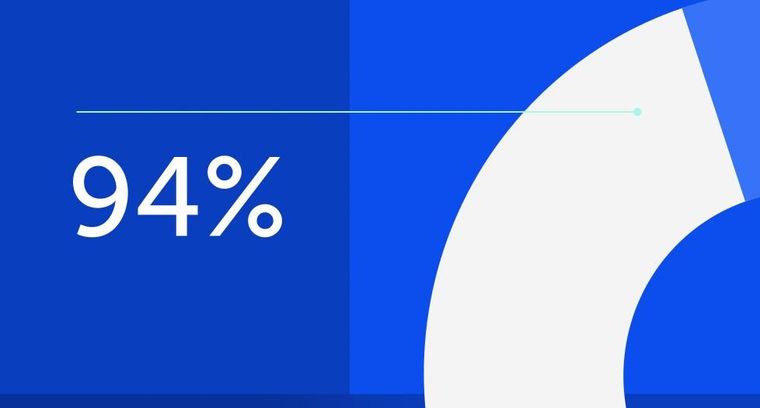
94% of researchers rate our articles as excellent or good
Learn more about the work of our research integrity team to safeguard the quality of each article we publish.
Find out more
ORIGINAL RESEARCH article
Front. Earth Sci., 01 November 2021
Sec. Interdisciplinary Climate Studies
Volume 9 - 2021 | https://doi.org/10.3389/feart.2021.699628
This article is part of the Research TopicThe Adaptation to and Mitigation of Climate-Sensitive Natural HazardsView all 21 articles
Understanding the precipitation variability and extreme precipitation over arid Central Asia (CA) has largely been hampered by the lack of daily precipitation observations. The gridded precipitation datasets over CA are large discrepancies. Here, three gauge-based gridded daily precipitation products from Asian Precipitation Highly-Resolved Observational Data Integration Towards Evaluation (APHRODITE), Global Precipitation Climatology Center (GPCC), and Climate Prediction Center Based Analysis of Global Daily Precipitation (CPC_global) were assessed and compared with 49 rain gauge daily observations precipitation (OBS) from January 1985 to December 2015 using different time-scales over CA and different climate regimes, specifically Northern CA with temperate continental climate (NCA), Southwestern CA with dry arid desert climate (SWCA), and Southeastern CA with Mediterranean continental climate (SECA). Four accuracy indices [correlation coefficient (R), Bias, root mean square error (RMSE), and relative bias (RBias)] were employed to evaluate the performance of the three products in depicting the spatiotemporal features of precipitation variation over CA at multiple time scales (including daily, monthly, seasonal, and yearly). The mean annual and daily precipitation of OBS and three gridded products exhibit the trend of a gradual precipitation decreased from SECA to NCA and SWCA. The best overall performance was obtained for APHRODITE and GPCC for daily and annual time-scale, whereas CPC shows noticeable underestimation precipitation in SECA. The monthly precipitation depicted distinct features with a bimodal pattern with a peak in March and another in December, include the SECA and SWCA regions. In contrast, precipitation was concentrated in summer with the peak in July over the NCA region. At monthly scale terms, APHRODITE was more accurate in the wet seasons (winter and spring months) in SWCA and SECA. Additionally, GPCC has fairly better capability in summer months in NCA. Considering the spatial distribution, the bias variability was largerly in mountainous areas than in the plains. Temporally, the bias largerly in the dry seasons than in the wet seasons. At the interannual variability scale, GPCC was capable of qualitatively increasing the CA (NCA and SECA) precipitation during the last 21 years, while APHRODITE underestimated the trends. The CPC overestimated the precipitation trends over all regions. This study can serve as a reference for selecting daily precipitation products with low densities of stations, complex topographies, and similar climatic regions.
Precipitation is an important component in hydrology and climate systems, and plays a vital role in driving land surface-atmosphere interactions (Yao et al., 2019; Tan et al., 2020). Accurate and reliable precipitation dataset on fine spatio-temporal resolutions is essential for water resources-related applications such as simulation hydrology and meteorology, prevention the extreme weather events, and mitigation natural hazards (Zhang et al., 2012; Schneider et al., 2016; Deng et al., 2017; Wu et al., 2019; He et al., 2020). A rain gauge is the most intuitive method of precipitation observation. Unfortunately, due to sparseness and unevenness distribution of in-situ observation, or the discontinuity in recording sequences, they do not provide a reliable spatiotemporal representation of precipitation (Gruber and Levizzani, 2008). This is specifically the case over complex regions where there is insufficient raingauge available and rainfall is distinguished by complex patterns especially over desert, mountainous terrain, and sparsely populated areas (Rana and McGregor, 2015; Yao et al., 2019). In this context, Gridded datasets are useful alternatives to ground observations and are widely used owing to their high resolution and open access (Serreze and Hurst, 2000; Silva et al., 2007; Pai et al., 2014; Guo et al., 2015a). Nevertheless, the multiple gridded precipitation datasets have pros and cons, so it is necessary to validate the applicability of products before further utilization (Henn et al., 2018; Sun et al., 2018; Tan et al., 2020).
With the development of instruments and techniques, gridded precipitation products have been generated based on in-situ observations and utilized different interpolation techniques, satellite estimates, numerical simulations, or a combination of these.They can be divided into three main categories: (1) the gauge-based data sets including the Climate Research Unit (CRU) (Harris et al., 2014), Global Precipitation Climatology Center (GPCC) (Ziese et al., 2018), CPC Gauge Based Analysis of Global Daily Precipitation (CPC_Global) (Chen et al., 2008), and University of Delaware (UDEL). (2) The satellite-based gridded datasets include Precipitation Estimation from Remotely Sensed Imagery Using Artificial Neural Networks (PERSIANN) (Sorooshian et al., 2000), CPC morphing technique (CMORPH) (Joyce et al., 2004), Global Satellite Mapping of Precipitation Moving Vector with Kalman filter (GSMaP_MVK) (Kubota et al., 2007), the Tropical Rainfall Measuring Mission (TRMM) Multi-satellite Precipitation Analysis (TMPA) (Huffman et al., 2007), and Multi-Source Weighted-Ensemble Precipitation (MSWEP) (Beck et al., 2017); (3) Reanalysis gridded products derived from physical and dynamical models, such as the National Center for Environmental Prediction (NCEP)/National Center for Atmospheric Research (NCAR) versions 1 and 2 (Kalnay et al., 1996; Kanamitsu et al., 2002), the European Centre for Medium-Range Weather Forecasts (ECMWF) systems (ERA-40 and ERA-Interim) (Uppala et al., 2005; Dee et al., 2011), Modern-Era Retrospective Analysis for Research and Application system (MERRA) (Rienecker et al., 2011) , and the Japanese 55-years Reanalysis (JRA55) (Ebita et al., 2011) and so on. Many studies have been conducted to assess the reliability of these datasets by comparing them with observed data (Dorigo et al., 2015; Henn et al., 2018; Ziese et al., 2018), and gridded precipitation products have been widely used in several aspects (Worqlul et al., 2015; Gao et al., 2018; Ahmed et al., 2019; Lemma et al., 2019).
Central Asia (CA) occupies the inland of the Eurasian continent, has more than 93% of the total ground as arid region (Yu et al., 2020), the development of society and economy is more sensitive than in humid regions (Huang et al., 2016). The climate of CA characterized by sharp temperature differences, intensive evaporation, and scarce precipitation (Lioubimtseva and Henebry, 2009; Zhang et al., 2017; Guan et al., 2019). As one of the world’s most arid areas are often regarded as primarily controlled by the midlatitude westerly at the continental scales (Dai and Wang, 2017). Given that CA features special geographical location and complex topography, this region is dominated by complex climate regimes. Precipitation is the principal source of the water resources in CA is limited to wet season rainfall and winter snow (Yao et al., 2020). Thus, changes precipitation over CA will alter regional hydrological cycles and influence on ecosystem and crucial for development of glaciers and accumulated snow (Lu et al., 2021). Hence, for a better understanding regulation of water resources in CA, it is crucial that one has good spatial and temporal precipitation datasets available. Some studies have documented that CA experienced a rapid warming trends (Chen et al., 2008), and accompanied with increased summer precipitation over the past half-century (Yao et al., 2016; Peng and Zhou, 2018). The frequency and intensity of extreme precipitation over CA increased significantly (Zhang et al., 2017; Yao et al., 2020). The precipitation variation over CA is significantly influenced by the Asian subtropical westerly jet, North Atlantic Oscillation (NAO), Arctic Oscillation (AO), El Niño-Southern Oscillation (ENSO), and Pacific Decadal Oscillation (PDO) (Aizen et al., 2001; Bothe et al., 2012; Tan and Shao, 2017; Wei et al., 2017; Chen et al., 2018). CA has a complex topography that also causes precipitation to present different regimes and most regions are sparsely populated deserts and mountain terrain with low density of rain gauges. This presents significant challenges in investigating spatial-temporal distribution and intensity of precipitation. it is important to systematically evaluate the daily precipitation products over CA.
A few studies have been carried out regarding the gridded precipitation products to detect climatic variations at local and regional scales. Using reanalyses, direct observations, and the output of climate model simulation driven by a global reanalysis, their abilities to represent the CA precipitation climate were compared (mean spatial distribution, seasonal cycle, and amplitude of interannual variability) (Schiemann et al., 2008). The results based on monthly gridded precipitation from the CRU analyzed the precipitation variability and regional difference over the CA during 1930–2009 (Chen et al., 2011). Guo et al. (2015b) used the TRMM v.7 dataset and compared it to the Asian Precipitation Highly Resolved Observational Data Integration Towards Evaluation (APHRODITE) for the period of 2004–2006. The comparison showed that TRMM significantly overestimated precipitation. Furthermore, both the satellite-only and gauge products exhibited poor performance in CA. Hu et al. (2017) evaluated reanalysis and spatially interpolated and remotely sensed precipitation datasets in CA, and found that MERRA dataset were more accurate than ERA-Interim and Climate Forecast System Reanalysis (CFSR) datasets, although they all overestimated the observed precipitation in CA. Song and Bai, 2016 used observational data and four gridded datasets (CRU, GPCC, MERRA, and TRMM) to describe regional and seasonal differences in precipitation climate characteristics over CA. Lai et al. (2020) investigated the fidelity of the APHRODITE in represent extreme precipitation over CA. Yang et al. (2020) using the daily MSWEP-V2.2 dataset from 1979 to 2016 across CA analyzed the spatiotemporal variability of the precipitation concentration and diversity. Lu (2021) While most evaluations have been conducted focus mainly on large temporal scales precipitation estimates. However, there is a need to investigate precipitation at daily resolution and consider specific geographic regions and different climatic status.
In the present study, three gauge-based gridded daily precipitation products (GPCC, APHRODITE, and CPC_global) were selected for assessing precipitation across CA and its sub-regions. First, we examined precipitation spatial characteristics between 1985 and 2005 based on 49 gauge site data over CA and three climatic zones. Then, the applicability of the three precipitation gridded products was systematically evaluated using multiple statistical analysis based on daily, monthly, seasonal, and annual time scales against observed data in the CA.
In this study, CA extending from 35° N to 55° N and from 45° E to 87° E, and covers five countries: Kazakhstan, Kyrgyzstan, Tajikistan, Uzbekistan, and Turkmenistan (Figure 1). The geomorphological features include high mountains and flat basins, with the elevations ranging from −132 m to about 7,500 m. Overall, CA is geographically high in the southeast and low in the northwest (Guo et al., 2017; Yang et al., 2020). The climate of CA is experiencing sharp differences in temperature, heterogeneous precipitation, and intense evaporation. Thus, it is sensitive and vulnerable to variations in climate change (Lioubimtseva and Henebry, 2009; De Beurs et al., 2018; Zhu et al., 2020). The annual precipitation can reach on 1,000 mm in the moutains areas in the southeastern CA, and the arid and desert climates with annual precipitation generally below 300 mm in the low areas in the northwestern CA (Lai et al., 2020; Lu et al., 2021).
FIGURE 1. Location of the topography and geographical position (A), and the rain gauges in different climatic zones (B) over CA (Df domain northern of the Kazakhstan (48°−54°N, 61°−86°E), BW domain southwestern of the Kazakhstan, Uzbekistan, Turkmenistan (35°−48°N, 54°−68°E), Ds mainly distributed in Kyrgyzstan and Tajikistan (37°−43°N, 68°−77°E).
According to the Köppen climate classification method (Chen and Chen, 2013. http://hanschen.org/koppen), we classified CA into three sub-regions (Figure 1B): temperate continental climate (Dfa) in the north (NCA), dry arid desert climate (BWk) in the southwest (SWCA), and Mediterranean continental like climate (Dsb) in the southeast (SECA). The total annual precipitation of CA is concentrated in cold seasons (Dai and Wang, 2017). Generally speaking, the CA is a typical region with complex rainfall conditions that deserves systematical evaluation the gridded daily precipitation products.
Global Historical Climatology Network-Daily (GHCN-D) is a composite of climate records from several sources (Menne et al., 2012). Some of the data provided here are based on data exchanged under the World Meteorological Organization (WMO) World Weather Watch Program according to WMO Resolution (Cg-XII). Thus, GHCN-D is the world’s largest collection of daily climatological data. GHCN-D includes over 40 meteorological elements (e.g., temperature daily maximum/minimum, precipitation, snowfall, and evaporation wind movement) and undergoes strict quality control (Durre et al., 2008; Durre et al., 2010). Daily meteorological observed precipitation data from a total of 568 stations covering the CA were obtained (ftp://ftp.ncdc.noaa.gov/pub/data). Given the observed data limited by the fall of the former Soviet Union since 1991, excluded the stations which records did not cover the period of January 1985 to December 2005, Furthermore, we selected the stations with observations data available for at least 90% of the number of days in any annual period during 1985–2005. Based on the two steps, after strict quality control and carefully checking by manually. As a result, 49 observation rain gauge sites were obtained, which are located in the CA. A total of 79.6% of the stations had complete data. The locations of the observed precipitation stations are shown in Figure 1B. Among them, 12, 19, and 18 sites were selected from the NCA, SWCA, and SECA subregions, respectively. These data are referred to as OBS in the following sections. The mean, maximum, standard deviation and the percentage of missing data of the OBS at different stations during 1985–2005 are listed in Table 1.
In this study, three gauge-based gridded daily precipitation products of APHRODITE, GPCC, and CPC_Global were selected to assess their performance in CA. The gauge-based gridded daily precipitation datasets in this study and their spatial and temporal resolutions and coverage are listed in Table 2. The subsection depicts the main features of the three gridded products, including their data sources, interpolation methods, and an assessment of errors.
1) APHRODITE: This product (Yatagai et al., 2009, 2012) uses the sufficient rain gauge observations of the entire Asian continent from 1951 to 2007 as the reference data set, after an initial quality control, improved Angular-Distance-Weighting interpolation, and improvement sphere map scheme, to build 0.25° × 0.25° and 0.5° × 0.5° gridded precipitation datasets. In this study, the 0.5° daily precipitation estimates from V11, covering 34°N–84°N and 15°E–165°W (APHRODITE_RU) were used. These data are referred to as APHRODITE in the following sections.
2) GPCC: This dataset, which is derived by the World Climate Research Program (WCRP), is based on a combination of observations from almost over 85,000 stations globally and has been compiled based on data from the Global Telecommunication System (GTS) (Xie and Arkin, 1997; Chen et al., 2002; Huffman et al., 2007; Becker et al., 2013).
3) CPC_Global: Global daily precipitation datasets from the CPC were developed by the American National Oceanic and Atmospheric Administration (NOAA), which provides daily 0.5° × 0.5° gridded precipitation data derived from the GTS gauge network (Fan and Van den Dool, 2008). An optimal interpolation was applied in the CPC.
The daily gridded data of APHRODITE, GPCC, and CPC from January 1, 1985 to December 31, 2005 were obtained in this study.
To evaluate the performance accuracy of gridded products in estimating the spatial and temporal variability of daily precipitation, four statistical metrics, correlation (R), root mean square error (RMSE), Bias, and relative bias (RBias) were used to measure the quantification of gridded precipitation products (Ghajarnia et al., 2015). Among them, R represents the linear correlation between OBS and the three gridded precipitation products. RMSE is sensitive to extreme error and can reflect the accuracy of gridded products, where RMSE values close to zero indicate smaller errors. Bias and RBias reflect the deviation between gridded products and rain gauge precipitation, describing the tendency of gridded precipitation to be over- or under-estimated as compared to observed precipitation. The formula for the statistical metrics mentioned above are listed in Table 3.
The spatial features of mean annual and seasonal precipitation from the 49 rain gauge measurements over CA during 1985–2005 have been presented in Figure 2. Mean annual precipitation exhibit the trend of a gradual precipitation decreased from southeastern to northwestern CA regions with precipitation hotspots located in SECA (more than 360 mm) and NCA (range of 200–300 mm).The area with the lowest average annual precipitation (less than 200 mm) expanded over the SWCA, which has an arid climate pattern and consists of desert areas (Figure 2A).
FIGURE 2. Spatial distribution of mean annual and seasonal precipitation in gauge-observation stations over the Central Asia during 1985−2005. (A), Annual; (B), Spring; (C), Summer; (D), Autumn; (E), Winter. unit: mm.
Figures 2B–E illustrate the spatial pattern of mean OBS seasonal precipitation over CA. In spring and winter, Areas with abundant precipitation are mainly distributed in the SECA (Figures 2B,E).The area-averaged precipitation was 99.6 and 86.4 mm, respectively. In contrast, less precipitation was observed in NCA during the cold seasons. The highest precipitation in the past 21 years over NCA concentrate in summer. In general, the annual and seasonal evolution of the OBS precipitation across CA were characterized by two major features: (1) mean annual precipitation decreased from the southeast to the north and southwest parts of the CA; and (2) there was more precipitation in the cold seasons (spring and winter) than in the warm seasons (summer and autumn). These two features of precipitation based on data from 49 rain gauges are consistent with those documented in the literature (Chen et al., 2009).
Figure 3 shows the spatial distribution of OBS, APHRODITE, GPCC, and CPC mean daily precipitation during 1985–2005 over CA. The OBS and three gridded products interpolated into each site displayed in (Figure 3A,B1–B3), and the gridded precipitation presented in (Figure 3C1–C3). All these three products accurately reproduced the spatial distribution of mean daily precipitation.
FIGURE 3. Spatial patterns of daily mean precipitation for gauge-observed and three gauge-based gridded products in the CA during 1985−2005. (A), OBS; (B1 And C1) APHRODITE; (B2 And C2), GPCC; (B3 And C3), CPC. unit: mm/day.
The spatial pattern of mean daily OBS precipitation (Figure 3A) was similar to the mean annual precipitation, presented an apparent decrease trend of precipitation from the southeast to north of CA. With the large mean daily precipitation in SECA (whithin 1–2 mm/ day), followed by NCA (whithin 0.6–0.8 mm/ day), and was the lowest in SWCA (less than 0.6 mm/ day). All three products captured the spatial distribution of mean daily precipitation characyeristics well, while CPC underestimated daily precipitation in the SECA area (Figure 3B3). The precipitation of five sites both in NCA and SWCA, and fifteen sites in SECA from CPC was lower than that of OBS. CPC had more sites where precipitation was underestimated as compared to APHRODITE and GPCC. Figures 3C1–C3 show the spatial distribution of gridded daily precipitation derived from APHRODITE, GPCC and CPC reflected the same features. However, GPCC showed more rainy in the NCA than APHRODITE and CPC. CPC showed that precipitation is scarce in SECA as compared to the other two products.
Figure 4 shows the density-colored scatterplots of 49 OBS mean daily precipitation versus three interpolated gridded products over the entire CA and subareas from 1985 to 2005 at daily scale. The correlation coefficients (R) derived from the three gridded products and OBS over the entire area and subareas were above 0.83, GPCC and APHRODITE more agreement with the OBS. The regression coefficient between GPCC and OBS was the highest, and almost coincided with the 1:1 line. In addition, the RMSE of GPCC displayed a lower value (0.28 mm/ day) and positive bias. APHRODITE also fitted well with OBS daily precipitation, while CPC showed worse performance with significantly underestimated values (RBias = −24.39%). Both APHRODITE and GPCC are performance better than CPC, as these has high Rs value (0.978 and 0.969) and low RMSEs value (0.24 mm/day and 0.28 mm/ day). CPC performs poorest as it exhibits the lowest R (0.84) and highest RMSE and RBias of 0.49 mm/ day and –24.39%, respectively. In the semi-arid climatic region (NCA, Figure 4B), all three products underestimated OBS daily precipitation, and the RBias were −12.39%, −3.02%, and −15.89% for APHRODITE, GPCC and CPC, respectively. RMSE values for APHRODITE, GPCC, and CPC were 0.57 mm/ day, 0.49 mm/ day, and 0.56 mm/ day, respectively. GPCC was obviously superior than other two precipitation products for higher R and lower RMSE. Based on the statistical index, APHRODITE performs best among the three products in SWCA region. GPCC ranks second with smaller Bias (Figure 4C). Figure 4D shows that the APHRODITE products had high accuracy with largest R (0.986) and lowest RMSE (0.42 mm/ day) at the daily scale in SECA region. The performance of GPCC was comparable than that of CPC, with the slightly better R and RMSE value. In general, CPC underestimated the daily precipitation over the whole CA. Based on RMSE that the error of CPC is larger than that of both APHRODITE and GPCC, the accuracy of CPC in daily scale is significantly lower than other two products.
FIGURE 4. Scatter plots of OBS daily precipitation versus APHRODITE (yellow circle), GPCC (green pentagon), CPC(blue triangle) over CA (A) and sub-regions (B–D) from January 11,985 to December 31, 2005. (B) represent in the NCA; (C) represent in the SWCA; (D) represent in the SECA.The black dotted lines are the 1:1 line, and the yellow, green, and blue solid lines represents the linear regression fitting lines for APHRODITE, GPCC, and CPC, respectively.
Figure 5 shows the spatial distribution of the four statistical metrics for APHRODITE, GPCC, and CPC products against with OBS over the CA from 1985 to 2005. Figures 5A–C show the spatial distribution of R derived from three interpolated gauge-based gridded precipitation datasets in each individual station. All three gridded products displayed a strong correlation, with R values higher than 0.8 accounting for 83.7, 83.7, and 65.3% of all stations for APHRODITE, GPCC and CPC, respectively. Figure 5A shows the higher R values of APHRODITE located in the SWCA and SECA. Figures 5B,C indicates the R values of GPCC (CPC) in NCA and SECA were marginally higher than those in the SWCA. Figures 5D–F show the spatial distribution of RMSE in each individual sites. The mean RMSE values of APHRODITE, GPCC, and CPC over the entire CA were 1.46 mm/ day, 1.5 mm/ day, and 1.73 mm/ day, respectively. The RMSE values of APHRODITE were higher than those of GPCC and CPC in the NCA. In the arid desert climatic zone (SWCA), the RMSEs were the lowest, the number of stations marked by blue (higher than 1.5 mm/ day) of CPC (Figure 5F) over SECA were more than that of APHRODITE and GPCC, which indicates that RMSE values of CPC were higher than those of APHRODITE and GPCC. The spatial distribution of Bias for each pair of products were depict in Figures 5G–I. Approximately 59.2% of the stations showed positive bias for APHRODITE and GPCC against OBS CPC underestimated daily precipitation over almost all the regions, 95.9% of the stations showed negative bias of CPC across the entire CA. The last column in Figure 5 shows the spatial distribution of RBias between gridded products versus OBS. Better performance in terms of RBias values at the range of −10 to 10% that was mainly distributed in SECA (SWCA) for the APHRODITE (GPCC) products. In general, both APHRODITE and GPCC products shows positive deviation in most regions, while CPC present large negative deiation over almost all the CA.
FIGURE 5. Spatial distribution of statistical metrics for three gridded daily precipitation products over the CA from 1985 to 2005. (Each row is APHRODITE, GPCC, and CPC. Each column is R, RMSE, Bias, and RBias. (A), (D), (G), and (J) for APHRODITE; (B), (E), (H), and (K) for GPCC; (C), (F), (I), and (L) for CPC).
The monthly change of regional precipitation for OBS and three interpolated products from 1985 to 2005 over CA and each sub-regions were given in Figure 6. It is clearly indicate that there are seasonal differences in each climatic zones. Figure 6A shows the precipitation in the entire CA is concentrated in spring and winter from March to May and from November to December, respectively. In SWCA (Figure 6C) and SECA (Figure 6D), a similar monthly precipitation pattern was illustrated as in CA, corresponding to the double peak precipitation type. On the contrary, monthly precipitation is concentrated in summer from May to August in NCA (Figure 6B), corresponding to the single peak type, where the maximum value occurred in July (43 mm/ month).
FIGURE 6. The annual cycle of the OBS (gray columnar), APHRODITE (yellow circled line), GPCC (Green pentagonal line), and CPC (Blue line with triangles) precipitation over the CA (A) and sub-regions (B), NCA; (C), SWCA; (D), SECA during 1985−2005. (The numbers in the upper left corner of each sub-figures represent the regional average precipitation in spring, summer, autumn and winter calculated by each product, respectively.)
Monthly precipitation obtained from all three gridded precipitation products was consistent with the variation of OBS in each region. In NCA (Figure 6B), the monthly precipitation estimated by the three interpolated gridded products reasonably described the actual observations while GPCC was the closest. In general, variations of monthly precipitation from three products were similar to the OBS, and the estimates of monthly precipitation from APHRODITE and GPCC were closer to OBS has better performance. while the CPC product underestimated the precipitation obtained from gauge measurements and showed poor performance.
The statistical metrics (R, RMSE, Bias, and RBias) are computed from gridded products against with OBS over CA and its sub-regions during 1985–2005. The specific results are shown in Figure 7. In terms of R, all three products showed strong correlations with OBS over CA at monthly scale, with the values ranges from 0.8 to 1 (Figure 7A). The RMSE was high from March to April and October to December, and low from June to September (Figure 7D). This is consistent with the monthly precipitation over CA, though CPC performed worse than APHRODITE and GPCC. As for Bias (Figure 7I), the results show that APHRODITE underestimated the precipitation, except in July. The GPCC overestimated the monthly precipitation from April to July. CPC had a large error and poor performance. Figures 7B,F,J,N illustrates that the agreement between OBS monthly precipitation and the three gridded products was higher over the NCA. The results of RMSE and Bias show that GPCC had a lower error and better performance than APHRODITE and CPC. APHRODITE and CPC underestimated and had the worst performance for precipitation during the rainy season (from June to August). In the mainly arid desert SWCA area, with respect to RMSE (Figure 7G), the three gridded products showed low errors in scarce precipitation months (from June to September), and APHRODITE had lower error than GPCC and CPC. APHRODITE and GPCC showed better performance than CPC, as reflected by the results of Bias (Figure 7K) and RBias (Figure 7K), and CPC had the highest negative deviation with the ranges from −22.9% to −13.7%. Based on the R values over the SECA region (Figure 7C), the three gridded products had relatively higher correlations in most months (except July and August). APHRODITE had lower RMSE values than GPCC and CPC at the monthly scale, APHRODITE and GPCC underestimated the precipitation during the rainy months and overestimated precipitation in the scarce precipitation months (from June to September), and CPC tended to underestimate precipitation. APHRODITE and GPCC showed better performance than CPC, as reflected by the results of RBias.
FIGURE 7. The statistics metrics of average precipitation comparing the OBS with APHRODITE (yellow), GPCC (green) and CPC (blue) at monthly scale for the different regions. (A), (E), (I), and (M) for CA; (B), (F), (J), and (N) for NCA; (C), (G), (K), and (O) for SWCA; (D), (H), (L), and (P) for SECA.
For CA and each sub-region, the results for statistic metrics of the three gridded products versus OBS precipitation at seasonal scale, sorted from bad to good performance was supplied in Figure 8. It can be seen that all three gridded products had higher correlations (>0.87, statistically significant at the 99% confidence level), and the R values of APHRODITE and GPCC were larger than that of CPC across CA in all seasons. The RMSE values of APHRODITE were lower than those of GPCC and CPC, Bias and RBias of GPCC were positive and the values were lower than those of APHRODITE and CPC across CA in Spring, Summer, and Winter. In all seasons, the GPCC product has a lower error and good performance than APHRODITE and CPC over NCA. In Spring, GPCC overestimated precipitation, and APHRODITE and CPC underestimations. The RBias values of APHRODITE, GPCC, and CPC were −9.68%, 0.6%, and −15.41%, respectively. The three gridded products underestimated precipitation in Summer, Autumn, and Winter. The RMSE of GPCC was less than that of APHRODITE and CPC in summer over SWCA (0.21 mm/ day for GPCC, 0.23 mm/ day for APHRODITE, and 0.26 mm/ day for CPC). APHRODITE performed better than GPCC and CPC during Spring, Autumn, and Winter seasons. Additionally, GPCC overestimated precipitation, and both APHRODITE and CPC were underestimated precipitation in all seasons over the SWCA area. APHRODITE and GPCC performed better than CPC in all seasons over SECA. Precipitation in the SECA region was overestimated by APHRODITE during Summer (RBias of 13.45%) and underestimated in other seasons. The GPCC product overestimated precipitation in Spring and Summer (RBias for Spring was 2.07% and for summer was 12.73%).
FIGURE 8. statistic metrics of daily precipitation between OBS and three gridded products over the CA and subregion at seasonal scale.
Figure 9 shows the time series of the interannual variability of precipitation derived from OBS and gridded products over CA and three sub-regions period of 1985–2005. The amplitude in terms of the interannual variability for three gridded precipitation products are basically consistent with OBS, but they had apperent differences in the magnitude and trends over whole CA and each sub-regions. Both GPCC and APHRODITE has a similar trend with OBS in each region, while GPCC performs outstand.
FIGURE 9. Annual precipitation variations for OBS (red line with square), APHRODITE (yellow circled line), GPCC (green pentagonal line), and CPC (blue line with triangles) in the CA (A), NCA (B), SWCA (C), and SECA (D) from 1985 to 2005 ( unit:mm ).
The dispersion of boxplots of the statistical metrics for three gridded precipitation products over CA and sub-areas during 1985–2005 were illustrated in Figure 10. APHRODITE showed largest R (within 0.93–0.99) and smallest RMSE (varied from 0.15 to 0.36 mm/ day, with the medians value at 0.23 mm/ day), which indicated that APHRODITE performed best among the three gridded products over the entire CA (Figures 10A,E). GPCC and CPC showed relatively large error based on RMSE, whoes values of GPCC and CPC varied from 0.16 to 0.49 mm/ day and from 0.26 to 0.76 mm/ day, respectively. For the perspective of RBias, APHRODITE and CPC were underestimated the annual precipitation while GPCC overestimated with the 0.74% medians of RBias (Figure 10I) over the entire CA.
FIGURE 10. Boxplots of the statistical metrics derive APHRODITE (yellow), GPCC (green) and CPC (blue) daily precipitation from OBS in the CA (A), (E), and (I); NCA (B), (F), and (J); SWCA (C), (G), and (K); SECA (D), (H), and (L). (Two dots in bottom and top for each box represent minimum and maximum value, respectively. Three lines from bottom to top represent 25th percentile, 50th percentile and 75th percentile, respectively. The inside box represent the mean values. ).
The second row shows the boxblots of statistical metrics from three precipitation products at annual scale over NCA (Figures 10B,F,J). Figure 10B shows thes R values of GPCC were higher than those of APHRODITE and CPC. The RMSE values of GPCC were lower than that of APHRODITE and CPC, the medians RMSE of GPCC, APHRODITE, and GPCC were 0.47 mm/ day, 0.54 mm/ day, 0.54 mm/day, respectively. (Figure 10F). The medians RBias values was −12.19% for APHRODITE, −2.79% for GPCC, and −15.88% for CPC. The large deviations are derived from OBS precipitation data and APHRODITE and CPC products. Figures 10C,G,K shows the results for R, RMSE, and RBias in the SWCA sub-area. APHRODITE accurately estimated precipitation, as reflected by a higher R (0.8–0.97 with medians value of 0.92), lower RMSE (0.19–0.44 mm/ day with a medians value of 0.29 mm/ day), and lower RBias (−11.7–2.29% with a medians value of −4.58%). GPCC performed better as the mean RBias values were lower (−3.02–17.63% with a medians value of 3.96%), which overestimated precipitation at the annual scale. In contrast, CPC showed the worst performance with lower R (0.53–0.96 with a medians value of 0.86), higher RMSE (0.21–0.69 mm/ day with a medians value of 0.39 mm/ day), and lower RBias (−0.15–34.42% with a mean value of −18.87%) over the SWCA area. Figures 10D,H,L shows the R, RMSE, and Bias of the three products in the SECA region. As shown in Figure 10D, the mean R values of both APHRODITE and GPCC precipitation gridded products were higher that of CPC (the medians R values were 0.98 for APHRODITE, 0.97 for GPCC, and 0.93 for CPC). Figure 10H shows that the mean RMSE value of APHRODITE was lower than those of GPCC and CPC (medians values were 0.39 mm/ day for APHRODITE, 0.51 mm/ day for GPCC, and 1.04 mm/ day for CPC). Figure 10L shows that the medians RBias value of APHRODITE was lower than those of GPCC and CPC (medians value of APHRODITE was −2.1%, of GPCC was 1.48%, and of CPC was −21.12%). APHRODITE and CPC underestimated the precipitation over SECA region.
To clarify the gauge-based gridded precipitation data in reproduce the spatial patten of the interannual variability, Figure 11 depicts the spatial distribution of the annual precipitation linear trends over CA and subregions during 1985–2005. As shown in Figure 11A, the OBS precipitation displayed widespread significant increasing trends across the entire region of CA with 85.71% of stations having a rate of 20.01 mm/10a (Figure 11A). The annual precipitation for NCA, SWCA, and SECA sub-regions of CA showed rising trends at 83.33, 89.47, and 83.33% of the stations, with rates of 18.7 mm/10a, 5.9 mm/10a, and 36 mm/10 a, respectively.
FIGURE 11. Spatial distribution of the annual precipitation trend of OBS (A), APHRODITE (B1), GPCC (C1) and CPC (D1) over the CA from 1985 to 2005 (the black dots represent trends were significant at the 0.05 level ).
Figures 11B1,C1,D1 displays three interpolated gridded precipitation products for each site for the period 1985–2005. The annual precipitation trends of APHRODITE were generally lower than those of GPCC and CPC (the trend of APHRODITE was 6.95 mm/10 a, of GPCC was 17.33 mm/10 a, and of CPC was 33.11 mm/10 a) over the whole CA. The GPCC product tendencies were in agreement with the OBS, while the APHRODITE underestimated and CPC significantly overestimated the precipitation trends for rain gauges over CA. In the NCA (Figure 11B1), the annual precipitation displayed increasing trends at 14.5 mm/10 a, 15.7 mm/10 a, and 23.4 mm/10a for APHRODITE, GPCC, and CPC, respectively. This indicates that APHRODITE and GPCC underestimated the precipitation trends, while the CPC product overestimated precipitation trends in the entire NCA. In the SWCA (Figure 11C1), APHRODITE generally matched the precipitation trends over 21 years of OBS (OBS = 5.9 mm/10 a, APHRODITE = 5.6 mm/10 a). GPCC and CPC overestimated the trends of precipitation (OBS = 7.4 mm/10 a, APHRODITE = 23.4 mm/10 a). In the SECA with higher elevation regions (Figure 11D1), APHRODITE underestimated the trends of annual precipitation, while CPC significantly overestimated (annual precipitation trend for APHRODITE was 12.8 mm/10 a and for CPC was 52.7 mm/10 a). The GPCC results were in agreement with OBS at a rate of 37.4 mm/10 a. Figures 11B2,C2,D2 shows the gridded distribution of annual precipitation trends of APHRODITE, GPCC, and CPC. The distribution of APHRODITE and GPCC was similar, though APHRODITE exhibited a decreasing trend in most parts of the SECA than GPCC. CPC showed an increasing trend in most regions of the CA, and overestimated the interannual variability for the underestimation of the climatology.
In this study, we systematical evaluated three gridded daily precipitation products (APHRODITE, GPCC, and CPC) by compared against with 49 rain gauge observations (OBS) employed four statistical metrics (R, Bias, RMSE, and RBias) in the period of 1985–2005 over CA, a region with complex climate conditions and topographic. The major conclusions are summarized as follows.
Based on the map of the köppen climatic classification divided the entire CA into three sub-regions, which is a temperate continental climate (Dfa) zone in the Northern CA (NCA), dry arid desert climate (BWk) zone in the Southwestern CA (SWCA), and the Mediterranean continental climate (Dsb) zone in the Southeastern CA (SECA). Overall, the three products can reproduce the mean climatology, annual cycle, and interannual variability of precipitation with OBS. The spatial distribution pattern of mean annual and daily precipitation decreased from the SECA to the NCA and SWCA, and the precipitation was highest in winter and spring and lower in summer over CA, SECA, and SWCA. On the contrary, the precipitation was mainly concentrated in the summer across NCA.
Considering the different time scales (daily, monthly, seasonal, and yearly), APHRODITE and GPCC has a fairly well performance than CPC. In terms of daily scale, the statistical results of R indicated that all three datasets had higher correlations with OBS in CA and each sub-region. According to the RMSE and Bias, GPCC performs best among three products with the lowest RMSE (0.28 mm/ day) and positive lowest RBias (0.71%). APHRODITE ranks second, CPC performs worst in the whole CA. The statistical metrics of GPCC (APHRODITE) to evaluated daily precipitation in the NCA (SECA and SWCA) is obviously superior than that in southern parts of CA (NCA). Since there exhibits different variation distribution in annual cycle precipitation over CA, the statistical metrics are display similar amplitudes with monthly precipitation. APHRODITE shows the better performance in CA, SWCA, and SECA regions with higher R and lower RMSE, and performs well in dry seasons than wet seasons. GPCC exhibits more accurary in wet months in NCA. CPC quantitatively underestimated the magnitude of precipitation over most regions of CA for all months. The bias in variability was larger in mountainous areas than in plain areas. The interannual variability reveal that APHRODITE and GPCC has capable of qualitatively changing the precipitation of CA, whereas CPC is not reliable.
All gridded products are associated with uncertain, the algorithm error, and influenced by limited number of stations to interpolate schemes. The multiple scales characteristics and errors of the APHRODITE, GPCC, and CPC products were quantitatively and qualitatively evaluated over CA the results provides interesting insights into daily precipitation estimation in data scarce regions, and benefit to many scientific studies and applications in CA.
The original contributions presented in the study are included in the article/Supplementary Materials, further inquiries can be directed to the corresponding author.
DT analyzed the results, and wrote the manuscript. JY conceived the work, reviewed and edited the manuscript. JC and JL made the contribution to the methodology, visualization and Investigation. YZ, WM, and LY reviewed and approved the manuscript.
This work was funded by the National Natural Science Foundation of China (U1903113, 42171038), Sichuan Science and Technology Program (2020JDJQ0050). Climate Change Special Project of China Meteorological Administration (CCSF202028).
The authors declare that the research was conducted in the absence of any commercial or financial relationships that could be construed as a potential conflict of interest.
All claims expressed in this article are solely those of the authors and do not necessarily represent those of their affiliated organizations, or those of the publisher, the editors and the reviewers. Any product that may be evaluated in this article, or claim that may be made by its manufacturer, is not guaranteed or endorsed by the publisher.
The authors would like to express their gratitude to all the providers of precipitation products.
Ahmed, K., Shahid, S., Wang, X., Nawaz, N., and Najeebullah, K. (2019). Evaluation of gridded precipitation datasets over arid regions of Pakistan. Water 11 (2), 210. doi:10.3390/w11020210
Aizen, E. M., Aizen, V. B., Melack, J. M., Nakamura, T., and Ohta, T. (2001). Precipitation and atmospheric circulation patterns at mid-latitudes of Asia. Int. J. Climatol. 21, 535–556. doi:10.1002/joc.626
Beck, H. E., van Dijk, A. I. J. M., Levizzani, V., Schellekens, J., Miralles, D. G., Martens, B., et al. (2017). MSWEP: 3-hourly 0.25° global gridded precipitation (1979-2015) by merging gauge, satellite, and reanalysis data. Hydrol. Earth Syst. Sci. 21 (1), 589–615. doi:10.5194/hess-21-589-2017
Becker, A., Finger, P., Meyer-Christoffer, A., Rudolf, B., Schamm, K., Schneider, U., et al. (2013). A description of the global land-surface precipitation data products of the Global Precipitation Climatology Centre with sample applications including centennial (trend) analysis from 1901–present. Earth Syst. Sci. Data 5, 71–99. doi:10.5194/essd-5-71-2013
Bothe, O., Fraedrich, K., and Zhu, X. (2012). Precipitation climate of central Asia and the large-scale atmospheric circulation. Theor. Appl. Climatol. 108, 345–354. doi:10.1007/s00704-011-0537-2
Chen, D., and Chen, H. W. (2013). Using the Köppen classification to quantify climate variation and change: An example for 1901-2010. Environ. Dev. 6, 69–79. doi:10.1016/j.envdev.2013.03.007
Chen, F., Huang, W., Jin, L., Chen, J., and Wang, J. (2011). Spatiotemporal precipitation variations in the arid central Asia in the context of global warming. Sci. China Earth Sci. 54, 1812–1821. doi:10.1007/s11430-011-4333-8
Chen, F. H., Chen, J. H., and Huang, W. (2009). A discussion on the westerly-dominated climate model in mid-latitude Asia during the modern interglacial period. Earth Sci. Front. 16, 23–32.
Chen, M., Xie, P., Janowiak, J. E., and Arkin, P. A. (2002). Global land precipitation: a 50-year monthly analysis based on gauge observations. J. Hydrometeor. 3, 249–266. doi:10.1175/1525-7541(2002)003<0249:GLPAYM>2.0.CO;2
Chen, M. P., Xie, P., and Co-authors, (2008). CPC Unified Gauge-based Analysis of Global Daily Precipiation, Western Pacific Geophysics Meeting. Australia: Cairns. 29 July - 1 August, 2008.
Chen, X., Wang, S. S., Hu, Z. Y., Zhou, Q. M., and Hu, Q. (2018). Spatiotemporal characteristics of seasonal precipitation and their relationships with ENSO in Central Asia during 1901-2013. J. Geogr. Sci. 28 (9), 1341–1368. doi:10.1007/s11442-018-1529-2
Dai, X.-G., and Wang, P. (2017). A new classification of large-scale climate regimes around the Tibetan Plateau based on seasonal circulation patterns. Adv. Clim. Change Res. 8, 26–36. doi:10.1016/j.accre.2017.01.001
De Beurs, K. M., Henebry, G. M., Owsley, B. C., and Sokolik, I. N. (2018). Large scale climate oscillation impacts on temperature, precipitation and land surface phenology in Central Asia. Environ. Res. Lett. 13, 065018. doi:10.1088/1748-9326/aac4d0
Dee, D. P., Uppala, S. M., Simmons, A. J., Berrisford, P., Poli, P., Kobayashi, S., et al. (2011). The ERA-Interim reanalysis: Configuration and performance of the data assimilation system. Q.J.R. Meteorol. Soc. 137 (656), 553–597. doi:10.1002/qj.828
Deng, X., Nie, S., Deng, W., and Cao, W. (2017). Statistical evaluation of the performance of gridded monthly precipitation products from reanalysis data, satellite estimates, and merged analyses over China. Theor. Appl. Climatol 132 (1–2), 621–637. doi:10.1007/s00704-017-2105-x
Dorigo, W. A., Gruber, A., De Jeu, R. A. M., Wagner, W., Stacke, T., Loew, A., et al. (2015). Evaluation of the ESA CCI soil moisture product using ground-based observations. Remote Sensing Environ. 162, 380–395. doi:10.1016/j.rse.2014.07.023
Durre, I., Menne, M. J., Gleason, B. E., Houston, T. G., and Vose, R. S. (2010). Comprehensive Automated Quality Assurance of Daily Surface Observations. J. Appl. Meteorol. Climatol. 49, 1615–1633. doi:10.1175/2010JAMC2375.1
Durre, I., Menne, M. J., and Vose, R. S. (2008). Strategies for evaluating quality assurance procedures. J. Appl. Meteorol. Climatol. 47, 1785–1791. doi:10.1175/2007JAMC1706.1
Ebita, A., Kobayashi, S., Ota, Y., Moriya, M., Kumabe, R., Onogi, K., et al. (2011). The Japanese 55-year Reanalysis "JRA-55": An Interim Report. SOLA 7, 149–152. doi:10.2151/sola.2011-038
Fan, Y., and Van den Dool, H. (2008). A global monthly land surface air temperature analysis for 1948–present. J. Geophys. Res. Atmos. 113, 1–18. doi:10.1029/2007JD008470
Gao, X. C., Zhu, Q., Yang, Z. Y., and Wang, H. (2018). Evaluation and Hydrological Application of CMADS against TRMM 3B42V7, PERSIANN-CDR, NCEP-CFSR, and gauge-based datasets in Xiang River Basin of China. Water 10 (9), 24. doi:10.3390/w10091225
Ghajarnia, N., Liaghat, A., and Arasteh, D. (2015). Comparison and evaluation of high resolution precipitation estimation products in Urmia Basin-Iran. Atmos. Res. 158-159 (50–65), 50–65. doi:10.1016/j.atmosres.2015.02.010
Gruber, A., and Levizzani, V. (2008). Assessment of Global Precipitation Products, A project of the World Climate Research Programme Global Energy and Water Cycle Experiment (GEWEX) radiation panel. WCRP Rep. No.128, WMO/TD No. 1430(50 pp.)
Guan, X. F., Yang, L. M., Zhang, Y. X., and Li, J. G. (2019). Spatial distribution, temporal variation, and transport characteristics of atmospheric water vapor over Central Asia and the arid region of China. Global Planet. Change 172, 159–178. doi:10.1016/j.gloplacha.2018.06.007
Guo, H., Bao, A., Ndayisaba, F., Liu, T., Kurban, A., and De Maeyer, P. (2017). Systematical Evaluation of Satellite Precipitation Estimates Over Central Asia Using an Improved Error-Component Procedure. J. Geophys. Res. Atmos. 122 (10), 906–910. doi:10.1002/2017JD026877
Guo, H., Chen, S., Bao, A., Hu, J., Gebregiorgis, A., Xue, X., et al. (2015b). Inter-comparison of high-resolution satellite precipitation products over Central Asia. Remote Sensing 7 (6), 7181–7211. doi:10.3390/rs70607181
Guo, H., Chen, S., Bao, A., Hu, J., Yang, B., and Stepanian, P. (2015a). Comprehensive Evaluation of High-Resolution Satellite-Based Precipitation Products over China. Atmosphere 7 (1), 6. doi:10.3390/atmos7010006
Harris, I., Jones, P. D., Osborn, T. J., and Lister, D. H. (2014). Updated high-resolution grids of monthly climatic observations - the CRU TS3.10 Dataset. Int. J. Climatol. 34 (3), 623–642. doi:10.1002/joc.3711
He, X., Pan, M., Wei, Z., Wood, E. F., and Sheffield, J. (2020). A global drought and flood catalogue from 1950 to 2016. B. Am. Meteorol. Soc. 101, E508–E535. doi:10.1175/bams-d-18-0269.1
Henn, B., Newman, A. J., Livneh, B., Daly, C., and Lundquist, J. D. (2018). An assessment of differences in gridded precipitation datasets in complex terrain. J. Hydrol. 556, 1205–1219. doi:10.1016/j.jhydrol.2017.03.008
Hu, Z., Zhou, Q., Chen, X., Qian, C., Wang, S., and Li, J. (2017). Variations and changes of annual precipitation in Central Asia over the last century. Int. J. Climatol 37 (S1), 157–170. doi:10.1002/joc.4988
Huang, J., Ji, M., Xie, Y., Wang, S., He, Y., and Ran, J. (2016). Global semi-arid climate change over last 60 years. Clim. Dyn. 46, 1131–1150. doi:10.1007/s00382-0150263608
Huffman, G. J., Bolvin, D. T., Nelkin, E. J., Wolff, D. B., Adler, R. F., Gu, G., et al. (2007). The TRMM Multisatellite Precipitation Analysis (TMPA): Quasi-global, multiyear, combined-sensor precipitation estimates at fine scales. J. Hydrometeorology 8 (1), 38–55. doi:10.1175/jhm560.1
Joyce, R. J., Janowiak, J. E., Arkin, P. A., and Xie, P. (2004). CMORPH: A Method that Produces Global Precipitation Estimates from Passive Microwave and Infrared Data at High Spatial and Temporal Resolution. J. Hydrometeor 5, 487–503. doi:10.1175/1525-7541(2004)005<0487:camtpg>2.0.co;2
Kalnay, E., Kanamitsu, M., Kistler, R., Collins, W., Deaven, D., Gandin, L., et al. (1996). The NCEP/NCAR 40-year reanalysis project. Bull. Amer. Meteorol. Soc. 77, 437–471. doi:10.1175/1520-0477(1996)077<0437:tnyrp>2.0.co;2
Kanamitsu, M., Ebisuzaki, W., Woollen, J., Yang, S.-K., Hnilo, J. J., Fiorino, M., et al. (2002). NCEP-DOE AMIP-II reanalysis (R-2). Bull. Amer. Meteorol. Soc. 83, 1631–1644. doi:10.1175/bams-83-11-1631
Kubota, T., Shige, S., Hashizume, H., Aonashi, K., Takahashi, N., Seto, S., et al. (2007). Global precipitation map using satellite-borne microwave radiometers by the GSMap project: Production and validation. IEEE Trans. Geosci. Remote Sensing 45 (7), 2259–2275. doi:10.1109/Tgrs.2007.895337
Lai, S., Xie, Z., Bueh, C., and Gong, Y. (2020). Fidelity of the APHRODITE dataset in representing extreme precipitation over Central Asia. Adv. Atmos. Sci. 37 (12), 1405–1416. doi:10.1007/s00376-020-0098-3
Lemma, E., Upadhyaya, S., and Ramsankaran, R. (2019). Investigating the performance of satellite and reanalysis rainfall products at monthly timescales across different rainfall regimes of Ethiopia. Int. J. Remote Sensing 40 (10), 4019–4042. doi:10.1080/01431161.2018.1558373
Lioubimtseva, E., and Henebry, G. M. (2009). Climate and environmental change in arid Central Asia: Impacts, vulnerability, and adaptations. J. Arid Environments 73, 963–977. doi:10.1016/j.jaridenv.2009.04.022
Lu, X. Y., Tang, G. Q., Liu, X. C., Wang, X. Q., and Wei, M. (2021). The potential and uncertainty of triple collocation in assessing satellite precipitation products in Central Asia. Atmos. Res. 252, 105452. doi:10.1016/j.atmosres.2021.105452
Menne, M. J., Durre, I., Vose, R. S., Gleason, B. E., and Houston, T. G. (2012). An overview of the Global Historical Climatology Network-Daily database. J. Atmos. Oceanic Technol. 29, 897–910. doi:10.1175/JTECH-D-11-00103.1
Pai, D. S., Sridhar, L., Rajeevan, M., Sreejith, O. P., Satbhai, N. S., and Mukhopadyay, B. (2014). Development of a new high spatial resolution (0. 25° × 0. 25°) long period (1901–2010) daily gridded rainfall data set over India and its comparison with existing data sets over the region. Mausam 65 (1), 1–18.
Peng, D., Zhou, T., Zhang, L., and Wu, B. (2018). Human contribution to the increasing summer precipitation in Central Asia from 1961 to 2013. J. Clim. 31, 8005–8021. doi:10.1175/JCLI-D-17-0843.1
Rana, S., McGregor, J., and Renwick, J. (2015). Precipitation seasonality over the Indian subcontinent: an evaluation of gauge, reanalyses, and satellite retrievals. J.Hydrometeorol. 16, 631–651. doi:10.1175/jhm-d-14-0106.1
Rienecker, M. M., Suarez, M. J., Gelaro, R., Todling, R., Bacmeister, J., Liu, E., et al. (2011). MERRA: NASA's Modern-Era Retrospective Analysis for Research and Applications. J. Clim. 24 (14), 3624–3648. doi:10.1175/JCLI-D-11-00015.1
Schiemann, R., Lüthi, D., Vidale, P. L., and Schär, C. (2008). The precipitation climate of Central Asia-intercomparison of observational and numerical data sources in a remote semiarid region. Int. J. Climatol. 28, 295–314. doi:10.1002/joc.1532
Schneider, U., Ziese, M., Meyer-Christoffer, A., Finger, P., Rustemeier, E., and Becker, A. (2016). The new portfolio of global precipitation data products of the Global Precipitation Climatology Centre suitable to assess and quantify the global water cycle and resources. Proc. IAHS 374, 29–34. doi:10.5194/piahs-374-29-2016
Serreze, M. C., and Hurst, C. M. (2000). Representation of mean Arctic precipitation from NCEP-NCAR and ERA reanalyses. J. Clim. 13, 182–201. doi:10.1175/1520-0442(2000)013<0182:romapf>2.0.co;2
Silva, V. B. S., Kousky, V. E., Shi, W., and Higgins, R. W. (2007). An improved gridded historical daily precipitation analysis for Brazil. J. Hydrometeorol 8, 847–861. doi:10.1175/jhm598.1
Song, S., and Bai, J. (2016). Increasing Winter Precipitation over Arid Central Asia under Global Warming. Atmosphere 7 (10), 139. doi:10.3390/atmos7100139
Sorooshian, S., Hsu, K.-L., Gao, X., Gupta, H. V., Imam, B., and Braithwaite, D. (2000). Evaluation of PERSIANN system satellite-based estimates of tropical rainfall. Bull. Amer. Meteorol. Soc. 81 (9), 2035–2046. doi:10.1175/1520-0477(2000)081<2035:eopsse>2.3.co;2
Sun, Q., Miao, C., Duan, Q., Ashouri, H., Sorooshian, S., and Hsu, K. L. (2018). A Review of Global Precipitation Data Sets: Data Sources, Estimation, and Intercomparisons. Rev. Geophys. 56, 79–107. doi:10.1002/2017RG000574
Tan, X., Ma, Z., He, K., Han, X., Ji, Q., and He, Y. (2020). Evaluations on gridded precipitation products spanning more than half a century over the Tibetan Plateau and its surroundings. J. Hydrol. 582, 124455. doi:10.1016/j.jhydrol.2019.124455
Tan, X., and Shao, D. (2017). Precipitation trends and teleconnections identified using quantile regressions over Xinjiang, China. Int. J. Climatol. 37, 1510–1525. doi:10.1002/joc.4794
Wei, W., Zhang, R., Wen, M., and Yang, S. (2017). Relationship between the Asian westerly jet stream and summer rainfall over Central Asia and north China: Roles of the Indian monsoon and the South Asian high. J. Clim. 30, 537–552. doi:10.1175/JCLI-D-15-0814.1
Worqlul, A. W., Collick, A. S., Tilahun, S. A., Langan, S., Rientjes, T. H. M., and Steenhuis, T. S. (2015). Comparing TRMM 3B42, CFSR and Ground-Based Rainfall Estimates as Input for Hydrological Models, in Data Scarce Regions: The Upper Blue Nile Basin, Ethiopia. Hydrological Earth Syst. Sci. Discuss 12, 2081–2112. doi:10.5194/hessd-12-2081-2015
Wu, Y., Guo, L., Zheng, H., Zhang, B., and Li, M. (2019). Hydroclimate assessment of gridded precipitation products for the Tibetan Plateau. Sci. Total Environ. 660, 1555–1564. doi:10.1016/j.scitotenv.2019.01.119
Xie, P., and Arkin, P. A. (1997). Global precipitation: a 17-year monthly analysis based on gauge observation, satellite estimates, and numerical model outputs. Bull. Amer. Meteorol. Soc. 78, 2539–2558. doi:10.1175/1520-0477(1997)078<2539:GPAYMA>2.0.CO;2
Yang, T., Li, Q., Chen, X., De, M. P., Yan, X., Liu, Y., et al. (2020). Spatiotemporal variability of the precipitation concentration and diversity in Central Asia. Atmos. Res. 241, 104954. doi:10.1016/j.atmosres.2020.104954
Yao, J., Chen, Y., Yu, X., Zhao, Y., Guan, X., and Yang, L. (2020). Evaluation of multiple gridded precipitation datasets for the arid region of northwestern China. Atmos. Res. 236, 104818. doi:10.1016/j.atmosres.2019.104818
Yao, J. Q., Mao, W. Y., Yang, Q., Xu, X. B., and Liu, Z. H. (2016). Annual actual evapotranspiration i n inland river catchments of China based on the Budyko framework. Stoch. Environ. Res. Risk Assess. 30, 1409–1421. doi:10.1007/s00477-016-1271-1
Yao, J. Q., Chen, Y. N., and Chen, J. (2020). Intensification of extreme precipitation in arid Central Asia. J. Hydrol., 125760. doi:10.1016/j.jhydrol.2020.125760
Yatagai, A., Arakawa, O., Kamiguchi, K., Kawamoto, H., Nodzu, M. I., and Hamada, A. (2009). A 44-year daily gridded precipitation dataset for Asia based on a dense network of rain gauges. Sola 5, 137–140. doi:10.2151/sola.2009-035
Yatagai, A., Kamiguchi, K., Arakawa, O., Hamada, A., Yasutomi, N., and Kitoh, A. (2012). APHRODITE: Constructing a Long-Term Daily Gridded Precipitation Dataset for Asia Based on a Dense Network of Rain Gauges. Bull. Am. Meteorol. Soc. 93 (9), 1401–1415. doi:10.1175/Bams-D-11-00122.1
Yu, Y., Chen, X., Disse, M., Cyffka, B., and Lei, J. (2020). Climate change in Central Asia: Sino-German cooperative research findings. Sci. Bull.. doi:10.1016/j.scib.2020.02.008
Zhang, M., Chen, Y., Shen, Y., and Li, Y. (2017). Changes of precipitation extremes in arid Central Asia. Quat. Int. 436, 16–27. doi:10.1016/j.quaint.2016.12.024
Zhang, Q., Singh, V. P., Li, J., Jiang, F., and Bai, Y. (2012). Spatio-temporal variations of precipitation extremes in Xinjiang, China. J. Hydrol. 434–435, 7–18. doi:10.1016/j.jhydrol.2012.02.038
Zhu, X., Wei, Z. G., Dong, W. J., Ji, Z. M., Wen, X. H., Zheng, Z. Y., et al. (2020). Dynamical downscaling simulation and projection for mean and extreme temperature and precipitation over central Asia. Clim. Dyn.. doi:10.1007/s00382-020-05170-0
Keywords: Central Asia, gauge-based gridded daily precipitation products, statistical assessments, spatial-temporal characteristics, climatic zones
Citation: Dilinuer T, Yao J, Chen J, Zhao Y, Mao W, Li J and Yang L (2021) Systematical Evaluation of Three Gridded Daily Precipitation Products Against Rain Gauge Observations Over Central Asia. Front. Earth Sci. 9:699628. doi: 10.3389/feart.2021.699628
Received: 23 April 2021; Accepted: 22 September 2021;
Published: 01 November 2021.
Edited by:
Diandong Ren, Curtin University, AustraliaReviewed by:
Weili Duan, Xinjiang Institute of Ecology and Geography (CAS), ChinaCopyright © 2021 Dilinuer, Yao, Chen, Zhao, Mao, Li and Yang. This is an open-access article distributed under the terms of the Creative Commons Attribution License (CC BY). The use, distribution or reproduction in other forums is permitted, provided the original author(s) and the copyright owner(s) are credited and that the original publication in this journal is cited, in accordance with accepted academic practice. No use, distribution or reproduction is permitted which does not comply with these terms.
*Correspondence: Junqiang Yao, eWFvanExOTg3QDEyNi5jb20=
Disclaimer: All claims expressed in this article are solely those of the authors and do not necessarily represent those of their affiliated organizations, or those of the publisher, the editors and the reviewers. Any product that may be evaluated in this article or claim that may be made by its manufacturer is not guaranteed or endorsed by the publisher.
Research integrity at Frontiers
Learn more about the work of our research integrity team to safeguard the quality of each article we publish.