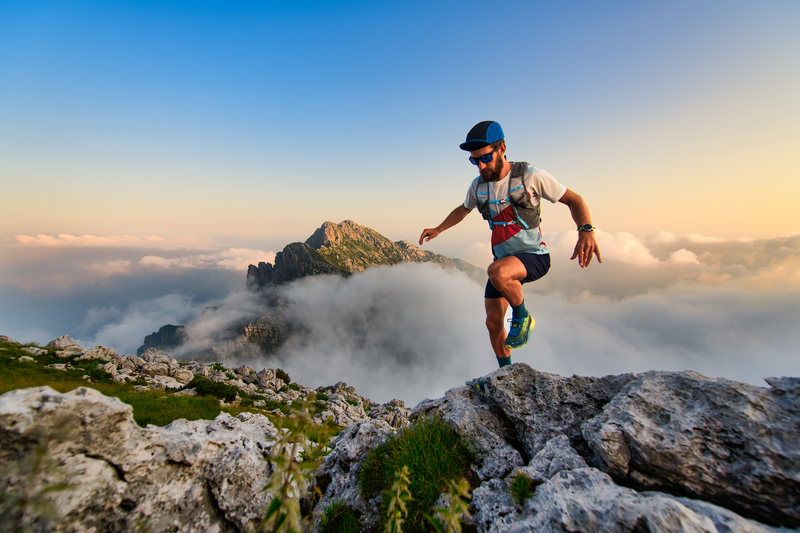
94% of researchers rate our articles as excellent or good
Learn more about the work of our research integrity team to safeguard the quality of each article we publish.
Find out more
ORIGINAL RESEARCH article
Front. Earth Sci. , 11 June 2021
Sec. Geohazards and Georisks
Volume 9 - 2021 | https://doi.org/10.3389/feart.2021.684421
This article is part of the Research Topic The New Frontiers of Earthquake Early Warning Systems View all 22 articles
The Geological Survey of Israel has upgraded and expanded the national Israeli Seismic Network (ISN), with more than 110 stations country-wide, as part of the implementation of a governmental decision to build a national Earthquake Early Warning (EEW) system named TRUAA. This upgraded seismic network exhibits a high station density and fast telemetry. The stations are distributed mainly along the main fault systems, the Dead Sea Transform, and the Carmel-Zfira Fault, which may potentially produce Mw 7.5 earthquakes. The system has recently entered a limited operational phase, allowing for initial performance estimation. Real-time performance during eight months of operation (41 earthquakes) matches expectations. Alert delays (interval between origin-time and Earthquake Early Warning alert time) are reduced to as low as 3 s, and source parameter errorstatistics are within expected values found in previous works using historical data playbacks. An evolutionary alert policy is implemented based on a magnitude threshold of Mw 4.2 and peak ground accelerations exceeding 2 cm/s2. A comparison between different ground motion prediction equations (GMPE) is presented for earthquakes from Israel and California using median ground motion prediction equations values. This analysis shows that a theoretical GMPE produced the best agreement with observed ground motions, with less bias and lower uncertainties. The performance of this GMPE was found to improve when an earthquake specific stress drop is implemented.
Earthquake Early Warning Systems (EEWS) are a tool to reduce earthquake risk. Their basic approach is to issue an alert as soon as possible following the occurrence of an earthquake, before damaging seismic waves arrive at a target. Some EEWS are based on identifying P-wave onsets or a preset acceleration threshold crossing, and to raise an alert that can be used to mitigate the effects of the expected ground shaking. Earthquake detection, by either single or multiple seismic or geodetic stations, can be done “on-site” close to the target or “regional” close to the source (Nakamura, 1988). Various algorithms are currently available—operational or under development around the world based on point-source, finite fault, and ground motion models (Allen and Melgar, 2019 and references therein).
Israel’s main seismic risk is attributed to the tectonically-active plate boundary fault system of the Dead Sea Transform (DST) and its branches (Figure 1) (e.g., Garfunkel et al., 1981), with earthquake magnitude potential of up to Mw7.5 (Hamiel et al., 2009). The increase in population density and in industrial and commercial infrastructures have amplified the seismic risk for Israel and led the Israeli government to appoint the Geological Survey of Israel (GSI) as responsible for establishing an EEWS, named TRUAA; TRUAA refers to the Hebrew word for the sounds of trumpet and of the traditional Jewish horn (Shofar), used for both, religious ceremonies and alerting (e.g. Joshua 6:20, Holy Bible: KJV).
FIGURE 1. Location map of TRUAA seismic stations network. Strong motion sensors are marked as yellow circles. Co-located broadbands are marked as red circles and GNSS receivers are marked as blue dots. Active faults (red lines) are after Sharon et al., 2020. DST—Dead Sea Transform, CF—Carmel Fault, Lod—Lod data center, Jer—Jerusalem data center.
The Israeli EEWS consists of three main components: 1) Seismic data acquisition system (Kurzon et al., 2020; 2) EEW Algorithm (Nof and Kurzon, 2021; 3) Dissemination system. While the former two are under the responsibility of the GSI, the latter is under the responsibility of the Home Front Command (HFC), of the Israeli Defense Force.
In Israel, several geographical and seismological considerations pose unique challenges for EEW. Since the country’s shape is narrow and sub-parallel to the main hazardous fault system (DST is oriented north-south), most of the population lives in proximity to potential earthquakes. Thus, the main challenge is the expected short response times, defined as the interval between earthquake alert issuing time and S-wave arrival time at a certain location. In addition, the DST coincides with the international border and the seismic network is deployed only on its western side. Therefore, the performance of the system suffers from limited azimuthal coverage, which results in reduced accuracy and increased uncertainties for automatic earthquake locations (Nof and Allen, 2016; Nof et al., 2019). Finally, EEW optimization and calibration using real time or historical playbacks, as commonly used elsewhere (Nof and Allen, 2016; Cochran et al., 2017; Meier et al., 2020), is challenged by the low seismicity rate (e.g., ∼ten times lower than California) and limited historical data.
Until 2017, the Israeli Seismic Network (ISN) consisted of 23 broadbands and short period sensors, collecting data in real-time at the Seismological Division data center formerly of the Geophysical Institute of Israel, located at the city of Lod (central Israel). Data acquisition and network geometry were not optimized for EEW, with high latencies (∼3 s) and large data packets (∼6 s), leading to poor performance in real-time (Nof and Allen, 2016).
TRUAA infrastructure follows one of the main principals set by the EEW international committee (Allen et al., 2012), requiring a state-of-the-art real-time seismic network, as the underlying foundation for efficient and reliable EEWS. Therefore, the ISN has gone through significant upgrades and modifications, since September 2017. In order to overcome the main challenge of short response times (see previous section), the network was designed to minimize alert delays, by densifying the station distribution (Kuyuk and Allen, 2013b) along the main seismic sources [DST and the Carmel-Zfira Fault System (CF)], and significantly reducing telemetry latencies. The resulting seismic network currently has 110 working stations, out of 121 planned stations, with below 1s data packets, and station-intervals of 5–10 km along the major faults (Figure 1). All stations are equipped with strong motion Titan accelerometers, and Centaur dataloggers, sampling in 200 samples per second; some (25 stations) have collocated broadband Trillium 120 s seismometers; seismic instrumentation is provided by Nanometrics. A full overview on TRUAA infrastructure is given by Kurzon et al. (2020). In order to increase reliability and robustness of the EEWS, several layers of redundancy were implemented at all network scales: 1) each station has two telemetry routes, the main and fast route, by ethernet or cellular, with latencies of 600–900 ms, and the backup route, by cellular or satellite, with latencies of 700–1500 ms; 2) there are two data centers synchronized and working in parallel using an active-active cluster configuration, the main one in Jerusalem and the backup in Lod; 3) Each data center hosts two parallel acquisition and processing chains (hence, a total of four independent chains).
The acquisition system is based on Nanometrics’ Apollo Servers which forward the data for both routine seismic monitoring procedures and the EEWS at each data center. Each data packet is multi-casted separately via the two routes and reaches each of the four separate acquisition systems. Since Jerusalem main data center is located ∼20 km from the DST, this redundant configuration of four independent EEWS is expected to provide high robustness in case of a catastrophic event.
The collected seismic data is processed using the United States. West Coast ShakeAlert’s Earthquake Point-source Integrated Code (EPIC) EEW algorithm (Given et al., 2018; Kohler et al., 2020), providing rapid alerts for potentially damaging earthquakes. The EPIC algorithm, formerly under the codename ElarmS (e.g. Chung et al., 2019), is a regional (network based) point source algorithm. In order to issue an alert, the algorithm requires P-wave detections by a minimum of four different stations and at least 40% of active stations in the P-phase wave-front area. The earthquake location is obtained by a 200 × 200 km grid search, in which the residuals of the calculated and observed arrival-times are minimized based on a one-dimensional layered global velocity model, AK135 (Kennett et al., 1995). Then, event magnitude is estimated from the hypocenteral distances combined with the peak displacement (Pd) values, calculated from accelerations or velocities measured at the different stations (Kuyuk and Allen, 2013a; Sadeh et al., 2014). The event’s magnitude estimate used is the average of the estimates at all near-source stations and an uncertainty on this value is also computed but not used for alerting purposes. As time progress and additional data become available, the origin-time, location, Pd values and magnitude are all updated with higher accuracy (e.g. Kohler et al., 2020). Modifications and developments of ShakeAlert are carefully examined and implemented after adjustments to the local seismic network and conditions (Nof and Allen, 2016; Nof and Kurzon, 2021). The TRUAA EEWS consists of TRUAA’s acquisition system, EPIC’s Waveform Processor (WP), and Event Associator (EA) that identifies events and sends alerts to a Decision Module (DM) that forwards alerts based on a predefined magnitude and geographic location. ActiveMQ messaging system (Kohler et al., 2018; Chung et al., 2019) is used for communication between the modules. In our implementation, EPIC EA alert messages, limited to M > 2.5, are shared between all four DM instances and each DM finally converges the EA alerts to produce a single EEW alert with updating intervals of 1 s. The HFC listens to the DM alerts and is responsible for disseminating proper alerts to the public. Although the HFC alert dissemination system is actively working for various threats, currently, earthquake alerts are distributed only to a limited test group via a cellular application. In addition to the HFC alerting channels, a test group receives alerts via a dedicated GSI Telegram bot.
The deployment phase of TRUAA, ongoing since September 2017, provided the opportunity to test the EEW algorithm in real-time. As data centers were being configured and additional stations deployed, telemetry and stations have shown variability in performance and availability, with occasional data outage in all or in parts of the network, and variable latencies or completeness levels. Nevertheless, the available data was sufficient to examine the performance of ElarmS, and carefully upgrade the EEW into EPIC (Nof and Kurzon, 2021). In this work, we attempt to assess the expected performance of the EEWS in terms of alert-delays and source parameter accuracy (location, origin-time and magnitude) by analyzing statistics of the available data between May 2020 and January 2021. Although most of the stations were already deployed during this period, we note that not all stations were available to the system at all times and occasional outages occurred. We use data from a real-time testing system utilizing a single processing chain (e.g. WP and EA) which has the most complete real-time data record and its performances are similar for the four operational processing chains.
The expected performance, in terms of alert delays, were estimated as the minimal alert delay for earthquakes occurring in and around Israel, i.e., estimating the theoretical time for EPIC to provide EEW to the HFC dissemination system, given an earthquake location and seismic network geometry (Nof and Kurzon, 2021). This analysis presented by Nof and Kurzon (2021; their Figure 3) shows that the minimal delay time is 4.7 s, where the seismic network is most dense. This estimation assumes an earthquake depth of 10 km and processing and telemetry times of 1 s, each. Typically, latency and processing times are less than 1 s. Longer telemetry delays as well as deeper hypocenters would result in longer alert delays. Alert delays are expected to be ∼5 s for events occurring along the main hazardous fault lines. Assuming a very conservative S-wave velocity of 3.5 km/s and depth 10 km this delay would translate into a ∼15 km blind-zone (∼22 km with added 2 s for alert dissemination), wherein S-wave would precede alert issuance. This is a significant improvement relative to previous blind-zone estimation of over 30 km (Pinsky, 2015). Slower S-wave velocity would yield smaller blind zone while shallower events might yield larger blind zone.
Since it’s installation on May 5th, 2020, EPIC version 3.2.1-2020-04-17 was running in real-time. Until January 1st, 2021, EPIC EA has identified and alerted on 154 events of M > 2.5 (EPIC magnitude estimation). In order to assess the EEW algorithm performance, the GSI catalog (earthquake.co.il last access 2021-03-01) is considered as a reference and a careful association is required between the two datasets. We first use large area catalog events to associate EPIC events and then process only those associated with catalog events located in a more local area that corresponds to the TRUAA coverage.
For this period, the GSI catalog includes a total of 2,674 events (525 with Mw > 3) located between 27–36 latitudes and 32–38 longitudes with maximal magnitude of Mw 6.7 (the 2020-10-30 11:51 Aegean Sea earthquake). While the catalog’s geographic coverage is larger than the expected EEW coverage (limited to ∼200 km from the seismic network stations) distant events may trigger an alert with large location errors and therefore should be considered. We associate the GSI catalog records with EPIC’s EA alerts based on a time window of 130 s to allow association of distant events. For the 2674 GSI catalog events only six are categorized as “Felt” (e.g. were reported by the public to be felt) and 1,610 events are categorized as quarry blasts or explosions (all with Mw < 3, some without recorded catalog magnitude).
Out of the 154 EPIC alerts, two were not associated with any catalog event (False alerts) and one was associated with an un-cataloged teleseismic event (2020-10-06 Mw 5.9 Fiji). The EPIC first magnitude estimation for these three events was in the range of 2.6—3.1. For the six felt events that occurred during this period, five were identified and alerted by EPIC and one missed - the Mw 5.3 2020-12-05 Near Antalya, Turkey. This missed felt event was a distant event (>450 km from ISN) and as such should not be considered, though it was widely felt in Israel (21 reports at the USGS “did you feel it” with MMI III).
As previously mentioned, TRUAA is yet to be fully operational and data acquisition in real-time suffer from different challenges. We use the EPIC alerts database to investigate the system performance in terms of alert delay times, magnitude errors and locations errors. These parameters are of high importance for decision makers in establishing the appropriate public alerting approach in Israel. Previous analysis of historical playbacks have found median and standard deviation (σ) of 3.7 km (σ 32.26 km) and 0.8 s (σ 4.33 s) for the location errors and time errors, respectively, and mean magnitude error of 0.41 (σ 0.43) for the population of all 49 felt events (2.8 < Mw < 4.8) during January 2012 to March 2020 (Nof and Kurzon, 2021). In order to compare these findings with current real-time performance we use all 41 catalog events (2.1 < Mw < 3.7) that occurred at the same spatial reference of 29o–35o Latitudes and 32.5o–36.5o Longitudes and their first EPIC EA alerts. Figure 2 summarizes these statistics with a mean magnitude error of 0.38 (σ 0.28), median location error of 5.08 km (σ 17.42 km) and median time errors of 0.8 s (σ 5 s). These obtained statistical values are similar to those previously found using historical events playbacks (Nof and Kurzon, 2021). The alert-delays (Figure 2D) show good fit with the expected alert delays, detailed in Expected Alert Delays in Israel section, especially for the stronger events with catalog magnitude of Mw ≥ 3. All four felt events in the analyzed subset are within ±1 s of the expected alert-delays. Significant deviations, with larger than the expected alert-delays can be explained by small events (Mw < 3) that require additional time to fulfill the required magnitude threshold of M ≥ 2.5 in order to trigger an alert by the algorithm or by distant location from the seismic network. Although the datasets are limited in numbers and magnitude range, the EEWS performance within reported felt events is in accordance with expectations in terms of alert delays (see Expected Alert Delays in Israe Section) and in terms of location and magnitude errors based on historical data estimations (Nof and Kurzon, 2021). The results show that the EEW algorithm performance in real-time is as expected and that decision makers may rely on these findings to determine the alert approach for Israel.
FIGURE 2. EPIC real-time performance evaluation. (A) magnitude errors; (B) location errors; (C) origin time errors; (D) measured alert delays vs. expected alert delays (Nof and Kurzon, 2021; See theirFigure 3for expected alert delays spatial distribution). Dots denote events with catalog magnitude of Mw < 3, gray circles denote events with catalog magnitude Mw > 3, red circles represent felt events, dark dashed line represents a 1:1 fit between expected and measured alert delay, gray dashed lines represent ±1 s from the 1:1 fit line; All errors are referenced to the ISN catalog.
The initial earthquake alert approach for Israel was to alert educational institutions country-wide in case of a Mw ≥ 5.5 earthquake. Nof and Kurzon (2021) discussed the potential limitations of TRUAA and proposed an alternative approach for Israel. This alternative approach adopted herein. The main challenge is the potential earthquake magnitude under or over-estimation, which may respectively lead to damage (structural, personal physical or psychological) when alert should have been issued or unnecessary disturbance when alert was not required.
Given the inherent EPIC point source algorithm limitations and the need to balance between the short time for disseminating useful alerts and reducing needless alerts (e.g., alerting when damage is not expected), the proposed alerting approach is now approved by the decision makers and will allow for an “evolutionary” alert to both educational institutions and the general public. Currently, it is not scheduled to deliver EEW to sensitive infrastructures due to the current high uncertainty of the solutions and the sensitivity of infrastructures to unrequired alerts. In the following, we present the current EEW policy in Israel and the considerations for this approach.
Following the recommendations of Nof and Kurzon (2021) for an “evolutionary” alert (Figure 3), similar to the USGS ShakeAlert’s approach (Given et al., 2018), alerts for magnitudes in the range of 4.2 ≤ Mw < 6.0 will be limited to areas where the ground shaking is expected to be above 2 cm/s2 (pink zone in Figure 3), which is well felt (MMI II-III), but should not cause any significant structural damage (Worden et al., 2012). For events with magnitude Mw ≥ 6—A country-wide alert will be assigned.
FIGURE 3. “Evolutionary” EEW alert. With time, magnitude estimation is updated and the alerted area (pink) increases. Population numbers represent units of 1,000 per alert area. In a different scenario, where magnitude estimation would not increase (e.g. magnitude is stable at Mw 4), the alerted area is minimal and other areas are not disturbed. Alert area limit is calculated as 2 cm/s2 using Lior and Ziv (2018) GMPE around the epicenter (red mark). Blue and red circles indicate the front of the P- and S- waves, respectively. Black and gray circles are 10 s intervals for S-wave front e.g. the expected S-wave front within 10 and 20 s, respectively.
The described approach is aimed to balance the need to alert as soon as possible for potentially damaging events and the need to avoid alerting for regions where no action is required (Cochran and Husker, 2019; Le Guenan et al., 2016; Minson et al., 2018). While public demand and expectations in some places are to receive alerts even when no physical damage is expected (MMI > III), such as following the 2019 Ridgecrest earthquake in California (Cochran and Husker, 2019), in other places alerts are expected only for more damaging events, such as in New Zealand (Becker et al., 2020). Common public alert approaches are aimed at higher intensities such as the JMA L5 in Japan (Hoshiba et al., 2008) and the initial alert threshold of MMI IV in California (Given et al., 2018). The relatively low threshold of 2 cm/s2, balanced with a low magnitude threshold of Mw 4.2, allows to account for local amplifications and the uncertainties in magnitude, location and ground motion prediction equations (GMPEs) (Minson et al., 2019). The apparent public acceptance and tolerance for receiving alerts even where no damage occurred (Allen, 2017; Allen et al., 2018) allows the use of such a low threshold to ensure alerts are sent only to areas that are expected to feel shaking (Worden et al., 2012) and reduce disturbance to the public where no action is required. The Mw 4.2 threshold mitigates the risk of delaying alerts for initially underestimated large earthquakes (Böse et al., 2012; Meier, 2017; Melgar and Hayes, 2017; Minson et al., 2018; Meier et al., 2020) while avoiding unrequired alerts to non-damaging lower magnitude events. Since for large magnitude earthquakes additional time might be required in order to converge to its maximal peak displacement (Trugman et al., 2019), hence, as additional information is gathered and becomes available for the EEWS, magnitude estimation is expected to be more accurate and corresponding alert area would increase. Initiating the alert to a limited area at an early stage reduces the “blind zone” where alerts are received after S-wave arrivals. In addition to the lower-bound magnitude threshold, a second threshold was selected at Mw six to spread an alert country-wide. Since most of the country is likely to be included in the 2 cm/s2 zone, extending the alert to the whole country mitigates the point-source algorithm potential saturation at magnitudes larger than Mw six (e.g. Brown et al., 2009).
Currently, disseminating alerts is the responsibility of the HFC. EPIC’s DM point source solutions are acquired from the ActiveMQ messaging system as XML format by a dedicated new module and translated into Common Alerting Protocol (CAP) format (Jones et al., 2010). A pre-calculated magnitude-distance table is used to set the alert radius around the epicenter, based on the selected thresholds and GMPEs. The EPIC alert is forwarded to the HFC disseminating system in less than 0.5 s (∼400 milliseconds). HFC can forward the alerts to the public using various methods (cellular application, emergency sirens, TV, radio etc.) which adds an additional 1–10 s depending on the alert medium. Currently, a test group of subscribers to the HFC alert applications can receive alerts. The subscriber can register to any location (city/settlement) and receive simple informative push messages in case of an alert to these locations. In addition, a full alert panel will appear in case of an alert to the device’s current location. The alert panel (Figure 4) includes red colors, earthquake icon, user location name, date, time and type of threat (earthquake in this case). A unique alerting sound and additional instructions are also available in Hebrew, English, Arabic and Russian. Instructions include information on how to react at different places (indoors, outdoors, cars, coastlines). Emergency sirens are currently designed to sound verbally the word “Earthquake” in Hebrew to distinguish from other threats. The system is yet to be operational on a national level.
FIGURE 4. Alert panel screenshot taken from the HCF app. Red frame surrounding dedicated earthquake icon with the name of user place, action instructions, date, time and type of threat (earthquake in this case). A unique alerting sound and additional instructions are also available. Instructions include information on how to react at different places (indoors, outdoors, cars, coastline).
Following real-time magnitude and location determination—alert issuance and alert region are determined based on GMPEs. GMPE development requires large earthquake dataset with a wide magnitude range. The low seismicity rate in the region limits the development of rigorous local GMPEs, thus, several global GMPEs are tested to determine the optimal one for TRUAA. The appropriate GMPEs should meet the following criteria: 1) regionally adjustable; 2) Based and validated on a large global dataset with 3 ≤ Mw ≤ 7; and 3) Yields low standard deviations between observed and predicted median values of ground motions, in particular when tested against regionally recorded earthquakes. The latter is crucial for EEW, due to the limited information available in real-time (Magnitude and epicentral distance). In this section, the performance of three different GMPEs is compared: the model-based GMPE of Lior and Ziv (2018, LZ18), the empirical GMPE of Abrahamson, Silva and Kamai (2014, ASK14) and the empirical GMPE of Cua et al., 2009, CH09. The LZ18 GMPE is formulated as follows (Eq. 20c in Lior and Ziv, 2018):
where PGA is peak ground accelerations,
where PGA is measured in cm, M is magnitude,
For ASK14, we use the basic form of the GMPE since many coefficients are unknown for Israel. This formulation is presented in Eqs 2–4 of Abrahamson, Silva and Kamai (2014):
Where
RRUP is the closest distance to the rupture plane, equivalent to the epicentral distance for small earthquakes. The following coefficient values were used: c4 = 4.5, M1 = 6.75, M2 = 5, a1 = 0.587, a2 = −0.79, a3 = 0.275, a4 = −0.1, a5 = −0.41, a6 = 2.154, a7 = 0.0, a8 = -0.015 and a17 = −0.0072 (Abrahamson et al., 2014). Both CH09 and ASK14 describe the median PGA while LZ18 describes a theoretical PGA, based on commonly used seismological models (Brune, 1970; Anderson and Hough, 1984). The validation of LZ18 to a diverse data set of earthquakes, mainly from Japan and California, revealed low uncertainties of 0.7 in natural log units (0.3 is 10 base log units) (Lior and Ziv, 2018), comparable to 0.31 determined for CH09 (10 base log units) (Cua and Heaton, 2009). For ASK14, standard deviation are not reported specifically for the base form of the GMPE used here.
All GMPEs meet criteria 2) so they will be appraised based on criteria 1) and 3). The tested GMPEs are compared using locally recorded earthquakes, as well as the 2019 Mw 6.4 and Mw 7.1 Ridgecrest earthquakes to compensate for the lack of large magnitude events in our local seismic record. In contrast to the empirical GMPEs (ASK14 and CH09), which are a function of magnitude and epicentral distance (under the EEW algorithm point source assumption), the LZ18 GMPEs also depend on stress drop. Since stress drop is currently not resolved in real-time, it will be estimated using the magnitude—stress drop relation established for California earthquakes by Lior and Ziv (2018; their Eq. 18a):
This relation, determined for earthquakes of similar mechanism (mostly strike-slip), provides a good fit to observed ground motions, as further shown.
To examine the performance of the different GMPEs, they are compared to recorded ground motions. Predicted PGA are calculated using the different GMPEs and catalog magnitudes and source-to-site distances. We focus on the median predictions of the GMPEs, neglecting their uncertainties, since the EEW alerts are binary—either alert or not and these GMPEs uncertainties cannot be considered in real-time. Thus, we do not perform a complete evaluation of each GMPE. Figure 5 shows the discrepancies between observed and predicted PGA (using different GMPEs) as functions of magnitude and distance, and Figure 6 shows observed PGA as a function of distance, along with a fit to the different GMPEs, for four different earthquakes in Israel and California. In Figure 5, only PGA >1 cm/s2 are considered, since lower values are of little interest for EEW. Figure 5 reveals that the residuals are invariant to magnitude and distance for all GMPEs. The average discrepancies and the standard deviations of the residuals (µ and σ, respectively, in the legends of Figure 5A and Figure 6) indicate that LZ18 produces a better fit to the observations compared with the two empirical GMPEs, showing a lower standard deviation, and a more symmetric residual distribution (µ closer to 0). The negative average discrepancies, typically observed for CH09 and ASK14, indicate a general ground motions underestimation. Such underestimation has been previously reported by Nof and Allen (2016) who implemented a simple globally derived GMPE for real-time magnitude estimation of Israeli earthquakes. Thus, we conclude that LZ18 is the more suitable GMPE, producing lower ground motion uncertainties, as required by criteria 3) defined earlier.
FIGURE 5. The logarithm of predicted PGA (PGAGMPE) minus the logarithm of observed PGA (PGAOBS) as functions of (A) catalog moment magnitude and (B) source-to-site distance. Discrepancies calculated using CH09, ASK14 and LZ18 are indicated by blue circle, black line and red dots, respectively. Average discrepancies (µ) and standard deviations to the residuals (σ) between predicted and observed PGAs are indicated in the legend.
FIGURE 6. PGA as a function of distance for (A) MW 3.9 in the eastern Mediterranean Sea, (B) MW 4.4 in north Israel, and (C) MW7.1 and (D) MW6.4 near Ridgecrest California. Magnitudes and the number of data points are indicated at the bottom left corner of each panel. The GMPEs of LZ18, ASK14, CH09, and LZ18 with earthquake specific stress drop (LZ18Δτ) are indicated by red, black, blue and green curves, respectively. Average discrepancies (μ) and standard deviations to the residuals (σ) are indicated in the legends.
Since LZ18 explicitly includes the stress drop, we test whether implementing an earthquake specific
The underestimation of PGA using CH09 and ASK14 (Figures 5, 6) typically results in smaller alert areas compared to those imposed by LZ18. To demonstrate this effect, Figure 7 shows magnitude as a function of the predicted alert area radius for the different GMPEs. Here, alert radius corresponds to the predefined threshold of peak ground accelerations >2 cm/s2. Similar alert radii are predicted for small magnitudes (M<∼4), while at larger magnitudes, the LZ18 GMPE is the most conservative, producing the largest alert areas. The latter characteristic is particularly appealing for the early stages of TRUAA’s operation, where public expectation and long-term performance are still unclear. We conclude that LZ18 will produce the most reliable and robust ground shaking predictions, which may be improved if a real-time stress drop estimate is available.
FIGURE 7. Magnitude as a function of alert radius for a PGA threshold of 2 cm/s2 (MMI II-III) using LZ18 (black solid line), ASK14 (black dotted line) and CH09 (gray dashed line).
The State of Israel’s TRUAA EEWS, along with its new real-time seismic network, achieve state-of-the-art real-time capabilities owing to the: 1) fast telemetry, 2) high station density along the main fault systems, and 3) local implementation of the EPIC algorithm. In this manuscript we demonstrated that the real-time performance of the EPIC EEW algorithm is in-line with the expected alert-delays and source parameters error uncertainty. We evaluated the real-time performance of TRUAA between May 5th, 2020 and January 1st, 2021 using 41 events with catalog magnitude range of (2.1 < Mw < 3.7) and found that all felt events within the seismic network coverage led to accurate and timely alerts. We found mean magnitude error of 0.38 (σ 0.28), median location error of 5.08 km (σ 17.42 km) and median time errors of 0.8 s (σ 5 s). These statistics are robust and similar to previously reported values (Kurzon et al., 2020; Nof and Kurzon, 2021). The suggested “evolutionary” alert has been approved and alerts to limited areas will be provided for 4.2 ≥ Mw < 6. For Mw ≥ 6 a country-wide alert will be issued. We note that the dataset used in this research lacks large magnitude events and the seismic network is yet to be fully completed. Thus, the performance statistics may change in the future and further assessments would be required.
This alerting approach is expected to balance the need for rapid alerts in the presence of high uncertainties, on the one hand, and the desire to reduce alerts for areas with low ground motions, on the other hand. This balance is achieved by setting a lower alert magnitude threshold, and alerting to a radial distance from the epicenter, where ground accelerations are predicted to be higher than 2 cm/s2. This threshold may be adjusted in the future, considering TRUAA’s performance and public expectations, to provide more accurate and targeted alerts. A comparison between three different GMPEs concluded that the model-based GMPE of Lior and Ziv (2018) produces the better agreements between predicted median values and observed PGA. In addition, this GMPE has the potential to be further adjusted by implementing real-time stress-drop, a parameter that can be reliably determined in real-time. Thus, the limited alert area is determined using this GMPE.
A complementary essential component to EEWS is public outreach, education and exercise of earthquake response (Allen et al., 2009 and references therein; Santos-Reyes, 2019; Wald, 2020). These are critical for a successful EEWS, especially in areas with low seismic activity, where public awareness is low, such as in Israel, and should be addressed in the near future, as TRUAA becomes fully operational.
The raw data supporting the conclusion of this article will be made available by the authors, without undue reservation.
RN and IK contributed to conception and design of the study. IK performed the seismic network analysis. RN performed the EPIC performance analysis. IL performed the GMPEs analysis. RN wrote the first draft of the manuscript. IK and IL wrote sections of the manuscript. All authors contributed to manuscript revision, read, and approved the submitted version.
The authors declare that the research was conducted in the absence of any commercial or financial relationships that could be construed as a potential conflict of interest.
We thank the GSI EEW workgroup and the National Steering Committee for Earthquake Preparedness for fruitful discussions on alerting strategy for Israel. We thank the U.C Berkeley Seismology Lab for making EPIC updates available for us. Figures were generated using Python’s modules Matplotlib (https://matplotlib.org/, last accessed Mar 1, 2021) and map using Python’s module Folium (https://python-visualization.github.io/folium/, last accessed Mar 1, 2021). Background map in Figure 2 obtained from ESRI (http://server.arcgisonline.com/ArcGIS/rest/services, last accessed Mar 1, 2021). Coastlines in Figure 1 obtained from Global Self-consistent Hierarchical High-resolution Geography (https://www.soest.hawaii.edu/pwessel/gshhg/, last update June 14, 2017, last accessed Mar 1, 2021). Population density data obtained from WorldPop (http://www.worldpop.org, last accessed June 25, 2020). Finally, We thank the editor and two anonymous reviewers who helped make this manuscript clearer and more accurate.
Abrahamson, N. A., Silva, W. J., and Kamai, R. (2014). Summary of the ASK14 Ground Motion Relation for Active Crustal Regions. Earthquake Spectra 30, 1025–1055. doi:10.1193/070913EQS198M
Allen, R., Cochran, E., Huggins, T., Miles, S., and Otegui, D. (2018). Lessons from Mexico's Earthquake Early Warning System. Lessons from Mexico’s Earthquake Early Warning System, 99. Washington. DC: Eos. doi:10.1029/2018eo105095
Allen, R. M., Baer, G., Clinton, J., Hamiel, Y., Hofstetter, R., Pinsky, V., et al. (2012). Earthquake Early Warning for Israel: Recommended Implementation Strategy. Jerusalem, Israel: Geological Survey of Israel.
Allen, R. M., and Melgar, D. (2019). Earthquake Early Warning: Advances, Scientific Challenges, and Societal Needs. Annu. Rev. Earth Planet. Sci. 47, 361–388. doi:10.1146/annurev-earth-053018-060457
Allen, R. M. (2017). Quake Warnings, Seismic Culture. Science 358, 1111. doi:10.1126/science.aar4640
Anderson, J. G., and Hough, S. E. (1984). A Model for the Shape of the Fourier Amplitude Spectrum of Acceleration at High Frequencies. Bull. Seismol. Soc. Am. 74, 1969–1993.
Becker, J. S., Potter, S. H., Vinnell, L. J., Nakayachi, K., McBride, S. K., and Johnston, D. M. (2020). Earthquake Early Warning in Aotearoa New Zealand: a Survey of Public Perspectives to Guide Warning System Development. Humanit. Soc. Sci. Commun. 7, 138. doi:10.1057/s41599-020-00613-9
Böse, M., Heaton, T. H., and Hauksson, E. (2012). Real-time Finite Fault Rupture Detector (FinDer) for Large Earthquakes. Geophys. J. Int. 191, 803–812. doi:10.1111/j.1365-246X.2012.05657.x
Brown, H. M., Allen, R. M., and Grasso, V. F. (2009). Testing ElarmS in Japan. Seismological Res. Lett. 80, 727–739. doi:10.1785/gssrl.80.5.727
Brune, J. N. (1970). Tectonic Stress and the Spectra of Seismic Shear Waves from Earthquakes. J. Geophys. Res. 75, 4997–5009. doi:10.1029/JB075i026p04997
Chung, A. I., Henson, I., and Allen, R. M. (2019). Optimizing Earthquake Early Warning Performance: ElarmS‐3. Seismol. Res. Lett. 90, 727–743. doi:10.1785/0220180192
Cochran, E. S., and Husker, A. L. (2019). How Low Should We Go when Warning for Earthquakes? Science 366, 957–958. doi:10.1126/science.aaz6601
Cochran, E. S., Kohler, M. D., Given, D. D., Guiwits, S., Andrews, J., Meier, M. A., et al. (2017). Earthquake Early Warning ShakeAlert System: Testing and Certification Platform. Seismol. Res. Lett. 89, 108–117. doi:10.1785/0220170138
Cua, G., Fischer, M., Heaton, T., and Wiemer, S. (2009). Real-time Performance of the Virtual Seismologist Earthquake Early Warning Algorithm in Southern California. Seismological Res. Lett. 80, 740–747. doi:10.1785/gssrl.80.5.740
Cua, G., and Heaton, T. H. (2009). Characterizing Average Properties of Southern California Ground Motion Amplitudes and Envelopes. Available at: https://resolver.caltech.edu/CaltechEERL:EERL-2009-05.
Garfunkel, Z., Zak, I., and Freund, R. (1981). Active Faulting in the Dead Sea Rift. Tectonophysics 80, 1–26. doi:10.1016/0040-1951(81)90139-6
Given, D. D., Allen, R. M., Baltay, A. S., Bodin, P., Cochran, E. S., Creager, K., et al. (2018). Revised Technical Implementation Plan for the ShakeAlert System-An Earthquake Early Warning System for the West Coast of the United States. Revised Technical Implementation Plan for the ShakeAlert System—An Earthquake Early Warning System for the West Coast of the United States. Reston, VA: USGS. doi:10.3133/ofr20181155
Hamiel, Y., Amit, R., Begin, Z. B., Marco, S., Katz, O., Salamon, A., et al. (2009). The Seismicity along the Dead Sea Fault during the Last 60,000 Years. Bull. Seismological Soc. America 99, 2020–2026. doi:10.1785/0120080218
Hoshiba, M., Kamigaichi, O., Saito, M., Tsukada, S. y., and Hamada, N. (2008). Earthquake Early Warning Starts Nationwide in Japan. Eos Trans. AGU 89, 73–74. doi:10.1029/2008EO080001
Jones, E., Systems, W., and Westfall, J. (2010). Common Alerting Protocol Version 1.2. 1–47. Available at: http://docs.oasis-open.org/emergency/cap/v1.2/CAP-v1.2.pdf.
Kennett, B. L. N., Engdahl, E. R., and Buland, R. (1995). Constraints on Seismic Velocities in the Earth from Traveltimes. Geophys. J. Int. 122, 108–124. doi:10.1111/j.1365-246X.1995.tb03540.x
Kohler, M. D., Cochran, E. S., Given, D., Guiwits, S., Neuhauser, D., Henson, I., et al. (2018). Earthquake Early Warning ShakeAlert System: West Coast Wide Production Prototype. Seismol. Res. Lett. 89, 99–107. doi:10.1785/0220170140
Kohler, M. D., Smith, D. E., Andrews, J., Chung, A. I., Hartog, R., Henson, I., et al. (2020). Earthquake Early Warning ShakeAlert 2.0: Public Rollout. Seismol. Res. Lett. 91, 1763–1775. doi:10.1785/0220190245
Kurzon, I., Nof, R. N., Laporte, M., Lutzky, H., Polozov, A., Zakosky, D., et al. (2020). The "TRUAA" Seismic Network: Upgrading the Israel Seismic Network-Toward National Earthquake Early Warning System. Seismol. Res. Lett. 91, 3236–3255. doi:10.1785/0220200169
Kuyuk, H. S., and Allen, R. M. (2013a). A Global Approach to Provide Magnitude Estimates for Earthquake Early Warning Alerts. Geophys. Res. Lett. 40, 6329–6333. doi:10.1002/2013GL058580
Kuyuk, H. S., and Allen, R. M. (2013b). Optimal Seismic Network Density for Earthquake Early Warning: A Case Study from California. Seismological Res. Lett. 84, 946–954. doi:10.1785/0220130043
Le Guenan, T., Smai, F., Loschetter, A., Auclair, S., Monfort, D., Taillefer, N., et al. (2016). Accounting for End-User Preferences in Earthquake Early Warning Systems. Bull. Earthquake Eng. 14, 297–319. doi:10.1007/s10518-015-9802-6
Lior, I., and Ziv, A. (2020). Generic Source Parameter Determination and Ground-Motion Prediction for Earthquake Early Warning. 110, 345–356. doi:10.1785/0120190140
Lior, I., and Ziv, A. (2018). The Relation between Ground Motion, Earthquake Source Parameters, and Attenuation: Implications for Source Parameter Inversion and Ground Motion Prediction Equations. J. Geophys. Res. Solid Earth 123, 5886–5901. doi:10.1029/2018JB015504
Madariaga, R. (1976). Dynamics of an Expanding Circular Fault. Bull. Seismol. Soc. Am. 66, 639–666. Available at: http://bssa.geoscienceworld.org/content/66/3/639.abstract.
Meier, M.-A. (2017). How "good" Are Real-Time Ground Motion Predictions from Earthquake Early Warning Systems? J. Geophys. Res. Solid Earth 122, 5561–5577. doi:10.1002/2017JB014025
Meier, M. A., Kodera, Y., Böse, M., Chung, A., Hoshiba, M., Cochran, E., et al. (2020). How Often Can Earthquake Early Warning Systems Alert Sites with High‐Intensity Ground Motion? J. Geophys. Res. Solid Earth 125, 2019JB017718. doi:10.1029/2019JB017718
Melgar, D., and Hayes, G. P. (2017). Systematic Observations of the Slip Pulse Properties of Large Earthquake Ruptures. Geophys. Res. Lett. 44, 9691–9698. doi:10.1002/2017GL074916
Minson, S. E., Baltay, A. S., Cochran, E. S., Hanks, T. C., Page, M. T., McBride, S. K., et al. (2019). The Limits of Earthquake Early Warning Accuracy and Best Alerting Strategy. Sci. Rep. 9, 1–13. doi:10.1038/s41598-019-39384-y
Minson, S. E., Meier, M.-A., Baltay, A. S., Hanks, T. C., and Cochran, E. S. (2018). The Limits of Earthquake Early Warning: Timeliness of Ground Motion Estimates. Sci. Adv. 4, eaaq0504. doi:10.1126/sciadv.aaq0504
Nof, R. N., and Allen, R. M. (2016). Implementing the ElarmS Earthquake Early Warning Algorithm on the Israeli Seismic Network. Bull. Seismological Soc. America 106, 2332–2344. doi:10.1785/0120160010
Nof, R. N., Chung, A. I., Rademacher, H., Dengler, L., and Allen, R. M. (2019). MEMS Accelerometer Mini-Array (MAMA): A Low-Cost Implementation for Earthquake Early Warning Enhancement. Earthquake Spectra 35, 21–38. doi:10.1193/021218EQS036M
Nof, R. N., and Kurzon, I. (2021). TRUAA-earthquake Early Warning System for Israel: Implementation and Current Status. Seismol. Res. Lett. 92, 325–341. doi:10.1785/0220200176
Pinsky, V. (2015). Modeling Warning Times for the Israel's Earthquake Early Warning System. J. Seismol. 19, 121–139. doi:10.1007/s10950-014-9454-z
Sadeh, M., Ziv, A., and Wust-Bloch, H. (2014). Real-time Magnitude Proxies for Earthquake Early Warning in Israel. Geophys. J. Int. 196, 939–950. doi:10.1093/gji/ggt407
Sharon, M., Sagy, A., Kurzon, I., Marco, S., and Rosensaft, M. (2020). Assessment of seismic sources and capable faults through hierarchic tectonic criteria: implications for seismic hazard in the Levant. Nat. Hazards Earth Syst. Sci., 20, 125–148. doi:10.5194/nhess-20-125-2020
Trugman, D. T., Page, M. T., Minson, S. E., and Cochran, E. S. (2019). Peak Ground Displacement Saturates Exactly when Expected: Implications for Earthquake Early Warning. J. Geophys. Res. Solid Earth 124, 4642–4653. doi:10.1029/2018JB017093
Keywords: TRUAA, earthquake early warning, epic, Israel, ground motion prediction
Citation: Nof RN, Lior I and Kurzon I (2021) Earthquake Early Warning System in Israel—Towards an Operational Stage. Front. Earth Sci. 9:684421. doi: 10.3389/feart.2021.684421
Received: 23 March 2021; Accepted: 21 May 2021;
Published: 11 June 2021.
Edited by:
Masumi Yamada, Kyoto University, JapanReviewed by:
Gemma Cremen, University College London, United KingdomCopyright © 2021 Nof, Lior and Kurzon. This is an open-access article distributed under the terms of the Creative Commons Attribution License (CC BY). The use, distribution or reproduction in other forums is permitted, provided the original author(s) and the copyright owner(s) are credited and that the original publication in this journal is cited, in accordance with accepted academic practice. No use, distribution or reproduction is permitted which does not comply with these terms.
*Correspondence: Ran N. Nof, cmFuLm5vZkBnbWFpbC5jb20=
Disclaimer: All claims expressed in this article are solely those of the authors and do not necessarily represent those of their affiliated organizations, or those of the publisher, the editors and the reviewers. Any product that may be evaluated in this article or claim that may be made by its manufacturer is not guaranteed or endorsed by the publisher.
Research integrity at Frontiers
Learn more about the work of our research integrity team to safeguard the quality of each article we publish.