and Structure Under Climate Change Conditions. Climate. 5 (1), 19. doi:10.3390/cli5010019
Vörösmarty, C. J., McIntyre, P. B., Gessner, M. O., Dudgeon, D., Prusevich, A., Green, P., et al. (2010). Global Threats to Human Water Security and River Biodiversity. Nature. 467 (7315), 555–561. doi:10.1038/nature09440
Wang, G., Zhang, J., Xu, Y., Bao, Z., and Yang, X. (2017). Estimation of Future Water Resources of Xiangjiang River Basin With VIC Model Under Multiple Climate Scenarios. Water Sci. Eng. 10, 87–96. doi:10.1016/j.wse.2017.06.003
Wang, G., Zhang, J., Jin, J., Liu, Y., He, R., Bao, Z., et al. (2014). Regional Calibration of a Water Balance Model for Estimating Stream Flow in Ungauged Areas of the Yellow River Basin. Quat. Int. 336, 65–72. doi:10.1016/j.quaint.2013.08.051
Wang, G., Zhang, J., Jin, J., Pagano, T. C., Calow, R., Bao, Z., et al. (2012). Assessing Water Resources in China Using PRECIS Projections and VIC Model. Hydrol. Earth Syst. Sci. Discuss. 8, 7293–7317. doi:10.5194/hessd-8-7293-2011
Wang, G., and Zhang, J. (2015). Variation of Water Resources in the Huang-Huai-Hai Areas and Adaptive Strategies to Climate Change. Quat. Int. 380-381, 180–186. doi:10.1016/j.quaint.2015.02.005
Wang, G., Zhang, J., He, R., Liu, C., Ma, T., Bao, Z., et al. (2016). Runoff Sensitivity to Climate Change for Hydro-Climatically Different Catchments in China. Stoch. Environ. Res. Risk Assess. 31 (4), 1011–1021. doi:10.1007/s00477-016-1218-6
Wang, Y., Xie, Z., Jia, B., Wang, L., Li, R., Liu, B., et al. (2020). Sensitivity of Snow Simulations to Different Atmospheric Forcing Data Sets in the Land Surface Model CAS‐LSM. J. Geophys. Res. Atmos. 125, e2019JD032001. doi:10.1029/2019JD032001
Xia, J., Duan, Q.-Y., Luo, Y., Xie, Z.-H., Liu, Z.-Y., and Mo, X.-G. (2017). Climate Change and Water Resources: Case Study of Eastern Monsoon Region of China. Adv. Clim. Change Res. 8, 63–67. doi:10.1016/j.accre.2017.03.007
Xin, X., Wu, T., Zhang, J., Yao, J., and Fang, Y. (2020). Comparison of Cmip6 and Cmip5 Simulations of Precipitation in China and the East Asian Summer Monsoon. Int. J. Climatol. 40 (15), 6423–6440. doi:10.1002/joc.6590
Yan, B., and Chen, L. (2013). Coincidence Probability of Precipitation for the Middle Route of South-To-North Water Transfer Project in China. J. Hydrol. 499, 19–26. doi:10.1016/j.jhydrol.2013.06.040
Yin, Y., Wang, L., Wang, Z., Tang, Q., Piao, S., Chen, D., et al. (2020). Quantifying Water Scarcity in Northern China Within the Context of Climatic and Societal Changes and South‐to‐North Water Diversion. Earth's Future. 8, 1–17. doi:10.1029/2020EF001492
Yu, Z., Gu, H., Wang, J., Xia, J., and Lu, B. (2017). Effect of Projected Climate Change on the Hydrological Regime of the Yangtze River basin, china. Stoch. Environ. Res. Risk Assess. 32 (1), 1–16. doi:10.1007/s00477-017-1391-2
Yuan, F., Tung, Y.-K., and Ren, L. (2016). Projection of Future Streamflow Changes of the Pearl River Basin in China Using Two Delta-Change Methods. Hydrol. Res. 47 (1), 217–238. doi:10.2166/nh.2015.159
Zhang, H., Wang, B., Liu, D. L., Zhang, M., Feng, P., Cheng, L., et al. (2019). Impacts of Future Climate Change on Water Resource Availability of Eastern Australia: A Case Study of the Manning River basin. J. Hydrol. 573, 49–59. doi:10.1016/j.jhydrol.2019.03.067
Zhang, J., and Wang, G. (2007). Impact of Climate Change on Hydrology and Water Resources. Beijing: Science Press.
Zhang, L., Qin, L., Hu, Z., and Zeng, S. (2010). Simulated Hydrologic Responses to Climate Change of Water Source Area in the Middle Route of South-To-North Water Transfer Project. J. Hydraul. Eng. 41 (11), 1261–1271. doi:10.13243/j.cnki.slxb.2010.11.001
Zhang, Q. (2009). The South-To-North Water Transfer Project of China: Environmental Implications and Monitoring Strategy. J. Am. Water Resour. Assoc. 45 (5), 1238–1247. doi:10.1111/j.1752-1688.2009.00357.x
Zhao, M., Su, B., Jiang, T., Wang, A., and Tao, H. (2021). Simulation and Projection of Precipitation in the Upper Yellow River Basin by CMIP6 Multi-Model Ensemble[J]. Plateau Meteorology. 40 (3), 547–558. doi:10.7522/j.issn.1000-0534.2020.00066
Keywords: climate change, water resources, South-to-North Water Diversion Project, water source areas, GCM projections, RCCC-WBM
Citation: Qiao C, Ning Z, Wang Y, Sun J, Lin Q and Wang G (2021) Impact of Climate Change on Water Availability in Water Source Areas of the South-to-North Water Diversion Project in China. Front. Earth Sci. 9:747429. doi: 10.3389/feart.2021.747429
Received: 26 July 2021; Accepted: 07 September 2021;
Published: 12 October 2021.
Edited by:
Xander Wang, University of Prince Edward Island, CanadaReviewed by:
Caihong Hu, Zhengzhou University, ChinaCopyright © 2021 Qiao, Ning, Wang, Sun, Lin and Wang. This is an open-access article distributed under the terms of the Creative Commons Attribution License (CC BY). The use, distribution or reproduction in other forums is permitted, provided the original author(s) and the copyright owner(s) are credited and that the original publication in this journal is cited, in accordance with accepted academic practice. No use, distribution or reproduction is permitted which does not comply with these terms.
*Correspondence: Guoqing Wang, Z3F3YW5nQG5ocmkuY24=
Disclaimer: All claims expressed in this article are solely those of the authors and do not necessarily represent those of their affiliated organizations, or those of the publisher, the editors and the reviewers. Any product that may be evaluated in this article or claim that may be made by its manufacturer is not guaranteed or endorsed by the publisher.
Research integrity at Frontiers
Learn more about the work of our research integrity team to safeguard the quality of each article we publish.