- 1Earth Science Department, Faculty of Sciences, Vrije Universiteit, Amsterdam, Netherlands
- 2VOF Kytalyk Carbon Cycle Research, Epse, Netherlands
- 3Plant Ecology and Nature Conservation Group, Wageningen University, Wageningen, Netherlands
- 4Remote Sensing and GIS Department, NLR, Marknesse, Netherlands
- 5Institute for Biological Problems of the Cryolithozone, Siberian Branch Russian Academy of Sciences, Yakutsk, Russia
Ice-ridge Yedoma terrain is susceptible to vertical surface displacements by thaw and refreeze of ground ice, and geomorphological processes of mass wasting, erosion and sedimentation. Here we explore the relation between a 3 year data set of InSAR measurements of vertical surface displacements during the thaw season, and geomorphological features in an area in the Indigirka Lowlands, Northeast Siberia. The geomorphology is presented in a geomorphological map, based on interpretation of high resolution visible spectrum satellite imagery, field surveys and available data from paleo-environmental research. The main landforms comprise overlapping drained thaw lake basins and lakes, erosion remnants of Late Pleistocene Yedoma deposits, and a floodplain of a high-sinuosity anastomosing river with ancient river terrace remnants. The spatial distribution of drained thaw lake basins and Yedoma erosion remnants in the study area and its surroundings is influenced by neotectonic movements. The 3 years of InSAR measurement include 2 years of high snowfall and extreme river flooding (2017–2018) and 1 year of modest snowfall, early spring and warm summer (2019). The magnitude of surface displacements varies among the years, and show considerable spatial variation. Distinct spatial clusters of displacement trajectories can be discerned, which relate to geomorphological processes and ground ice conditions. Strong subsidence occurred in particular in 2019. In the wet year of 2017, marked heave occurred at Yedoma plateau surfaces, likely by ice accumulation at the top of the permafrost driven by excess precipitation. The spatial variability of surface displacements is high. This is explored by statistical analysis, and is attributed to the interaction of various processes. Next to ground ice volume change, also sedimentation (peat, colluvial deposition) and shrinkage or swelling of soils with changing water content may have contributed. Tussock tundra areas covered by the extreme 2017 and 2018 spring floods show high subsidence rates and an increase of midsummer thaw depths. We hypothesize that increased flood heights along Siberian lowland rivers potentially induce deeper thaw and subsidence on floodplain margins, and also lowers the drainage thresholds of thaw lakes. Both mechanisms tend to increase floodplain area. This may increase CH4 emission from floodplains, but also may enhance carbon storage in floodplain sedimentary environments.
Introduction
Permafrost is increasingly influenced by climate warming. Permafrost thaw is expected to enhance greenhouse gas emissions from permafrost soils and to decrease stability of soils, in particular in ice-rich permafrost areas (e.g. IPCC, 2013; AMAP, 2017). A specific type of ice-rich permafrost formation that occurs over large areas in Siberia and Alaska is Yedoma, also known as Ice Complex: periglacial loess and fluvial silt deposits that were deposited during Quaternary glacials in unglaciated terrain, which obtained large masses of excess ice by the growth of syngenetic ice wedges during sedimentation (Schirrmeister et al., 2013; Murton et al., 2015; Strauss et al., 2017). Yedoma contains a considerable carbon stock (Zimov et al., 2006; Strauss et al., 2017). These deep ice-rich deposits (several tens of meters and more) are very vulnerable to abrupt thaw (i.e. thermokarst) with rapid erosion, or more gradual subsidence, and may release carbon to the atmosphere as CO2 and CH4 (Schneider von Deimling et al., 2015; Vonk et al., 2013; Vonk et al., 2015). Surface displacements related to permafrost thaw also affect the stability of human infrastructure in permafrost areas. Structural instability can lead to severe environmental damage when urban, industrial or mining infrastructure is affected (e.g. Shiklomanov et al., 2017; Hjort et al., 2018).
Climate-related changes that may enhance permafrost thaw are higher air temperatures in winter and summer, and changes in precipitation that affect snow cover thickness, soil water saturation, water ponding, river flooding, fire frequency and vegetation change (e.g. reviews by Anisimov and Nelson, 1997; Nelson et al., 2002; Jorgenson et al., 2010; Nauta et al., 2014). Vegetation change also may delay permafrost thaw (Blok et al., 2010; Jorgenson et al., 2010; Kanevskiy et al., 2017). The effects of these climatic factors act unevenly across the landscape, since they are affected by geomorphologically determined terrain characteristics as slope, insolation, drainage and exposure to river flooding and erosion.
Net loss of ice by thaw of ice-rich permafrost over several years is reflected by a trend of surface subsidence, superimposed on the yearly surface displacements due to thaw at the top of the permafrost in summer (subsidence) and regrowth of ice in autumn and winter (frost heave). These surface displacements can be detected by synthetic aperture radar (SAR) interferometry from satellites (InSAR), and can assist in outlining areas that are most vulnerable to soil subsidence from permafrost thaw (Liu et al., 2010; Liu et al., 2012; Liu et al., 2014; Teshebaeva et al., 2020). Ice content is known to be an important parameter in surface subsidence (e.g. Jorgenson et al., 2010; Schneider von Deimling et al., 2015; Turetsky et al., 2019; van Huissteden, 2020), at least on a local scale. The soil ice distribution in Yedoma landscapes is also related to geomorphology and (cryo) stratigraphy of deposits, that determine duration and mode of ice accumulation: growth of wedge ice and small-scale cryostructure ice. This varies from large scale variation, e.g. large ice volumes in deep syngenetic wedges in Yedoma vs. lower ice volumes in deposits of Holocene thaw lake beds and floodplains (Kanevskiy et al., 2013; Morgenstern et al., 2013; Strauss et al., 2017), and variations determined by sedimentary patterns of floodplain and thaw lake sediments (Ulrich et al., 2014). Thus, the spatial variation of vulnerability to surface subsidence is expected to be strongly related to geomorphology.
Here, we present a geomorphological map of an Eastern Siberian Yedoma area in the Indigirka Lowlands, combined with InSAR surface displacement measures using Sentinel-1A/B data collected over 3 years. This time series is too short to quantify long term surface displacement trends. However, the data result from years with strongly contrasting seasonal weather patterns: 2 years of high winter precipitation resulting in pronounced spring flooding, and a year with low winter precipitation, early spring and warm summer. Therefore these data contain information that permits detection of differences in surface stability between terrain units, differences among years with contrasting weather patterns, and inferences on the processes that determine vertical surface displacements, such as ground ice thaw and accumulation, river flooding, erosion/sedimentation and soil processes. Also neotectonics influence the landscape on a large scale.
We explore the relation of surface displacements with geomorphological terrain units, and the interaction of these with the contrasting yearly weather patterns, by statistical analysis. Our analysis shows, that patterns of surface displacements vary with geomorphological position, and between years.
The carbon cycle and greenhouse gas emission impacts of geomorphological changes, changes in active layer thickness (ALT), soil subsidence and erosion as detected by InSAR are potentially large (e.g. Liu et al., 2010; Liu at al., 2014; Abbott and Jones, 2015; Natali et al., 2015). We discuss the potential carbon cycle effects of our findings in general terms.
Study Area
The study area is located at the Kytalyk/Chokurdagh tundra research station (70°49′N, 147°29′E), approximately 30 km WNW of the town of Chokurdagh, and located just north of the Berelegh river, a tributary to the Indigirka river (Figure 1). From 2003 onwards, data were collected on the carbon balance of the tundra and permafrost soil (Van der Molen et al., 2007; Blok et al., 2010; Parmentier et al., 2011a; Parmentier et al., 2011b; Parmentier et al., 2011c; Nauta et al., 2014; Dean et al., 2020), as well as data on vegetation, heat balance, soils, soil ice content and environmental history (Juszak et al., 2014; Beermann et al., 2015; Juszak et al., 2016; Teltewskoi et al., 2016; Weiss et al., 2016; Juszak et al., 2017; Li et al., 2017; Wang et al., 2018; Magnússon et al., 2020). The site is located on continuous permafrost. It is dominated by three main terrain units: the Berelegh river plain, Yedoma terrace remnants dating from the Last Glacial, and Holocene drained thaw lake basins (DTLB). The area has recently (2007, 2017, 2018) experienced several high to extremely high spring snowmelt floods (This paper; Tei et al., 2020). The geomorphological map is based on high resolution visible spectrum images (Geo-Eye, Worldview), high resolution digital elevation models (DEMs) derived from radar satellites, and terrain surveys.
The permafrost is characterized by a shallow active layer, overlying continuous permafrost of over 300 m thickness. Near the surface, it is underlain by ice-rich Yedoma deposits (Schirrmeister et al., 2011), Holocene fluvial deposits of the Berelegh river and drained thaw lake basin fills (Alas deposits hereafter). The nearest weather station is that of Chokurdagh airport, WMO station code 21,946, 27 km away from the study site. The mean annual air temperature is −13.4°C, with an average July temperature of 10.3°C (1981–2010). Mean annual precipitation is 196 mm, with 76 mm falling in June to August (1981–2010) (Trouet and Van Oldenborgh, 2013).
The data from the weather station (Figure 2A, NOAA, 2020) show a conspicuous warming trend, in particular in the autumn months (October, November), winter and late winter (January, March, April). The winter precipitation shows marked variability in recent years, with three consecutive years of excessive snowfall in 2016–2018 (Figure 2B). The high snowfall winters of 2016–2017 and 2017–2018 are also demonstrated by the ERA5 reanalysis precipitation data in Figure 2C. The years 2017 and 2018 are similar in terms of precipitation and temperature. The year 2019 deviates from the previous 2 years, being considerably warmer and dryer than the previous years, both in summer and winter. This is demonstrated by the freezing and thawing degree days indices and cumulative winter and summer precipitation derived from the reanalysis data (Figure 2D).
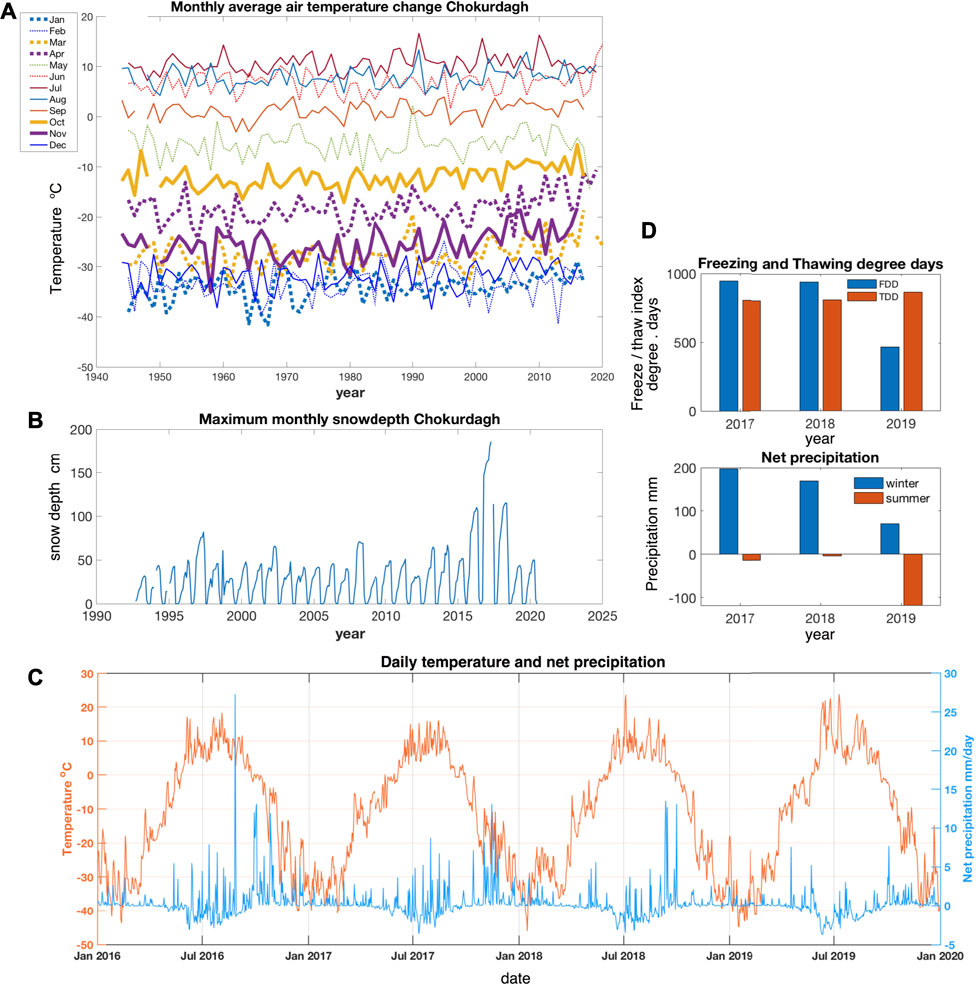
FIGURE 2. (A): Mean monthly air temperatures (1944–2020) of Chokurdagh weather station. Thick lines represent months with a significant temperature trend (linear regression, p < 0.01). From 2017 onwards data are not always complete; months with >9 missing observation days are not included. (B): Maximum monthly snow depth (cm) of Chokurdagh weather station, 1993–2020. (C): Temperature and net precipitation (precipitation-evaporation) from ERA5 reanalysis (Hersbach et al., 2020); data of a 1° square around the study area, from 2016 to 2020. (D) above: freezing degree days (FDD) and thawing degree days (TDD), and below: winter (October-April) and summer (May-Sept) net precipitation totals for 2017–2019 derived from the ERA5 data.
The Berelegh river is an approximately west-east running tributary to the lower Indigirka river. The Berelegh is an anastomosing, multi-channel river with highly sinuous channels and wide marshy floodbasins with lakes. To the north of the floodplain, the landscape is dominated by Late Pleistocene-Holocene aged thaw lakes and drained thaw lake basins (DTLB’s), and plateau-like erosion remnants of the Pleistocene Yedoma deposits. In the DTLB’s, pingos are common. The DLTB relief is subdued with an elevation in the order of 10–12 m ASL; the DTLB floors are only a few meters higher than the floodplain surface (8–10 m), whilst the Yedoma plateaus rise some 10–25 m above the DTLB floors to elevations up to 35 m ASL. To the south of the Berelegh floodplain, the elevation of the Yedoma surface is higher (40–60 m ASL; Allaikhovsky Highland), and the area of lakes and DTLB is comparatively smaller (Figure 3; Schirrmeister et al., 2011).
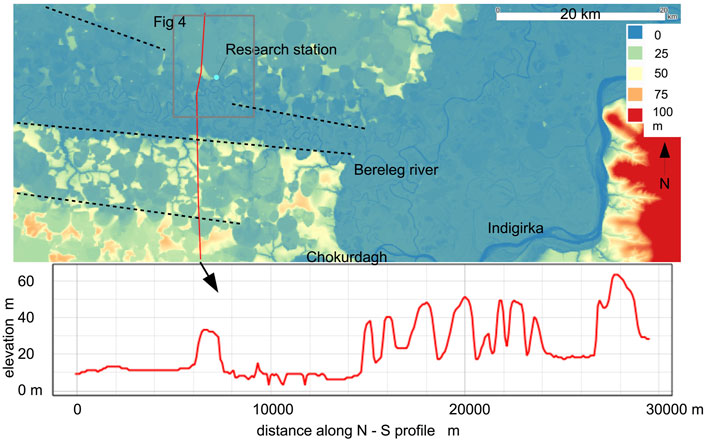
FIGURE 3. Tandem-X elevation map of the study area and its surroundings. The elevation profile (below) is situated slightly west from Kytalyk Tundra Research Station (blue dot). The grey square indicates the map area of Figure 4. Broken black lines indicate possible tectonic lineaments.
The area is largely covered with Pliocene-Pleistocene deposits. At Chokurdagh and on the east side of the Indigirka river Mesozoic basaltic rock and Paleogene-Neogene sandstone crops out. In the Dzhelon-Sisi upland west of the study site, the Eocene Tastakh Formation consisting of clays and sands with coal lenses and gravel is exposed (Schirrmeister et al., 2011; Ren et al., 2013; Akhmetiev, 2015; Drachev, 2016). Below the Yedoma deposits, the Plio-Pleistocene Keremesit and Olyor Formations occur, which consist of fluvial and lacustrine silts and sands, with similar ice-rich characteristics as the Yedoma (Shmelev et al., 2017).
Tectonically, the area lies within the interaction zone of the Eurasian and North American plates (Imaeva et al., 2016a; Imaeva et al., 2016b; Drachev, 2016). The area is situated on the North American plate, consisting here of terranes of various origin. The Cenozoic sedimentary cover is bordered to the south by the Polousnyi mountain ridge, which is an active thrust belt with an WNW-ESE strike. To the SW of the area, the Chersky seismic belt is located (Fujita et al., 2009). Its seismic activity reaches to just south of the study area, with several reports of small (magnitude <4) earthquakes (Imaeva et al., 2016a; Imaeva et al., 2016b). To the north of the area, the intensely deformed Late Jurassic-Early Cretaceous South Anyui suture zone is buried beneath the Cenozoic sedimentary cover (Drachev, 2016).
Data and Methods
Data
The SAR dataset used in this study was acquired by Sentinel-1 A/B C-band (∼5.7 cm wavelength) satellite sensor, covering the seasonal time period from June to September for 2017–2019 years. We used 30 Sentinel-1A/B IW SLC SAR images acquired in descending orbits with an incidence angle of 37–39° and a pixel spacing of 2.3 × 14.1 m (range x azimuth).
A Digital Elevation Model (DEM) from TanDEM-X mission is used for InSAR processing, at 90 m spatial resolution. TanDEM-X (TerraSAR-X add-on for Digital Elevation Measurements) is an Earth observation radar mission that consists of a SAR interferometer built by two almost identical satellites flying in close formation. With a typical separation between the satellites of 120–500 m a global Digital Elevation Model (DEM) was generated (Wessel et al., 2018).
In addition a high resolution global DEM from ALOS PRISM (Tadono et al., 2014; Takaku at al., 2016) with spatial resolution of 5 m is used for geomorphology analyses.
The high resolution visible spectrum images have a resolution 0.5 m in panchromatic mode and 1.8 m in multispectral mode and were acquired by the following satellites and dates: GeoEye-1, 0.41 m/1.64 m resolution in panchromatic, resp. multispectral mode, image date 2010-08-19), Worldview 2 (0.46 m/1.84 m panchrom./multispectral, date 2015-07-10 and 2019-08-01), Worldview 3 (0.31/1 0.24 m panchrom./multispectral, date 2018-07-05). The images from 2015 to 2019 were completely cloud-free. The image of 2015 was chosen as baseline for comparison with the velocity data, because it represents approximately average summer soil moisture conditions.
InSAR Processing
The InSAR technique exploits multiple SAR images and applies appropriate data processing and analysis procedures to separate the contribution of the phase caused by the deformation, from the other phase components. The technique focuses on the identification of pixels in the SAR image characterized by small noise, which have typically two properties: the radar response is dominated by a strong reflecting object, and remains constant over time (Persistent Scatterer, PS) (Ferretti at al., 2001).
The SAR imagery is processed using ESA’s Sentinel Application Platform (SNAP) S1-toolbox (Veci et al., 2014) and Stanford Method for Persistent Scatterers (StaMPS) by the University of Leeds (Hooper, 2008). We used statistical-cost, network-flow phase-unwrapping algorithm (SNAPHU) for unwrapping of the interferometric phase (Chen and Zebker, 2002). The topographic phase is removed using TanDEM-X 90 m DEM (Wessel et al., 2018).
The standard deviation of the mean line-of-sight InSAR velocity is estimated as 4–5 mm/yr. See Supplementary Information for further details on error sources and data processing. A drawback of the InSAR data is the uneven spatial distribution of data points, as is determined by the persistent scatterer algorithm in InSAR processing, which requires stable surface properties over time. This introduces a location bias. Winter data (with variable snow cover thickness) are not included, and the dynamic floodplain (ARF) with varying water cover has a low data point density.
We concentrate in our analysis on the thaw season surface displacement processes. However, summer subsidence may be compensated in winter by heave (Supplementary Figures S1, S2), as indicated by positive surface displacement between the last images of 2017 and 2018 and the first images of the next year. This is attributed to spatially varying ice accumulation in the active layer during the winter.
Soil Ice Content and Active Layer Thickness
Ice content data have been collected by Weiss et al. (2016) in 2012, and in 2013 by the authors. The samples were collected by hammering a steel pipe into the top of permafrost, and carefully extruding the sample in 5–10 cm increments. The samples are weighed and dried, to determine the gravimetric ice content. The ALT at selected sites was measured by probing with a thin steel rod (0.8 cm thickness). Gravelly or stony material is absent in the study area.
Geospatial Analysis
Geospatial data analysis was done with Quantum GIS version 2.18 (QGIS.org, 2016). A landform and surface micro-relief map is produced based on a synthesis of all satellite images, DEM data and field surveys made over the period 2004–2018. The main landform classes are: Yedoma remnant (YR hereafter), drained thaw lake basins (DTLB), active river floodplain (ARF) and river terrace (RT). Table 1 shows further subdivisions in geomorphological details and micro-relief (mainly ice-wedge polygons and their morphology and degree of visibility; for slopes also erosion features such as rills).

TABLE 1. Subdivisions of the main geomorphological map units into subunits, and micro-relief classification for these units.
Pond density classes were visually estimated. For the DTLB class, an estimate of the relative age was made based on overlapping relations, in combination with ice wedge polygon and surface micro-relief development, which can be used as indicator of age (Hinkel et al., 2003). Based on image time series, flooding frequency classes were derived (permanently flooded, seasonally flooded, extreme floods only and no flooding). ARF flooding frequency was checked by a longer time series of lower resolution Landsat images (Cheung, 2019). The visible spectrum images were also used to derive the normalized difference water index (NDWI, McFeeters, 1996) and normalized difference vegetation index NDVI (near infrared-red)/near infrared+red). NDWI (green + near infrared)/(green−near infrared), indicates the amount of open water within a pixel, NDVI indicates the amount of green biomass. The NDWI data of the 2015 image, with average wetness conditions and completely cloud-free, were combined with the landform data.
Statistical Analysis of InSAR and Terrain Data
The InSAR dataset contains both spatial and temporal variation, and interaction between these sources of variation. The analysis is based on the assumption that spatial variation is related to terrain characteristics expressed by the geomorphological map data, and the temporal variation is caused by the weather patterns throughout the observation years, affecting loss or gain of ice, water or sediment. Interaction between spatial and temporal variation is likely since the weather conditions may influence terrain units differently; e.g. by variation in soil drainage conditions that affect soil water content and thermal properties of the soil. To explore these relations, the InSAR data have been compared with the categorical scale data of the geomorphological map and ratio scale data derived from DEMs and visible spectrum images.
To characterize temporal variability of each data point, we performed a hierarchical cluster analysis (Euclidian distance, Ward’s method, divisive algorithm), which groups all data points into dissimilar groups of displacement trajectories, based on the vertical displacements.
Next we applied two pathways of analyzing the relation between terrain characteristics and vertical displacements. The first pathway considers the interval/ratio scale data from the ALOS DEM and visible spectrum Worldview 2 image of 2015 separately. These data are elevation (ELEV), panchromatic greyscale (GS), NDVI and NDWI derived from the Worldview image. ELEV represents drainage conditions and geomorphology, NDVI and GS represent vegetation biomass and type. High values of NDWI indicate areas with much ponded water relative to vegetation, low GS also indicates presence of ponds. First we calculated the Pearson correlation coefficients between these terrain units and respectively the total summer displacement over the 3 years, and the total for each year separately. Next we applied principal component analysis on this dataset (mean and variance standardized) to explore which combination of properties explain most of the variance, and for dimensionality reduction.
The second pathway includes the categorical scale data in the geomorphological map. For relations of surface displacement with the categorical data of the geomorphological map we considered as potential driver variables:
• the large landform units (Drained thaw lake basins, river floodplain and terrace, Yedoma)
• erosion, sedimentation or ground ice thaw phenomena
• pond density
• river flooding classes
A one-way analysis of variance (ANOVA) for each combination of yearly InSAR data and the categorical classes Landform, EST, Pond density and Flooding) was applied, to detect which of the these categories are linked to surface displacements. A Tukey-Kramer multi-comparison was used to identify classes that differ significantly from others.
Next, the numerical and categorical data selected by PCA and ANOVA are combined into a generalized linear regression model which was fitted stepwise to the surface displacement data of each year separately, and to the summed surface displacement over all years (Σ years). Interaction terms were not included. The regression model gives information on the strength of relationships.
Results
Landforms
The area is dominated by overlapping DTLB (54.5%) and the recent floodplain (ARF, 39.1%). Yedoma remnants (YR, 3.7%) and the river terrace (RT, 2.8%) make up a small fraction of the area. The YR are plateau-like features with a nearly flat upper surface, likely representing an older land surface. An isolated hill consisting of Yedoma deposits testifies former expansion of the Yedoma surface in the northern part of the study area (1 in Figure 4). This old surface slopes gently towards the river (Supplementary Figure S3). The Yedoma deposits have a Late Pleistocene age; calibrated 14C ages of organic matter and bone at a lake bank exposure are 31,745 and 20,829 years BP, ages of cryoturbated organic matter in the top of the succession are 1,674 and 11,449 years BP (Weiss et al., 2016). The youngest date likely contains young soil material.
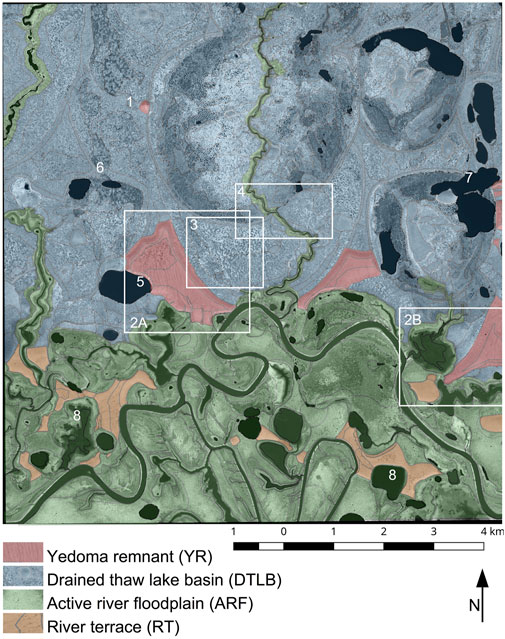
FIGURE 4. Main landform classes in the study area. Backdrop: 2019 Worldview panchromatic image. Numbers refer to locations discussed in text, rectangles to locations of other figures. WorldView © 2019 MAXAR.
The YR surface is generally well-drained, but on larger plateau areas low center polygons with intermittent ponded water occur. Tussock tundra vegetation dominates; surface drainage occurs through sedge-lined diffuse water courses, parallel to each other. On steeper slopes these grade into small rills between tussocks, and eventually in gullies where these rills concentrate.
The RT has similar surface characteristics as the YR. The RT may be the river valley equivalent of the YR, although traces of channel systems are not visible. In one location (2B in Figure 4; Supplementary Figure S3B), lateral continuity is suggested by a gradual slope from Yedoma plateau to terrace. Dating information from RT’s is not available; a younger (Holocene) age than the Yedoma cannot be excluded. The RT is likely underlain by ice-rich permafrost, as shown by erosion features at RT edges.
The DTLB surface has a 10–20 m elevation difference with the YR surface. It is covered by overlapping drained lake basins. Differences in elevation up to 3 m are common, representing sedimentary features of the lakes, and elevation differences between individual basins. The basins differ in surface micro-relief, ranging from well-defined low center polygons in younger basins, to areas where ice wedge polygons are obscured by peat formation in older basins (Teltewskoi et al., 2016; Weiss et al., 2016). In the latter case ice wedges are visible by the formation of linear thaw ponds. A pattern that appears to develop over time on slightly sloping surfaces is a ribbed pattern with parallel, diffuse drainage channels with a dense sedge vegetation, alternating with ±0.5–1 m higher ridges covered by Betula nana heath (Figure 5). Well-drained areas are dominated by tussock tundra. Features indicating ground ice thaw (thaw ponds) are ubiquitous in DTLB’s (Figures 5, 6). Furthermore, Sphagnum peat growth is widespread, indicated on panchromatic images by light tones.
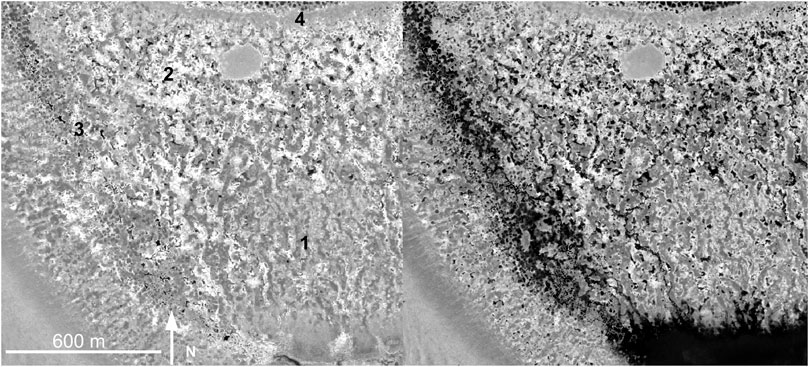
FIGURE 5. Left: August 2019 Worldview image. Surface patterns in old drained thaw lake basins. 1: Ribbed pattern with sedge-lined diffuse drainage lines alternating with Betula nana heath (dark grey). 2. Area dominated by extensive Sphagnum growth and small thaw ponds. 3: Low and high centered polygons with pond formation by ice wedge thaw. 4. Stable low centered ponds in a younger basin (see also Supplementary Figure S4). Right: the same area with widespread flooding, July 2017. Location 2 of the image see Figure 4 at nr 3. WorldView © 2019 MAXAR.
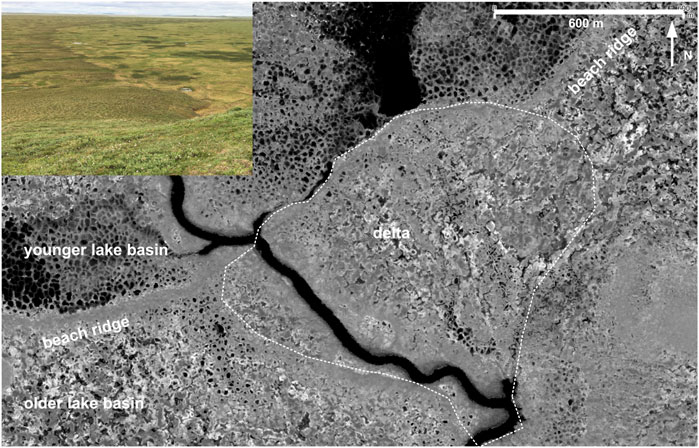
FIGURE 6. Border between two lake basins with different age and surface micro-relief. The elevation difference is less than 1 m. The border of the younger lake is marked by a low ridge. In the center a former river delta. Inset: beach ridge of the same lake seen from point 1 in Figure 4; in the lower left corner the beach ridge is overridden by a thaw slump debris lobe, photo J. vanHuissteden. Location of the image frame: see Figure 4 at nr 4. WorldView © 2019 MAXAR.
Individual basins are lined with a low ridge marking their former banks, interpreted as a beach ridge or ice shove ridge built up by material bulldozed ashore by a floating lake ice cover (Figure 6). The elevation difference between individual basins is small, 1 m or less. Figure 6 also shows a fluvial delta, built into a lake by a channel connecting the lake with the river floodplain. At present, drainage through this channel has reversed, with flow towards the river, likely caused by river downcutting. Other relief elements are slightly elevated shore platforms that line the lake banks on the lake side, and a central bulge of the lake floor, shown as a better drained area in the center of the lake basin.
The overlapping relations of the lake basins were reconstructed, based on polygon maturity (Hinkel et al., 2003), surface elevation, and intersection of beach ridges (Supplementary Figure S4). Only from the older lake bed depicted in Figures 5, 614C dates are available (Schirrmeister et al., 2011; Weiss et al., 2016). Weiss et al. report a calibrated age of 3,251 years BP from a buried peat bed, and ages of 2,884 and 8,016 years BP for cryoturbated peat. Schirrmeister et al. (2013) report uncalibrated ages of 1,632 ± 32 and 2,144 ± 33 from peat. The age of 8,016 years BP indicates that the lake was drained in the Early Holocene. Renewed clastic sedimentation led to the burial of a peat bed after 3,251 years BP. Teltewskoi et al. (2016) report a calibrated basal 14C age of 3,630 ± 40 for the surface of this lake bed.
Within the DTLB unit, lakes are common. Some of these are actively expanding thaw lakes (e.g. at 5 in Figure 4). Other lakes are remnants after partial lake drainage, or infilling of lakes with peat or clastic sediment (e.g. at 6 in Figure 4). These remnant lakes also may start to expand again, which occurred at 7 in Figure 4. New lakes are created by surface subsidence and merging of polygon ponds and thaw ponds; these are generally smaller and shallower lakes or ponds and have an irregular shape.
Pingos (bulgannyakhs) of all sizes occur abundantly on the drained lake basins (Supplementary Figure S4). Some consist of irregular shaped complexes. The pingos are of the hydrostatic type (Mackay, 1998). A field survey of pingos in the central section of the study area did not show any water seepage activity associated with these pingos, suggesting low or absent activity (Mackay, 1998).
Actively eroding slopes between YR and DTLB occur only near expanding lakes (5 in Figure 4); erosion is dominated by thaw slumps (e.g. at 1 and 5 in Figure 4). When lake bank erosion stops, slump activity continues for some time; at 1 in Figure 4 lobes of thaw slump debris overriding the adjacent DTLB are found. Older slopes have lost their slump morphology by rill erosion and colluviation. Erosion occurs by rills between vegetation hummocks, along ice wedges and occasionally gully erosion. On lower slopes, colluviation and deposition of small alluvial fans likely dominates.
The ARF is separated by clear terrace scarps from the RT and often also from the adjacent DTLB surface; those separating ARF and RT are highest. This indicates two phases of downcutting of the river, after formation of the RT surface and after formation of the oldest DTLB’s.
The ARF has a large proportion of lakes. By contrast to the lakes in DTLB, the water level and size of most of these lakes varies with the flood level of the river (e.g. Figure 7, Cheung, 2019). Many lakes likely have an origin as thaw lakes which have become connected with the river (1, 3 in Figure 7), or as thaw lakes eroding the RT unit (e.g. 2 in Figure 7). Several deeper lakes maintain a stable water level at low river stands. These lakes are not connected with outlet channels to the river channel, but nevertheless receive river water during high flood stages.
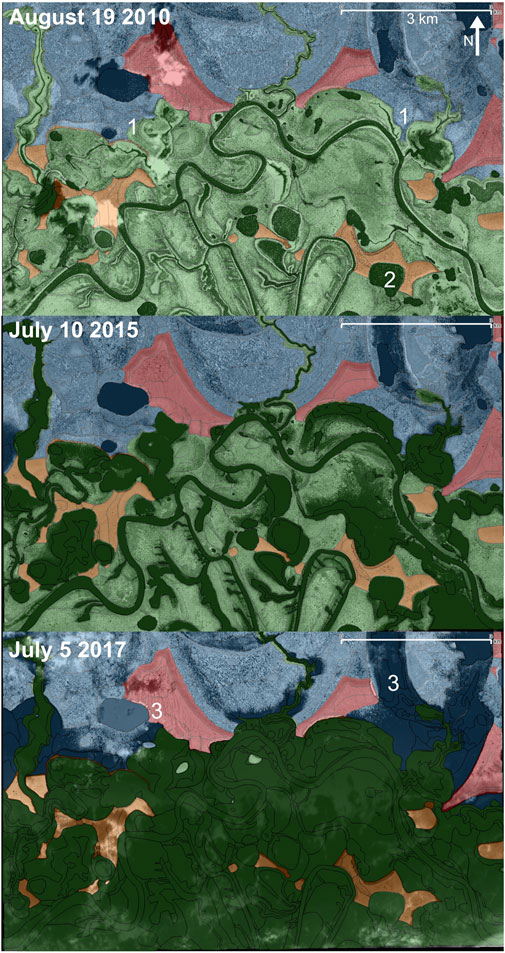
FIGURE 7. Open water on the river floodplain at different river stages. Backdrop: geomorphological map units, see Figure 4 for legend. Top: low stage, end of summer; mid: normal, ± bankfull river stage, below: extremely high flood, exceeding the normal floodplain limits. 1: river-connected thaw lakes; 2: thaw lake developed on floodplain in river terrace; 3: locations where connections between thaw lake and river is developing during high flood. WorldView © 2019 MAXAR.
The river channel pattern is characterized as a high sinuosity river with multiple channels, but dominated by one main channel (Figure 8). At low stage, the non-lake floodplain surface in between the channels consists of mostly vegetated, non-flooded flood basins, dominated by grasses and sedges, and channel levees with willow brushes. A part of these flood basins consist of point-bars with ridge and swale topography created by river bend expansion. The vegetation is denser and more productive than on similar flat areas outside the floodplain (DTLB, YR plateaus). The lowest floodplain levels (channel banks, drained lakes) are unvegetated. Ice wedge polygons are ubiquitous. At low stage in winter, the channel ice surface is some 2–3 m below the floodplain surface, exposing steep channel banks. These banks are sources of aeolian dust, as shown by streaks of dust over the winter snow surface.
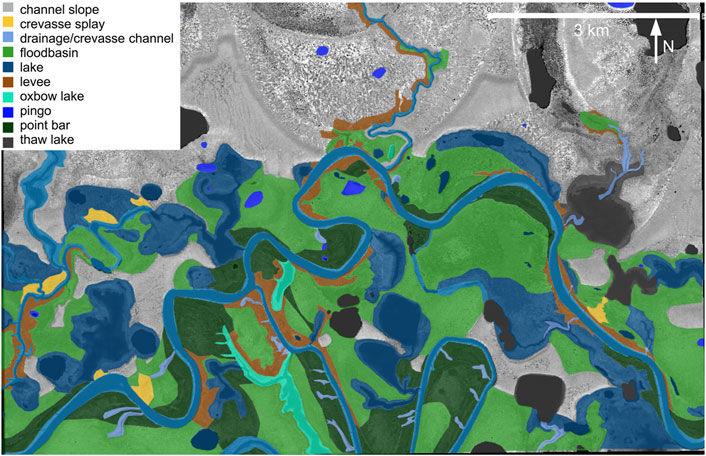
FIGURE 8. Geomorphology of the active floodplain (ARF) with subunits. Backdrop: Lower half of Worldview 2019 image in Figure 4. Unclassified areas are the RT (old river terrace), YR and DTLB areas. WorldView © 2019 MAXAR.
Active sedimentation occurs on floodplain surfaces on levees and point bars, but also by suspended sediment and organic debris deposition in flood basins. Sediment cores in flood basins show successions of vegetation horizons, alternating with layers of fine-grained clastic material. In a core in flood basin sediments taken on a crevasse splay, a sedimentation rate of 0.48 ± 0.10 cm yr−1 was determined by radiocarbon dating of a vegetation horizon (185 ± 30 years, Groningen lab. nr. GrA59784, at depth of 90–95 cm). This sedimentation rate is corrected for a 15 vol. % excess ice content below the 0.5 m thick active layer.
Ice Content and Active Layer Depth
The ice content at the top of the permafrost varies strongly over short distances. The average ice content for DTLB is 42.60 ± 20.32% (n = 34), for YR 38.5 ± 18.46% (n = 12) and for ARF 26.45 ± 19.45% (n = 12). The YR and DTLB have approximately equal topsoil ice content. The ice content of ARF is lower than that of YR and DTLB; it differs significantly from that of DTLB (Tukey-Kramer test, p = 0.04). Fresh YR exposures show large ground ice masses in ice wedges. A 7 m deep borehole in DTLB sediments near the research station showed a decrease of ground ice after the top 3 m (G. Iwahana, pers. comm.).
On 19–20 July 2018, three transects of midsummer active layer thickness (ALT) were measured perpendicular to the maximum flood lines in 2017 and 2018 (Figure 9), and compared with ALT measurements in grids outside the flooded area. Transect 1–3 are located entirely on usually mesic tussock tundra, transect 4 in a wet area dominated by hummocky Sphagnum and Salix vegetation and a thicker surface organic horizon. The ALT for transect 1 to 3 show a significant increase at the flood limits (t-test, p < 0.01); this thickness increase is absent in the fourth transect. The grids a (100 × 100 m) and b (10 × 10 m) are active layer monitoring sites; grid a is located outside the flood limits (vegetation: tussock tundra and Betula nana heath), grid b (Sphagnum and Salix) inside the flood limits. The 100 point average of these grids, measured in the same week as the transects, are shown alongside the transect data. The average ALT of grid a is lower than that of transect 1–3 outside the flood limit; that of grid b is similar to transect 4.
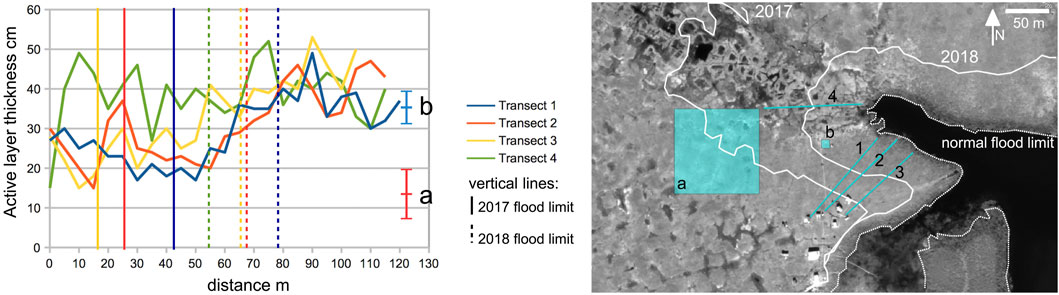
FIGURE 9. Active layer thickness transects through the 2017 and 2018 flood limits. Left: ALT thicknesses plotted against distances from the start of the transects (from ± east to west). Right: location of the transects (1–4). The average and standard deviation of ALT of two gridded plots, one largely outside the flood limits (left, a) and one within the 2018 and 2017 limits (left, b), is indicated to the right of the transects in the graph on the left side. Scale bar 50 m. WorldView © 2019 MAXAR.
Statistical Analysis of Velocity Data
The cumulative surface displacement over the thaw seasons of 2017–2019 are shown in Figure 10. There are clear regions where data points show similar displacement trends, either positive or negative. These displacement patterns vary among the years (Supplementary Figure S5). A hierarchical cluster analysis (Euclidian distance, Ward’s method) using the displacements detected from the successive SAR images is used to cluster the data points into 11 groups of trajectories. In Figure 11, most clusters also represent fairly tight spatial clusters.
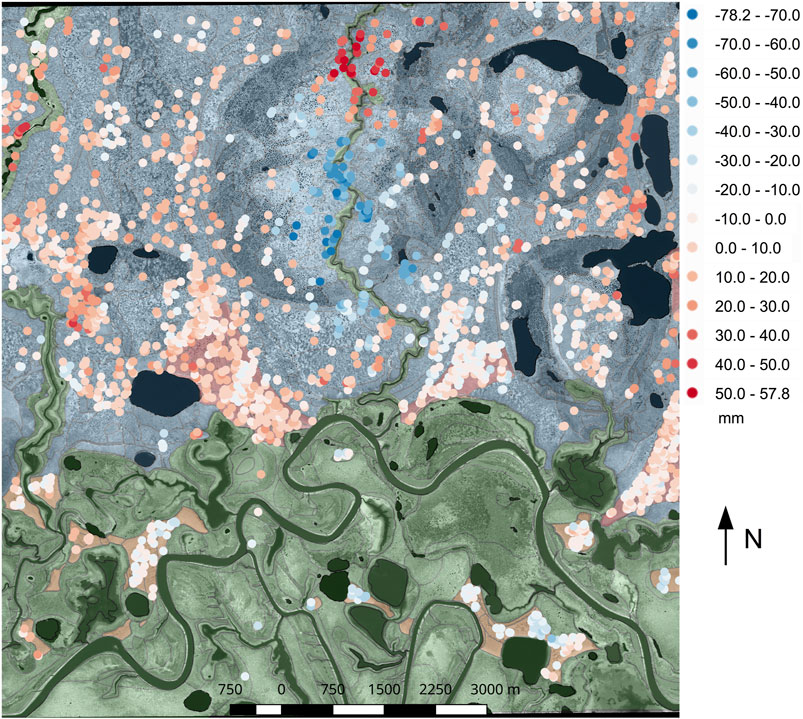
FIGURE 10. Cumulative thawing season surface displacement over 3 years; subsidence (−) and heave (+) velocities in mm. Background: 2019 Worldview image with landform class (Figure 4). See also Supplementary Figure S5 for cumulative displacement of every year and Supplementary Figure S2 for average surface displacement velocities over 3 years. WorldView © 2019 MAXAR.
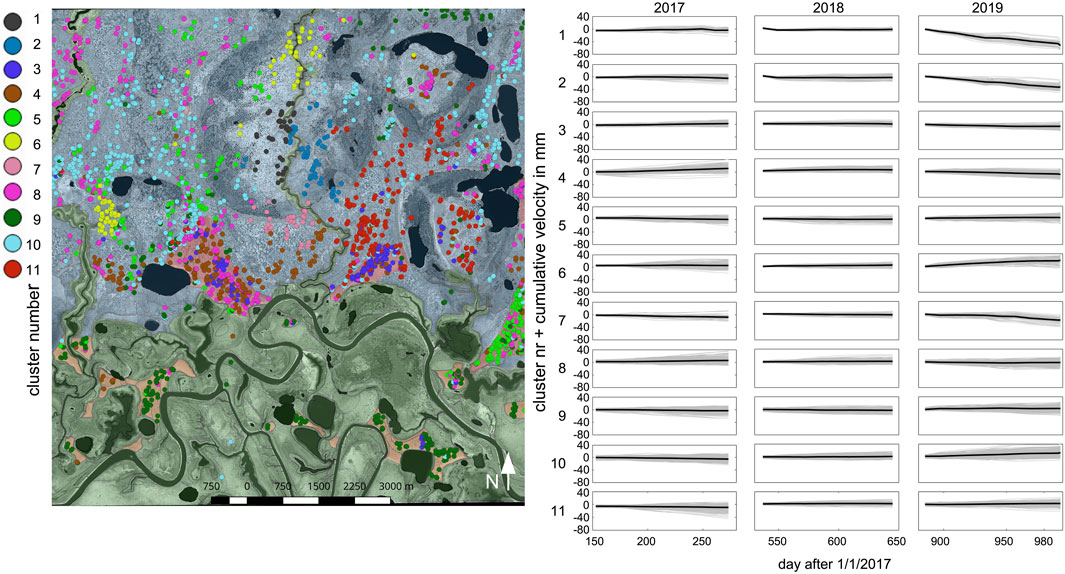
FIGURE 11. Left: velocity trajectory clusters to which the InSAR data points were assigned by cluster analysis of single InSAR image data (see text), plotted on the geomorphological map underlain by Worldview image of 2019. Scale bar 3 km. Right: Graphs of cumulative velocities plotted against image date for each cluster for each year. Black lines in each graph represent the cluster average velocity over time, grey lines individual point trajectories.
To evaluate relations of surface displacement with quantitative parameters of geomorphology, drainage and vegetation we have compared the total surface displacement over 3 years and the summed surface displacements over each year, with surface cover data derived from the Worldview image of 2015 (NDWI, Panchromatic greyscale (GS), NDVI) and the ALOS DEM. GS is related to both vegetation type and water ponding, NDVI reflects vegetation, NDWI water ponding. Table 2 shows the correlations of surface displacement with the quantitative image data and DEM. NDWI, GS and NDVI correlate with surface displacement, but not equally among years and with varying signs. The surface displacement in 2017 correlates weakly negative with NDVI and NDWI; for 2018 there are no significant correlations; for 2019, positive correlations are found. Because of their relation with ponded water, GS - NDWI, and NDVI - NDWI correlate negatively.
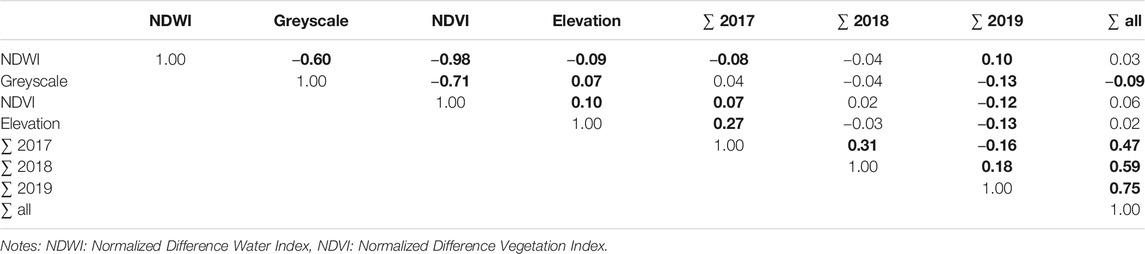
TABLE 2. Pearson correlation coefficients of yearly InSAR surface displacement sums and quantitative data derived from the Worldview 2015 image and ALOS DEM. Bold numbers are significantly strong correlations (p < 0.01).
Elevation has a strong positive correlation with surface displacement in 2017 (higher areas - less subsidence or more heave), but negative in 2019 (higher areas-more subsidence). Although there are significant correlations of displacement with vegetation-related parameters NDVI, greyscale and NDWI, these are weak in comparison to elevation. It indicates poor correlation with vegetation-related parameters and surface displacement, although this may have been affected to some extent by differences in spatial resolution between the InSAR and NDVI/NDWI data (see Data and Methods). Correlations of surface displacement between years have different signs; 2018 deviates, with negative correlation between the previous year and the next. These spatially different patterns of displacement in successive years is also visible in the trajectory clusters of Figure 11.
A principal component analysis on the standardized data shows that three components explain 95% of the total variance. The first principal component explains 60% of the variance, with a strong loading on the total surface displacement of 2019 (Supplementary Figure S6). The second principal component contains 27% of the variance, with the highest loading on the 2017 data. From the other variables, only elevation determines a small part of the total variance, in the third principal component (8% of total). This indicates that variability of the surface displacement is dominated by variability between years, related to elevation, but not to vegetation or drainage.
The categorical classes of the geomorphological tested in the further analysis are:
• the landform units (DTLB, ARF, YR, RT)
• erosion, sedimentation or ground ice thaw phenomena (EST hereafter)
• pond density (POND) classes (from 0-absent to 5 very high)
• river flooding (FLOOD) classes, ranging from none, flooding at extreme flood heights only (2017 and 2018 floods) and the normal seasonal flooding of the floodplain based on field surveys.
The ANOVA to detect the effect of the categorical classes of the geomorphological map (Landform, EST (erosion, sedimentation or ground ice thaw), POND and FLOOD) yields significant differences at the p < 0.01 level (Table 3, Supplementary Figure S7). A Tukey-Kramer multi-comparison was used to identify classes that differ significantly from others. The multi-comparison tests of class differences indicate significant differences (p < 0.01) for most of the classes, except for the EST and POND, where several classes do not differ significantly. In Table 3, only those classes have been listed that differ significantly from other classes within the same variable, and depart more than the uncertainty range (3 mm) from 0 mm displacement.
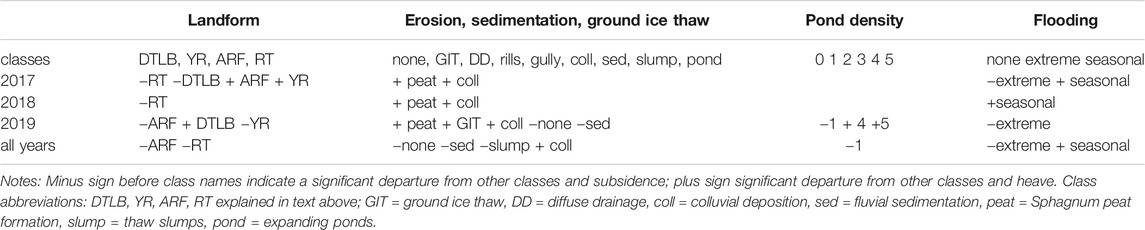
TABLE 3. Categorical variables and their classes used in anova, and multi-comparison (Tukey-Kramer) test results of differences between classes for each years and sum of all years.
The categorical variables from the geomorphological map explain a significant amount of the variability of the InSAR surface displacement. However, the behavior of each class is not consistent between years. For the landform classes, the floodplain and river terrace (ARF, RT) shows the most consistent behavior with predominantly subsidence throughout all years. For EST, classes that indicate net sedimentation in non-flooded areas (colluvial deposition and peat growth) show clear, and among the years consistent, net positive surface displacement. However, sedimentary environments on the river floodplain or DTLB areas show net subsidence. Areas with inferred ground ice thaw (indicated by ice wedge thaw ponds) do not show subsidence, and even positive displacement in 2019. Pond density is the least predictive, only the “very low” density class (1 in Table 3) shows significant subsidence in 2019, while the high pond density classes 4 and 5 show heave in 2019. The FLOOD classes show consistent subsidence for data points that were flooded during the extreme floods in 2017 and/or 2018, while the seasonally flooded class shows heave in 2017 and 2018.
A generalized linear regression model (Table 4) was fitted stepwise to the surface displacement data of each year and the summed surface displacement over all years (Σ years), with Elevation (ELEV), and the categorical geomorphological map classes listed in Table 4 as predictor variables. Elevation was included because of its contribution to the variance in the principal component analysis. Interaction terms were not included. All models capture a significant amount of the variance in the data (p < 0.01, F test).
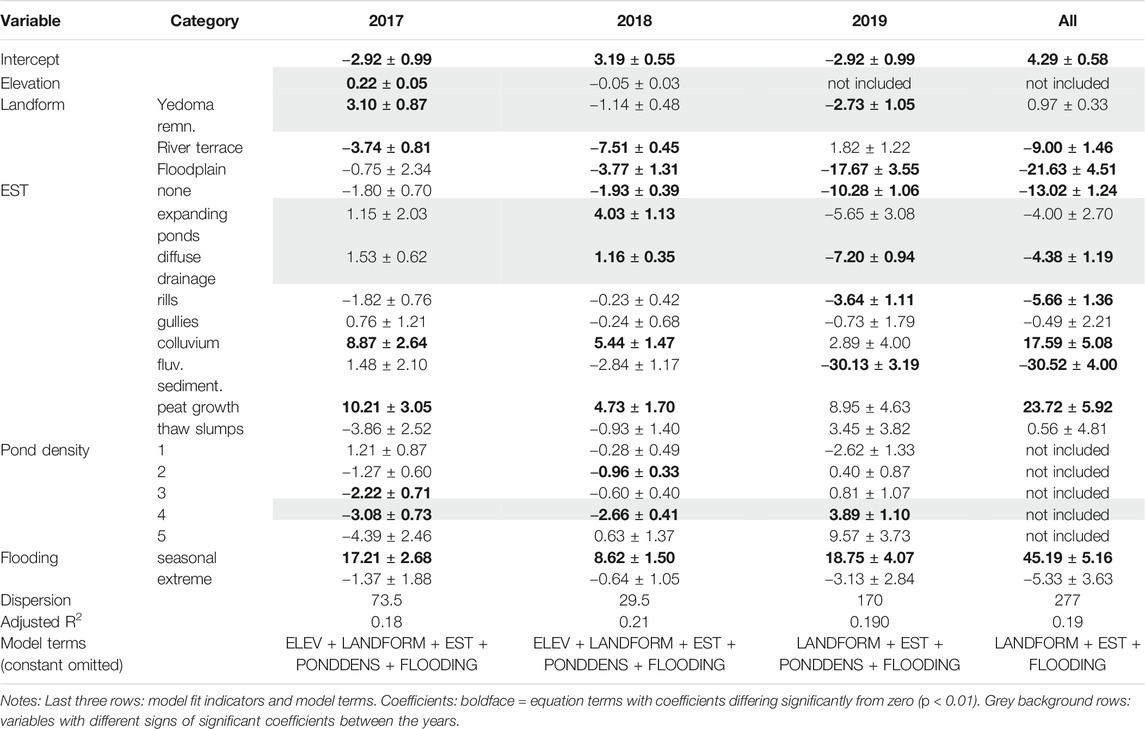
TABLE 4. General linear regression models of surface displacements with predictors elevation (ELEV), and categorical variables Landform group, EST (erosion/sedimentation/ground ice thaw), Pond density (PONDDENS) and Flooding.
For 2017 and 2019, all variables are retained by Akaike’s (Akaike, 1974) criterion; for 2019, ELEV was removed from the model (Table 2). For Σyears, the model is reduced to LANDFORM, EST and FLOODING variables; for 2017, the positive coefficient for ELEV is significant.
Within the Landform group, the DTLB class was taken as the reference variable. For 2017, the coefficient for LANDFORM-YR is positive, in agreement with the positive coefficient of ELEV in that year and the higher elevation of YR. In 2019 however, the YR term has a strongly negative coefficient. Of the other LANDFORM groups, the ARF and RT have negative coefficients, indicating subsidence relative to DTLB throughout all years.
The EST variable includes processes that can result either positive or negative surface displacements. The widely occurring class with evidence of ground ice thaw (mostly ice wedge thaw) was taken as reference here. Colluvial deposition on slopes and peat growth show pronounced positive coefficients for 2017 and 2018, in line with expected positive displacement for these classes. However, fluvial sedimentation mostly linked to floodplain locations has a pronounced negative coefficient in 2019, indicating net subsidence. Areas of expanding ponds and diffuse drainage, where negative surface displacements might be expected by ground ice thaw or erosion, have positive coefficients in 2018. Most classes have clearly negative coefficients in 2019, except for peat growth and colluvial deposition.
The visually estimated pond density (POND, class 0 absence of ponds as reference class) does not have a large and consistent effect. Pond density classes 2, 3 and 4 (low, intermediate, high) have negative coefficients in 2017 and 2018, indicating subsidence. However, in 2018 only class 4 contributes significantly with a positive coefficient. POND is removed from the regression equation for Σyears. The FLOOD variable (reference class No Flooding) is included for all years, with significant positive coefficients for the seasonally flooded class; the extreme flood class has all negative (but not significant) coefficients. Seasonally flooded points are all located in vegetated flood basins on the floodplain, whilst points reached by extreme floods only, are situated outside the floodplain but within the 2017 flood limits.
Discussion
Geomorphology and Late Quaternary Development
Figure 12 summarizes the landscape development of our study area that we deduced from our geomorphological and geological data. Drained thaw lake basins (55%) and the present river floodplain of the Berelegh river (39%) dominate the study area. We assume that the ice-rich Yedoma deposits have had a much wider extension but have been reworked by ground ice thaw and erosion. This is based on an erosion remnant (at 1 in Figure 4), and presence of Yedoma hills north of the study area.
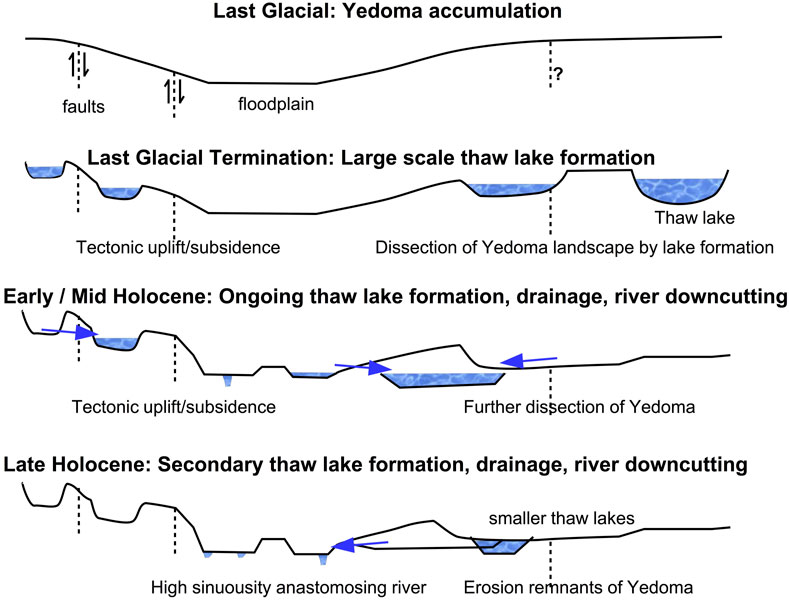
FIGURE 12. Schematic summary of the Late Glacial and Holocene landscape development along a hypothetical North-South topographical profile across the study area.
The original Yedoma surface had an elevation of 30–38 m above present sea level in the study area, sloping gently towards the river (Supplementary Figure S3). The erosion remnants at larger distance of 2 km do not indicate higher levels than 38 m, suggesting an essentially flat surface at distance from the river. The DTLB surfaces have an elevation between ±10 and ±14 m, indicating the removal of atleast 16 m of Yedoma deposits and ground ice over a large area, and subsequent redistribution in fluvial and lacustrine sediment. Remarkably, most of the Yedoma remnants occur at short distance from the river. A possible explanation that emerged from landscape-scale thaw lake modeling, is that expansion of thaw lakes close to rivers is restricted since the probability of early lake drainage is higher near the river, protecting Yedoma to large scale thaw lake erosion (Van Huissteden et al., 2011).
We assume that the RT unit is of the same age as the Yedoma surface. Its surface is higher by several meters (up to 20 m) than the highest DTLB surfaces (up to 14 m). Former fluvial channels are invisible on the terrace remnants, but may be obliterated by surface processes (sedimentation of loess, overbank river sediments, peat, or erosion).
Thaw lake formation started between at least 20,829 years BP (youngest 14C date on Yedoma deposits) and 8,016 years BP (oldest 14C on drained thaw lake basin fill) in the study area. In a recent analysis of Arctic-wide lake sediment basal dates (Brosius et al., 2021) two peaks in northern lake formation, including thaw lakes, were detected at 13,200 and 10,400 years ago, both following rapid increases in North Atlantic air temperature. This agrees well with the radiocarbon dates in our study area. The lake basin development in East Siberia is assumed to last until the end of the Boreal period (9.0–7.5 kyr BP, Romanovskii et al., 2004; Kaplina, 2009; Morgenstern et al., 2013).
Figures 4, 8 indicate the formation of large, elliptical to egg-shaped lake basin with smooth shores. This indicates the operation of wind-driven longshore currents during lake formation (Grosse et al., 2013), and a tendency to elongation in southerly direction. The overlapping relations of lake basins (Supplementary Figure S4) show that lake basin formation was well underway before the large, prominent lake basins in the center of Figure 4 have been formed. The lake basin at 3 in Figure 4 was drained before 8,016 years BP, but overlaps older lake bottoms (Supplementary Figure S4, right side of the basin). This suggests an early, Late Glacial start of widespread lake formation, similar to the East Siberian area described by Morgenstern et al. (2013). After drainage of this lake, thaw lake activity shifted to the north with the formation of a new large lake basin at a slightly lower level (at 4 in Figure 4), indicating that the development of lakes continued after ca 8,000 years BP. This lake received river sediment, as shown by the presence of a delta (Figure 7). The presence of an elevated central bulge in many lake basins is attributed to a longer duration of sediment accumulation in the centre of expanding lakes, compared to near-shore areas where subsidence and sedimentation began later, as demonstrated by modeling by Kessler et al. (2012).
Present actively expanding thaw lakes are small compared to these older drained lake basins. They are restricted to the older basins floors, but may expand rapidly into remaining Yedoma (at 5 in Figure 4). At 7 in Figure 4, a remnant lake, left after lake drainage, appears to have been rejuvenated and is expanding again. Thaw lake formation and expansion needs large amounts of ground ice in the subsurface (Jorgenson and Shur, 2007; Morgenstern et al., 2008; Morgenstern et al., 2013), and therefore old thaw lake basin floors are less susceptible to rapid lake expansion. In the older lake basin floors significant accumulation of ice wedges and ice-rich organic deposits has occurred (Teltewskoi et al., 2016; Weiss et al., 2016) but most of the ground ice volume is restricted to the upper meters as shown by deeper boreholes (See Results-Ice Content and Active Layer Depth).
After major thaw lake expansion, a small 1–2 m high terrace scarp was created between ARF and DTLB, likely by fluvial downcutting. The downcutting occurred after the formation of the DTLB at 4 in Figure 4. Before drainage of this basin, a delta existed in this lake, with sediment supply from the river towards the lake through a feeder channel (Figure 5). Presently, the drainage is reversed towards the river. Fluvial downcutting should have facilitated this reversal, and subsequent lake drainage and the northward extension of the channel into the drained basin. The age of this river downcutting is assumed to be younger than Mid Holocene age, based on the age of the DTLB’s.
On the ARF, several lakes have a morphology that suggest ground ice thaw as mechanism for their expansion: steep banks and rounded shapes, in particular bordering the RT unit. At 8 in Figure 4, thaw lakes developed within the river terrace. Some of these lakes are drained by the river channel during low flows (Figure 7, top), but others maintain a drainage threshold with the channel. Within the DTLB unit, lakes occur which appear to be thaw lakes that have been connected with the river quite recently, and drain during low stands (e.g. at 2A and 2B in Figure 4; see also Figure 7 at 1 and 2). During extreme floods, also lakes at larger distance from the floodplain have become connected to the river (at 3 in Figure 7 bottom).
On non-permafrost floodplains, lateral erosion is the primary mechanism for floodplain expansion (e.g. Howard, 1996). However, in our study area the capture of thaw lakes by subsidence and erosion of drainage thresholds may be induced by ground ice thaw during floods, representing a mechanism of floodplain expansion (see discussion of InSAR data). Capture of thaw lakes with subsequent avulsion of river channels through the former lakes may also be the cause of the irregular, patchy distribution of the river terrace (RT) unit on the floodplain, rather than the more common location of river terraces on valley sides. The height of the threshold between the lakes and the river system strongly affects river water input to the lakes, and as a consequence sediment and nutrient supply to the lake water (Marsh et al., 1999; Emmerton et al., 2007). Once connected to the floodplain, sedimentary infilling of these lakes may accelerate.
The compressional tectonic stress in the area has resulted in fault movements. In the DEM of Figure 3, two clear W-E trending lineaments can be discerned: the steep and nearly straight southern limit of the Berelegh floodplain, and a further terrain step of about 10 m to the south of it. Furthermore, there is a marked elevation difference between the Yedoma plateaus in the Allaikhovsky Highland (±50 m) to the south of the Berelegh river and the lower elevation of Yedoma plateau surfaces (30–38 m) north of the river. This suggests neotectonic movement along these lineaments, with a downward warping of the floodplain. On a North-South profile, the InSAR data indicate an average velocity of −5.3 ± 3.1 mm yr−1 north of the lineament bordering the Berelegh floodplain, south of this lineament −0.17 ± 3.2 mm yr−1, indicating a relative displacement of −3.6 mm yr−1. The elevation differences of 12–20 m, likely developed since the Late Glacial, results in an estimate of the tectonic subsidence rate of 1–2 mm yr−1. Further lineaments, with a less clear terrain expression, occur north of the Berelegh valley (Figure 3), but apparently did not result in a large amount of displacement.
Tectonic subsidence may have contributed to the extensive lake formation in our study area by impeding drainage and creating more flood-prone areas. Jorgenson and Shur (2007) invoke a large scale water balance change at the Last Glacial termination to explain the rapid expansion of large thaw lakes. In a poorly drained subsiding basin this should have had larger impact than in an area without subsidence.
Tectonic movements resulting in base level changes, as well as climate change resulting in the change of the drainage basin water balance and sediment supply are likely causes of river downcutting, and may have acted in concert. For the prominent downcutting phase that formed the RT river terrace water balance changes at the Last Glacial Termination are a likely cause, next to tectonism. Jorgenson and Shur (2007) cite evidence for water balance changes at the LGT that also initiated thaw lake formation in Alaska. Another mechanism of floodplain erosion is catastrophic drainage of large thaw lakes, which could create peak flows larger than normal spring floods (Marsh and Neumann, 2001; Marsh et al., 2009; Arp et al., 2020). This likely occurred repeatedly in the drainage basin of the Berelegh river given the large DLTB areas north of the river.
Surface Displacements Detected by InSAR
We did not consider a tectonic component in the InSAR data analysis over the study area of Figure 4, which is entirely north of the most active lineaments. The principal component analysis shows that variation among years explains 87% of the variance in the first two principal components (Supplementary Figure S6). The large contrast between the weather conditions in 2019 and 2017–2018 (Figure 2) causes most of the variability. Terrain variable Elevation appears in the third principal component, which explains 8% of the variance while the contribution of vegetation (NDVI) or its ponded water component (NDWI) is negligible.
This is likely the result of variation in weather conditions. The year 2019 was exceptionally warm and dry (Figure 2), with a warm winter, an early spring and warm summer. The year 2017 and 2018 had high precipitation during the preceding winter. The net summer precipitation in 2017–2018 showed a small precipitation deficit, without long periods of warming in summer. By contrast, 2019 had a large summer precipitation deficit, and prolonged periods of hot weather. The dominance of the surface displacements in 2019 in the first principal component (60% variance), indicate that in particular warm and dry weather conditions induce surface subsidence on a large scale.
Although the yearly weather conditions dominate, the trajectory clusters in Figure 11 show rather consistent spatial clustering, indicating that the terrain conditions contribute significantly to the spatial variation in surface displacements. This is also confirmed by the ANOVA and regression analysis. Weather conditions interact with terrain conditions. Several groups of weather and climate related processes could contribute to surface displacements:
1. Thaw or aggradation of ground ice (Liu et al., 2010; Liu et al., 2012; Liu et al., 2014), induced by changes in heat transport into the soil, by summer heat, a thick snow cover, change of surface vegetation, forest fire, flooding; e.g. Nauta et al., 2014; Liu et al., 2014; Jones et al., 2015; references in van Huissteden, 2020).
2. Shrinkage or swelling of soils on decrease or increase of soil water content. In particular fine-grained soils and peat are sensitive (e.g. Camporese et al., 2006; Succow and Joosten, 2012); this shrinkage/swelling can be detected by InSAR (Cuenca, 2008).
3. Surface displacements resulting from peat decomposition have been detected by InSAR (Cuenca, 2008). Decomposition of peat is affected by soil moisture and temperature (e.g. Succow and Joosten, 2012). The reverse process, peat accumulation, increases surface elevation. Sphagnum peat growth occurs widely in the study area, in particular within the DTLB unit (Magnússon et al., 2020).
4. Erosion and sedimentation are triggered by increased runoff and flooding; in permafrost areas the release of water by ground ice thaw on slopes triggers thaw slumps and active layer slides (Lewkowicz, 2007; Lewkowicz and Way, 2019).
The summer warmth and dryness in 2019 has contributed to significant surface subsidence compared to the previous years, for several of the clusters of data points in Figure 11, notably clusters. 1, 2 and 7. The regression analysis (Table 3) shows that in 2019 most categorical terrain variables have negative coefficients, indicating dominant subsidence. Most terrain classes show smaller positive coefficients, more negative coefficients, or reversals from positive to negative, notably the Yedoma landform class. A notable exception is Pond density class 4, of which the regression coefficient turns significantly positive in 2019, from negative in 2017–2018.
Both loss of ground ice and shrinkage of peat soils could have contributed to the pronounced surface subsidence in 2019. Trajectory clusters 1, 2 and to a lesser extent 7 all show pronounced subsidence in 2019 compared to 2017 and 2018. Clusters 1 and 2 are located in the large DLTB on the center-north of the study area (Figure 11), 7 in a neighboring area immediately to the south in an older DTLB. Both are areas with peat growth. Cluster 1 and 2 show low center polygons, cluster 7 represents an older DTLB area where polygons have been overgrown with peat (Figure 6). Shrinkage of peat during the dry summer therefore could have contributed to subsidence. However, small perennial frost mounds (without peat growth) in these clusters also show subsidence, indicating that ground ice thaw has occurred also.
Positive surface displacements in 2019 occur in cluster 6 and 10. Most of these points are areas with intermediate - high pond density and ice wedge polygons (cluster 6), or areas on the lower slopes of Yedoma remnants and higher ground in DTLB’s (cluster 10). The lower slopes of the YR may have been subject to colluvial deposition or downslope creep of soil material by increased water release from thawing permafrost in 2019. In 2017 and 2018, areas with potential colluvial deposition and peat growth also showed aggradation (Supplementary Figure S5 and Table 3). For areas with ponds in 2019, vegetation and Sphagnum peat growth may have been stimulated by decrease of water depth in ponds (Magnússon et al., 2020).
Positive surface displacements in 2017 correlate significantly with elevation (Table 1), the YR landform (Supplementary Figure S6 and Table 3) and trajectory cluster 4 (Figure 11). This is less clear in 2018, and in 2019 elevation correlates negatively with surface displacement. Pronounced positive surface displacement data points in 2017 are located on higher, well-drained parts of the Yedoma surface and DTLB surfaces. These areas have dry soils in summer with a thick active layer, consisting of silt and organic matter. The release of large amounts of meltwater from the thick snowpack after the winter of 2017, followed by a summer with relatively high amounts of precipitation (Figure 2) likely increased soil moisture. Downward movement of this additional water towards the transition layer at the top of the permafrost contributes to ice lens formation and frost heave in summer (Mackay, 1983). In addition, swelling of the soil by high moisture content could have contributed to the surface heave.
Seasonal flooding may contribute to sedimentation in floodplain environments, reflected by the positive regression coefficients for seasonal flooding in the regression data for all years in Table 4. However, this is probably a spurious effect; the number of points on seasonally flooded floodplain areas is very small.
The areas outside the floodplain that are reached by extreme floods only, show significant subsidence in 2017 and 2019 in the ANOVA results (Table 3), but the regression coefficients for extreme flooding in Table 4 remain just below the p = 0.01 significance level. The extreme flooding areas are represented by a low number of points, in clusters 4, 5, 9 and 10. Nevertheless, from Figure 9 a pronounced increase of summer thaw depth of dry soils that were flooded in 2017 and 2018 (transect 1, 2 and 3 in Figure 9) can be inferred at flooded locations. It is unlikely that the thaw depth recovers later in summer. Ponded water and wet soil conditions after flooding result in increased heat flux into the soil by changing the heat balance at the surface and increasing the soil thermal conductivity (e.g. Westermann et al., 2016), creating a thaw depth legacy of the flooding after flood recession. Nearby floodplain areas that are seasonally flooded have a thicker late summer ALT than non-flooded areas (van Huissteden et al., 2005; van Huissteden et al., 2009). Therefore, flooding is expected to increase ALT throughout the summer and fall with loss of ground ice (Figure 11), by heat transfer from flood water into the soil. However, this depends on soil conditions; wet soil with a Sphagnum peat cover was not affected (transect 4 in Figure 9).
Also for the RT landform unit, subsidence is pronounced (Figures 10, 11). During extreme floods, the RT are small isolated islands on the river floodplain surrounded by water (Figure 7). Prolonged flooding in 2017, 2018 likely adds to heat transfer in this unit.
Given the absence of river gaging data, it is difficult to specify a flood return period for the high floods of 2017 and 2018. However, the low terrace scarp bordering the floodplain, which limits most floods, was exceeded over a large distance in 2017 and 2018. Downstream in the Indigirka river, the return period of the 2017 flood level was estimated at ≥49 years (Tei et al., 2020). A study of Landsat images of the study area during the spring flood period dating back to 1999 did not show any higher flood limits than the 2017 flood (Cheung, 2019).
A higher frequency of extreme floods likely induces subsidence at ice-rich floodplain borders, making these areas in turn more flood-prone. Additionally, subsidence of drainage thresholds could facilitate the connection of thaw lakes and DTLB areas to the floodplain. The sites numbered 3 in Figure 7 are critical areas in that respect. For the westernmost point, the drainage threshold was just not exceeded in 2017, for the easternmost point, the flood invaded two lakes in a large DTLB, and a channel connecting these lakes towards the floodplain is forming. We hypothesize that ground ice thaw by an increase of flood extremes will expand floodplains in ice-rich permafrost. A future increase of flood height and flooding frequency therefore may have a large impact on floodplain development in ice-rich permafrost floodplains (Shkolknik et al., 2017).
So far, we have only considered thaw season surface displacement processes. However, as Supplementary Figures S1, S2 show, surface subsidence is compensated by spatially varying heave in winter. This is attributed to ice lens growth, determined by local water availability in the active layer. Points with strong subsidence in one or more thaw seasons may have gained in height overall years when winter heave is included. Overall, net subsidence dominates in the study area over 2017–2019, but there are marked areas where net heave occurred.
For instance, in the relatively young drained thaw lake basin in the north-central area of the study area, a cluster of points occurs with pronounced subsidence in 2019, resulting in considerable cumulative subsidence over the thaw season (Figure 11 cluster 1, 2, Figure 10), but the net effect of winter heave and summer subsidence over 3 years is substantial heave. (Supplementary Figure S2). This is an area of active Sphagnum peat growth, with a high soil water content. Also the Yedoma surfaces show net heave over the 3 years, which is strongest in 2017 (cluster 3, 4 and 8 in Figure 11). This is mainly driven by soil water accumulation in 2017 as discussed above.
Pingos that were visited in the study area do not show any recent growth activity. Compared to normal DTLB surfaces, pingos tend to subside a few mm over the years in the thaw season (t-test, p < 0.01) and also on average over the 3 years, which is like the result of slope processes and loss of ice.
Carbon Cycle Implications
The InSAR data presented here do not allow estimates on the carbon loss or greenhouse gas emissions from thawing permafrost, since this would require quantitative data on soil moisture conditions, active layer depth and carbon content of the soil. However, general trends can be inferred:
1. Increased flooding enhances ground ice thaw and active layer depth along floodplain limits and may expand floodplains. This enhances CH4 emission (van Huissteden et al., 2005; van Huissteden et al., 2009), by exposing old permafrost carbon to anaerobic decomposition, and potentially by creating a more productive wetland vegetation through nutrient supply by flood water. Carbon emission in floodplain environments is fueled by higher ecosystem productivity of floodplains; most of the released fluvial-derived carbon is of recent origin (Dean et al., 2020). However, floodplain subsidence and capture of thaw lakes also creates storage space for burial and sequestration of organic-rich sediments (Emmerton et al., 2007; Van Huissteden et al., 2013; Vonk et al., 2015). In this location a sedimentation rate of organic-rich silt of 0.5 cm year−1 was recorded in a borehole. However, tectonic subsidence also contributes to the sedimentation rate.
2. Outside floodplain areas, warm or wet summer conditions contribute to thaw of ice-rich permafrost, subsidence and enhanced exposure of permafrost organic matter to aerobic or anaerobic decomposition (e.g. Dorrepaal et al., 2009; Natali et al., 2015). However, the net effect on carbon loss and the ratio between CO2 and CH4 emission is not straightforward, since multiple interactions on various time scales between vegetation primary production, nutrient liberation by permafrost carbon decomposition and soil water content occur (Natali et al., 2015). Subsidence therefore cannot be used as a proxy for carbon loss.
3. Wet soil conditions enhance subsidence, as shown by increased subsidence in 2017–2018 in areas with intermediate-high pond density. On the other hand, during the warm/dry year the well-drained Yedoma was more prone to subsidence, and areas with high pond density showed positive surface displacement, probably linked to enhanced vegetation growth/peat accumulation (see at 3 below). Thaw ponds in the DTLB unit in this area release mostly contemporary CH4 and CO2 carbon from recent vegetation and soil (Nauta et al., 2014; Dean et al., 2020). This indicates that release of old carbon is minor. However, the loss of recent vegetation and soil carbon by pond formation may be substantial, and the release of CH4 decreases the greenhouse gas sink or increases the net greenhouse gas emission of the area. Greenhouse gas emission of ponds is partly compensated by recolonization of ponds by vegetation (Magnússon et al., 2020); see also previous section).
4. Some of the areas where peat formation by Sphagnum mosses occurs, showed pronounced subsidence in dry and warm 2019. This suggest dryer conditions by shrinkage and/or ground ice thaw. Dry conditions may limit peat growth and its carbon sequestration.
5. Both warm and wet years enhance mass wasting and colluviation on slopes of Yedoma landforms. Mass wasting by thaw slumps, active layer slides and gully erosion exposes permafrost and vegetation carbon to decomposition; sedimentation of mass wasted material and colluviation buries soil and vegetation carbon (Grosse et al., 2011; Abbott and Jones, 2015).
Conclusion
In our study area, erosion remnants of a paleo-surface of the Glacial Yedoma landscape testify of the former morphology of this landscape. The Yedoma surface extended with gentle slopes into the present river floodplain, where an old terrace level testifies of the floodplain surface at that time. The fluvial downcutting that created this terrace could relate to tectonic movements and/or change of the water balance of the drainage basin.
The formation of thaw lakes during the Late Glacial and Early Holocene eroded most of the Yedoma deposits, leaving only minor erosion remnants near the river floodplain. Probably formation of large thaw lakes was enhanced by tectonic subsidence. The Yedoma remnants were preserved preferentially near the river floodplain, because drainage by fluvial erosion limited the growth of thaw lakes. Catastrophic lake drainage could have contributed to floodplain erosion.
The potential for short-term geomorphological changes is shown by the InSAR surface displacement data. These data, and midsummer active layer thickness data collected after the floods of 2017 and 2018, show that heat transfer into the permafrost by flooding during high flood stages results in subsidence along floodplain margins, lowering drainage thresholds of thaw lakes near the river. We hypothesize that flood-induced subsidence is an important mechanism for floodplain expansion if future climate change increases spring flood heights.
Variability between the observation years contributes most to the variance of the InSAR data, but interacts with the local terrain characteristics. Surface displacement patterns differ considerably between the wet years 2017 and 2018, and the warm and dry year 2019. In general, during the wet years well-drained, elevated terrain appears less sensitive to subsidence by ground ice thaw and even may show heave. During the warm and drier year 2019, overall subsidence is more prominent.
Subsidence or heave are generally caused by changes in the ice volume at the top of the permafrost. Increased soil water availability in wet years contributes to gain of ice at the top of the permafrost, in particular at relatively dry sites. However, variations in soil moisture content also may contribute to surface displacement by shrinking or swelling of peat and fine-grained Yedoma soils. Peat growth and colluvial deposition on lower slopes result in positive surface displacement. However, detailed observations on changes in ice content and sedimentation are necessary to constrain contribution of each of these processes.
The relation between subsidence and the carbon cycle is not simple because of the many soil - vegetation interactions on various time scales. Combined with water ponding, subsidence increases CH4 emission. Carbon cycle implications of floodplain expansion are the creation of a larger storage space for organic-rich fluvial sedimentation, but also expansion of areas with a high CH4 emission.
Data Availability Statement
The raw data supporting the conclusion of this article will be made available by the authors, without undue reservation.
Author Contributions
JH did the geomorphological interpretation of the satellite images and field data, the statistical analysis and wrote most of the text. KT analyzed the radar images and computed the InSAR data. YC analyzed river flood levels from older Landsat image data, the data were provided by NLR and her work was supervised by HN. RM participated in fieldwork, discussions and provided a part of high resolution satellite image data. SK participated in fieldwork organization and data collection. TM and AD supervised fieldwork organization and contributed to discussion of the research results.
Funding
This research was financed by the User Support Program Space Research of the Netherlands Space Office, Grant No. ALW-GO/16-13, “Radar mapping of permafrost degradation and methane emission hotspots”, and by the Netherlands Polar Program of the Dutch Research Council (NWO), grant number ALWPP.2016.008. The geomorphological work was initiated and funded by the PAGE21 (Changing Permafrost in the Arctic and its Global Effects in the 21st Century) Large-scale integrating project EU FP7-ENV-2011 Grant Nr 282700.
Conflict of Interest
The authors declare that the research was conducted in the absence of any commercial or financial relationships that could be construed as a potential conflict of interest.
Publisher’s Note
All claims expressed in this article are solely those of the authors and do not necessarily represent those of their affiliated organizations, or those of the publisher, the editors and the reviewers. Any product that may be evaluated in this article, or claim that may be made by its manufacturer, is not guaranteed or endorsed by the publisher.
Acknowledgments
The authors acknowledge the European Space Agency (ESA) for Sentinel-1 images used in this study. We thank the crew of the Sakha Republic Nature Conservancy and Fisheries Inspection at Chokurdagh for their valuable support in the field. ERA5 reanalysis data have been provided under the terms of the Copernicus Products License Agreement version 1.2.
Supplementary Material
The Supplementary Material for this article can be found online at: https://www.frontiersin.org/articles/10.3389/feart.2021.680565/full#supplementary-material
References
Abbott, B. W., and Jones, J. B. (2015). Permafrost Collapse Alters Soil Carbon Stocks, Respiration, CH4, and N2O in upland Tundra. Glob. Change Biol. 21 (12), 4570–4587. doi:10.1111/gcb.13069
Akaike, H. (1974). A New Look at the Statistical Model Identification. IEEE Trans. Automat. Contr., 19 (6):716–723. doi:10.1109/TAC.1974.1100705
Akhmetiev, M. A. (2015). High-latitude Regions of Siberia and Northeast Russia in the Paleogene: Stratigraphy, flora, Climate, Coal Accumulation. Stratigr. Geol. Correl. 23 (4), 421–435. doi:10.1134/s0869593815040024
Amap, (2017). “Snow,Water,Ice and Permafrost in the Arctic (SWIPA) 2017,” in Arctic Monitoring and Assessment Programme (AMAP). Oslo, Norway, 269.
Anisimov, O., and Nelson, F. (1997). Influence of Climate Change on Permafrost in the Northern Hemisphere. Russ. Meteorology Hydrol. 5, 47–53.
Arp, C. D., Jones, B. M., Hinkel, K. M., Kane, D. L., Whitman, M. S., and Kemnitz, R. (2020). Recurring Outburst Floods from Drained Lakes: an Emerging Arctic hazard. Front. Ecol. Environ. 18 (7), 384–390. doi:10.1002/fee.2175
Beermann, F., Teltewskoi, A., Fiencke, C., Pfeiffer, E-M., and Kutzbach, L. (2015). Stoichiometric Analysis of Nutrient Availability (N, P, K) within Soils of Polygonal Tundra. Biogeochemistry 122 (2-3), 211–227. doi:10.1007/s10533-014-0037-4
Blok, D., Heijmans, M. M. P. D., Schaepman-strub, G., Kononov, A. V., Maximov, T. C., and Berendse, F. (2010). Shrub Expansion May Reduce Summer Permafrost Thaw in Siberian Tundra. Glob. Change Biol. 16 (4), 1296–1305. doi:10.1111/j.1365-2486.2009.02110.x
Brosius, L. S., Anthony, K. M. W., Treat, C. C., Lenz, J., Jones, M. C., Bret-Harte, M. S., et al. (2021). Spatiotemporal Patterns of Northern lake Formation since the Last Glacial Maximum. Quat. Sci. Rev. 253, 106773. doi:10.1016/j.quascirev.2020.106773
Camporese, M., Ferraris, S., Putti, M., Salandin, P., and Teatini, P. (2006). Hydrological Modeling in Swelling/shrinking Peat Soils. Water Resour. Res. 42 (6). doi:10.1029/2005wr004495
Chen, C. W., and Zebker, H. A. (2002). Phase Unwrapping for Large SAR Interferograms: Statistical Segmentation and Generalized Network Models. IEEE Trans. Geosci. Remote Sensing 40 (8), 1709–1719. doi:10.1109/tgrs.2002.802453
Cheung, Y. (2019). Siberian Permafrost. A Temporal and Spatial Analysis on Flooding Frequency and Thaw Lakes. Amsterdam: Vrije Universteit Faculty os Science/HAS University of Applied Sciences/NLR.
Cuenca, M. C. (2008). “Hanssen R Subsidence Due to Peat Decomposition in the Netherlands, Kinematic Observations from Radar Interferometry,” in Proc. Fringe 2007 Workshop, 1–6.
Dean, J. F., Meisel, O. H., Martyn Rosco, M., Marchesini, L. B., Garnett, M. H., Lenderink, H., et al. (2020). East Siberian Arctic Inland Waters Emit Mostly Contemporary Carbon. Nat. Commun. 11 (1), 1627. doi:10.1038/s41467-020-15511-6
Dorrepaal, E., Toet, S., van Logtestijn, R. S. P., Swart, E., van de Weg, M. J., Callaghan, T. V., et al. (2009). Carbon Respiration from Subsurface Peat Accelerated by Climate Warming in the Subarctic. Nature 460 (7255), 616–619. doi:10.1038/nature08216
Drachev, S. S. (2016). Fold Belts and Sedimentary Basins of the Eurasian Arctic. arktos 2 (1), 21. doi:10.1007/s41063-015-0014-8
Emmerton, C. A., Lesack, L. F., and Marsh, P. (2007). Lake Abundance, Potential Water Storage, and Habitat Distribution in the Mackenzie River Delta, Western Canadian Arctic. Water Resour. Res. 43 (5). doi:10.1029/2006wr005139
Ferretti, A., Prati, C., and Rocca, F. (2001). Permanent Scatterers in SAR Interferometry. IEEE Trans. Geosci. Remote Sensing 39 (1), 8–20. doi:10.1109/36.898661
Fujita, K., Koz'min, B. M., Mackey, K. G., Riegel, S. A., McLean, M. S., and Imaev, V. S. (2009). 4. Göttingen, Germany: Copernicus Publications, 117–145. doi:10.5194/smsps-4-117-2009Seismotectonics of the Chersky Seismic Belt, Eastern Sakha Republic (Yakutia) and Magadan District, RussiaStephan Mueller Spec. Publ. Ser.
Grosse, G., Harden, J., Turetsky, M., McGuire, A. D., Camill, P., Tarnocai, C., et al. (2011). Vulnerability of High-Latitude Soil Organic Carbon in North America to Disturbance. J. Geophys. Res. 116. doi:10.1029/2010jg001507
Grosse, G., Jones, B., and Arp, C. (2013). “8.21 Thermokarst Lakes, Drainage, and Drained Basins,” in Treatise on Geomorphology Editors J. Shroder, R. Giardino, and J. Harbor (San Diego, CA: Academic Press) 8, 325–353. doi:10.1016/b978-0-12-374739-6.00216-5
Hersbach, H., Bell, B., Berrisford, P., Hirahara, S., Horányi, A., Muñoz‐Sabater, J., et al. (2020). The ERA5 Global Reanalysis. Q.J.R. Meteorol. Soc. 146 (730), 1999–2049. doi:10.1002/qj.3803
Hinkel, K. M., Eisner, W. R., Bockheim, J. G., Nelson, F. E., Peterson, K. M., and Dai, X. (2003). Spatial Extent, Age, and Carbon Stocks in Drained Thaw lake Basins on the Barrow Peninsula, Alaska. Arctic, Antarctic, Alpine Res. 35 (3), 291–300. doi:10.1657/1523-0430(2003)035[0291:seaacs]2.0.co;2
Hjort, J., Karjalainen, O., Aalto, J., Westermann, S., Romanovsky, V. E., Nelson, F. E., et al. (2018). Degrading Permafrost Puts Arctic Infrastructure at Risk by Mid-century. Nat. Commun. 9 (1), 5147. doi:10.1038/s41467-018-07557-4
Hooper, A. (2008). A Multi-Temporal InSAR Method Incorporating Both Persistent Scatterer and Small Baseline Approaches. Geophys. Res. Lett. 35. doi:10.1029/2008GL034654
Howard, A. D. (1996). “Modeling Channel Evolution and Floodplain Morphology,” in Floodplain Processes. Editors M Anderson, E Walling, and P. D Bates (Chichester: Wiley), 15–62.
Imaeva, L. P., Imaev, V. S., and Koz’min, B. M. (2016a). Structural-dynamic Model of the Chersky Seismotectonic Zone (continental Part of the Arctic-Asian Seismic belt). J. Asian Earth Sci. 116, 59–68. doi:10.1016/j.jseaes.2015.11.010
Imaeva, L. P., Imaev, V. S., Mel’nikova, V. I., and Koz’min, B. M. (2016b). Recent Structures and Tectonic Regimes of the Stress-Strain State of the Earth's Crust in the Northeastern Sector of the Russian Arctic Region. Geotecton. 50 (6), 535–552. doi:10.1134/s0016852116060030
IPCC (2013). Climate Change 2013: The Physical Science Basis. Contribution of Working Group I to the Fifth Assessment Report of the Intergovernmental Panel on Climate Change. Cambridge University Press. doi:10.1017/CBO9781107415324
Jones, B. M., Grosse, G., Arp, C. D., Miller, E., Liu, L., Hayes, D. J., et al. (2015). Recent Arctic Tundra Fire Initiates Widespread Thermokarst Development. Sci. Rep. 5, 15865. doi:10.1038/srep15865
Jorgenson, M. T., and Shur, Y. (2007). Evolution of Lakes and Basins in Northern Alaska and Discussion of the Thaw lake Cycle. J. Geophys. Res. Earth Surf. 112 (F2). doi:10.1029/2006jf000531
Jorgenson, M. T., Romanovsky, V., Harden, J., Shur, Y., O’Donnell, J., Schuur, E. A. G., et al. (2010). Resilience and Vulnerability of Permafrost to Climate changeThis Article Is One of a Selection of Papers from the Dynamics of Change in Alaska's Boreal Forests: Resilience and Vulnerability in Response to Climate Warming. Can. J. For. Res. 40 (7), 1219–1236. doi:10.1139/x10-060
Juszak, I., Erb, A. M., Maximov, T. C., and Schaepman-Strub, G. (2014). Arctic Shrub Effects on NDVI, Summer Albedo and Soil Shading. Remote sensing Environ. 153, 79–89. doi:10.1016/j.rse.2014.07.021
Juszak, I., Eugster, W., Heijmans, M. M. P. D., and Schaepman-Strub, G. (2016). Contrasting Radiation and Soil Heat Fluxes in Arctic Shrub and Wet Sedge Tundra. Biogeosciences 13, 4049–4064. doi:10.5194/bg-13-4049-2016
Juszak, I., Iturrate-Garcia, M., Gastellu-Etchegorry, J.-P., Schaepman, M. E., Maximov, T. C., and Schaepman-Strub, G. (2017). Drivers of Shortwave Radiation Fluxes in Arctic Tundra across Scales. Remote sensing Environ. 193, 86–102. doi:10.1016/j.rse.2017.02.017
Kanevskiy, M., Shur, Y., Jorgenson, M. T., Ping, C.-L., Michaelson, G. J., Fortier, D., et al. (2013). Ground Ice in the Upper Permafrost of the Beaufort Sea Coast of Alaska. Cold Regions Sci. Tech. 85, 56–70. doi:10.1016/j.coldregions.2012.08.002
Kanevskiy, M., Shur, Y., Jorgenson, T., Brown, D. R. N., Moskalenko, N., Brown, J., et al. (2017). Degradation and Stabilization of Ice Wedges: Implications for Assessing Risk of Thermokarst in Northern Alaska. Geomorphology 297, 20–42. doi:10.1016/j.geomorph.2017.09.001
Kaplina, T. N. (2009). Alasnye Komplexy Severnoy Yakutii (Alas Complex of Northern Yakutia). Kriosfera Zemli (Earth Cryosphere) 13, 3–17.
Kessler, M., and Plug, L. J., and Walter Anthony, K. M. (2012). Simulating the Decadal‐to Millennial‐scale Dynamics of Morphology and Sequestered Carbon Mobilization of Two Thermokarst Lakes in NW Alaska. J. Geophys. Res. Biogeosciences 117 (G2). doi:10.1029/2011jg001796
Lewkowicz, A. G. (2007). Dynamics of Active-Layer Detachment Failures, Fosheim Peninsula, Ellesmere Island, Nunavut, Canada. Permafrost Periglac. Process. 18 (1), 89–103. doi:10.1002/ppp.578
Lewkowicz, A. G., and Way, R. G. (2019). Extremes of Summer Climate Trigger Thousands of Thermokarst Landslides in a High Arctic Environment. Nat. Commun. 10 (1), 1329. doi:10.1038/s41467-019-09314-7
Li, B., Heijmans, M. M., Blok, D., Wang, P., Karsanaev, S. V., Maximov, T. C., et al. (2017). Thaw Pond Development and Initial Vegetation Succession in Experimental Plots at a Siberian lowland Tundra Site. Plant and Soil 420 (1-2), 147–162. doi:10.1007/s11104-017-3369-8
Liu, L., Schaefer, K., Zhang, T., and Wahr, J. (2012). Estimating 1992–2000 Average Active Layer Thickness on the Alaskan North Slope from Remotely Sensed Surface Subsidence. J. Geophys. Res. Earth Surf. 117 (F1). doi:10.1029/2011jf002041
Liu, L., Zhang, T., and Wahr, J. (2010). InSAR Measurements of Surface Deformation over Permafrost on the North Slope of Alaska. J. Geophys. Res. Earth Surf. 115 (F3). doi:10.1029/2009jf001547
Liu, L., Jafarov, E. E., Schaefer, K. M., Jones, B. M., Zebker, H. A., Williams, C. A., et al. (2014). InSAR Detects Increase in Surface Subsidence Caused by an Arctic Tundra Fire. Geophys. Res. Lett. 41 (11), 3906–3913. doi:10.1002/2014gl060533
Mackay, J. R. (1983). Downward Water Movement into Frozen Ground, Western Arctic Coast, Canada. Can. J. Earth Sci. 20, 120–134. doi:10.1139/e83-012
Mackay, J. R. (1998). Pingo Growth and Collapse, Tuktoyaktuk Peninsula Area, Western Arctic Coast, Canada: A Long-Term Field Study. Géographie physique et Quaternaire 52 (3), 271–323.
Magnússon, R. Í., Limpens, J., Huissteden, J., Kleijn, D., Maximov, T. C., Rotbarth, R., et al. (2020). Rapid Vegetation Succession and Coupled Permafrost Dynamics in Arctic Thaw Ponds in the Siberian Lowland Tundra. J. Geophys. Res. Biogeosci. 125 (7), e2019JG005618. doi:10.1029/2019jg005618
Marsh, P., Lesack, L. F. W., and Roberts, A. (1999). Lake Sedimentation in the Mackenzie Delta, NWT. Hydrol. Process. 13 (16), 2519–2536. doi:10.1002/(sici)1099-1085(199911)13:16<2519::aid-hyp935>3.0.co;2-t
Marsh, P., and Neumann, N. N. (2001). Processes Controlling the Rapid Drainage of Two Ice-Rich Permafrost-Dammed Lakes in NW Canada. Hydrol. Process. 15 (18), 3433–3446. doi:10.1002/hyp.1035
Marsh, P., Russell, M., Pohl, S., Haywood, H., and Onclin, C. (2009). Changes in Thaw lake Drainage in the Western Canadian Arctic from 1950 to 2000. Hydrol. Process. 23 (1), 145–158. doi:10.1002/hyp.7179
McFeeters, S. K. (1996). The Use of the Normalized Difference Water Index (NDWI) in the Delineation of Open Water Features. Int. J. remote sensing 17 (7), 1425–1432. doi:10.1080/01431169608948714
Morgenstern, A., Grosse, G., and Schirrmeister, L. (2008). Genetic, Morphological, and Statistical Characterization of Lakes in the Permafrost-Dominated Lena Delta.
Morgenstern, A., Ulrich, M., Günther, F., Roessler, S., Fedorova, I. V., Rudaya, N. A., et al. (2013). Evolution of Thermokarst in East Siberian Ice-Rich Permafrost: A Case Study. Geomorphology 201, 363–379. doi:10.1016/j.geomorph.2013.07.011
Murton, J. B., Goslar, T., Edwards, M. E., Bateman, M. D., Danilov, P. P., Savvinov, G. N., et al. (2015). Palaeoenvironmental Interpretation of Yedoma Silt (Ice Complex) Deposition as Cold-Climate Loess, Duvanny Yar, Northeast Siberia. Permafrost Periglac. Process. 26 (3), 208–288. doi:10.1002/ppp.1843
Natali, S. M., Schuur, E. A. G., Mauritz, M., Schade, J. D., Celis, G., Crummer, K. G., et al. (2015). Permafrost Thaw and Soil Moisture Driving CO 2 and CH 4 Release from upland Tundra. J. Geophys. Res. Biogeosci. 120 (3), 525–537. doi:10.1002/2014jg002872
Nauta, A. L., Heijmans, M. M. P. D., Blok, D., Limpens, J., Elberling, B., Gallagher, A., et al. (2014). Permafrost Collapse after Shrub Removal Shifts Tundra Ecosystem to a Methane Source. Nat. Clim Change 5 (1), 67–70. doi:10.1038/nclimate2446
Nelson, F. E., Anisimov, O. A., and Shiklomanov, N. I. (2002). Climate Change and hazard Zonation in the Circum-Arctic Permafrost Regions. Nat. Hazards 26 (3), 203–225. doi:10.1023/a:1015612918401
NOAA (2020). Gobal Summary of the Day. Available at: https://www.ncei.noaa.gov/access/search/data-search/global-summary-of-the-day (Accessed July, , 2020).
Parmentier, F., Van Der Molen, M., Van Huissteden, J., Karsanaev, S., Kononov, A., Suzdalov, D., et al. (2011b). Longer Growing Seasons Do Not Increase Net Carbon Uptake in the Northeastern Siberian Tundra. J. Geophys. Res. Biogeosciences 116 (G4). doi:10.1029/2011jg001653
Parmentier, F. J. W., van Huissteden, J., Kip, N., Op den Camp, H. J. M., Jetten, M. S. M., Maximov, T. C., et al. (2011c). The Role of Endophytic Methane-Oxidizing Bacteria in Submerged Sphagnum in Determining Methane Emissions of Northeastern Siberian Tundra. Biogeosciences 8 (5), 1267–1278. doi:10.5194/bg-8-1267-2011
Parmentier, F. J. W., van Huissteden, J., van der Molen, M. K., Schaepman-Strub, G., Karsanaev, S. A., Maximov, T. C., et al. (2011a). Spatial and Temporal Dynamics in Eddy Covariance Observations of Methane Fluxes at a Tundra Site in Northeastern Siberia. J. Geophys. Res. 116 (G3). doi:10.1029/2010jg001637
Ren, J., Niu, B., Wang, J., Jin, X., and Xie, L. (2013). 1: 5000000 International Geological Map of Asia. Beijing: Geological Publishing House.,
Romanovskii, N., Hubberten, H-W., Gavrilov, A., Tumskoy, V., and Kholodov, A. (2004). Permafrost of the East Siberian Arctic Shelf and Coastal Lowlands. Quat. Sci. Rev. 23 (11-13), 1359–1369. doi:10.1016/j.quascirev.2003.12.014
Schirrmeister, L., Pestryakova, L., Wetterich, S., and Tumskoy, V. (2011). Joint Russian-German Polygon Project East Siberia 2011-2014: The Expedition Kytalyk 2011AWI, Potsdam/Yakutsk.
Schirrmeister, L., Froese, D., Tumskoy, V., Grosse, G., and Wetterich, S. (2013). Encyclopedia of Quaternary Science. 2nd edition. Elsevier, 542–552. doi:10.1016/b978-0-444-53643-3.00106-0PERMAFROST and PERIGLACIAL FEATURES | Yedoma: Late Pleistocene Ice-Rich Syngenetic Permafrost of Beringia
Schneider von Deimling, T., Grosse, G., Strauss, J., Schirrmeister, L., Morgenstern, A., Schaphoff, S., et al. (2015). Observation-based Modelling of Permafrost Carbon Fluxes with Accounting for Deep Carbon Deposits and Thermokarst Activity. Biogeosciences 12 (11), 3469–3488. doi:10.5194/bg-12-3469-2015
Shiklomanov, N. I., Streletskiy, D. A., Swales, T. B., and Kokorev, V. A. (2017). Climate Change and Stability of Urban Infrastructure in Russian Permafrost Regions: Prognostic Assessment Based on GCM Climate Projections. Geographical Rev. 107 (1), 125–142. doi:10.1111/gere.12214
Shkolnik, I., Pavlova, T., Efimov, S., and Zhuravlev, S. (2017). Future Changes in Peak River Flows across Northern Eurasia as Inferred from an Ensemble of Regional Climate Projections under the IPCC RCP8.5 Scenario. Clim. Dyn. 50, 215–230. doi:10.1007/s00382-017-3600-6
Shmelev, D., Veremeeva, A., Kraev, G., Kholodov, A., Spencer, R. G. M., Walker, W. S., et al. (2017). Estimation and Sensitivity of Carbon Storage in Permafrost of North-Eastern Yakutia. Permafrost Periglac. Process. 28 (2), 379–390. doi:10.1002/ppp.1933
Strauss, J., Schirrmeister, L., Grosse, G., Fortier, D., Hugelius, G., Knoblauch, C., et al. (2017). Deep Yedoma Permafrost: A Synthesis of Depositional Characteristics and Carbon Vulnerability. Earth-Science Rev. 172, 75–86. doi:10.1016/j.earscirev.2017.07.007
Tadono, T., Ishida, H., Oda, F., Naito, S., Minakawa, K., and Iwamoto, H. (2014). Precise Global DEM Generation by ALOS PRISM. ISPRS Ann. Photogramm. Remote Sens. Spat. Inf. Sci. II-4 (4), 71–76. doi:10.5194/isprsannals-ii-4-71-2014
Takaku, J., Tadono, T., Tsutsui, K., and Ichikawa, M. (2016). Validation of "Aw3D" Global Dsm Generated from Alos Prism. ISPRS Ann. Photogramm. Remote Sens. Spat. Inf. Sci. III-4, 25–31. doi:10.5194/isprsannals-iii-4-25-2016
Tei, S., Morozumi, T., Nagai, S., Takano, S., Sugimoto, A., Shingubara, R., et al. (2020). An Extreme Flood Caused by a Heavy Snowfall over the Indigirka River basin in Northeastern Siberia. Hydrological Process. 34 (3), 522–537. doi:10.1002/hyp.13601
Teltewskoi, A., Beermann, F., Beil, I., Bobrov, A., De Klerk, P., Lorenz, S., et al. (2016). 4000 Years of Changing Wetness in a Permafrost Polygon Peatland (Kytalyk, NE Siberia): A Comparative High-Resolution Multi-Proxy Study. Permafrost Periglac. Process. 27 (1), 76–95. doi:10.1002/ppp.1869
Teshebaeva, K., van Huissteden, K. J., Puzanov, A. V., Balykin, D. N., Sinitsky, A. I., and Kovalevskaya, N. (2020). Permafrost Seasonal Surface Changes Revealed from Sentinel-1 InSAR Time-Series, Yamal peninsula. Proc. IAHS 382, 183–187. doi:10.5194/piahs-382-183-2020
Trouet, V., and Van Oldenborgh, G. J. (2013). KNMI Climate Explorer: a Web-Based Research Tool for High-Resolution Paleoclimatology. Tree-Ring Res. 69 (1), 3–13. doi:10.3959/1536-1098-69.1.3
Turetsky, M. R., Abbott, B. W., Jones, M. C., Walter Anthony, K., Olefeldt, D., Schuur, E. A. G., et al. (2019). Permafrost Collapse Is Accelerating Carbon Release. Nature 569, 32–34. doi:10.1038/d41586-019-01313-4
Ulrich, M., Grosse, G., Strauss, J., and Schirrmeister, L. (2014). Quantifying Wedge-Ice Volumes in Yedoma and Thermokarst Basin Deposits. Permafrost Periglac. Process. 25 (3), 151–161. doi:10.1002/ppp.1810
Van der Molen, M. K., Van Huissteden, J., Parmentier, F. J. W., Petrescu, A. M. R., Dolman, A. J., Maximov, T. C., et al. (2007). The Growing Season Greenhouse Gas Balance of a continental Tundra Site in the Indigirka Lowlands, NE Siberia. Biogeosciences 4 (6), 985–1003. doi:10.5194/bg-4-985-2007
Van Huissteden, J., Berrittella, C., Parmentier, F. J. W., Mi, Y., Maximov, T. C., and Dolman, A. J. (2011). Methane Emissions from Permafrost Thaw Lakes Limited by lake Drainage. Nat. Clim Change 1 (2), 119–123. doi:10.1038/nclimate1101
van Huissteden, J., Maximov, T. C., and Dolman, A. J. (2009). Correction to "High Methane Flux from an Arctic Floodplain (Indigirka Lowlands, Eastern Siberia)". J. Geophys. Res. 114, a–n. doi:10.1029/2009JG001040
van Huissteden, J., Maximov, T. C., and Dolman, A. J. (2005). High Methane Flux from an Arctic Floodplain (Indigirka Lowlands, Eastern Siberia). J. Geophys. Res. 110, a–n. doi:10.1029/2005JG000010
van Huissteden, J. (2020). “Thawing Permafrost,” in Permafrost Carbon in a Warming Arctic. Springer Nature AG. doi:10.1007/978-3-030-31379-1
Van Huissteden, J., Vandenberghe, J., Gibbard, P. L., Lewin, J., Elias, A., and Mock, C. (2013). PERMAFROST and PERIGLACIAL FEATURES | Periglacial Fluvial Sediments and Forms. 2nd edition. Amsterdam: The Encyclopedia of Quaternary Science, 490–499. doi:10.1016/b978-0-444-53643-3.00108-4
Veci, L., Prats-Iraola, P., Scheiber, R., Collard, F., Fomferra, N., and Engdahl, M. (2014). The sentinel-1 Toolbox, 1–3.
Vonk, J. E., Mann, P. J., Davydov, S., Davydova, A., Spencer, R. G. M., Schade, J., et al. (2013). High Biolability of Ancient Permafrost Carbon upon Thaw. Geophys. Res. Lett. 40 (11), 2689–2693. doi:10.1002/grl.50348
Vonk, J. E., Tank, S. E., Bowden, W. B., Laurion, I., Vincent, W. F., Alekseychik, P., et al. (2015). Reviews and Syntheses: Effects of Permafrost Thaw on Arctic Aquatic Ecosystems. Biogeosciences 12 (23), 7129–7167. doi:10.5194/bg-12-7129-2015
Wang, P., Limpens, J., Nauta, A., van Huissteden, C., Quirina van Rijssel, S., Mommer, L., et al. (2018). Depth-based Differentiation in Nitrogen Uptake between Graminoids and Shrubs in an Arctic Tundra Plant Community. J. Veg Sci. 29 (1), 34–41. doi:10.1111/jvs.12593
Weiss, N., Blok, D., Elberling, B., Hugelius, G., Jørgensen, C. J., Siewert, M. B., et al. (2016). Thermokarst Dynamics and Soil Organic Matter Characteristics Controlling Initial Carbon Release from Permafrost Soils in the Siberian Yedoma Region. Sediment. Geology. 340, 38–48. doi:10.1016/j.sedgeo.2015.12.004
Wessel, B., Huber, M., Wohlfart, C., Marschalk, U., Kosmann, D., and Roth, A. (2018). Accuracy Assessment of the Global TanDEM-X Digital Elevation Model with GPS Data. ISPRS J. Photogrammetry Remote Sensing 139, 171–182. doi:10.1016/j.isprsjprs.2018.02.017
Westermann, S., Langer, M., Boike, J., Heikenfeld, M., Peter, M., Etzelmüller, B., et al. (2016). Simulating the thermal Regime and Thaw Processes of Ice-Rich Permafrost Ground with the Land-Surface Model CryoGrid 3. Geosci. Model. Dev. 9 (2), 523–546. doi:10.5194/gmd-9-523-2016
Keywords: InSAR (interferometric synthetic aperture radar), Yedoma, geomorphology, Thaw lakes, floodplain, neotectonics
Citation: van Huissteden J, Teshebaeva K, Cheung Y, Magnússon RÍ, Noorbergen H, Karsanaev SV, Maximov TC and Dolman AJ (2021) Geomorphology and InSAR-Tracked Surface Displacements in an Ice-Rich Yedoma Landscape. Front. Earth Sci. 9:680565. doi: 10.3389/feart.2021.680565
Received: 14 March 2021; Accepted: 06 August 2021;
Published: 24 August 2021.
Edited by:
Annett Bartsch, b.geos GmbH, AustriaReviewed by:
Simon Zwieback, University of Alaska Fairbanks, United StatesMatthias Siewert, Umeå University, Sweden
Copyright © 2021 van Huissteden, Teshebaeva, Cheung, Magnússon, Noorbergen, Karsanaev, Maximov and Dolman. This is an open-access article distributed under the terms of the Creative Commons Attribution License (CC BY). The use, distribution or reproduction in other forums is permitted, provided the original author(s) and the copyright owner(s) are credited and that the original publication in this journal is cited, in accordance with accepted academic practice. No use, distribution or reproduction is permitted which does not comply with these terms.
*Correspondence: J. van Huissteden, j.van.huissteden@vu.nl