- 1Department of Natural Resources, Cornell University, Ithaca, NY, United States
- 2College of Life Science, Northwest A and F University, Yangling, China
- 3Department of Microbiology, Cornell University, Ithaca, NY, United States
Converting forest and wetland landscapes to agriculture has shown to result in a loss of organic matter, structure, and microbial diversity in the converted soil but recovery of post-agricultural soils remains poorly understood. Here we coupled landscape-scale surveys of soil 1) carbon and nitrogen levels, 2) aggregation, and 3) bacterial metagenomes to investigate soil recovery after 30 years in sites with soils ranging from well drained to poorly drained. Sites with no evidence of past agriculture (Reference) served as recovery endpoints. A secondary aim evaluated the role of nitrogen-fixing symbiosis, here associated with alder (Alnus incana) trees, in soil restoration. Soil carbon levels in restored sites (3.5%) were comparable to levels in a present-day farm (3.4%) but much lower than in Reference sites (>7.3%). The same trend occurred with soil nitrogen levels. Sites with alder trees had more acidic soil pH values. Alder trees promoted soil structure with macroaggregates being the largest fraction of bulk soil (75%). Natural abundance of stable nitrogen isotopes suggested extensive decay of organic matter within aggregates. Comparison of total reads from the soil metagenomes indicated the bacterial community in restored sites were more comparable to the present-day farm than Reference sites, except for a well-drained soil with alder. Dissimilarity among sites in terms of gene abundances in soil bacterial community occurred in carbon metabolism, membrane transport, and genetic repair pathways. Soil recovery in post-agricultural landscapes is slow when agriculture caused a large loss of soil organic matter, as is the case in our study, and when the soil bacterial community structure changed markedly, as it did in our study. However, fairly rapid recovery of soil structure, as we noted in our study, is promising, and now we need a better understanding of plant species that improve soil structure for restoration of both well-drained and poorly drained soils.
Key Points
‐Bulk soils in both well-drained and poorly drained (wetland) sites showed no increase in organic matter with 30 years post-agricultural recovery.
‐Soil structure, measured by aggregation, was promoted by alder trees established in both restored and natural sites.
‐Microbial functions, measured as gene abundance, distinguished between sites with well-drained soils and poorly drained soils, but only an upland site with alder trees had more similar values to a natural site than the present-day farm.
Introduction
Agriculture covers 38% of Earth’s land surface with much of it on land that naturally supports forests and wetlands. However, converting forests and wetlands to agriculture often initiates a downward spiral in soil conditions (Kuzyakov and Zamanian, 2019). When drastic enough, agricultural production drops or fails altogether forcing abandonment. While many studies examine recovery of forest and wetland plant communities in post-agricultural landscapes (cf., Flinn and Vellend, 2005), a crucial uncertainty concerns how concomitant soils reclaim their natural conditions (Lal, 2015).
Although many studies have addressed individual mechanisms and specific drivers of soil degradation (cf., Bunemann et al., 2018), there are still no standards and comprehensive ways to differentiate between stages of degradation. Nonetheless, degraded soils experience to some degree: 1) loss of soil organic matter, 2) deterioration of soil structure, 3) scant or excessive levels of nutrients, and 4) altered microbial diversity and activity (Carter, 2002). The reclamation of each is still a matter of conjecture.
For example, the stock of soil organic matter represents the long-term balance between input of plant residue to the soil and decomposition of that residue by soil microorganisms. Agriculture has been shown to upset this balance, largely by increasing the rate of organic matter decomposition (Magdoff and Weil, 2004). One way that organic matter escapes microbial decomposers is by stabilization on the surfaces of soil minerals that are occluded within soil aggregates (Six et al., 2000; Lehmann et al., 2020). Tillage is especially destructive to soil aggregation and facilitates microbial access to organic matter. Thus, recovery of soil organic matter is linked to improved soil structure that restores the balance between inputs and decomposition of plant residue (Six et al., 2000).
A wide variety of approaches has been used to examine soil microorganisms, mostly in terms of community composition, diversity, and assembly mechanisms (Nemergut et al., 2013; Fierer, 2017). Yet additional information exists in the relative abundance of genes that soil microorganisms have and use, i.e., the soil metagenome. Although we know that genes alone do not determine microbial activity per se (Jansson and Hofmockel, 2018), we still expect a larger suite of genes to process plant residue that has a diverse biochemical composition or when nutrient fertilizers have been added compared to a uniform plant residue and nutrient poor soils (cf., Castañeda and Barbosa, 2017; Hermans et al., 2020).
Successful recovery of degraded soil also depends on knowing the endpoint that restoration is expected to achieve. When agriculture occurs on a landscape composed of different habitats, knowing the recovery endpoint can be complicated (Crews and Rumsey, 2017). For example, in many forested landscapes in temperate regions, the predominant forest established on mostly well-drained soil often also includes wet habitats with poorly drained soil. These wet habitats include permanent and seasonal pools, glades, stream-sides (riparian), and wetland marshes and swamps (Flinn et al., 2008; Cohen et al., 2016). Having comparable vestiges removes some of the uncertainty in the recovery endpoint. However, when agriculture is extensive and no natural sites exist, it is best to have more than one recovery endpoint for comparison with the restored site (Aronson et al., 1995).
The work described here is a survey of soil conditions in a post-agricultural landscape in central New York State. The landscape was originally upland forest with embedded wetlands that was converted to agriculture for ∼70 years and then abandoned in the mid-1980s. Not long after agriculture ceased a restoration effort was undertaken. Natural stream flow across the site was restored. One portion of the study area (described below) was maintained as an old field dominated by herbaceous plants (Stover and Marks, 1998), whereas the other portion had more advanced succession and was dominated by speckled alder (Alnus incana) trees. Roots of alder are associated with soil microorganisms that 'fix' atmospheric nitrogen into a bioavailable form (Hurd et al., 2001), thereby adding nutrient nitrogen to the soil (Preem et al., 2012). This land use history is common in the region (Flinn and Vellend, 2005).
We focused on three soil parameters: 1) quantifying carbon and nitrogen in 11 sites including those with current agriculture (Farm), sites in the processes of recovery from agriculture (Restored), and sites with no previous agriculture (Reference); 2) measuring soil aggregation and aggregate stability in a subset of these soils; and 3) characterizing metagenomes to measure microbial gene abundances in the full suite of soils. We expected slow recovery of soil organic matter, in particular, if loss during agriculture created a large difference in levels between the Farm and Reference sites. We expected more rapid recovery of soil structure, which is promoted differently by different plant species (Scott, 1998) but especially by alder trees (Graf and Frei, 2013). Finally, although soil microorganisms have fast growth, we expected persistent legacy of agriculture on bacterial community structure as has been seen in the region (Hudgens and Yavitt, 1997).
Materials and Methods
Study Site and Experimental Design
The research was conducted in central New York State. The region has a humid, continental climate with a mean monthly temperature of −4°C in January and 22°C in July. The mean frost-free season is 146 days, and mean annual precipitation is 890 mm, including 171 cm of snow. The vegetation falls into the Allegheny section of E. L. Braun’s (in Dyer, 2006) hemlock–white pine–northern hardwoods region. Mesic, upland forests are dominated by sugar maple (Acer saccharum) and American beech (Fagus grandifolia).
The region lies on the glaciated Allegheny Plateau. Topography is a relatively flat plain at 300 to 450 m above sea level. About 90% of the bedrock is overlain by glacial till of variable thickness and composition. Gravelly or sandy outwash and terraces of ice-contact stratified drift characterize the lowest lying land along streams and account for the remaining 10% of the landscape. Nearly all of the abandoned farmland is underlain by glacial till with low to medium carbonate content, whereas till with a great carbonate content are still in production. Our upland sites have coarse-loamy, well-drained soils formed from medium-lime tills and classified as Typic Dystrudepts. Our lowland sites have fine-loamy, poorly drained soils formed in low-to medium-lime till and classified as Aeric Fragiaquepts.
We established 11 study sites in total. Seven sites were within the Goetchius Wetland Preserve (42°23′18.114″N 76°18′1.9188″W). Here we established two sites in primary forest (Reference Upland) that had never been used for agriculture, as indicated by the pit and mound topography across the surface soil. Plowing levels this microtopography, which is not regained until mature trees, >100 years old begin to fall (Flinn and Marks, 2007). A portion of the Reference Upland has beech-maple forest with alder trees and was designated Reference Upland + alder and another portion has the beech-maple forest but no alder trees and was designated Reference Upland - alder. A third site was an active farm (Farm), the only one on the Preserve. Agriculture management on the Farm consists of 3-years continuous planting of corn followed by 3-years of perennial forage, alfalfa (Medicago sativa) plus reed canarygrass (Phalaris arundinacea). We sampled in the third year of the forage production. The fourth and fifth sites have relatively well-drained soil in which tillage ceased in 1989. A portion dominated by old-field, herbaceous plants was designated Restored Upland–alder, and a second old field with alder trees was designated Restored Upland + alder. The remaining two sites at Goetchius were associated with a small stream that cut across the site that had been diverted during the period of agriculture with natural flow restored in 1989. This area has poorly drained soil. One portion was dominated by cattails (Typha latifolia) and sedges (Carex sp.) growing in about 25 cm of open water and was designated Restored Wetland-alder, whereas the other has cattails and sedges with alder trees and was designated Restored Wetland + alder.
Since no Reference Wetlands occur in the Goetchius site, we used two other locations in the same region as references. One location was the Dorothy McIlroy Bird Sanctuary (42°40′09.9912″N 76°17′24.0792″W), which is the headwater of Fall Creek at the outlet of Lake Como. The wetland is a hummocky, streamside fen, dominated by sedges. One portion has sedges with the nitrogen-fixing shrub sweet gale (Myrica gale; Schwintzer, 1979) and was designated Reference Streamside Wetland + Myrica. A second portion has sedges but no Myrica and was designated Reference Streamside Wetland - Myrica. A second location was the headwater of Michigan Creek at the outlet of Jennings Pond (42°19′41.5128″N 76°28′41.0952″W). This wetland is a marshy, sedge fen (Bernard and Solsky, 1977), dominated by a dense cover of lake sedge (Carex lacustris). One portion has sedges with scattered alder trees and was designated Reference Depressional Wetland + alder, whereas a second portion has sedges with no alder trees and was designated Reference Depressional Wetland-alder. Soil in the depressional wetland is 1-m deep peat soil, whereas soil in the streamside wetland is mostly clay with no peat accumulation. Although no peat soil occurs in the Goetchius study site, we included the depressional location because this wetland type is common in the region (Ballantine and Schneider, 2009). Also, agricultural on peat soils is known to result in complete loss of the peat (Welsch and Yavitt, 2007).
Study 1: Bulk Soil
In each of the 11 study sites, we established three locations to collect soils. The locations were determined at random from a numbered x-y grid over a map of the site and a random number generator. At each sample location we collected 100 g of soil from three points (0 to 10-cm depth interval) that were combined to make a composite sample for a total of 33 samples. We measured moisture content gravimetrically on fresh portions of each soil, collected in early spring, i.e., the wettest time of the year. Other portions were air-dried before extracting available cations and phosphorus using the Mehlich III extractant solution (Mehlich, 1984): elemental analysis was done using atomic emission–inductively coupled plasma (AE–ICP) spectroscopy at Cornell University Laboratories.
Study 2: Soil Structure
We characterized the distribution of soil mass, carbon, and nitrogen in hierarchical aggregates from nine sites in the study area. We excluded peat soils from the Reference Wetland +/− alder, given the organic nature of the soil. Soil aggregates were fractionated by wet sieving of an air-dried portion (ca. 25 g) of the <2 mm fraction of soil following the scheme in Elliott (1986). Briefly, soil was spread evenly on a sieve with an opening of >250 μm, the sieve was immersed in water for 5 min (slaking). The sieve was then moved up and down at a rate of 50 strokes in 2 min. Floatable material was decanted and saved (designated free POM; fPOM) since it was part of the <2 mm fraction. Material that passed through the 250-µm sieve was allowed to collect on or pass through a sieve with an opening of 53 µm. The three fractions were washed into pre-weighed pans, i.e., macroaggregates (>250 µm), free microaggregates (53–250 μm), and free < 53 µM (silt and clay) fraction which were then oven-dried at 60°C.
A subsample of the macroaggregate fraction was further separated. Briefly, 15 g of oven-dried macroaggregates were slaked in deionized water for 5 min. These samples were then gently shaken with 50 stainless-steel bearings (4 mm dia.) while submerged on top of a 250-μm sieve until all macroaggregates were broken. A continuous stream of water flushed the <250 μm material through the mesh in order to avoid the disruption of occluded microaggregates released from the macroaggregates. Further sieving of the <250 μm fraction through a 53 μm sieve resulted in three size fractions isolated from the macroaggregates, i.e., occluded POM (>250 μm), occluded microaggregates (53–250 μm) and occluded <53 µm (silt and clay). Each of these fractions was rinsed into pre-weighed aluminum pans, oven-dried at 60°C.
Soil carbon and nitrogen concentration and isotopic composition were measured at the Cornell Stable Isotope Laboratory in Ithaca, NY, using a Finnigan MAT Delta Plus mass spectrometer following combustion with an elemental analyzer (Carlo Erba NC2500; Thermo Finnigan, San Jose, CA, United States).
Study 3: Soil Metagenomics
We used soil from all 11 sites to assess environmental metagenomics. DNA was extracted using the MoBio PowerSoil DNA Isolation Kit following the manufacturer's protocol. Triplicate DNA extractions from the same soil core were combined to account for inner-core variability and to ensure sufficient DNA for sequencing. This was done for each of the three samples from the 11 sites, yielding a total of 33 DNA samples for sequencing. The DNA was sequenced at the Cornell University Institute of Biotechnology and was carried out using the NextSeq500 platform yielding 150 base long, single end reads.
Quality control was carried out using tools available at kbase (Arkin et al., 2018). Trimming of adapters and read quality assessment was carried out using trimmomatic using the kbase default settings (Bolger et al., 2014). Sample quality was then assessed using FastQC (Andrews, 2010), with default settings.
Sample dissimilarity analysis was performed using Mash with default settings on all reads. Mash utilizes a shared k-mer-based approach to compare sample read profiles and generate pairwise dissimilarity measures (Ondov et al., 2016). Sequence comparisons to identify reads derived from N-oxide genes were done using DIAMOND (Buchfink et al., 2015). For DIAMOND, sequence reads were used as a query in a blastx analysis against custom N-oxide protein data sets obtained from sequenced genomes available at JGI IMG. The maximum e-value for DIAMOND was set to 1 × 10–3 and downstream filtering limited alignments to those with a sequence identity of ≥60% and a contiguous match length of ≥25 amino acids. All reported read abundances are scaled to reads per million total reads to account for differing sequencing depths. Details are given in Nadeau et al. (2019).
Functional analysis of the metagenomic reads was determined using an open-sourced, stand-alone pipeline FMAP (Kim et al., 2016). Gene sequences were mapped to Kyoto Encyclopedia of Genes and Genomes (KEGG) Orthologies (KOs) database (Kanehisa et al., 2007, 2016) and were binned into pathways and modules. Default settings in FMAP were used to identify reads and to assign enrichment significance in the log2 (fold change) in the normalized read occurrence in comparisons of the triplicate samples between particular sites. In default mode differential abundances are determined using the Kruskal-Wallis rank-sum tests with normalization done using reads per kilobase per million.
Data Analyses
The preceding techniques produced three data sets: 1) carbon, nitrogen, pH and water content of bulk soil; 2) distribution of bulk soil among aggregate fractions; and, 3) gene abundances. We subjected all to simple exploratory analysis, calculating means +standard error (S.E., N = 3).
Site differences were evaluated with one-way Analysis of Variance (ANOVA). The low statistical power in the experimental design main effects (n = 11 sites; n = 2 +/− nitrogen fixers; n = 3 land use; n = 2 soil types) and interactions constrained our ability to draw real biological inferences with a full factorial ANOVA. Furthermore, the unbalanced experimental design with the present-day Farm, made it difficult to determine if non-significant results were caused by a true lack of differences or simply an artifact of sample size. Also, we follow the suggestion of Amrhein et al. (2019) and report means, estimates of variation, sample size, and p-values.
We used a repeated-measures ANOVA to evaluate how the aggregate fractions of soil varied across nine sites. A repeated measures design is the appropriate test because aggregate fractions are not independent of each other.
Differential responses by soils to the presence of nitrogen-fixing plants were assessed using Cohen's d effect sizes (Nakagawa and Cuthill, 2007). Cohen's d standardizes the direction and magnitude of the response by comparing where nitrogen-fixing plants (+ fixer) are present to the paired site with no fixer (− fixer). The units of d are Standard Deviations of the effect, and the effect is taken as statistically significantly different when the 95% confidence interval of d does not cross zero. This test is considered more informative than the t-test for paired comparisons, which provides only a dichotomous decision of significantly different, or not. The effect size test gives additional information on the magnitude of the difference (Sullivan and Feinn, 2012).
To examine the direct relationships, we calculated correlations among all pairs of variables in the data set. We examined both linear (Pearson r) and non-linear (Spearman rank rho) relationships. The large number of pairwise comparisons increased the likelihood of Type II errors (false negatives), and thus we used a Bonferroni correction (significance level P, times the number of variables, divided by the number of pairwise comparisons). This increased the confidence in positive findings at the cost of disregarding weaker correlations.
We did not employ higher-level multivariate statistical analysis for relationships among the data sets because assign variables to be dependent upon other variables could lead to spurious conclusions. Specifically, we now know there are feedbacks among soil organic matter, soil structure, and microbial community structure (Neal et al., 2020). For example, microbial community structure could depend upon soil organic matter and soil structure, whereas organic matter and soil structure could depend upon the soil microbial community involved in soil development. Therefore, correlation avoids spurious relationships.
Given the large number of genes in the metagenomic data set, we attempted to reduce the number of pairwise comparisons. To do this we relied on the KEGG database for orthhology and pathways (see below).
Results
Study 1: Bulk Soils
Concentrations of carbon and nitrogen in bulk soils varied significantly across the 11 sites (Table 1). However, values in soils from both the Restored Upland and Restored Wetland sites were statistically similar to values in soils in the Farm (Ps > 0.05). In comparison, concentrations of carbon and nitrogen in soils from the Restored Upland sites were about 50% of the values in soils from the Reference Upland. Likewise, soils in the Restored Wetlands had significantly lower concentrations of carbon and nitrogen than soils in the Reference Wetlands.
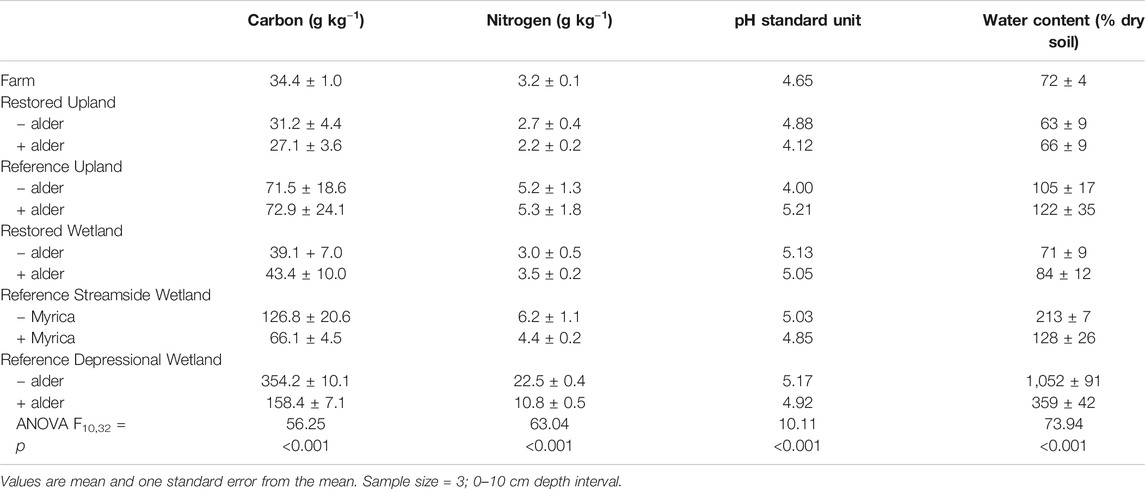
TABLE 1. Carbon and nitrogen concentrations and pH of soils from the 11 sites investigated in this study.
Nitrogen-fixing plants had a significant effect on carbon and nitrogen in bulk soils for all of the pairs of sites, except for the Reference Upland (Table 2). Positive values for Cohen's d in Table 2 indicate lower concentrations of carbon and nitrogen associated with nitrogen fixers, and thus the negative value in the Restored Wetland indicates that nitrogen-fixing alder enhanced concentrations of carbon and nitrogen in the bulk soils. Soil pH values ranged from about 4.00 to 5.21 with more acidic values associated with nitrogen fixers, except in the Reference Forest which had the highest pH among the soils measured. In the Reference wetlands, saturated soil water content was much less in sites with nitrogen fixers compared to paired sites without fixers.

TABLE 2. Standardized mean effect sizes Cohen's d and 95% confidence intervals (CI) for the influence of nitrogen-fixing plants in five location on bulk soil carbon, nitrogen, and pH.
Study 2: Soil Aggregates
The proportion of bulk soil in the four (free) aggregate fractions (Table 3) varied significantly across nine sites (repeated-measures ANOVA F3,78 = 13.55, p < 0.0001). Macroaggregates were the largest fraction in soil from the present-day Farm, followed by free microaggregates then free <53 µm size class. In the other sites macroaggregates also were the largest fraction but its proportion was significantly greater in soils with nitrogen-fixing plants. Without nitrogen fixers, macroaggregates were generally <50% of the bulk soil and free microaggregates and the < 53 µm size were about 2-times more abundant compared to the paired site with alder trees.
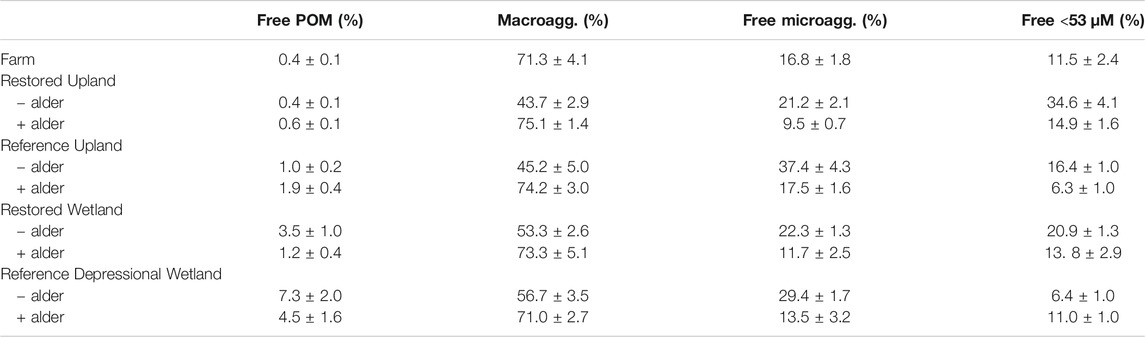
TABLE 3. Distribution of bulk soil mass in four free fractions of hierarchical aggregates from nine sites investigated in this study.
However, for the distribution of soil mass among the three fractions (occluded) within macroaggregates (Table 4), the analysis did not show a significant difference among sites (repeated-measures ANOVA F2,52 = 0.33, p = 0.7194). Yet a few patterns were evident. In the Restored Upland and Restored Wetland sites occluded microaggregates and the occluded <53 µm size class were about equal proportions in each site with values of about 15% each to 45% each. In contrast, in the Reference Upland and Reference Wetland sites, the occluded microaggregate fraction was larger than the occluded <53 µm size class.
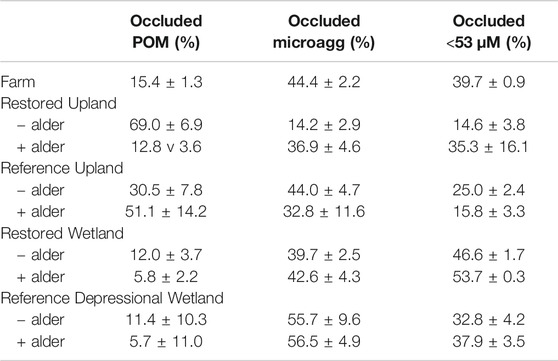
TABLE 4. Distribution of macroaggregate soil in three occluded fractions (occluded) from nine sites investigated in this study.
For most of the aggregate fractions, concentrations of carbon and nitrogen as well as the natural abundance of their stable isotopes varied statistically (p < 0.0071 Bonferroni Corrected) across the nine sites (Table 5). Across sites, the three occluded fractions accounted for more than 50% of soil carbon and soil nitrogen (Figure 1), with the proportion being more pronounced in the Restored sites than in the Reference sites. The distribution of carbon and nitrogen among these three occluded fractions varied considerably, with the occluded microaggregate fraction being the largest in some, but not all cases. Overall, however, more than 50% of the soil carbon and soil nitrogen was associated with free plus occluded microaggregate fractions.
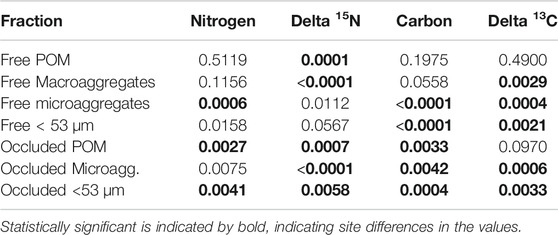
TABLE 5. Statistical p values averaged across nine sites investigated in this study for concentrations of nitrogen and carbon and natural abundance of stable isotopes in different fractions of hierarchical aggregates.
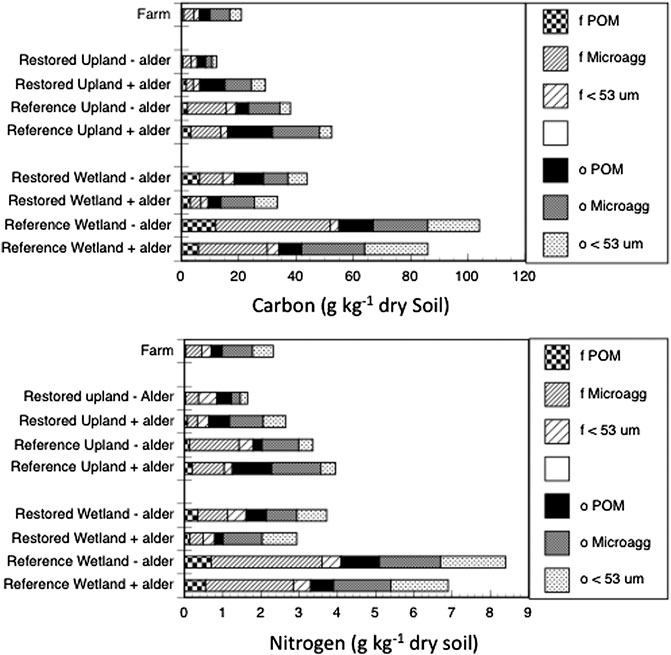
FIGURE 1. Carbon and nitrogen in aggregate fractions of soil from nine sites. Bars are free fractions in bulk soil (f) and fractions occluded within macroaggregates (o).
The natural abundance of stable carbon and nitrogen isotopes showed lighter values in free POM than in the free microaggregate and free < 53 µm size class (Figure 2A). Notable, however, are enriched values for stable nitrogen isotopes in free POM from the soil from three sites: Farm, Restored Upland - alder, and Restored Wetland -alder. Although values for stable isotopes were more variable across the nine sites for occluded fractions, there was still a trend of heavier values between occluded POM vs. occluded microaggregates and occluded < 53 µm size class, except for stable carbon isotopes in soil from the Restored Upland - alder and Restored Wetland–alder (Figure 2B).
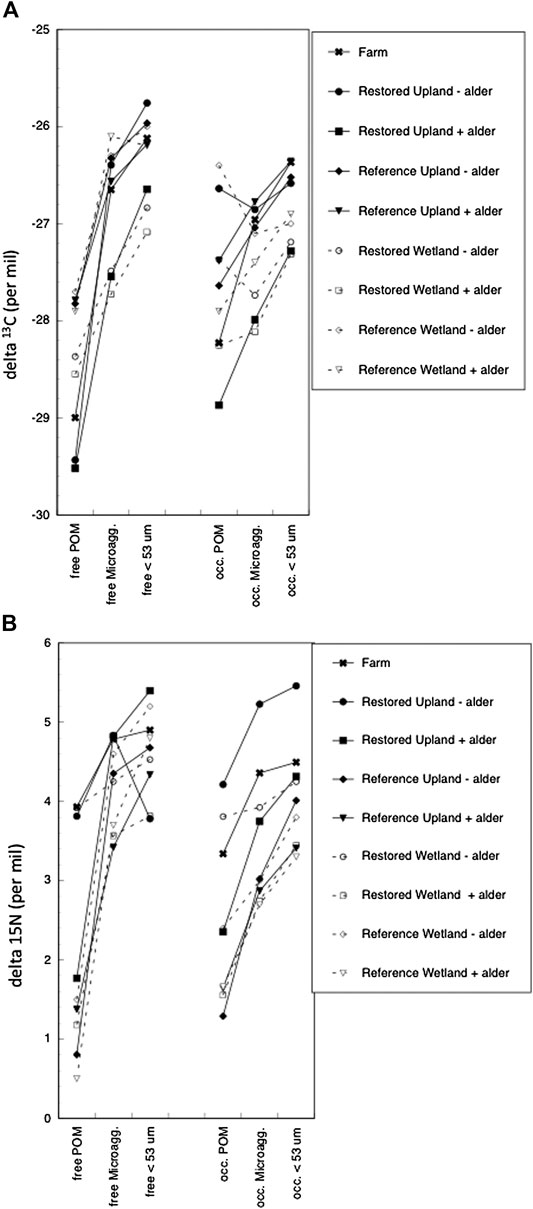
FIGURE 2. Natural-abundance of stable isotope ratios for carbon (A) and nitrogen (B) in aggregates of bulk soils from nine sites.
Study 3: Soil Metagenomics
A PCA plot based on a comparison of all the metagenomic reads (Figure 3) indicated that, with only a few exceptions, sites showed differences along axis one but there was some clustering of sites along axis 2. For example, the Restored Upland + alder site grouped with the Reference Upland along axis 1, whereas the Restored Upland-alder and the two Restored Wetland sites grouped with the Farm soil along axis 2. For the four Reference Wetland sites, the two streamside sites +/− Myrica clustered with each other, but for the Reference Wetland +/− alder the two sites were more dissimilar to each other.
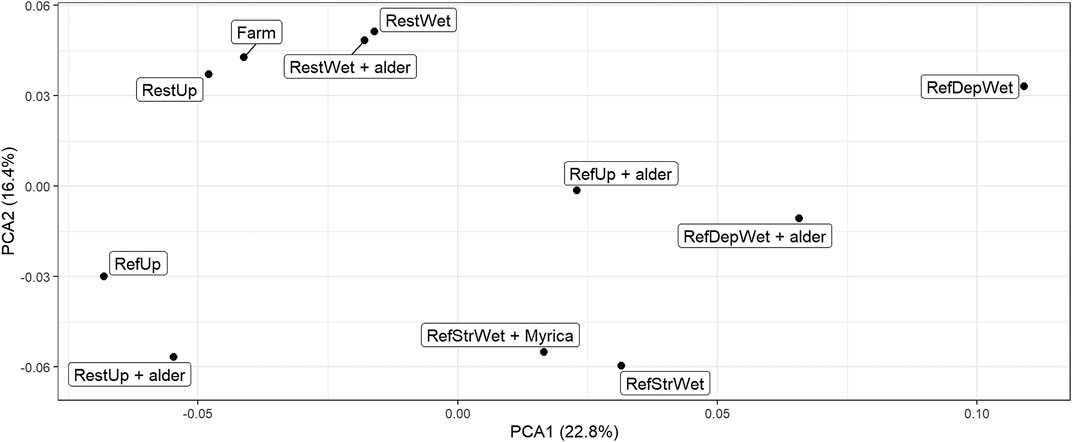
FIGURE 3. PCA plot of metagenomic sample composition from the 11 sites using total metagenomic reads. Triplicate read sets were concatenated into one set for this comparison giving one point per site. Sample abbreviations are: RestUp–Restored Upland, RefUp–Reference Upland, RestWet–Restored Wetland, RefStrWet–Reference Streamside Wetland and RefDepWet–Reference Depressional Wetland.
Given the experimental design with sites +/− alder and the impact of added nitrogen, a more focused read analysis was made for the genes involved in denitrification (Figure 4). Reads from nitrate reductases, the initial step in denitrification, were in most sites mainly from the respiratory form (Nar) instead of the periplasmic form (Nap). The exceptions were the Restored Upland +/− alder and the Reference Upland-alder where occurrence of reads from both forms were similar. All sites had similar levels of reads assigned to nap. There was only limited variation in the occurrence of reads for nirK, which encodes the copper-containing nitrite reductase, with the Restored Wetland +/− alder having slightly higher read values than the other sites. Reads from the gene encoding the cd1-type reductase, nirS, were relatively low in all sites and showed limited variation. Reads for the cytochrome c-oxidizing nitric oxide reductase (Nor), cnor, showed a similar pattern to nirS in that they were relatively low occurrence in the reads and showed little difference between the sites. Reads from the quinol oxidizing Nor, qnor, occurred at significantly higher numbers than cnor. qnor reads also showed high variation within sample replicates, particularly in the Farm samples. Reads from the gene encoding the nitrous oxide reductase, nosZ, were relatively low in number but exhibited a somewhat similar pattern among sites as reads from the nar gene, in that samples from the reference upland +/− alder and the Reference Upland - alder had lower read occurrence than the other sites.
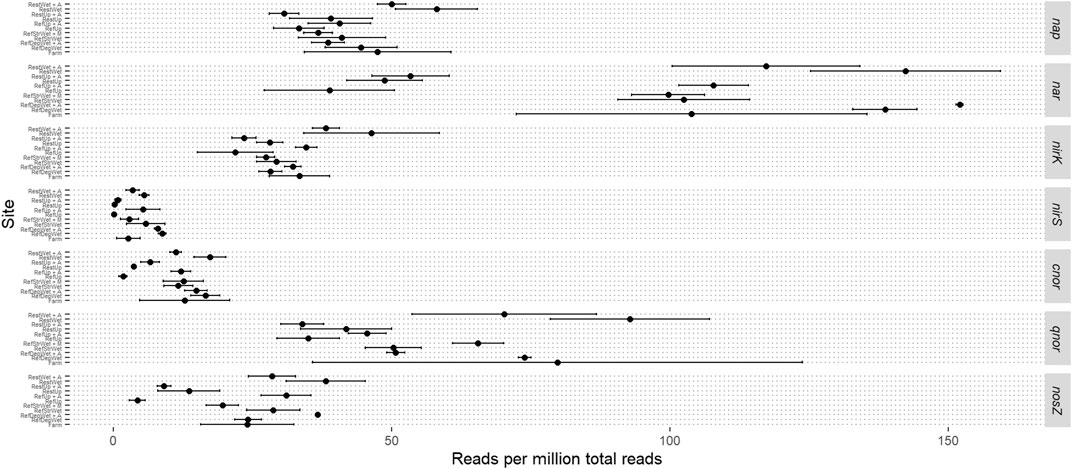
FIGURE 4. Mean read abundance from individual denitrification genes. Sample abbreviations are: RestUp–Restored Upland, RefUp–Reference Upland, RestWet–Restored Wetland, RefStrWet–Reference Streamside Wetland and RefDepWet–Reference Depressional Wetland. Error bars represent one standard deviation from the mean.
Given the experimental design with well-drained vs. poorly drained soil and potential methane production, a more focused read analysis was done for the mcrA gene as a marker for methanogenesis (Table 6). Reads coming from mcrA were most prominent in the Reference Wetland +/− alder, but significantly less in the portion where alder trees. Read abundance in the Reference Wetland +/- Myrica was lower than the Reference Wetland +/− alder and did not show a difference whether the nitrogen fixer, Myrica, was present, or not. Reads from mcrA were relatively more frequent in the Restored wetland and again with greater abundance in the situation without alder than when the tree was present. mcrA read abundance was below detection in the Farm and Upland sites. Reads from the methane oxidation gene pmoA were found in all 11 sites. The relative frequency of the gene was significantly less in the Restored Upland sites than in the Reference Forest sites. Interestingly, relative abundance of pmoA reads in the Restored Wetland sites were similar to values for the Reference Wetland sites.
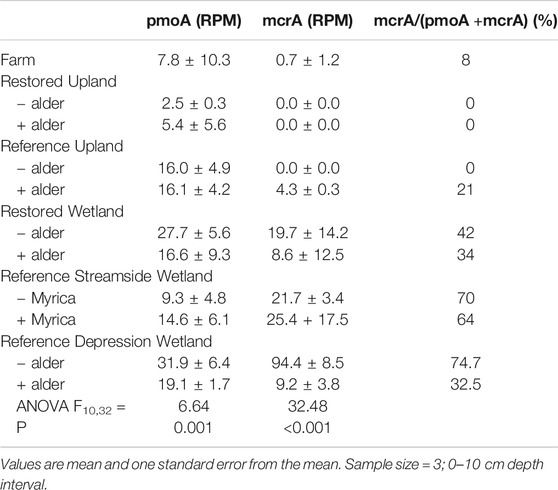
TABLE 6. Occurrence of genes associated with either methane oxidation genes (pmoA) or methanogenesis (mcrA) and normalized ratio of genes from 11 sites investigated in this study.
To gain a broader view of bacterial community structure we used FMAP, which assigns function to reads using the KEGG ortholog database as reference (Kim et al., 2016). Given the experimental design with a large number of pairwise comparisons of sites (N = 55 pairs of sites), as well as the number of ortholog sequences in the KEGG database (>20,000), we only report the number of genes that were significantly enriched in some of the more relevant pairwise comparisons (Table 7). These differences serve as a proxy for similarities or differences in the physiological potential in the bacterial community. As expected, pairwise comparisons of sites that were adjacent in the PCA plot (Figure 3) generated relatively low numbers of orthologs with significant enrichment in one or the other of the paired sites. For example, for the Reference Streamside Wetlands +/− Myrica, which were fairly close on both PCA1 and PCA2, there were 93 orthologs that were significantly enriched in one or the other. In contrast, sites that showed large dissimilarity in the PCA plot had a relatively larger number of orthologs that showed significant enrichment in one or the other of the paired sites. For example, there were 2,286 significantly enriched orthologs in a pairwise comparisons of reads from the Reference upland - alder sites to those from the Reference Depressional Wetland - alder.
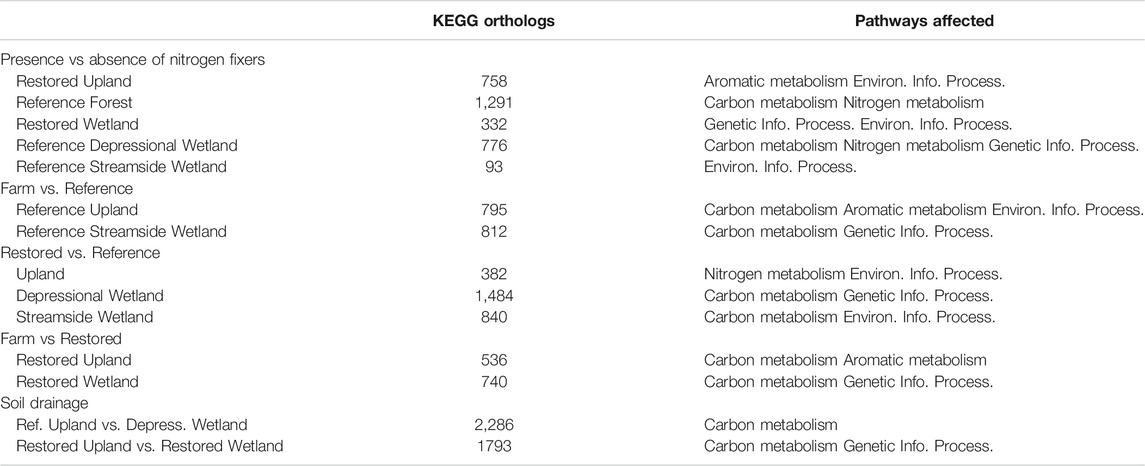
TABLE 7. Number of KEGG orthologs with statistically significant greater abundance in pairwise comparison between sites. KEGG Pathways affected by differential read abundance.
The FMAP data (Table 7) also show that restoration of the soil bacterial community has progressed further in the uplands than in the wetlands. The restored uplands have only 382 KEGG orthologs with differential abundance between Restored and Reference, whereas there are still 536 KEGG orthologs with differential abundance between Restored and Farm. In contrast, the restored wetlands have 840 KEGG orthologs with differential abundance between Restored and Reference Streamside Wetland and 740 KEGG orthologs with differential abundance between Restored and Farm.
Discussion
General
The recovery of post-agricultural land is undoubtedly related to its land use history (Bell et al., 2020). The work of Flinn and Marks (2007) provide a useful description of the agricultural history in the region that includes our study sites. Tompkins County in New York State was completely forested in 1790 prior to European colonization. Land use change for agriculture accelerated after 1850 reaching a peak in 1900. Dairy farming was the predominant form of agriculture resulting in a mix of pasture, hay and other crops. Woods, brush, and fallow were intermixed across the landscape. Widespread farm abandonment in the 1900s allowed forests to reclaim much of the landscape, and by 1995 forests covered 54% of the county (Flinn et al., 2005). Wetlands in the region were drained, mostly by ditching and dikes to channel water away and create more agricultural land (Walters and Shrubsole, 2003). Many of the wetlands were likely covered with soil that eroded from uplands (McCarty et al., 2009) that buried remnants of the original wetland, making it difficult to know the endpoint per se for wetland restoration.
A pertinent question in agriculture is the amount of nitrogen fertilizer used. Unfortunately, we do not have a complete record for the site. However, widespread use of synthetic fertilizers occurred mostly after 1960 (Davidson, 2009) and might not have been extensive in the region due to its excessive cost. Since synthetic fertilizers are produced by fixation of atmospheric nitrogen, they typically have a natural abundance for stable nitrogen isotope value of 0 (Nikolenko et al., 2018), similar to that for plants with symbiotic nitrogen fixation (Soper et al., 2015). But this did not dominate values for bulk soils or soil aggregates (discussed below). However, we cannot rule out manure having been applied to the soils, which generally has an isotope value of >+5 (Bateman et al., 2005), which is similar the that for decomposed organic matter.
Bulk Soils
Unfortunately, we also do not know the level of organic matter in bulk soil when agriculture was abandoned on the site. Nevertheless, comparison of the present-day Farm with the Reference Upland sites suggests that agriculture reduced bulk soil carbon by about 55%, whereas nitrogen was reduced by about 40% (Table 1). This reduction in soil carbon is larger than the reduction of 30% in soil carbon often assumed to occur as a consequence of cultivation (Davidson and Ackerman, 1993; Murty et al., 2002). Thus, over-worked soil is likely one reason agriculture was abandoned on the site.
For the poorly drained soils, our findings suggest bulk soils lost more than 80% of its carbon and 50% of its nitrogen as a result of agriculture (Table 1). There is some evidence in the literature that soils in poorly drained areas suffer less agriculture-induced soil carbon losses compared to better-drained soils (Jelinski and Kucharik, 2009). However, it is difficult to generalize about the fate of the missing carbon and nitrogen in the study area. For instance, agriculture increases the likelihood of wind erosion of the soil, in particular, for depressional wetlands. This occurred in relatively deep soils in depression wetlands along the south shore of Lake Ontario in New York State that were converted to agriculture and were productive for only about 50 years due to drastic loss of soil (Kojima, 1947), The rate of soil loss was much faster than could be explained by microbial decomposition of plant residue and soil organic matter (Welsch and Yavitt, 2003, 2007) suggesting that the fine texture organic matter was wind eroded when fields were fallow.
Thus, our findings provide virtually no evidence that organic matter levels have recovered in the bulk soil samples since agricultural abandonment. This is perhaps not surprising as recovery of carbon and nitrogen in post-agricultural soils can be a slow process (McLauchlan, 2006), with estimates ranging from tens to several hundred years (Jandl et al., 2007; Krause et al., 2016). A slow recovery rate also suggests a long mean residence time for the pool of soil organic matter. According to reservoir theory (Eriksson, 1971), recovery from agricultural practices takes, at least, one residence time and three residence times for nearly complete recovery.
The findings here did not present a clear picture for alder contributions to restoration in these sites (Table 2). Typically, it is assumed that nitrogen added via fixation simulates plant production, which ultimately increases soil organic matter (Knops et al., 2002). Although this seems to be occurring in the Restored Wetland, there was no evidence for increased soil organic matter associated with alder in the Restored Upland site. Also, none of the Reference sites had greater amounts of soil organic matter from plants associated with nitrogen fixation. One possible explanation is that plants growing with nitrogen fixers do take-up the extra nitrogen, but the outcome is merely plant tissue that decomposes more readily (Hoogmoed et al., 2014; Averill and Waring, 2018)), without enhancing soil organic matter.
Soil Structure
Our study of soil aggregation produced several unanticipated findings. Despite the well-known finding that macroaggregates and, perhaps, even free microaggregates are disrupted by tillage (Six et al., 2000), macroaggregates were a prominent feature of bulk soils in the present-day Farm. The finding here is likely related to the type of agricultural practice used in the region, which is rotation of corn, hay, clover, and cover crops. At the time of sampling, the present-day Farm had a mixture of grasses and clover. Common pasture grasses tend to be colonized by arbuscular mycorrhizal fungi, some of which are very good at promoting soil aggregation (Lehmann et al., 2017). Indeed, Milne and Haynes (2004) showed that some grasses can restore aggregate stability quite quickly especially when tillage does not occur annually. In contrast, macroaggregates being a smaller proportion of the bulk soil in the Restored Upland-alder site might be related to dominance by goldenrod plants (Solidago sp.) that have been shown to have little impact on soil structure (Zubek et al., 2020). The contrasts in soil aggregation among sites in our survey show there is still much to be learned about plant species' influences on soil aggregation.
For example, our findings show that alder roots are particularly good at promoting soil aggregation. This is likely related to the diversity of mycorrhizae associated with alder roots. Alder forms well-known relationships with ectomycorrhizal fungi (Põlme et al., 2013) and actinobacterial Frankia, which is responsible for the nitrogen-fixing capacity. However, young alder trees also often form associations with arbuscular mycorrhizal fungi (Chatarpaul et al., 1989) that are much better at soil aggregate formation. Indeed, Enkhtuya and Vosatka (2005) showed that mycorrhizae on young alder trees were able to make direct connections with grasses that further promoted soil aggregation. This suggests a benefit of alder promoting soil structure that goes beyond its nitrogen-fixing capability.
Across the sites, the predominance of soil carbon and nitrogen within macroaggregates is not surprising, given the well-known protection afforded organic matter when physically removed from soil decomposers (Lehmann et al., 2020). Furthermore, both free and occluded microaggregates had the largest amount of soil carbon and nitrogen. According to the hierarchy theory of aggregate formation (Tisdall and Oades, 1982) microaggregates form more readily within macroaggregates, and thus free microaggregates are thought to be those released from macroaggregates via disturbance, i.e., tillage. Although the timing of microaggregate formation depends on many soil factors (Totsche et al., 2018) it is generally believed to occur on a century rather than decade time scale. Thus, it is likely in our sites have not had enough time for complete formation of microaggregates occluded within macroaggregates. Therefore, the free microaggregates were likely inherited from pre-agriculture soil and released during the agricultural phase.
Although the stable isotope values for POM were generally lighter than values for microaggregates and <53 µm size class, of particular interest are the relatively large (enriched) values for stable nitrogen isotopes in free POM from the Farm and Restored Upland and Restored Wetland sites (Figure 2). The most likely explanation for heavier values in POM is extensive decomposition of the relatively fresh plant residue that is the origin of free POM in the mineral soil. For microaggregates and the <53 µm size class the relatively large (enriched) values for stable isotopes also suggest that protected organic matter is what remains after microbial decomposition. It is possible that the protected organic matter was derived from manure applied to the soil during the agricultural phase. Regardless of the source, and despite some evidence in the literature for fresher organic matter in occluded fractions in restored sites (cf., Kalinina et al., 2018), the results point to well decomposed organic material being protected with soil aggregation. This finding seems to apply across all the land uses, farm, restored, and reference, whereby fresher organic matter that is unprotected decomposes quickly. This is perhaps not surprising and has been observed in other systems (Liao et al., 2006).
Soil Metagenomes
Many studies have accessed the soil microbial community in terms of taxonomic identity of community members and statistical inference of turnover in community composition among treatment sites (cf., Turley et al., 2020). Here we focused on gene abundances instead of taxonomic identity as gene abundances provide deeper insight into the microbial processes that are occurring among sites, i.e., via metabolic pathways (cf. Yavitt et al., 2020).
As a result, we found that only one of the restored sites, the Restored Upland + alder, clustered with a plausible recovery endpoint, the Reference Upland − alder (Figure 3). The other three Restored sites showed closer affinity to the present-day Farm, suggesting relatively minor recovery of soil bacterial community structure. We have no a priori reason why the Restored Upland + alder site showed recovery. Since the site had alder, this gives a role for alder trees in restoration of post-agricultural soils that extends beyond the symbiotic nitrogen-fixing capacity of the tree.
Examination of gene abundances does help us resolve the appropriate endpoint for restoration of the post-agricultural wetlands, i.e., Depressional or Streamside. We do not know with certainty since the original wetland was lost long before we sampled the soils. However, the Restored Wetland had a soil bacterial metagenome with closer affinity to the Reference Streamside Wetland (+/− Myrica) than the Depressional Wetland (+/− alder). This conclusion is based on separation of the Restored Wetland from the Reference Depressional Wetland on the first axis of the PCA plot, whereas separation from the Reference Streamside Wetland occurred on the second axis. This indicates greater dissimilarity on the first axis (Lever et al., 2017). Another reason is there being fewer significantly different orthologs between the Restored Wetland and the Reference Streamside Wetland than a similar comparison but with the Reference Depressional Wetland (Table 7). Both types of wetlands occur in the region. Therefore, comparisons of metagenomes appear to be an insightful way to link habitats together at the landscape scale.
The examination of denitrification genes revealed only a few notable differences among sites. On one hand, this is surprising since the landscape contained soil ranging from well drained to poorly drained, with an a priori prediction of greater presence of denitrification genes in the poorly drained soils (Groffman and Tiedje, 1989). On the other hand, homogenization of the landscape during the agricultural period might have eliminated differences that have been slow to recover (Zhu et al., 2017). Moreover, there was no consistent effect of nitrogen fixers, alder or Myrica, on the abundance of denitrification genes. One notable exception was in the Reference Upland, where, for most of the denitrification genes, abundance was greater in the situations with alder than without. Presumably, this reflects the addition of fixed nitrogen to the well-drained soil with a long-established presence of alder.
For methane cycling, reads associated with methane oxidation (pmoA) but no reads associated with methane production (mcrA) in the well-drained soils suggest that the sole source of methane was atmospheric. This is well-known and has been observed many times in a wide range of soils (cf., Cai et la. 2016). Here greater abundance of pmoA reads in the Reference Forest than in Farm and Restored soils suggest that methane-oxidizing bacteria were impaired by agriculture and have been slow to recovery. There is evidence that the recovery of atmospheric methane consumption might take more than 50 years in post-agricultural soils (Hudgens and Yavitt, 1997). Although differences in the abundance of reads for pmoA occurred here, the three KEGG pathways associated with methane oxidation (M00344 xylulose monophosphate pathway; M00345 ribulose monophosphate pathway; and, M00346 serine pathway) did not show significant differences in any of the pairwise comparisons. Hence, further work is needed to assess whether rates of atmospheric methane consumption differ across the landscape.
All of the poorly drained soils showed mcrA reads, which is not surprising for wetland soils. Here the number of reads was statistically similar between the Restored Wetlands and Reference Wetland soils, suggesting rapid recovery of methane production capacity when wetland hydrology is restored. How mcrA persists in a landscape when, presumably, all of the wetlands have been drained, farmed, and allowed to dry is still unclear.
A straightforward interpretation of community structure provided by FMAP is an index of dissimilarity, in this case in gene abundances, between pairs of sites. This view of dissimilarity is akin to dissimilarity in community composition used by ecologists (Anderson et al., 2011). The largest differences, in our cases, between sites with different soil drainage (Table 7) makes sense since soil drainage, well drained vs. poorly drained, has shown to influence bacterial community composition (Suriyavirun et al., 2019). The FMAP analyses also support the conclusion that soil restoration is occurring faster for the Upland sites than the Wetland sites.
It is perhaps not surprising that most of the genes still showing differential abundances between pairs of sites were in KEGG pathways carbon and nitrogen metabolism, genetic and environmental information processing, and aromatic metabolism (Table 7). The Carbon Metabolism group likely reflects catabolism of new compounds in the soil derived from shifting patterns of plant species associated with farming, restoration, and natural vegetation. This is consistent with dissimilarity in the Environmental Information Processing pathway, which largely consists of genes involved in transport of a variety of compounds across cell membranes (Rice et al., 2014). Genetic Information Processing includes genes involved in repair of nucleic acids but also transposable elements. Transposable elements are often associated with the spread of novel catabolic genes within communities (Sun and Badgley, 2019).
Relationships in the Data Set
Admittedly, the study has a complex experimental design with 11 sites, two soil drainage classes, forested and non-forested sites, and current, past and not any agricultural practices. Although complex, such post-agricultural landscapes are common. A complication is how the three soil parameters examined here (organic matter, structure, and gene abundances in the bacterial community) are dependent on each other, which is less clear than often presented. We hesitate assigning cause and effect intuitively among the three parameters to avoid spurious relationships.
Therefore, we rely upon correlation analyses (Table 8). For example, our finding strong relationships between percentage of occluded microaggregates and abundance of denitrification reads (Pearson r = 0.49), pmoA reads (r = 0.62), and mcrA reads (r = 0.53) go along with the explanation that denitrification and methane cycling occur within these highly protected soil structures (cf., Neal et al., 2020). On the other hand, before labeling strong correlations between the percentage of occluded microaggregates and concentrations of carbon and nitrogen (r = 0.62) as organic matter protection, it is important to recognize that carbon and nitrogen compounds are the glue that hold microaggregates together.
Conclusion
It is an exciting time to study soil recovery from agricultural practices. The potential for carbon sequestration in soil organic matter has implications for atmospheric carbon cycling and global climate, despite still being uncertain (cf., Clark and Johnson, 2011). Long-term sequestration of nitrogen has implications for downstream water quality (cf., Van Meter et al., 2016). Increased genomic information is offering insight into the physiological potential of the bacterial community not possible even five years ago. Integrating bacterial functions with the dynamics of soil organic matter and soil structure is a challenge. However, linking soil organic matter to structure to soil organisms will generate a robust understanding of soil recovery.
Data Availability Statement
The datasets presented in this study can be found in online repositories. The names of the repository/repositories and accession number(s) can be found below: NCBI BioProject, accession no: PRJNA687526.
Author Contributions
JY and GP conceived the study. JY, GP, EO, and JZ did the field work. GP, EO, and JZ carried out the laboratory analyze in support of the study. JY and JS analyzed the findings and wrote the initial manuscript. All authors contributed to the final version of the manuscript.
Funding
This work was supported by a USDA National Institute of Food and Agriculture, Hatch project (1477309). Any opinions, findings, conclusions, or recommendations expressed in this publication are those of the author (s) and do not necessarily reflect the view of the National Institute of Food and Agriculture (NIFA) or the United States Department of Agriculture (USDA).
Conflict of Interest
The authors declare that the research was conducted in the absence of any commercial or financial relationships that could be construed as a potential conflict of interest.
Acknowledgments
We thank the Finger Lakes Land Trust (https://www.fllt.org) for allowing access to the field locations for scientific research. We thank ad hoc reviewers for comments and suggestions that greatly improved the clarity of the presentation.
References
Amrhein, V., Greenland, S., and McShane, B. (2019). Scientists rise up against statistical significance. Nature 567, 305–307. doi:10.1038/d41586-019-00857-9
Anderson, M. J., Crist, T. O., Chase, J. M., Vellend, M., Inouye, B. D., Freestone, A. L., et al. (2011). Navigating the multiple meanings of β diversity: a roadmap for the practicing ecologist. Ecol. Lett. 14, 19–28. doi:10.1111/j.1461-0248.2010.01552.x
Andrews, S. (2010). FastQC: a quality control tool for high throughput sequence data. Reference Source. doi:10.1109/hpcs.2010.5547068
Arkin, A. P., Cottingham, R. W., Henry, C. S., Harris, N. L., Stevens, R. L., Maslov, S., et al. (2018). KBase: the United States department of energy systems biology knowledgebase. Nat. Biotechnol. 36, 566. doi:10.1038/nbt.4163
Aronson, J., Dhillion, S., and Le Floc'h, E. (1995). On the need to select an ecosystem of reference, however imperfect: a reply to Pickett and Parker. Restor. Ecol. 3, 1–3.
Averill, C., and Waring, B. (2018). Nitrogen limitation of decomposition and decay: how can it occur?. Global Change Biol. 24, 1417–1427. doi:10.1111/gcb.13980
Ballantine, K., and Schneider, R. (2009). Fifty‐five years of soil development in restored freshwater depressional wetlands. Ecol. Applic. 19, 1467–1480. doi:10.1890/07-0588.1
Bateman, A. S., Kelly, S. D., and Jickells, T. D. (2005). Nitrogen isotope relationships between crops and fertilizer: implications for using nitrogen isotope analysis as an indicator of agricultural regime. J. Agr. Food Chem. 53, 5760–5765. doi:10.1021/jf050374h
Bell, S. M., Barriocanal, C., Terrer, C., and Rosell-Melé, A. (2020). Management opportunities for soil carbon sequestration following agricultural land abandonment. Environ. Sci. Pol. 108, 104–111. doi:10.1016/j.envsci.2020.03.018
Bernard, J. M., and Solsky, B. A. (1977). Nutrient cycling in a Carex lacustris wetland. Can. J. Bot. 55, 630–638. doi:10.1139/b77-077
Bolger, A. M., Lohse, M., and Usadel, B. (2014). Trimmomatic: a flexible trimmer for Illumina sequence data. Bioinformatics 30, 2114–2120. doi:10.1093/bioinformatics/btu170
Buchfink, B., Xie, C., and Huson, D. H. (2015). Fast and sensitive protein alignment using DIAMOND. Nat. Method. 12, 59–60. doi:10.1038/nmeth.3176
Bünemann, E. K., Bongiorno, G., Bai, Z., Creamer, R. E., De Deyn, G., de Goede, R., et al. (2018). Soil quality–a critical review. Soil Biol. Biochem. 120, 105–125. doi:10.1016/j.soilbio.2018.01.030
Cai, Y., Zheng, Y., Bodelier, P. L., Conrad, R., and Jia, Z. (2016). Conventional methanotrophs are responsible for atmospheric methane oxidation in paddy soils. Nat. Commun. 7, 11728–11810. doi:10.1038/ncomms11728
Carter, M. R. (2002). Soil quality for sustainable land management. Agron. J. 94, 38–47. doi:10.2134/agronj2002.0038
Castañeda, L. E., and Barbosa, O. (2017). Metagenomic analysis exploring taxonomic and functional diversity of soil microbial communities in Chilean vineyards and surrounding native forests. Peer J 5, e3098. doi:10.7717/peerj.3098
Chatarpaul, L., Chakravarty, P., and Subramaniam, P. (1989). Studies in tetrapartite symbioses. Plant Soil 118, 145–150. doi:10.1007/bf02232800
Clark, J. D., and Johnson, A. H. (2011). Carbon and nitrogen accumulation in post‐agricultural forest soils of western New England. Soil Sci. Soc. Am. J. 75, 1530–1542. doi:10.2136/sssaj2010.0180
Cohen, M. J., Creed, I. F., Alexander, L., Basu, N. B., Calhoun, A. J., Craft, C., et al. (2016). Do geographically isolated wetlands influence landscape functions?. Proc. Natl. Acad. Sci. USA 113, 1978–1986. doi:10.1073/pnas.1512650113
Crews, T. E., and Rumsey, B. E. (2017). What agriculture can learn from native ecosystems in building soil organic matter: a review. Sustainability 9, 578. doi:10.3390/su9040578
Davidson, E. A., and Ackerman, I. L. (1993). Changes in soil carbon inventories following cultivation of previously untilled soils. Biogeochemistry 20, 161–193. doi:10.1007/bf00000786
Davidson, E. A. (2009). The contribution of manure and fertilizer nitrogen to atmospheric nitrous oxide since 1860. Nat. Geosci. 2, 659–662. doi:10.1038/ngeo608
Dyer, J. M. (2006). Revisiting the deciduous forests of eastern North America. Bioscience 56, 341–352. doi:10.1641/0006-3568(2006)56[341:rtdfoe]2.0.co;2
Elliott, E. T. (1986). Aggregate structure and carbon, nitrogen, and phosphorus in native and cultivated soils. Soil Sci. Soc. Am. J. 50, 627–633. doi:10.2136/sssaj1986.03615995005000030017x
Enkhtuya, B., and Vosatka, M. (2005). Interaction between grass and trees mediated by extraradical mycelium of symbiotic arbuscular mycorrhizal fungi. Symbiosis 38, 261–277.
Eriksson, E. (1971). Compartment models and reservoir theory. Annu. Rev. Ecol. Systemat. 2, 67–84. doi:10.1146/annurev.es.02.110171.000435
Fierer, N. (2017). Embracing the unknown: disentangling the complexities of the soil microbiome. Nat. Rev. Microbiol. 15, 579–590. doi:10.1038/nrmicro.2017.87
Flinn, K. M., Lechowicz, M. J., and Waterway, M. J. (2008). Plant species diversity and composition of wetlands within an upland forest. Am. J. Bot. 95, 1216–1224. doi:10.3732/ajb.0800098
Flinn, K. M., and Marks, P. L. (2007). Agricultural legacies in forest environments: tree communities, soil properties, and light availability. Ecol. Applic. 17, 452–463. doi:10.1890/05-1963
Flinn, K. M., Vellend, M., and Marks, P. L. (2005). Environmental causes and consequences of forest clearance and agricultural abandonment in central New York, United States. J. Biogeogr. 32, 439–452. doi:10.1111/j.1365-2699.2004.01198.x
Flinn, K. M., and Vellend, M. (2005). Recovery of forest plant communities in post‐agricultural landscapes. Front. Ecol. Environ. 3, 243–250. doi:10.1890/1540-9295(2005)003[0243:rofpci]2.0.co;2
Graf, F., and Frei, M. (2013). Soil aggregate stability related to soil density, root length, and mycorrhiza using site-specific Alnus incana and Melanogaster variegatus sl. Ecol. Eng. 57, 314–323. doi:10.1016/j.ecoleng.2013.04.037
Groffman, P. M., and Tiedje, J. M. (1989). Denitrification in north temperate forest soils: relationships between denitrification and environmental factors at the landscape scale. Soil Biol. Biochem. 21, 621–626. doi:10.1016/0038-0717(89)90054-0
Hermans, S. M., Taylor, M., Grelet, G., Curran-Cournane, F., Buckley, H. L., Handley, K. M., et al. (2020). From pine to pasture: land use history has long-term impacts on soil bacterial community composition and functional potential. FEMS Microbiol. Ecol. 96, fiaa041. doi:10.1093/femsec/fiaa041
Hoogmoed, M., Cunningham, S. C., Baker, P., Beringer, J., and Cavagnaro, T. R. (2014). N-fixing trees in restoration plantings: effects on nitrogen supply and soil microbial communities. Soil Biol. Biochem. 77, 203–212. doi:10.1016/j.soilbio.2014.06.008
Hudgens, D. E., and Yavitt, J. B. (1997). Land-use effects on soil methane and carbon dioxide fluxes in forests near Ithaca, New York. Ecoscience 4, 214–222.doi:10.1080/11956860.1997.11682398
Hurd, T. M., Raynal, D. J., and Schwintzer, C. R. (2001). Symbiotic N. Oecologia 126, 94–103. doi:10.1007/s004420000500
Jandl, R., Lindner, M., Vesterdal, L., Bauwens, B., Baritz, R., Hagedorn, F., et al. (2007). How strongly can forest management influence soil carbon sequestration?. Geoderma 137, 253–268. doi:10.1016/j.geoderma.2006.09.003
Jansson, J. K., and Hofmockel, K. S. (2018). The soil microbiome—from metagenomics to metaphenomics. Curr. Opin. Microbiol. 43, 162–168. doi:10.1016/j.mib.2018.01.013
Jelinski, N. A., and Kucharik, C. J. (2009). Land‐use effects on soil carbon and nitrogen on a United States Midwestern Floodplain. Soil Sci. Soc. Am. J. 73, 217–225. doi:10.2136/sssaj2007.0424
Kalinina, O., Cherkinsky, A., Chertov, O., Goryachkin, S., Kurganova, I., de Gerenyu, V. L., et al. (2018). Post-agricultural restoration: implications for dynamics of soil organic matter pools. Catena 181, 104096. doi:10.1016/j.catena.2019.104096
Kanehisa, M., Araki, M., Goto, S., Hattori, M., Hirakawa, M., Itoh, M., et al. (2007). KEGG for linking genomes to life and the environment. Nucleic Acids Res. 36, D480–D484. doi:10.1093/nar/gkm882
Kanehisa, M., Sato, Y., Kawashima, M., Furumichi, M., and Tanabe, M. (2016). KEGG as a reference resource for gene and protein annotation. Nucleic Acids Res. 44, D457–D462. doi:10.1093/nar/gkv1070
Kim, J., Kim, M. S., Koh, A. Y., Xie, Y., and Zhan, X. (2016). FMAP: functional mapping and analysis pipeline for metagenomics and metatranscriptomics studies. BMC Bioinf. 17, 420. doi:10.1186/s12859-016-1278-0
Knops, J. M. H., Bradley, K. L., and Wedin, D. A. (2002). Mechanisms of plant species impacts on ecosystem nitrogen cycling. Ecol. Lett. 5, 454–466. doi:10.1046/j.1461-0248.2002.00332.x
Kojima, R. T. (1947). Soil organic nitrogen: I. Nature of the organic nitrogen in a muck soil from Geneva, New York. Soil Sci. 64, 157–165. doi:10.1097/00010694-194708000-00007
Krause, A., Pugh, T. A., Bayer, A. D., Lindeskog, M., and Arneth, A. (2016). Impacts of land-use history on the recovery of ecosystems after agricultural abandonment. Earth Syst. Dynam. 7, 745–766. doi:10.5194/esd-7-745-2016
Kuzyakov, Y., and Zamanian, K. (2019). Reviews and syntheses: agropedogenesis-humankind as the sixth soil-forming factor and attractors of agricultural soil degradation. Biogeosciences 16, 4783–4803. doi:10.5194/bg-16-4783-2019
Lal, R. (2015). Restoring soil quality to mitigate soil degradation. Sustainability 7, 5875–5895. doi:10.3390/su7055875
Lehmann, A., Leifheit, E. F., and Rillig, M. C. (2017). “Mycorrhizas and soil aggregation,” in Mycorrhizal mediation of soil. Editors N. Collins Johnson, C. Gehring, and J. Jansa (Amsterdam: Elsevier), 241–262.
Lehmann, J., Hansel, C. M., Kaiser, C., and Markus, K., Kate, M., Stefano, M., et al. (2020). Persistence of soil organic carbon caused by functional complexity. Nat. Geosci. 13, 529–534. doi:10.1038/s41561-020-0612-3
Lever, J., Krzywinski, M., and Altman, N. (2017). Points of significance: principal component analysis. Nat. Methods 14, 641–642. doi:10.1038/nmeth.4346
Liao, J. D., Boutton, T. W., and Jastrow, J. D. (2006). Organic matter turnover in soil physical fractions following woody plant invasion of grassland: evidence from natural 13C and 15N. Soil Biol. Biochem. 38, 3197–3210. doi:10.1016/j.soilbio.2006.04.004
Magdoff, F., and Weil, R. R. (2004). “Soil organic matter management strategies,” in Soil organic matter in sustainable agriculture. F. Magdoff, and R. R. Weil (Boca Raton: CRC Press), 45–65.
McCarty, G., Pachepsky, Y., and Ritchie, J. (2009). Impact of sedimentation on wetland carbon sequestration in an agricultural watershed. J. Environ. Qual. 38, 804–813. doi:10.2134/jeq2008.0012
McLauchlan, K. (2006). The nature and longevity of agricultural impacts on soil carbon and nutrients: a review. Ecosystems 9, 1364–1382. doi:10.1007/s10021-005-0135-1
Mehlich, A. (1984). Mehlich 3 soil test extractant: a modification of Mehlich 2 extractant. Commun. Soil Sci. Plant Anal. 15, 1409–1416.
Milne, R. M., and Haynes, R. J. (2004). Soil organic matter, microbial properties, and aggregate stability under annual and perennial pastures. Biol. Fertil. Soils 39, 172–178. doi:10.1007/s00374-003-0698-y
Murty, D., Kirschbaum, M. F. M. M., and McGilvray, R. E. H. (2002). Does conversion of forest to agricultural land change soil carbon and nitrogen? A review of the literature. Global Change Biol. 8, 105–123. doi:10.1046/j.1354-1013.2001.00459.x
Nadeau, S. A., Roco, C. A., Debenport, S. J., Anderson, T. R., Hofmeister, K. L., Walter, M. T., et al. (2019). Metagenomic analysis reveals distinct patterns of denitrification gene abundance across soil moisture, nitrate gradients. Environ. Microbiol. 21, 1255–1266. doi:10.1111/1462-2920.14587
Nakagawa, S., and Cuthill, I. C. (2007). Effect size, confidence interval and statistical significance: a practical guide for biologists. Biol. Rev. 82, 591–605. doi:10.1111/j.1469-185X.2007.00027.x
Neal, A. L., Bacq-Labreuil, A., Zhang, X., Clark, I. M., Coleman, K., Mooney, S. J., et al. (2020). Soil as an extended composite phenotype of the microbial metagenome. Sci. Rep.-UK 10, 1–16. doi:10.1038/s41598-020-67631-0
Nemergut, D. R., Schmidt, S. K., Fukami, T., O'Neill, S. P., Bilinski, T. M., Stanish, L. F., et al. (2013). Patterns and processes of microbial community assembly. Microbiol. Mol. Biol. Rev. 77, 342–356. doi:10.1128/MMBR.00051-12
Nikolenko, O., Jurado, A., Borges, A. V., Knӧller, K., and Brouyѐre, S. (2018). Isotopic composition of nitrogen species in groundwater under agricultural areas: a review. Sci. Total Environ. 621, 1415–1432. doi:10.1016/j.scitotenv.2017.10.086
Ondov, B. D., Treangen, T. J., Melsted, P., Mallonee, A. B., Bergman, N. H., Koren, S., et al. (2016). Mash: fast genome and metagenome distance estimation using MinHash. Genome Biol. 17, 132. doi:10.1186/s13059-016-0997-x
Põlme, S., Bahram, M., Yamanaka, T., Nara, K., Dai, Y. C., Grebenc, T., et al. (2013). Biogeography of ectomycorrhizal fungi associated with alders (Alnus spp.) in relation to biotic and abiotic variables at the global scale. New Phytol. 198, 1239–1249. doi:10.1111/nph.12170
Preem, J. K., Truu, J., Truu, M., Mander, Ü., Oopkaup, K., Lõhmus, K., et al. (2012). Bacterial community structure and its relationship to soil physico-chemical characteristics in alder stands with different management histories. Ecol. Eng. 49, 10–17. doi:10.1016/j.ecoleng.2012.08.034
Rice, A. J., Park, A., and Pinkett, H. W. (2014). Diversity in ABC transporters: type I, II and III importers. Crit. Rev. Biochem. Mol. Biol. 49, 426–437. doi:10.3109/10409238.2014.953626
Schwintzer, C. R. (1979). Nitrogen fixation by Myrica gale root nodules Massachusetts wetland. Oecologia 43, 283–294. doi:10.1007/BF00344955
Scott, N. A. (1998). Soil aggregation and organic matter mineralization in forests and grasslands: plant species effects. Soil Sci. Soc. Am. J. 62, 1081–1089. doi:10.2136/sssaj1998.03615995006200040032x
Six, J. Α. Ε. Τ., Elliott, E. T., and Paustian, K. (2000). Soil macroaggregate turnover and microaggregate formation: a mechanism for C sequestration under no-tillage agriculture. Soil Biol. Biochem. 32, 2099–2103. doi:10.1016/s0038-0717(00)00179-6
Soper, F. M., Boutton, T. W., and Sparks, J. P. (2015). Investigating patterns of symbiotic nitrogen fixation during vegetation change from grassland to woodland using fine scale δ15 N measurements. Plant Cell Environ. 38, 89–100. doi:10.1111/pce.12373
Stover, M. E., and Marks, P. L. (1998). Successional vegetation on abandoned cultivated and pastured land in Tompkins County, New York. J. Torrey Bot. Soc. 125, 150–164. doi:10.2307/2997302
Sullivan, G. M., and Feinn, R. (2012). Using effect size—or why the P value is not enough. J. Grad. Med. Educ. 4, 279–282. doi:10.4300/JGME-D-12-00156.1
Sun, S., and Badgley, B. D. (2019). Changes in microbial functional genes within the soil metagenome during forest ecosystem restoration. Soil Biol. Biochem. 135, 163–172. doi:10.1016/j.soilbio.2019.05.004
Suriyavirun, N., Krichels, A. H., Kent, A. D., and Yang, W. H. (2019). Microtopographic differences in soil properties and microbial community composition at the field scale. Soil Biol. Biochem. 131, 71–80. doi:10.1016/j.soilbio.2018.12.024
Tisdall, J. M., and Oades, J. M. (1982). Organic matter and water-stable aggregates in soils. J. Soil Sci. 33, 141–163. doi:10.1111/j.1365-2389.1982.tb01755.x
Totsche, K. U., Amelung, W., Gerzabek, M. H., Guggenberger, G., Klumpp, E., Knief, C., et al. (2018). Microaggregates in soils. J. Plant Nutr. Soil Sci. 181, 104–136. doi:10.1002/jpln.201600451
Turley, N. E., Bell‐Dereske, L., Evans, S. E., and Brudvig, L. A. (2020). Agricultural land‐use history and restoration impact soil microbial biodiversity. J. Appl. Ecol. 57, 852–863. doi:10.1111/1365-2664.13591
Van Meter, K. J., Basu, N. B., Veenstra, J. J., and Burras, C. L. (2016). The nitrogen legacy: emerging evidence of nitrogen accumulation in anthropogenic landscapes. Environ. Res. Lett. 11, 035014. doi:10.1088/1748-9326/11/3/035014
Walters, D., and Shrubsole, D. (2003). Agricultural drainage and wetland management in Ontario. J. Environ. Manag. 69, 369–379. doi:10.1016/j.jenvman.2003.09.013
Welsch, M., and Yavitt, J. B. (2003). Early stages of decay of Lythrum salicaria L. and Typha latifolia L. in a standing-dead position. Aquat. Bot. 75, 45–57. doi:10.1016/s0304-3770(02)00164-x
Welsch, M., and Yavitt, J. B. (2007). Microbial CO2 production, CH4 dynamics and nitrogen in a wetland soil (New York State, United States) associated with three plant species (Typha, Lythrum, Phalaris). Eur. J. Soil Sci. 58, 1493–1505. doi:10.1111/j.1365-2389.2007.00955.x
Yavitt, J. B., Roco, C. A., Debenport, S. J., Barnett, S. E., and Shapleigh, J. P. (2020). Community organization and metagenomics of bacterial assemblages across local scale pH gradients in northern forest soils. Microb. Ecol. doi:10.1007/s00248-020-01613-7
Zhu, Y. G., Gillings, M., Simonet, P., Stekel, D., Banwart, S., and Penuelas, J. (2017). Microbial mass movements. Science 357, 1099–1100. doi:10.1126/science.aao3007
Zubek, S., Majewska, M. L., Kapusta, P., Stefanowicz, A. M., Błaszkowski, J., and Rożek, K. (2020). Solidago canadensis invasion in abandoned arable fields induces minor changes in soil properties and does not affect the performance of subsequent crops. Land Degrad. Dev. 31, 334–345. doi:10.1002/ldr.3452
Keywords: natural abundance stable isotopes, New York State, restoration, shotgun metagenomics, soil aggregates, wetlands
Citation: Yavitt JB, Pipes GT, Olmos EC, Zhang J and Shapleigh JP (2021) Soil Organic Matter, Soil Structure, and Bacterial Community Structure in a Post-Agricultural Landscape. Front. Earth Sci. 9:590103. doi: 10.3389/feart.2021.590103
Received: 31 July 2020; Accepted: 07 January 2021;
Published: 08 February 2021.
Edited by:
Matthias Peichl, Swedish University of Agricultural Sciences, SwedenReviewed by:
Ember Morrissey, West Virginia University, United StatesChristopher Craft, Indiana University, United States
Manqiang Liu, Nanjing Agricultural University, China
Copyright © 2021 Yavitt, Pipes, Olmos, Zhang and Shapleigh. This is an open-access article distributed under the terms of the Creative Commons Attribution License (CC BY). The use, distribution or reproduction in other forums is permitted, provided the original author(s) and the copyright owner(s) are credited and that the original publication in this journal is cited, in accordance with accepted academic practice. No use, distribution or reproduction is permitted which does not comply with these terms.
*Correspondence: Joseph B. Yavitt, amJ5MUBjb3JuZWxsLmVkdQ==