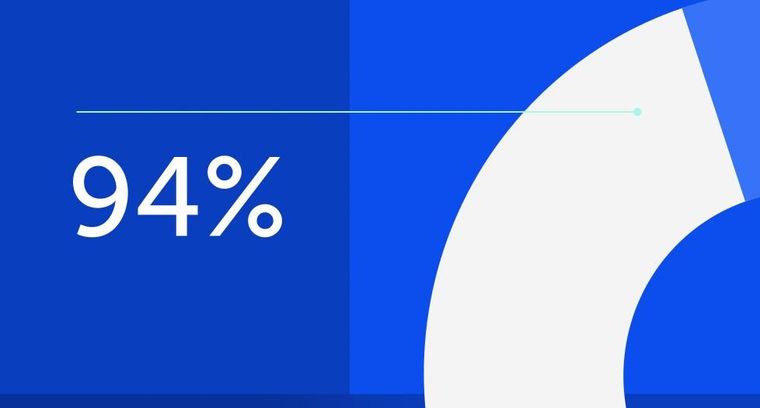
94% of researchers rate our articles as excellent or good
Learn more about the work of our research integrity team to safeguard the quality of each article we publish.
Find out more
ORIGINAL RESEARCH article
Front. Earth Sci., 26 May 2021
Sec. Atmospheric Science
Volume 9 - 2021 | https://doi.org/10.3389/feart.2021.663917
Leaf optical properties (LOPs, i.e., leaf reflectance and transmittance), as a fundamental property of vegetation, are a key parameter in the canopy radiative transfer process. LOPs have a direct impact on the surface solar radiation partition and further affect surface flux exchanges. Recent works have provided reliable LOP data and mentioned that notable differences exist between the prescribed LOP values in current land surface models and measured LOP values, especially in the near-infrared (NIR) band. To evaluate the effects of different LOP values in land surface modeling, we ran two land surface models (the Community Land Model and the Common Land Model) with their default prescribed and measured values to examine the differences in simulated surface radiation partitions and fluxes. Our analyses show that differences in LOP values can lead to a large discrepancy in albedo, radiation partition, sensible heat flux and net radiation simulations. By using the measured LOP values, in the boreal forest zone, Southeast China, and the eastern United States, both models have a significantly increased surface albedo in the NIR band, with the difference exceeding 10% during JJA. Thus, the measured LOP values can improve the negative albedo bias in the boreal forest zone during summertime. Moreover, both models simulate less net radiation with a maximum reduction of 11
Leaf optical properties (LOPs, i.e., leaf reflectance and transmittance) are among the most important driving factors of the Earth’s surface energy balance. Reliable LOP data are also required for the parameterization of the two-stream transfer model (Dickinson, 1983; Sellers, 1985), which is widely adopted in land surface models (LSMs). Thus, LOPs can directly impact surface albedo, which is one of the crucial parameters in the land surface radiation budget and energy balance (Zhai et al., 2014). Many kinds of studies also show that albedo plays an important role in land surface temperature change (Dickinson and Henderson-Sellers, 1988; Bounoua et al., 2002; Holland and Bitz, 2003; Winton, 2006) and has a significant effect on rainfall (Xue and Shukla, 1993; Dirmeyer and Shukla, 1996; Knorr et al., 2001; Levine and Boos, 2017). Feedbacks between albedo and climate are also critical for climate predictions (Pu and Dickinson, 2012; Kovenock and Swann, 2018). Thus, surface albedo shapes the Earth’s climate and climate change (Soden and Held, 2006; Randall et al., 2007).
In LSMs, the LOP values are generally prescribed for two broad bands in the shortwave region, i.e., the visible band (VIS) and the near-infrared band (NIR), and for different land cover types or plant functional types (PFTs), such as needleleaf evergreen tree (NET), needleleaf deciduous tree (NDT), broadleaf evergreen tree (BET) and broadleaf deciduous tree (BDT). These PFTs can be further classified as tropical (Tro), temperate (Tem) and boreal (Bor) by broad geoclimatic zones. Many current LSMs (e.g., the Community Land Model (CLM), the Jena Scheme of Atmosphere Biosphere Coupling in Hamburg (JSBACH), and the Joint United Kingdom Land Environment Simulator (JULES)) either rely on the “time-invariant optical properties look-up table” of the Simple Biosphere (SiB) model presented 30 years ago by Dorman and Sellers (1989) or lack references for the properties they do employ (Majasalmi and Bright, 2019). By examining the prescribed single-scattering albedo [SSA, i.e., the sum of reflectance (
FIGURE 1. Comparison of measured LOP values in the NIR band (A) and VIS band (B) with prescribed values in the different models.
Recent work by researchers has provided LOP data through various kinds of methods. For example, LOPs can be measured by using optical instruments (Middleton and Sullivan, 2000; Göttlicher et al., 2011; Lukeš et al., 2013; Mottus et al., 2014; Hovi et al., 2017; Rautiainen et al., 2018), simulated by leaf-level modeling of LOP models (Jacquemoud and Baret, 1990; Malenovský et al., 2007; Feret et al., 2008; Zhang et al., 2017) or retrieved by inversion of remote sensing data sets (Hagolle et al., 2005; Pinty et al., 2011; Verrelst et al., 2015). The reported LOP values from early literature (e.g., Goudriaan, 1977; Dickinson, 1983) are different from those in the listed models (Figure 1). More recently, Majasalmi and Bright (2019) used various spectral databases to synthesize and harmonize the key optical property information of the PFT classification shared by many leading LSMs and found notable differences between the CLM default and measured LOP values in the NIR band. The LOP values for different PFTs provided by Majasalmi and Bright (2019) are highlighted in Figure 1 and referred to as “measured”. Except for tropical broadleaf trees (i.e., BET-Tro and BDT-Tro), the measured SSA values are generally 0.1–0.29 higher than the prescribed values in the NIR band (Figure 1A). The most significant difference occurs for the needleleaf trees (i.e., NET-Tem, NET-Bor and NDT). In the VIS band, no difference greater than 0.04 is found (Figure 1B). Thus, Majasalmi and Bright (2019) suggested that NIR optical properties require an update.
To date, research has focused on the acquisition of LOP data. Although substantial work has been completed, these data have not been applied in LSMs. The effects of such a large difference in LOP values between prescribed model and measured values in model simulations are still unclear. To this end, in this study, we incorporate the measured LOP values, which are provided by Majasalmi and Bright (2019), in CLM5 and CoLM and compare the difference in simulated albedo as a result of changed LOP values. Moreover, we analyze the changes in surface radiation partition, surface fluxes, net radiation, and land surface temperatures. Finally, differences in simulated global annual gross primary productivity (GPP) are also compared.
In this study, we use two widely adopted land surface models, the Community Model (CLM5) and the Common Land Model (CoLM2014), to conduct offline simulations. Both models calculate radiative transfer through the canopy and the ground surface using the two-stream radiative transfer model. CLM5 is the latest version of the land component in the Community Earth System Model (CESM) (Danabasoglu et al., 2020) and builds on the progress made in CLM4.5. Lawrence et al. (2019) present an overview of model developments. More detailed descriptions can be found in the technical manual (Lawrence et al., 2018).
CoLM combines the advantages of three land surface models: NCAR LSM (Bonan, 1996; Bonan, 1998), Biosphere-Atmosphere Transfer Scheme (BATS) (Dickinson et al., 1993) and Institute of Atmospheric Physics LSM (IAP94) (Dai and Zeng, 1997). CoLM2014 (http://globalchange.bnu.edu.cn/research/models) is an update of CoLM2005 (Dai et al., 2004; Ji and Dai, 2010) and CoLM (Dai et al., 2003).
For the purpose of examining the effects of different LOP values on land surface modeling, we run offline simulations of CLM5 and CoLM2014 with their default LOP values (named CLM and CoLM) and with the measured LOP values (named
TABLE 1. Default prescribed and measured leaf optical property values for each PFT set in CLM (CoLM) and
FIGURE 2. Fractional coverage of different vegetation cover types in CLM5.0 (A–D) and CoLM2014 (E–H).
Using the remotely sensed leaf area index, CLM and
We first compare the black-sky albedo in the NIR band simulated by CLM and
FIGURE 3. Differences in mean seasonal albedo between CLM and
Figure 4 presents the difference in the black-sky albedo in the NIR band between CoLM and
FIGURE 4. Information the same as that in Figure 3 but for CoLM.
We also compare the simulated albedo of each model with observations derived from CERES-EBAF data (Kato et al., 2013), which are applied for ILAMB (Collier et al., 2018). The CERES provides monthly albedo from 2000 to 2013 at a 0.5° spatial resolution. We use data from 2005–2009 and grid them to the CLM5 resolution in comparison to the CLM and
Figures 6A,D show the differences in the zonal average reflected solar radiation between CLM (CoLM) and
FIGURE 6. Zonal average differences in reflected solar radiation (A,D), solar absorption (B,E) and sensible heat flux (C,F) between CLM (CoLM) and
LOP values can also directly influence the energy partition between the canopy and ground. Differences in the zonal average solar radiation absorbed by vegetation and the ground between CLM (CoLM) and
The changes in radiation partition lead to a difference in the sensible heat (SH) flux of vegetation and the ground (Figure 6C and Figure 6F). There is a significant positive correlation between solar radiation absorption (Figures 6B,E) and SH (Figures 6C,F). The difference in SH is also mainly located between 40°–60°N and 30°–55°S. As shown in Figures 6C,F, at 60°N,
It should be noted that the LOP uncertainty has a considerable effect on the total SH (Figure 7). The most obvious change is in the growing season. Therefore, we only show the differences during MAM and JJA. Due to the significant reduction in vegetation absorption for
As shown in Figure 8, it is apparent that
FIGURE 8. The information is the same as that in Figure 7 but for net radiation.
We also compared the differences in 2 m air temperature, ground (vegetation) temperature and skin temperature (i.e., radiative temperature). In both CLM and CoLM, “2 m” is defined as 2 m above the apparent sink for sensible heat (Dai et al., 2001; Lawrence et al., 2018). Figure 9 shows the differences in the land surface temperature between CLM (CoLM) and
FIGURE 9. Zonal average differences in 2-m air temperature (A,D), vegetation (ground) temperature (B,E) and skin temperature (C,F) between CLM (CoLM) and CLM_mLOP (CoLM_mLOP). The solid line and dashed line in (B,C,E,F) represent vegetation and the ground, respectively.
By examining the prescribed LOPs of 6 LSMs, it was found that there is a difference of 0.1–0.29 from the measured SSA. The effects of such a large difference in LOP values between the prescribed model values and measured values of land surface modeling are still unclear. To determine the effects, we employed two widely used land surface models (CLM5 and CoLM2014) to examine the potential effects by incorporating the measured LOP values. The results indicate that the measured LOP values have a significant effect on surface albedo simulation, radiation partitioning and SH exchange in the boreal forest zone, Southeast China and the eastern United States. By using the measured LOP values, the surface albedo in the NIR band increases by 3–10% in the abovementioned regions, especially in JJA. Thus, the negative bias of surface albedo between the model and observational data can be significantly improved in the boreal forest zone during JJA. The energy partition can also be directly influenced by the LOP values. Both models generally have increased ground radiation absorption and less canopy radiation absorption. The measured LOP values also have a considerable effect on the net radiation and SH calculation. The net radiation of
The measured LOP values provided by Majasalmi and Bright (2019) may not be “correct” per se. However, compared with the LOP values used in today’s LSMs, these data synthesize various observational data and spectral databases (Majasalmi and Bright, 2019). The purpose of this study is not to identify which optical parameter is the “correct” or better but to examine the effects of LOP values on surface radiation transfer and flux exchanges. As demonstrated from the results, LOP values could induce large uncertainties. In recent years, LSMs have aimed to develop more accurate and realistic physical processes. As a 1-D vertical canopy structure model, the two-stream model uses the fixed LOP values from a look-up table of “time-invariant” optical properties. It should be noted that LOPs generally show seasonal changes with vegetation growth and senescence stages (Yuan et al., 2017). In the future, a database of optical property data of tree species will be developed. These data could be used to realistically describe vegetation properties in LSMs to improve the accuracy of the model simulations. On the other hand, the two-stream model may be unrealistic in its assumption of the canopy structure and may introduce a large bias. In LSMs, the representation of canopy processes is given by the “big-leaf” model, which replaces the entire canopy with a single vegetation element regardless of the canopy profile (McGrath et al., 2016). However, different vegetation canopies may coexist and form multiple canopy layers, and significant three-dimensional canopy structures may exist (Yuan et al., 2014). And due to the non-linear response of photosynthesis, it is difficult to find a single value of leaf physiological properties to adequately represent the entire canopy under all conditions (McGrath et al., 2016). The shortwave radiation absorbed for photosynthesis is also used in the calculation of the energy budget. Therefore, using multilayer radiation transfer models to accurately describe the process of photosynthesis and radiation transfer within the canopy is the principal direction of current research and progress has already been made (Yuan et al., 2014; McGrath et al., 2016; Qiu et al., 2016). Yuan et al. (2014) developed a three-layer canopy radiation transfer model based on the three-dimensional structural canopy effect. McGrath et al. (2016) presented a multi-level radiation transfer scheme for the ORganising Carbon and Hydrology In Dynamic EcosystEms. And Qiu et al. (2016) developed a generalized radiative transfer scheme with nonuniform optical properties of adaxial and abaxial leaf surfaces and the nonuniform canopy structure in the vertical direction. In these researches, the radiation transfer model usually has more complex and realistic assumptions and shows a good improvement in some cases. In this study, we only operated offline simulations and found that LOP has a considerable impact on model simulation, such as albedo simulation. However, albedo is important not only in LSMs but also in land-atmosphere interaction and coupling models (Betts, 2000; Betts, 2001; Berbet and Costa, 2003; Boisier et al., 2012). Therefore, the effects of LOP values on coupled land-atmosphere model simulations are also worth considering.
Publicly available datasets were analyzed in this study. This data can be found here: CERES albedo: http://redwood.ess.uci.edu/mingquan/www/ILAMB/
HY and YD contributed to the conception and design of the study. WD and LH performed the model simulation. WD contributed to the data process, prepare figures, perform the statistical analysis and write the first draft of the manuscript. JP contributed to the data curation. WD, HY, SZ, RZ, and HL contributed to manuscript revision. All authors read and approved the submitted version.
This research is supported by the National Key R&D Program of China (Grant No. 2017YFA0604300), Natural Science Foundation of China (Grants No. 42075160 and 41730962), Innovation Group Project of Southern Marine Science and Engineering Guangdong Laboratory (Zhuhai) (Grant No. 311021009), Guangdong Province Key Laboratory for Climate Change and Natural Disaster Studies (Grant No. 2020B1212060025), and National Key Scientific and Technological Infrastructure project “Earth System Science Numerical Simulator Facility” (EarthLab).
The authors declare that the research was conducted in the absence of any commercial or financial relationships that could be construed as a potential conflict of interest.
Berbet, M. L. C., and Costa, M. H. (2003). Climate Change after Tropical Deforestation: Seasonal Variability of Surface Albedo and its Effects on Precipitation Change. J. Clim. 16 (12), 2099–2104. doi:10.1175/1520-0442(2003)016<2099:CCATDS>2.0
Betts, R. A. (2000). Offset of the Potential Carbon Sink from Boreal Forestation by Decreases in Surface Albedo. Nature. 408 (6809), 187–190. doi:10.1038/35041545
Betts, R. (2001). Biogeophysical Impacts of Land Use on Present-Day Climate: Near-Surface Temperature Change and Radiative Forcing. Atmos. Sci. Lett. 2 (1-4), 39–51. doi:10.1006/asle.2001.0023
Boisier, J. P., de Noblet-Ducoudré, N., Pitman, A. J., Cruz, F. T., Delire, C., van den Hurk, B. J. J. M., et al. (2012). Attributing the Impacts of Land-Cover Changes in Temperate Regions on Surface Temperature and Heat Fluxes to Specific Causes: Results from the First LUCID Set of Simulations. J. Geophys. Res. 117, D12116. doi:10.1029/2011jd017106
Bonan, G. B. (1996). Land Surface Model (LSM Version 1.0) for Ecological, Hydrological, and Atmospheric Studies: Technical Description and Users Guide. Technical Note. Boulder, CO, United States: National Center for Atmospheric Research.
Bonan, G. B. (1998). The Land Surface Climatology of the NCAR Land Surface Model Coupled to the NCAR Community Climate Model. J. Clim. 11 (6), 1307–1326. doi:10.1175/1520-0442(1998)011<1307:TLSCOT>2.0
Bounoua, L., DeFries, R., Collatz, G. J., Sellers, P., and Khan, H. (2002). Effects of Land Cover Conversion on Surface Climate. Climatic Change. 52 (1-2), 29–64. doi:10.1023/A:1013051420309
Burakowski, E., Tawfik, A., Ouimette, A., Lepine, L., Novick, K., Ollinger, S., et al. (2018). The Role of Surface Roughness, Albedo, and Bowen Ratio on Ecosystem Energy Balance in the Eastern United States. Agric. For. Meteorology. 249, 367–376. doi:10.1016/j.agrformet.2017.11.030
Clark, D. B., Mercado, L. M., Sitch, S., Jones, C. D., Gedney, N., Best, M. J., et al. (2011). The Joint UK Land Environment Simulator (JULES), Model Description - Part 2: Carbon Fluxes and Vegetation Dynamics. Geosci. Model. Dev. 4 (3), 701–722. doi:10.5194/gmd-4-701-2011
Collier, N., Hoffman, F. M., Lawrence, D. M., Keppel‐Aleks, G., Koven, C. D., Riley, W. J., et al. (2018). The International Land Model Benchmarking (ILAMB) System: Design, Theory, and Implementation. J. Adv. Model. Earth Syst. 10 (11), 2731–2754. doi:10.1029/2018MS001354
Dai, Y., Dickinson, R. E., and Wang, Y.-P. (2004). A Two-Big-Leaf Model for Canopy Temperature, Photosynthesis, and Stomatal Conductance. J. Clim. 17 (12), 2281–2299. doi:10.1175/1520-0442(2004)017<2281:ATMFCT>2.0.CO
Dai, Y. J., Zeng, X., and Dickinson, R. E. (2001). The Common Land Model (CLM): Technical Documentation and User’s Guide. Atlanta, GA: Georgia Institute of Technology.
Dai, Y., and Zeng, Q. (1997). A Land Surface Model (IAP94) for Climate Studies Part I: Formulation and Validation in Off-Line Experiments. Adv. Atmos. Sci. 14 (4), 433–460. doi:10.1007/s00376-997-0063-4
Dai, Y., Zeng, X., Dickinson, R. E., Baker, I., Bonan, G. B., Bosilovich, M. G., et al. (2003). The Common Land Model. Bull. Am. Meteorol. Soc. 84 (8), 1013–1024. doi:10.1175/BAMS-84-8-1013
Danabasoglu, G., Lamarque, J. F., Bacmeister, J., Bailey, D. A., DuVivier, A. K., Edwards, J., et al. (2020). The Community Earth System Model Version 2 (CESM2). J. Adv. Model. Earth Syst. 12 (2), e2019MS001916. doi:10.1029/2019ms001916
Dickinson, R. E., Henderson-Sellers, A., and Kennedy, J. (1993). Biosphere-atmosphere transfer scheme (BATS) version 1e as coupled to the NCAR community climate model. Technical Note NCAR/TN-387+STR. Boulder, CO: National Center for Atmospheric Research. doi:10.5065/D67W6959
Dickinson, R. E., and Henderson-Sellers, A. (1988). Modelling Tropical Deforestation: A Study of GCM Land-Surface Parametrizations. Q. J R. Met. Soc. 114 (480), 439–462. doi:10.1002/qj.49711448009
Dickinson, R. E. (1983). Land Surface Processes and Climate-Surface Albedos and Energy Balance. Adv. Geophys. 25, 305–353. doi:10.1016/s0065-2687(08)60176-4
Dirmeyer, P. A., and Shukla, J. (1996). The Effect on Regional and Global Climate of Expansion of the World's Deserts. Q.J R. Met. Soc. 122 (530), 451–482. doi:10.1002/qj.49712253008
Dorman, J. L., and Sellers, P. J. (1989). A Global Climatology of Albedo, Roughness Length and Stomatal Resistance for Atmospheric General Circulation Models as Represented by the Simple Biosphere Model (SiB). J. Appl. Meteorology Climatology. 28 (19), 833–855. doi:10.1175/1520-0450(1989)028<0833:agcoar>2.0.co;2
Feret, J.-B., François, C., Asner, G. P., Gitelson, A. A., Martin, R. E., Bidel, L. P. R., et al. (2008). PROSPECT-4 and 5: Advances in the Leaf Optical Properties Model Separating Photosynthetic Pigments. Remote Sensing Environ. 112 (6), 3030–3043. doi:10.1016/j.rse.2008.02.012
Georgievski, G., and Hagemann, S. (2018). Characterizing Uncertainties in the ESA-CCI Land Cover Map of the Epoch 2010 and Their Impacts on MPI-ESM Climate Simulations. Theor. Appl. Climatol 137 (1-2), 1587–1603. doi:10.1007/s00704-018-2675-2
Göttlicher, D., Albert, J., Nauss, T., and Bendix, J. (2011). Optical Properties of Selected Plants from a Tropical Mountain Ecosystem - Traits for Plant Functional Types to Parametrize a Land Surface Model. Ecol. Model. 222 (3), 493–502. doi:10.1016/j.ecolmodel.2010.09.021
Goudriaan, J. (1977). Crop Micrometeorology: A Simulation Study. Wageningen, Netherlands: Center for Agricultural Publishing and Documentation.
Hagolle, O., Lobo, A., Maisongrande, P., Cabot, F., Duchemin, B., and De Pereyra, A. (2005). Quality Assessment and Improvement of Temporally Composited Products of Remotely Sensed Imagery by Combination of VEGETATION 1 and 2 Images. Remote Sensing Environ. 94 (2), 172–186. doi:10.1016/j.rse.2004.09.008
Hartley, A. J., MacBean, N., Georgievski, G., and Bontemps, S. (2017). Uncertainty in Plant Functional Type Distributions and its Impact on Land Surface Models. Remote Sensing Environ. 203, 71–89. doi:10.1016/j.rse.2017.07.037
Haverd, V., Smith, B., Nieradzik, L., Briggs, P. R., Woodgate, W., Trudinger, C. M., et al. (2018). A New Version of the CABLE Land Surface Model (Subversion Revision R4601) Incorporating Land Use and Land Cover Change, Woody Vegetation Demography, and a Novel Optimisation-Based Approach to Plant Coordination of Photosynthesis. Geosci. Model. Dev. 11 (7), 2995–3026. doi:10.5194/gmd-11-2995-2018
Holland, M. M., and Bitz, C. M. (2003). Polar Amplification of Climate Change in Coupled Models. Clim. Dyn. 21 (3-4), 221–232. doi:10.1007/s00382-003-0332-6
Hovi, A., Raitio, P., and Rautiainen, M. (2017). A Spectral Analysis of 25 Boreal Tree Species. Silva Fenn. 51 (4), 1–16. doi:10.14214/sf.7753
Jacquemoud, S., and Baret, F. (1990). PROSPECT: A Model of Leaf Optical Properties Spectra. Remote sensing Environ. 34 (2), 75–91. doi:10.1016/0034-4257(90)90100-Z
Ji, D., and Dai, Y. (2010). The Common Land Model (CoLM) Technical Guide. Beijing, China: GCESS, Beijing Normal University
Kato, S., Loeb, N. G., Rose, F. G., Doelling, D. R., Rutan, D. A., Caldwell, T. E., et al. (2013). Surface Irradiances Consistent with CERES-Derived Top-Of-Atmosphere Shortwave and Longwave Irradiances. J. Clim. 26 (9), 2719–2740. doi:10.1175/jcli-d-12-00436.1
Knorr, W., Schnitzler, K.-G., and Govaerts, Y. (2001). The Role of Bright Desert Regions in Shaping North African Climate. Geophys. Res. Lett. 28 (18), 3489–3492. doi:10.1029/2001gl013283
Kovenock, M., and Swann, A. L. S. (2018). Leaf Trait Acclimation Amplifies Simulated Climate Warming in Response to Elevated Carbon Dioxide. Glob. Biogeochem. Cycles 32 (10), 1437–1448. doi:10.1029/2018gb005883
Lawrence, D., Fisher, R., Koven, C., Oleson, K., Swenson, S., Vertenstein, M., et al. (2018). Technical Description of Version 5.0 of the Community Land Model (CLM). Boulder, CO: National Center for Atmospheric Research, University Corporation for Atmospheric Research
Lawrence, D. M., Fisher, R. A., Koven, C. D., Oleson, K. W., Swenson, S. C., Bonan, G., et al. (2019). The Community Land Model Version 5: Description of New Features, Benchmarking, and Impact of Forcing Uncertainty. J. Adv. Model. Earth Syst. 11 (12), 4245–4287. doi:10.1029/2018ms001583
Levine, X. J., and Boos, W. R. (2017). Land Surface Albedo Bias in Climate Models and its Association with Tropical Rainfall. Geophys. Res. Lett. 44 (12), 6363–6372. doi:10.1002/2017GL072510
Lukeš, P., Stenberg, P., Rautiainen, M., Mõttus, M., and Vanhatalo, K. M. (2013). Optical Properties of Leaves and Needles for Boreal Tree Species in Europe. Remote Sensing Lett. 4 (7), 667–676. doi:10.1080/2150704x.2013.782112
Majasalmi, T., and Bright, R. M. (2019). Evaluation of Leaf-Level Optical Properties Employed in Land Surface Models. Geosci. Model. Dev. 12 (9), 3923–3938. doi:10.5194/gmd-12-3923-2019
Malenovský, Z., Albrechtová, J., Lhotáková, Z., Zurita‐Milla, R., Clevers, J. G. P. W., Schaepman, M. E., et al. (2007). Applicability of the PROSPECT Model for Norway Spruce Needles. Int. J. Remote Sensing 27 (24), 5315–5340. doi:10.1080/01431160600762990
McGrath, M. J., Ryder, J., Pinty, B., Otto, J., Naudts, K., Valade, A., et al. (2016). A Multi-Level Canopy Radiative Transfer Scheme for ORCHIDEE (SVN R2566), Based on a Domain-Averaged Structure Factor. Geosci. Model. Dev. Discuss. 2016, 1–22. doi:10.5194/gmd-2016-280
Middleton, E. M., and Sullivan, J. (2000). BOREAS TE-10 Leaf Optical Properties for SSA Species. Oak Ridge, Tennessee, USA:ORNL DAAC. doi:10.3334/ORNLDAAC/531
Mottus, M., Sulev, M., and Hallik, L. (2014). Seasonal Course of the Spectral Properties of Alder and Birch Leaves. IEEE J. Sel. Top. Appl. Earth Observations Remote Sensing 7 (6), 2496–2505. doi:10.1109/jstars.2013.2294242
Niu, G.-Y., Yang, Z.-L., Mitchell, K. E., Chen, F., Ek, M. B., Barlage, M., et al. (2011). The Community Noah Land Surface Model with Multiparameterization Options (Noah-MP): 1. Model Description and Evaluation with Local-Scale Measurements. J. Geophys. Res. 116, D12109. doi:10.1029/2010JD015139
Pinty, B., Andredakis, I., Clerici, M., Kaminski, T., Taberner, M., Verstraete, M. M., et al. (2011). Exploiting the MODIS Albedos with the Two-Stream Inversion Package (JRC-TIP): 1. Effective Leaf Area Index, Vegetation, and Soil Properties. J. Geophys. Res. 116, D09105. doi:10.1029/2010jd015372
Pu, B., and Dickinson, R. E. (2012). Examining Vegetation Feedbacks on Global Warming in the Community Earth System Model. J. Geophys. Res. 117, D20110. doi:10.1029/2012jd017623
Qiu, B., Guo, W., Xue, Y., and Dai, Q. (2016). Implementation and Evaluation of a Generalized Radiative Transfer Scheme within Canopy in the Soil-Vegetation-Atmosphere Transfer (SVAT) Model. J. Geophys. Res. Atmos. 121 (20), 12145–12163. doi:10.1002/2016jd025328
Randall, D. A., Wood, R. A., Bony, S., Colman, R., Fichefet, T., Fyfe, J., et al. (2007). “Climate Models and Their Evaluation,” in Climate Change 2007: The Physical Science Basis. Contribution of Working Group I to the Fourth Assessment Report of the Intergovernmental Panel on Climate Change. Editors S. Solomon, D. Qin, M. Manning, Z. Chen, M. Marquis, K. B. Averytet al. (Cambridge, United Kingdom: Cambridge University Press).
Rautiainen, M., Lukeš, P., Homolová, L., Hovi, A., Pisek, J., and Mõttus, M. (2018). Spectral Properties of Coniferous Forests: A Review of In Situ and Laboratory Measurements. Remote Sensing 10 (2), 207. doi:10.3390/rs10020207
Sellers, P. J. (1985). Canopy Reflectance, Photosynthesis and Transpiration. Int. J. Remote Sensing 6 (8), 1335–1372. doi:10.1080/01431168508948283
Soden, B. J., and Held, I. M. (2006). An Assessment of Climate Feedbacks in Coupled Ocean-Atmosphere Models. J. Clim. 19 (14), 3354–3360. doi:10.1175/JCLI3799.1
Verrelst, J., Camps-Valls, G., Muñoz-Marí, J., Rivera, J. P., Veroustraete, F., Clevers, J. G. P. W., et al. (2015). Optical Remote Sensing and the Retrieval of Terrestrial Vegetation Bio-Geophysical Properties - A Review. ISPRS J. Photogrammetry Remote Sensing. 108, 273–290. doi:10.1016/j.isprsjprs.2015.05.005
Winton, M. (2006). Amplified Arctic Climate Change: What Does Surface Albedo Feedback Have to Do with it?. Geophys. Res. Lett. 33 (3), L03701. doi:10.1029/2005gl025244
Xue, Y., and Shukla, J. (1993). The Influence of Land Surface Properties on Sahel Climate. Part 1: Desertification. J. Clim. 6 (12), 2232–2245. doi:10.1175/1520-0442(1993)006<2232:TIOLSP>2.0
Yuan, H., Dickinson, R. E., Dai, Y., Shaikh, M. J., Zhou, L., Shangguan, W., et al. (2014). A 3D Canopy Radiative Transfer Model for Global Climate Modeling: Description, Validation, and Application. J. Clim. 27 (3), 1168–1192. doi:10.1175/jcli-d-13-00155.1
Yuan, H., Dai, Y., Dickinson, R. E., Pinty, B., Shangguan, W., Zhang, S., et al. (2017). Reexamination and Further Development of Two-Stream Canopy Radiative Transfer Models for Global land Modeling. J. Adv. Model. Earth Syst. 9 (1), 113–129. doi:10.1002/2016ms000773
Zhai, J., Liu, R., Liu, J., Zhao, G., and Huang, L. (2014). Radiative Forcing over China Due to Albedo Change Caused by Land Cover Change during 1990-2010. J. Geogr. Sci. 24 (5), 789–801. doi:10.1007/s11442-014-1120-4
Keywords: leaf optical properties, canopy radiative transfer, albedo, energy balance, surface radiation partition, surface fluxes, net radiation, land surface model
Citation: Dong W, Yuan H, Zhang R, Li H, Huang L, Zhu S, Peng J and Dai Y (2021) Effects of Incorporating Measured Leaf Optical Properties in Land Surface Models. Front. Earth Sci. 9:663917. doi: 10.3389/feart.2021.663917
Received: 04 February 2021; Accepted: 04 May 2021;
Published: 26 May 2021.
Edited by:
Yuqing Wang, University of Hawaii at Manoa, United StatesReviewed by:
Zong-Liang Yang, University of Texas at Austin, United StatesCopyright © 2021 Dong, Yuan, Zhang, Li, Huang, Zhu, Peng and Dai. This is an open-access article distributed under the terms of the Creative Commons Attribution License (CC BY). The use, distribution or reproduction in other forums is permitted, provided the original author(s) and the copyright owner(s) are credited and that the original publication in this journal is cited, in accordance with accepted academic practice. No use, distribution or reproduction is permitted which does not comply with these terms.
*Correspondence: Hua Yuan, eXVhbmgyNUBtYWlsLnN5c3UuZWR1LmNu
Disclaimer: All claims expressed in this article are solely those of the authors and do not necessarily represent those of their affiliated organizations, or those of the publisher, the editors and the reviewers. Any product that may be evaluated in this article or claim that may be made by its manufacturer is not guaranteed or endorsed by the publisher.
Research integrity at Frontiers
Learn more about the work of our research integrity team to safeguard the quality of each article we publish.