- 1Regional Climate Group, Department of Earth Sciences, University of Gothenburg, Gothenburg, Sweden
- 2State Key Laboratory of Hydraulics and Mountain River, Sichuan University, Chengdu, China
- 3State Key Laboratory of Hydroscience and Engineering, Department of Hydraulic Engineering, Tsinghua University, Beijing, China
- 4Jet Propulsion Laboratory, California Institute of Technology, Pasadena, CA, United States
- 5Joint Institute for Regional Earth System Science and Engineering, University of California, Los Angeles, CA, United States
- 6Department of Earth System Science, Tsinghua University, Beijing, China
Recent climate change has induced widespread soil thawing and permafrost degradation in the Tibetan Plateau. Significant advances have been made in better characterizing Tibetan Plateau soil freeze/thaw dynamics, and their interaction with local-scale ecohydrological processes. However, factors such as sparse networks of in-situ sites and short observational period still limit our understanding of the Tibetan Plateau permafrost. Satellite-based optical and infrared remote sensing can provide information on land surface conditions at high spatial resolution, allowing for better representation of spatial heterogeneity in the Tibetan Plateau and further infer the related permafrost states. Being able to operate at “all-weather” conditions, microwave remote sensing has been widely used to retrieve surface soil moisture, freeze/thaw state, and surface deformation, that are critical to understand the Tibetan Plateau permafrost state and changes. However, coarse resolution (>10 km) of current passive microwave sensors can add large uncertainties to the above retrievals in the Tibetan Plateau area with high topographic relief. In addition, current microwave remote sensing methods are limited to detections in the upper soil layer within a few centimetres. On the other hand, algorithms that can link surface properties and soil freeze/thaw indices to permafrost properties at regional scale still need improvements. For example, most methods using InSAR (interferometric synthetic aperture radar) derived surface deformation to estimate active layer thickness either ignore the effects of vertical variability of soil water content and soil properties, or use site-specific soil moisture profiles. This can introduce non-negligible errors when upscaled to the broader Tibetan Plateau area. Integrating satellite remote sensing retrievals with process models will allow for more accurate representation of Tibetan Plateau permafrost conditions. However, such applications are still limiting due to a number of factors, including large uncertainties in current satellite products in the Tibetan Plateau area, and mismatch between model input data needs and information provided by current satellite sensors. Novel approaches to combine diverse datasets with models through model initialization, parameterization and data assimilation are needed to address the above challenges. Finally, we call for expansion of local-scale observational network, to obtain more information on deep soil temperature and moisture, soil organic carbon content, and ground ice content.
Introduction
The Tibetan Plateau has an average elevation of 4,000 m and encompasses an area of approximately 2.5 × 106 km2 (Figure 1). It is the home to ∼100,000 km2 of glaciers (Yao et al., 2012) and possesses the largest alpine permafrost area in the world (Ran et al., 2018; Cheng et al., 2019). The Tibetan Plateau is also the headwater of many major Asian rivers including the Yangtze, Yellow, Mekong, Indus, and Ganges (Immerzeel et al., 2010). Due to its vast domain and high elevation, the Tibetan Plateau is extremely sensitive to climate change and has a profound influence on the regional climate (Duan and Wu, 2005).
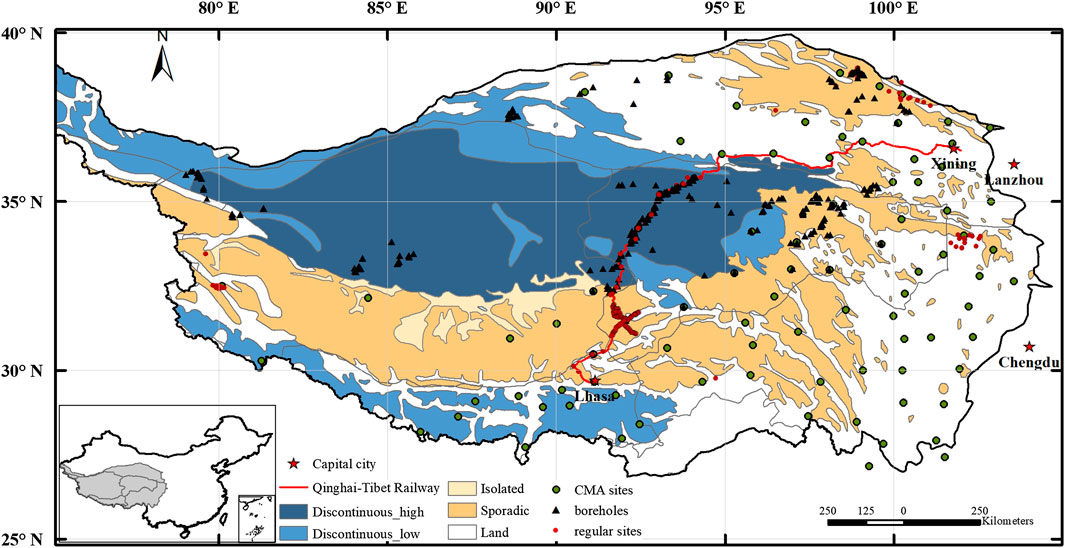
FIGURE 1. The distribution of permafrost and in-situ observations over the Tibetan Plateau region. The permafrost classification is based on the international permafrost association permafrost map (Brown et al., 1997), and the “high” and “low” in the legend refer to the ice content of permafrost. Green dots represent meteorological stations from China Meteorological Administration, black triangles refer to the borehole sites with deeper soil temperature measurements from previous studies (Wu and Zhang, 2008; Luo et al., 2012; Cao et al., 2019), and red dots are regular observation sites with soil temperature observations within a depth of a few meters (data source including Yang et al., 2013a; Cao et al., 2019; Zheng et al., 2020).
Long-term in-situ surface meteorology measurements show that the Tibetan Plateau has been experiencing a significant warming trend since 1960s, with an average rate of 0.3–0.4°C per decade, which exceeds the global average during the same period (Chen et al., 2015a). Previous studies further indicated such warming trend is elevation-dependent. Specifically, the warming rate increases with elevation for lower altitude regions (<∼4,500–5,000 m) and this phenomenon is more obvious during autumn and winter (Yan and Liu, 2014), while this warming trend is absent or lower at higher elevations (>∼5,000 m) based on satellite-based temperature datasets (Guo et al., 2019; Pepin et al., 2019). Other changes include slight increases in precipitation, wind speed weakening, solar radiation declining and mixed trends of relative humidity (Yang et al., 2014; Bibi et al., 2018). These changes can have significant impact on regional water and energy balance, and cause non-negligible changes in the cryosphere, including glacier retreat, soil warming and permafrost degradation.
Frozen ground occupies the most area of the Tibetan Plateau (Figure 1), with approximately 40% coverage of permafrost and 55% coverage of seasonally frozen ground (Zou et al., 2017). Since the 1970s, several in-situ observation networks have been established to monitor the thermal state of Tibetan Plateau frozen ground (Yang et al., 2010). These in-situ soil temperature records have demonstrated substantial changes occurred in Tibetan Plateau frozen ground including permafrost degradation over the past few decades. For example, the in-situ observations in the Beiluhe region indicated that the active layer thickness has increased at a rate of ∼4.26 cm yr−1 from 2002 to 2012 while the permafrost temperatures at 10 m depth have increased by ∼0.14°C during the same period (Wu et al., 2015). In the inner Tibetan plateau, maximum frozen depth decreased at a rate of 0.71 cm yr−1 during the period 1967–1997 (Zhao et al., 2004), and reduction in the maximum frozen depth has accelerated since 2000 (Peng et al., 2017). Previous study also revealed that a distinct talik layer separating the permafrost from overlying active layer has already formed along the Qinghai-Tibet Highway (Jin et al., 2008).
Previous studies based on the in-situ observations provided detailed assessments of permafrost thermal state at local scales. However, extrapolating site-level observations across the Tibetan Plateau is likely to entail large uncertainties, due to the sparse in-situ stations, shallow observation depth at most sites, inconsistency in the measurement methods and data gaps. Most in-situ observations and boreholes (up to 10 m) were established along the Qinghai-Tibet Railway/Highway to meet the engineering demand (Wu and Zhang, 2008; Jin et al., 2011; Wu et al., 2020), while most of the meteorological stations from China Meteorological Administration are located in the seasonally frozen ground of the central or eastern Tibetan Plateau (Figure 1).There are limited in-situ observations in the western Tibetan Plateau, with only a few boreholes located in A-erh-chin Mountain, Gaize and west Kunlun (Zou et al., 2017). The limited number of in-situ sites poses great challenges for a comprehensive, regional-scale assessment of the Tibetan Plateau permafrost thermal state. For example, there are large discrepancies among the current Tibetan Plateau permafrost coverage maps (Zou et al., 2017; Wu et al., 2018; Zheng et al., 2020), and it is difficult to accurately evaluate these maps due to the different compilation approaches and insufficient in-situ observations (Cao et al., 2019).
Satellite or airborne remote sensing can provide information on environmental conditions and freeze/thaw state related to underlying permafrost properties with improved sensitivity to subsurface soil properties (Jorgenson and Grosse, 2016), which can provide critical constraints on assessing regional permafrost vulnerability. With a wide range of satellite and airborne observations available from existing and upcoming missions, remote sensing data are increasingly becoming an essential element for regional permafrost monitoring (NRC, 2014). However, current satellite remote sensing systems cannot provide information on deeper (>∼10 cm) soils; therefore, an integration of remote sensing data with process-based models are needed to obtain information on permafrost properties and dynamics. This review summarizes recent progress and challenges using multi-source satellite remote sensing data and process-based models to improve regional permafrost monitoring across Tibetan Plateau. Airborne remote sensing also plays an important role in regional permafrost studies (Miller et al., 2019). For example, the airborne electromagnetic method has shown a great potential in mapping Alaskan permafrost (Minsley et al., 2012; Rey et al., 2019). However, there is very limited airborne experiment in the Tibetan Plateau; therefore, we only briefly discuss its application in regional permafrost studies, which mainly occur in other permafrost areas (Discussion).
The paper is structured as follows: we first discuss the unique characteristics of Tibetan Plateau permafrost environment (Environmental Controls on Tibetan Plateau Permafrost Distribution and Soil Freeze/Thaw Dynamics), and then summarize the recent advances in regional Tibetan Plateau permafrost studies using various remote sensing technologies, and through combination of remote sensing with modeling approaches (Regional Monitoring Approaches and Associated Challenges). We also discuss the unique challenges in Tibetan Plateau permafrost monitoring, the potential of geophysical measurements and other methods to characterize subsurface variability and spatial heterogeneity in permafrost areas (Discussion). We conclude by addressing research priorities and future studies needed to accurately simulate the evolution of Tibetan Plateau permafrost and eco-hydrology (Research Priorities and Recommendations).
Environmental Controls on Tibetan Plateau Permafrost Distribution and Soil Freeze/Thaw Dynamics
The Tibetan Plateau is characterized by relatively thin and warm permafrost with low ice content, potentially more vulnerable to ongoing and future warming due to the unique environmental conditions, arid climate, high elevation and steep geothermal gradient (Wang and French, 1995; Yang et al., 2010; Zhao et al., 2010; Cheng et al., 2019; Zhao et al., 2020). Therefore, an improved understanding of permafrost sensitivity to environmental conditions in the Tibetan Plateau region is essential for effectively monitoring potential changes and vulnerability of Tibetan Plateau permafrost (Figure 2).
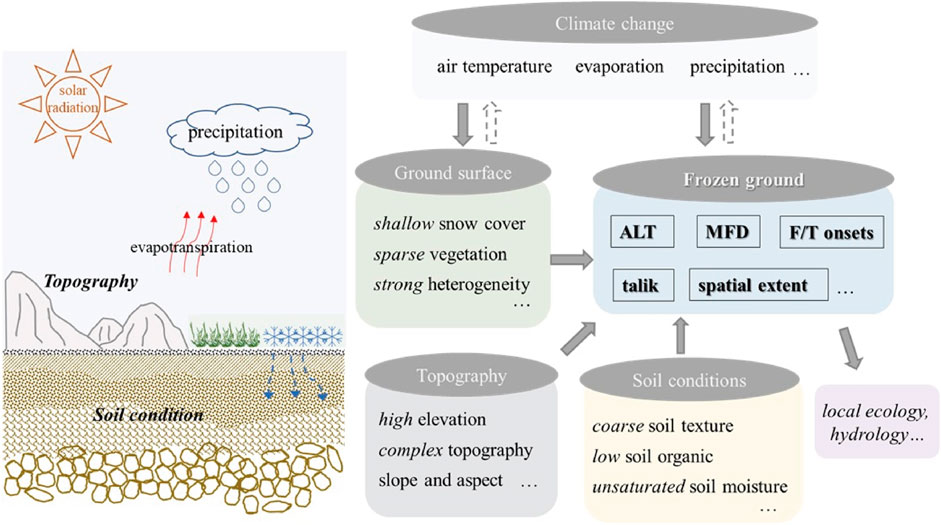
FIGURE 2. Interaction among different environmental factors and soil freeze/thaw (F/T) dynamics in the Tibetan Plateau region. Solid and dash arrows indicate the influences and feedbacks respectively. ALT and MFD represent active layer thickness (defined for permafrost area) and maximum frozen depth (defined for seasonally frozen ground) respectively.
Permafrost distribution in the Tibetan Plateau is controlled by the high elevation and complex topography, and has characteristics different from permafrost in other regions (Wang and French, 1995). Topographic factors such as elevation affect regional climate through the effects on precipitation, temperature lapse rates and solar radiation loading (Gruber et al., 2017). Therefore, topography can affect soil freeze/thaw dynamics by altering the land surface temperature and the surface energy budget. Field investigations show that the lower limit of permafrost in the Yellow River region is ∼4,400 a.s.l., while seasonally frozen ground occurs at lower elevations such as major river valleys (Luo et al., 2012). Other topographic factors, such as aspect and slope, also influence permafrost distribution. Due to different solar radiation loading on different slope and aspect, the lower limit of Tibetan Plateau permafrost on the north slope is usually lower than that on the south slope with more sunny conditions (Cheng and Wu, 2007). In-situ data from the central Tibetan Plateau show that the average frost depth was much deeper on north facing slopes than on south facing slopes, and varied considerably with elevation on both slopes (Ma et al., 2015).
Soil conditions also have a significant influence on permafrost thermal state. Soil texture is one of the major factors determining soil thermal conductivity, heat capacity, and hydraulic conductivity (Chen et al., 2012; Yi et al., 2018a). For example, gravel soils have a larger thermal conductivity and can result in a rapid response of soil temperatures to climate change. The existence of gravels also tends to reduce the soil water holding capacity and enhance hydraulic conductivity and drainage (Pan et al., 2017). As the consequence of slow soil-forming processes and strong erosion, gravel soils are widespread in the Tibetan Plateau and has distinct physical properties from agricultural soils (Arocena et al., 2012; Yi et al., 2018a). In addition, given that bedrock has quite different hydrological and thermal properties from upper soil layers, soil depth (or the depth to bedrock) also has significant influence on soil freeze/thaw dynamics especially for deep soils. Soil moisture content also affects the soil freeze/thaw processes through soil water phase change and changing physical properties such as soil thermal and hydraulic conductivity (Scherler et al., 2010). Higher soil moisture content is generally associated with a longer zero-curtain period, and thus delay soil freeze/thaw onsets (Luo et al., 2014; Jiang et al., 2018). Tibetan Plateau soils are usually unsaturated and soil moisture shows a strong heterogeneity both vertically and spatially (Yang et al., 2013a), and how it affects the Tibetan Plateau soil freeze/thaw dynamics and permafrost changes remains under investigated (Yang et al., 2010). Moreover, although soil organic content in the Tibetan Plateau is relatively low compared with the northern high-latitude region, it can strongly influence soil thermal conditions due to its correlation with soil properties such as high soil porosity and high soil moisture content (Chen et al., 2012; Zhou et al., 2013).
In addition, surface conditions, such as vegetation cover and snow cover, act as important environmental controls on soil freeze/thaw dynamics and permafrost distribution. Vegetation cover affects the partition among the sensible, latent and soil heat fluxes, and therefore plays an important role in surface water and energy balances (Li et al., 2015a). Although most Tibetan Plateau permafrost region is dominated by alpine meadows or grasslands with sparse vegetation, Wang et al. (2012a) still found that decrease of alpine meadow and alpine swamps in the Tibetan Plateau were related to the increasing sensitivity of soil to climate changes and the greater shifts in soil temperature and water dynamics. Furthermore, different types of vegetation cover may exert different impacts on soil thawing (Wang et al., 2012b). Winter snow cover also has an important effect on soil freeze/thaw dynamics and permafrost temperature (Zhang, 2005; Yi et al., 2015). Snow cover plays an important role in determining how soil responds to surface warming due to its strong insulation effects and impact on surface energy balance associated with changes in surface albedo and snow water phase change (Zhang, 2005). Different from the overall thicker snow cover in the northern high latitude region, snow in the Tibetan Plateau often melts quickly due to strong solar radiation, and snow cover is generally shallow and ephemeral, with overall low albedo of fresh snow and limited insulation effects (Wang et al., 2020; Zheng et al., 2020). Therefore, snow cover in the Tibetan Plateau may have a cooling effect on underlying soil temperature due to the dissipated latent heat resulted from frequent snow melting or sublimation (Zhu et al., 2017). Moreover, snow meltwater that infiltrates into the soil could result in additional soil temperature fluctuations due to soil heat transport through convection and soil water phase change (Scherler et al., 2010; Luo et al., 2014).
Regional Monitoring Approaches and Associated Challenges
Remote Sensing of Tibetan Plateau Permafrost
Current Progress
The sparse distribution of in-situ observation sites over the Tibetan Plateau (Figure 1) highlights the potential of satellite remote sensing to significantly improve regional permafrost monitoring across Tibetan Plateau. Remote sensing technology, including optical, near- and thermal-infrared, passive and active microwave remote sensing, has been used to directly or indirectly monitor the near-surface soil freeze/thaw and permafrost state in the Tibetan Plateau. The wavelength ranges from 0.4–0.7, 0.7–3.0, and 3.0–100 μm for optical, near- and thermal-infrared remote sensing, respectively; microwave remote sensing covers the spectrum from 1 mm to ∼1 m in wavelength and has a much deeper penetration depth (Ulaby et al., 1982). Optical and near-infrared remote sensing are often used to detect the vegetation canopy and land surface properties, while microwave sensors can provide information on land surface moisture and temperature variations due to strong sensitivity to changes in land surface dielectric properties and emissivity. The applications of remote sensing in permafrost can be largely grouped into two categories: 1) identifying and mapping surface features and objects typical for permafrost areas; and 2) retrieving physical variables directly or indirectly relevant to subsurface thermal conditions (Tedesco, 2014). The first category was dominated by the application of high spatial-resolution optical and near-infrared images, while the second category covers a wider range of remote sensing systems from optical to microwave sensors as discussed below.
Permafrost Monitoring Using Optical and Infrared Remote Sensing
Optical and near-infrared remote sensing with high spatial resolutions (<100 m) has been widely used to identify geological structures and landscapes associated with permafrost, e.g., hummock, thermokarst lakes, freeze/thaw boils, and surface classification, which can be used to infer the presence of underlying permafrost (Panda et al., 2010; Wang et al., 2014; Niu et al., 2018; Ran et al., 2018; Huang et al., 2020). Data from some high-resolution satellites such as Landsat-8 and GaoFen-1, 2, can be used to extract the landscape features and structures (Niu et al., 2018). This is especially useful for permafrost research in mountain regions characterized by heterogeneous surface condition like Tibetan Plateau. On the other hand, optical and near-infrared remote sensing can also provide information on land surface variables that can be used as auxiliary data to empirical or process-based models to infer the permafrost thermal state (Wang et al., 2014; Dai et al., 2018; Ran et al., 2018). For example, regional snow cover extent, surface albedo and vegetation cover products, which are available from optical sensors such as MODIS (250-m resolution), Landsat (30-m resolution), and Sentinel-2 (20-m resolution), have been used as model ancillary inputs to simulate the permafrost thermal state due to significant influences of vegetation and snow cover on surface energy and water balance (Niu et al., 2018; Zheng et al., 2019b). In addition, many thermal infrared satellite systems (e.g., Terra, Aqua, and geostationary platforms like GOES and METEOSAT) could monitor the land surface temperature at spatial resolutions of 1–50 km, which is more directly linked to the subsurface ground thermal state (Holmes et al., 2009). Optical and thermal infrared remote sensing can provide information at high spatial resolution; however, it should be noted that factors such as frequent clouds and mixing pixels will introduce large uncertainties to these land surface products, and affect their application in permafrost studies (Kou et al., 2017).
Optical and thermal infrared imaging methods provide valuable regional datasets; however, permafrost is essentially a subsurface phenomenon and these methods are limited to imaging the land surface. Permafrost monitoring requires knowledge of soil temperature and heat transfer at deep layers; therefore, statistical or empirical models have been developed to utilize surface parameters derived from optical and infrared remote sensing to map permafrost extent and distribution (Zou et al., 2017; Aalto et al., 2018; Obu et al., 2019). Statistical models, e.g., decision tree or logistic regression models, have been applied to Tibetan Plateau permafrost mapping through building relationship between permafrost indices and multiple environmental factors including satellite-based land surface temperature and snow cover extent products. More recent studies are also developed using deep learning methods to create Tibetan Plateau permafrost maps (Wang et al., 2019a; Huang et al., 2020). Empirical models such as the TTOP (temperature at the top of permafrost) model and Kudryavtsev model that are derived from simplified heat transfer equations and require fewer inputs have been also widely used in Tibetan Plateau permafrost mapping (Zhao et al., 2017a; Zou et al., 2017).
Permafrost Monitoring Using Microwave Remote Sensing
Microwave remote sensing shows strong sensitivity to soil dielectric changes induced by landscape and particularly soil freeze/thaw state, and can operate under all-weather conditions (Ulaby et al., 2014); therefore, it has been widely used to map surface soil freeze/thaw status and permafrost changes in both high latitude and high elevation regions (e.g., Li et al., 2012a; Li et al., 2013; Park et al., 2016).
Brightness temperature (Tb) measured by passive microwave remote sensing is associated with changes in surface emissivity and temperature conditions, both of which are closely associated with soil freeze/thaw status. Depending on the frequency and its penetration ability, relatively higher frequency (e.g., Ka-band, ∼27–40 GHz) is commonly used to detect the freeze/thaw state of landscape surface elements, while lower frequencies such as L-band (∼1–2 GHz) and P-band (∼250–500 MHz) provide enhanced sensitivity to soil surface and profile freeze/thaw conditions (Du et al., 2015; Naderpour and Schwank, 2018). The Tb at Ka-band shows less sensitivity to freeze/thaw induced changes in surface emissivity, and is more correlated with land surface temperature especially for the vertical polarization (Holmes et al., 2009). A negative spectral gradient between 18 and 37 GHz was also found for the frozen soils due to volume scatter darkening within the frozen soil (Zuerndorfer and England, 1992). Therefore, a combination of low Tb37V (37 GHz Tb at vertical polarization) and negative 18–37 GHz spectral gradient values has been widely used in frozen soil classification (Zhao et al., 2011; Han et al., 2015). Surface conditions such as dry snow may confound the soil freeze/thaw classification, and a number of groups have explored combining different indices to identify different surface conditions. For example, Jin et al. (2009) developed a decision tree algorithm to classify surface soil freeze/thaw state, through incorporating Tb37V, polarization Tb difference at 19 GHz, and a scattering index to distinguish scattering and non-scattering surfaces. Zhao et al. (2011) developed a discriminant algorithm that employs the Tb37V and the quasi-emissivity of Tb18.7H based on model simulations and Fisher linear discrimination analysis of different surface ground conditions. More recent studies also explored the values of L-band Tb in soil freeze/thaw classifications due to its deeper penetration depth (∼50 and ∼5 cm for the frozen and thaw soils, respectively) (Rautiainen et al., 2016; Zheng et al., 2019a).
Despite with high revisit frequency (less than a few days), current passive microwave sensors have the spatial resolutions on the order of 10–50 km, which can add large uncertainties to freeze/thaw classifications in an area with high topographic relief like the Tibetan Plateau (Li et al., 2013). Alternatively, space-borne synthetic aperture radar (SAR) sensors provide measurements of radar backscatter at much finer scales (∼10–100 m) but have a much longer temporal revisit (>10 days). SAR signals share similar frequency-dependent responses to vegetation canopy and surface soil dielectric changes as microwave emission techniques, and radar backscatter at different frequencies (eg C-band, ∼4–8 GHz; Ku-band, ∼12–18 GHz) can be potentially used to characterize surface freeze/thaw and soil moisture status in the Tibetan Plateau (Van der Velde and Su, 2009; Han et al., 2011). The integration of active and passive microwave remote sensing provides a promising way to monitor surface and soil freeze/thaw and soil moisture states of Tibetan Plateau permafrost, leveraging the strengths of each method (Dente et al., 2014). A few studies also explored the value of combining passive microwave remote sensing and MODIS land surface temperature for high-resolution freeze/thaw mapping in the Tibetan Plateau (Zhao et al., 2017b; Kou et al., 2017).
More recently, interferometric synthetic aperture radar (InSAR) has shown great potential for regional mapping of active layer thickness. InSAR works by detecting surface deformation resulting from soil freezing induced uplift and thawing induced subsidence through measuring the phase shifts between repeat pass SAR images acquired at different times (Liu et al., 2012; Li et al., 2015b; Daout et al., 2017; Jia et al., 2017; Wang et al., 2018). It should be noted that this technique requires repeat passes to be obtained with less than 5 m deviation to enable accurate interferograms and phase shifts to be produced. Different techniques have been developed to generate interferograms and reconstruct the long-term series and seasonal trends in the surface deformation, which are linked with the long-term permafrost thaw and seasonal active layer thawing rate, respectively. Empirical approaches have been used to convert the surface deformation to active layer thickness assuming a uniform soil saturation profile (e.g., Liu et al., 2012; Jia et al., 2017). A more recent study accounts for the soil saturation differences among different land cover types in the Tibetan Plateau (Wang et al., 2018), which results in different response curves of surface deformation to thaw depth. Li et al. (2015b) developed a new approach that uses the time lag between the periodic features of InSAR-observed surface deformation and land surface temperature to estimate the time interval between maximum surface air temperature and maximum seasonal thaw depth.
Challenges
Currently, there are no direct remote sensing methods to detect deeper soil freeze/thaw and thermal conditions. Most satellite detection of soil freeze/thaw is generally limited to upper soil layer within a few centimeters of the surface. A potential approach to overcome this limitation is to introduce lower frequency sensors such as P-band SAR for soil profile characterization (Chen et al., 2019) and incorporate multi-frequency microwave observations such as joint L- and P-band SAR for enhanced delineations of soil profile freeze/thaw characteristics (Du et al., 2015). On the other hand, algorithms that can link surface properties and soil freeze/thaw indices to permafrost properties still need improvements. For example, most methods using InSAR derived surface deformation to estimate active layer thickness either ignore the effects of vertical variability of soil water content and soil properties (Liu et al., 2012; Li et al., 2015b; Jia et al., 2017), or use site-specific soil moisture profiles (Wang et al., 2018). This can introduce non-negligible errors when upscaled to the broader Tibetan Plateau, which is characterized by strong heterogeneous soil conditions, deep active layer and more variable soil moisture profiles. A drier surface layer can result in a delayed thawing subsidence period, comparing with the Arctic permafrost region (Daout et al., 2017). In addition, prior knowledge of ground ice content is important to interpret the long-term trend of surface deformation, but such information is still lacking at regional scale in the Tibetan Plateau.
Current satellite sensors still cannot provide measurements with sufficient spatial and temporal resolution to meet requirements for regional soil freeze/thaw and permafrost monitoring in the Tibetan Plateau. Satellite passive microwave remote sensing measurements are available at 10–50 km resolutions, which may not accurately represent the surface and subsurface conditions for each resolution element, especially in the heterogeneous mountain regions (Li et al., 2014). In addition, ambiguity often exists in the remote sensing retrievals using a single frequency due to its sensitivity to multiple land surface properties. More comprehensive evaluations on the accuracy of current satellite measurements or retrievals should be conducted through integrating point-scale in-situ measurements, multi-resolution satellite observations and forward radiative transfer model simulations (Dai et al., 2017). Moreover, it is highly desirable to understand the scaling effects of freeze/thaw state and bridge the multi-sensor retrievals especially for landscape freeze/thaw monitoring over complex terrain and diverse land cover types (Li et al., 2014; Du et al., 2015). On the other hand, the Tibetan Plateau is characterized by large diurnal temperature range and rapid freeze/thaw changes, with long freeze/thaw transition periods (up to a few months) (Jin and Li, 2009). Therefore, remote sensing data with finer temporal resolution are needed for better monitoring the diurnal freeze/thaw cycles in the Tibetan Plateau permafrost area.
Process-Based Modeling of Tibetan Plateau Permafrost
As mentioned above, current satellite remote sensing is largely restricted to the measurements of near-surface soils and their properties, while subsurface properties are more relevant to permafrost conditions. Many studies have combined satellite remote sensing data with empirical or statistical models to estimate the presence of permafrost as discussed above. However, these models usually oversimplify or overlook key processes controlling soil freeze/thaw and permafrost dynamics (Zheng et al., 2019b). Additionally, simple models also generally assume that the frozen subsurface is in equilibrium with the current climate, which may not capture the transient changes in frozen ground (Zhao et al., 2017a). Process-based models can better represent the underlying processes with the potential to provide more reliable estimates of regional permafrost distribution and changes.
Current Progress
Frozen soil parameterizations in early process models was implemented using analytic or approximate solution of Stefan problem due to computational limitations (Li and Koike, 2003). With increased computational power, numerical models with high precision have been widely used in Tibetan Plateau. Most numerical models simulate the soil temperature in one dimension by employing a finite-difference or finite-element form of heat transfer equation, while many of them also consider other processes such as soil water migration, and link soil freeze/thaw process with hydrological process (Riseborough et al., 2008). A range of models have been used to model Tibetan Plateau permafrost distribution and changes, including soil process model GIPL2.0, land surface model CoupModel, CLM, and some hydrological models incorporating soil freeze/thaw scheme into water transfer process like VIC and GBEHM (Geomorphology-Based Eco-Hydrological Model) (Guo and Wang, 2013; Lan et al., 2015; Qin et al., 2017; Zheng et al., 2019b).
Earlier permafrost models like GIPL 2.0 (Qin et al., 2017) do not simulate surface energy balance, and usually directly set land/ground surface temperature as the upper boundary condition for soil heat transfer. These models are able to simulate soil temperature for deep layers which could be used to identify the presence of permafrost, while other processes like surface energy balance, snow melting are generally not included (Qin et al., 2017; Sun et al., 2019). These models have relatively lower requirements on climatic forcing data and usually use land/ground surface temperature and external soil moisture data as inputs. Despite the simplified heat-water transfer processes, it can reduce the uncertainties that may be introduced by external inputs such as highly uncertain precipitation data in the Tibetan Plateau. Nevertheless, current soil moisture products available from global satellite remote sensing and land model data assimilation system also show large uncertainties in the Tibetan Plateau region (Yang et al., 2013a; Zhao et al., 2014; Chen et al., 2017). In addition, although using ground surface temperature as upper boundary conditions can reduce the need of calculating surface energy balance budget, those data may not be widely available at the regional scale. The remotely sensed land surface temperature can be used as a substitute, but the difference between ground surface temperature and land surface temperature can be large due to the buffering effects of surface vegetation or snow cover (Luo et al., 2018; Luo et al, 2020).
A number of regional and global land hydrological models are also adapted for permafrost simulation in the Tibetan Plateau region (Table 1). Some hydrological models link the soil freeze/thaw scheme with hydrological-related process. For example, GBEHM model is developed from a hillslope-based hydrology model (GBHM) (Yang et al., 2015; Zheng et al., 2019b). Land models describe the complex land system, and therefore often include many processes related to soil freeze/thaw, such as surface energy balance processes, snow melting and infiltration process, soil water migration, and variability in soil thermal properties due to soil organic content changes etc. In addition to traditional numerical solution used in most models, there are also some variations in the model concepts and solution in recent models. For example, there is a new solution of 1-D heat transfer based on enthalpy in a land surface model HydroSiB2, showing good performance in soil freeze/thaw modeling in the Tibetan Plateau (Bao et al., 2016; Wang et al., 2017). These models have been applied to either site scale or regional scale in the Tibetan Plateau, with differences in the forcing data and representations of essential physical processes.
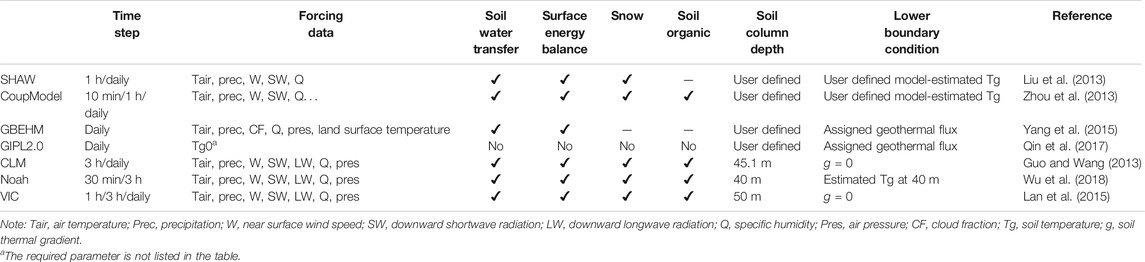
TABLE 1. Comparisons of models used for Tibetan Plateau permafrost simulation. All models include soil heat transfer process, which is not included here.
The parametrizations or representations of hydrological and thermal processes are different among different land hydrological models, such as the soil column depth and lower boundary conditions (Table 1), resulting in varying model performance. For example, SHAW model assumes that the soil thermal conductivity is a weighting average of various components in the soil, while CoupModel calculate the soil thermal conductivity based on three soil freeze/thaw state (i.e., unfrozen, frozen and partially frozen). Yang et al. (2013b) compared the simulation results from CoupModel and SHAW model at northern Tibetan Plateau sites, and found that soil heat transfer scheme used in CoupModel showed a better performance than SHAW model. Usually, these models require meteorological forcing including air temperature, wind speed, precipitation and radiation to solve the surface energy and water balance. It is generally difficult to directly use satellite data (such as land surface temperature) to drive these land models, which imposes additional difficulties for high-resolution (∼≤1 km) simulations as high-resolution surface meteorology datasets are not readily available in the Tibetan Plateau. Moreover, most land surface models are originally developed for surficial soil layers with a focus on the energy and mass exchange between the surface and the atmosphere, and therefore generally have simplified representation of the soil properties and thermal processes in deep soil layers (Sun et al., 2019).
Challenges
Large uncertainties exist in the reanalysis data, surface meteorology, surface and soil datasets in the Tibetan Plateau region, which are required for model inputs and parameterization, limiting a comprehensive evaluation of model processes and structure across the region (Dai et al., 2019). Ground-based meteorological data are particularly sparse in the Tibetan Plateau, with current stations mainly located in the central plateau and along the Qinghai-Tibet Railway/Highways, and complex topography adds additional challenges to upscaling local meteorological data to regional scale. For example, Zheng et al. (2019b) showed that the uncertainties associated with the extrapolation or interpolation of meteorological data were comparable to the uncertainties of model parameterizations. Previous studies also found that similar uncertainties were associated with model simulated frozen ground using different atmospheric forcing data comparing with model simulations using different parameterizations (Wang et al., 2016a; Guo et al., 2017). On the other hand, meteorological forcing data generated from global climate models has a coarse spatial resolution and cannot represent the local or small-scale climate variability (Su et al., 2013; Westermann et al., 2015). The unique topography and strong heterogeneous surface conditions in the Tibetan Plateau make it particular challenging to downscale coarse-resolution meteorology datasets (Yue et al., 2016). A recently released China meteorological forcing data incorporating meteorological stations, satellite data and re-analysis data has somewhat improved quality in the Tibetan Plateau and has been widely used; however, its uncertainty remains higher in the Tibetan Plateau than other regions of China (He et al., 2020). In addition, regional-scale soil datasets in the Tibetan Plateau, including soil properties (such as soil texture and soil organic carbon content), soil depth and ground ice content are extremely limited, which are important for model parameterization due to their significant impacts on soil heat and water transfer (Chen et al., 2015b; Cao et al., 2019).
Process representation in current models also needs improvement. A large gradient can exist in the surface air-ground temperature in the Tibetan Plateau region due to arid climate and high solar radiation (Wang and French, 1995). Without accounting for this temperature gradient, large biases can exist in the model simulated soil temperatures. Soil properties in global land models are generally derived from agriculture soil samples, while the physical properties of gravel soils in Tibetan Plateau are less considered (Yi et al., 2018a). Although most models have coupled soil heat transfer with soil water movement, the soil moisture profile is not generally well represented, partly due the complex interactions between topography, soil organic carbon content, vegetation distribution and their coupled effects on the dynamics of soil moisture (Wang et al., 2012a). Some key factors affecting the soil heat transfer unique to the mountain areas are also not adequately represented in current models (Zhou et al., 2013). For example, lateral water flow is generally ignored in most models, which may be of particular importance in the mountain areas (Zheng et al., 2019b). Simulating ground ice content in the Tibetan Plateau permafrost is also a challenge. Accurate representation of ground ice content especially near the permafrost table will have a large impact on projecting future permafrost dynamics due to potentially large latent heat release and thermokarst activity as a result of thawing ground ice (Chen et al., 2015b; Liljedahl et al., 2016). On the other hand, model performance is highly affected by boundary and initial conditions, which vary greatly among models. Zero heat flux at lower boundary is widely adopted in most land models, while the geothermal gradient in the Tibetan Plateau show high spatial variability (Wu et al., 2010). Assigning a zero or constant geothermal flux might lead to large biases in model simulated deep soil temperature and permafrost depth especially at longer time scale (Xiao et al., 2013). More data on deep-soil layer like borehole observations are needed to improve deep soil parameterization and representation of lower boundary conditions in the permafrost models.
Integrate Remote Sensing Data With Process-Based Models to Improve Tibetan Plateau Permafrost Monitoring
Remote sensing can provide spatially continuous landscape and climatic forcing data across large scale, which is especially useful for regional modeling in a heterogeneous terrain like the Tibetan Plateau. Here we summarize recent efforts that use multi-source remote sensing datasets to improve regional modeling of Tibetan Plateau permafrost or closely related environmental parameters, through model initialization, parameterization and data assimilation.
Climatic factors (such as air temperature and precipitation) in the Tibetan Plateau area show evident elevation dependence. In many process-based simulations, the SRTM (Shuttle Radar Topographic Mission) DEM (Digital Elevation Model) is used as a basis to extrapolate the in-situ meteorology observations to regional scale and generate model forcing data (e.g., Guo and Wang, 2013; Gao et al., 2018). However, in addition to elevation, other factors such as vegetation, soil wetness, slope, and aspect, also play important roles on the regional climate variability, and large uncertainties may exist in the spatial extrapolation using sparse ground observations (Wang et al., 2019b; Zheng et al., 2019b). Previous studies found that MODIS land surface temperature is able to capture the spatial pattern of near-surface air temperature and is especially useful in reducing the spatial interpolation errors in data-scarce regions (Zhu et al., 2013; Shamir and Georgakakos, 2014). In the northeast Tibetan Plateau, Wang et al. (2016b) used the lapse rate derived from MODIS land surface temperature product during the spatial interpolation of air temperature, and found the model simulated snow processes was improved comparing with simulations using the interpolated air temperature data. Regarding precipitation, previous studies found that the precipitation-elevation relationship contrasts between the northern and southern Tibetan Plateau, and the satellite-based precipitation data can approximately capture such patterns (Tang et al., 2018; Wang et al., 2019b). Nevertheless, very few studies have investigated the impacts of such satellite-based improvements in spatial extrapolation of climatic forcing on frozen soil simulations.
In addition to producing regional climatic forcing data, satellite remote sensing data has been also directly used as model parameters, such as vegetation coverage, slope and aspect, to improve regional simulations of Tibetan Plateau frozen ground. For example, based on the SRTM DEM, Zhang et al. (2018) quantified the topographic effects of solar radiation on permafrost distribution in the northeast Tibetan Plateau and the simulated permafrost coverage increased by 8% when accounting for the impact of topographic shadows on surface energy budget. Li et al. (2019) found that introducing MODIS-based vegetation coverage data into the CoLM can reduce the warming biases in land surface temperature and surface soil temperature by ∼10°C in sparse vegetated areas in the Tibetan Plateau. Jin and Li (2009) assimilated the satellite microwave-based brightness temperature into SHAW model at the Amdo station in the central Tibetan Plateau, and found that the errors of simulated upper (0–2.58 m) soil temperature during wintertime reduced by 0.76°C. Through assimilating in-situ soil moisture data, Zhou et al. (2008) found the accuracy of frozen depth simulation has improved.
A process-based model fully driven by satellite observations would bridge the data gap in data-scarce region such as the Tibetan Plateau with the potential to improve regional permafrost simulation. As discussed above, most process-based models heavily rely on surface meteorological forcing data (Zheng et al., 2019b; Guo et al., 2017), while satellite observations are not fully consistent with the requirements of these models. For example, satellite remote sensing is not able to observe the near-surface wind speed, which, however, is an essential factor in solving the surface energy balance. In a recent study, Zheng et al. (2019b) used a maximum entropy production-based parameterization of surface energy balance to replace the originally turbulent theory-based scheme in GBEHM to make it fully driven by satellite data and capable of capturing the water-heat coupled processes within soil layers. The model was applied to simulate the frozen soil distribution and recent changes across the entire Tibetan Plateau (Zheng et al., 2020). The satellite-based model simulations showed overall higher accuracy, comparing with previous Tibetan Plateau permafrost maps generated using ground-based measurements (Figure 3, adapted from Zheng et al., 2020). In addition to the physics-based scheme, machine learning and artificial intelligence have also obtained reliable performance in solving the surface energy budget (Adnan et al., 2017; Zhao et al., 2019) as well as in regional permafrost mapping (Pastick et al., 2015; Aalto et al., 2018). A hybrid approach that combines machine learning-based surface energy balance and process-based heat-water coupled processes seems to be a potentially useful tool for regional mapping of Tibetan Plateau permafrost, while such studies are still lacking.
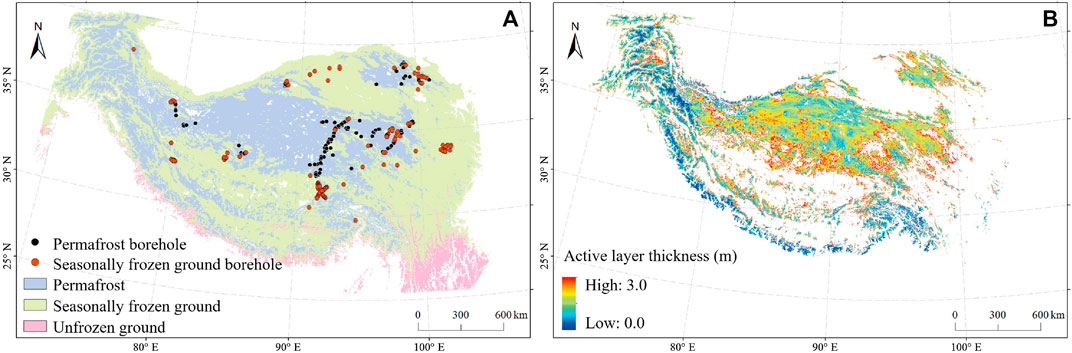
FIGURE 3. Mean spatial patterns of permafrost distribution (A) and active layer thickness (B) over the Tibetan Plateau during the period of 2002–2016, simulated using a remote sensing driven hydrology model (Zheng et al., 2019b; Zheng et al., 2020). The satellite-based permafrost map indicates that permafrost and seasonally frozen ground account for ∼37% and ∼56% of the Tibetan Plateau respectively, with an overall accuracy of 79.3–90.7% in identifying permafrost boreholes and 76.5–86.4% in identifying seasonally frozen ground boreholes. The accuracy is generally higher than permafrost maps generated using ground-stations, which demonstrates the advantage of satellite remote sensing-based methods in simulating frozen soils in data-scarce regions.
Although currently there are very limited studies using satellite data to directly improve model performance in simulating Tibetan Plateau permafrost through data assimilation, many efforts have been devoted to improving the simulation of variables closely related to the permafrost thermal state, such as soil moisture (Zhao et al., 2014; Yang et al., 2020), snow cover and snow depth (Zhang et al., 2014; Wang et al., 2020), and other key environmental variables including air temperature and net radiation (Li et al., 2012b; Lin et al., 2016). Through assimilating the satellite-based microwave brightness temperature at 6.9 and 18.7 GHz, Yang et al. (2007a) found the accuracy of simulated surface energy budget was improved. Lin et al. (2016) found that assimilating MODIS snow cover would be especially important in reducing uncertainties in air temperature predictions in the Tibetan Plateau. Those results could potentially improve the model simulated soil thermal regime and thus permafrost distribution. Despite its potential advantages in improving frozen soil simulation, the application of data assimilation is greatly limited due to large uncertainties in current satellite products and retrieval algorithms in the Tibetan Plateau (Chen et al., 2017). In addition, satellite-based soil moisture and snow cover retrievals from passive microwave remote sensing data have generally coarse spatial resolution, and appropriate downscaling scheme is needed to characterize the spatial heterogeneities within the coarse-grid in the mountain areas (Zhao et al., 2014).
Discussion
Unique Challenges in Tibetan Plateau Permafrost Monitoring
Unique environmental conditions in the Tibetan Plateau result in permafrost characteristics different from that in the northern high latitudes or other alpine environments as discussed above. The topography in the Tibetan Plateau is complex and characterized by strong heterogeneity at small spatial scales, exerting strong control on local permafrost distribution (Luo et al., 2012), while most satellite data or models have relatively coarse resolutions (>1 km) and cannot well represent such local-scale variability (Yang et al., 2010). Moreover, the complex topography in the Tibetan Plateau can result in strong spatial heterogeneity in local-scale snow cover and land surface temperature conditions, which adds additional uncertainties to satellite-based freeze/thaw detection (Zhao et al., 2017b; Dai et al., 2018). For example, snow accumulated in shady areas can result in strong scattering, impacting the accuracy of coarse-resolution passive microwave remote sensing retrievals (Dai et al., 2017). Accurately accounting for the impact of topography on Tibetan Plateau soil freeze/thaw dynamics and permafrost distribution requires improved remote sensing algorithms and models.
Other surface or subsurface conditions affecting the Tibetan Plateau permafrost are also different from those in the high-latitude regions. The Arctic is mostly underlain by continuous permafrost (Brown et al., 1997), characterized by overall high soil carbon content, thicker snow cover and more saturated soil condition (Hinzman et al., 2013). Previous study showed the uncertainty in spatial and vertical soil organic distribution is the main factor affecting the model simulated active layer thickness in Alaska, followed by the impacts of soil moisture (Yi et al., 2018b). The timing and amount of snow cover also play an important role in affecting pan-Arctic soil temperatures, especially in deeper soils, due to its strong insulation effects (Yi et al., 2015; Jones et al., 2016). However, the frozen ground in the Tibetan Plateau is generally characterized by unsaturated soils, low organic carbon content, shallow snow cover, and shorter snow duration (Li et al., 2008; Kang et al., 2010). Large uncertainties exist in Tibetan Plateau remote sensing and model soil moisture products due to strong spatial and vertical variability in soil moisture. The uncertainties limit their usefulness in regional permafrost monitoring (Han et al., 2015; Bi et al., 2016). For example, most InSAR-based active layer retrieval algorithms assume a saturated soil profile within the active layer, while Tibetan Plateau permafrost generally shows a much deeper and drier active layer compared with that in the Arctic region (Jia et al., 2017). Other Tibetan Plateau environmental factors also need to be carefully considered in model parameterization. For example, the insulation effects of soil organic carbon in the Tibetan Plateau is likely limited due to its lower content; however, soil carbon content can significantly affect Tibetan Plateau soil freeze/thaw dynamics through altering the soil hydraulic properties and soil moisture distribution (Zhou et al., 2013; Jiang et al., 2020). Shallow snow cover may have a cooling effect on subsurface soil temperature through reducing the absorption of solar radiation (Wang et al., 2020). In addition, the coarse-fragment soil with a larger thermal conductivity is widespread in the Tibetan Plateau, unlike the prevailing peat soils in the Arctic. This should be considered in the model parameterization when simulating the Tibetan Plateau permafrost (Arocena et al., 2012; Pan et al., 2017; Yi et al., 2018a).
Potential of Geophysical Measurements in Regional Permafrost Studies
Current satellite remote sensing cannot directly detect deep soils and the permafrost, while geophysical techniques have been proven to be useful for subsurface soil characterization and permafrost detection in a minimally invasive manner. Examples of geophysical approaches include thermistors and dielectric sensors, ground penetrating radar, and electromagnetic methods (Dafflon et al., 2016). Among these techniques, electromagnetic methods are becoming increasingly popular, which calculates the ground conductivity or resistivity through measuring the changes in the eddy currents and induced magnetic fields in the subsurface (Hauck et al., 2001). Based on the distinct difference of resistivity between frozen and unfrozen materials, the electromagnetic method can detect permafrost existence with a relatively high spatial resolution. It has the advantage of being sensitive to the liquid soil water content, and providing information on the structure and content of the deep soil layer, which is lacking but usually required by models (Minsley et al., 2012; Mikucki et al., 2015). In addition to the surface geophysical measurements, airborne electromagnetic methods have been widely used in the polar regions to detect the permafrost over diverse landscapes at the regional scale (Minsley et al., 2012; Rey et al., 2019). Airborne electromagnetic methods have less terrain-induced noise than ground measurements, and may be particular useful for permafrost mapping in mountain regions (Hauck et al., 2001; Su et al., 2020), while such applications are still lacking in the Tibetan region.
The geophysical measurements in combination with other datasets have shown great potential to improve model representation of subsurface properties in permafrost region. For example, Dafflon et al. (2016) combined electromagnetic method and other types of observations, and successfully mapped subsurface variability in polygon shaped Arctic tundra area and document the key controls on the spatial distribution of soil properties. Léger et al. (2019) used the soil temperature data from a spatially distributed temperature profiling system and electrical resistivity data to identify correspondences between surface and subsurface property distribution in discontinuous permafrost regions. The results from these studies provide the basis for model parameterization and initialization, while other studies have directly used such observations to improve process representation in the permafrost models (Tran et al., 2017, Tran et al., 2018). Due to strong influences of organic carbon content on soil hydrological-thermal parameters, Tran et al. (2017) performed joint inversion of multiple datasets including soil temperature, moisture and electrical resistivity data to estimate the soil organic carbon profile along a transect in Barrow, Alaska, through linking the petrophysical and geophysical models with the CLM model. Combining the CLM model and electrical resistivity data, a follow-on study further investigated the soil thaw depth and its controlling factor at a high spatiotemporal resolution over a long period (Tran et al., 2018). However, it should be noted that additional errors may be introduced into the model estimates due to uncertainties in electrical resistivity inversion method and retrievals.
Improving Model Representation of Spatial Heterogeneity
An important feature of permafrost affected landscapes is the large spatial heterogeneity in soil active layer conditions, which are generally poorly represented in global land models (Muster et al., 2012; Mishra et al., 2017). Information on active layer soil conditions at landscape scales is needed to better represent the spatial heterogeneity and scaling functions in global models and reconcile coarser model simulations with more extreme local heterogeneity in active layer and permafrost conditions. Many studies have combined in-situ measurements, satellite remote sensing and models to address this problem. However, most studies focus on the high-latitude permafrost landscapes while Tibetan Plateau permafrost studies represent a relatively new research topic. Therefore, we summarize relevant studies in the high-latitudes, and hope to provide some insights for similar applications to the Tibetan Plateau region.
Local to landscape scale variations in active layer conditions can be effectively monitored using in-situ ground measurements (Brown et al., 2000; Romanovsky et al., 2010) or ground-based remote sensing including ground penetrating radar and electrical resistivity measurements (Hubbard et al., 2013; Schaefer et al., 2015). While these methods provide detailed assessments of active layer properties, they are generally applied over limited local areas (<1 km2) and are unsuitable for mapping and monitoring over large regions. Regionally refined active layer products upscaled from carefully designed in-situ network measurements using remote sensing based geospatial data layers offer the potential to improve the representation of permafrost active layer properties in global land models. Earlier studies used empirical models driven by in-situ ground observations to map permafrost and active layer properties over larger areas (e.g., Anisimov et al., 2002; Panda et al., 2010). More recent studies used machine learning data-fusion approaches and remote sensing data, including optical-infrared and LIDAR data, to extend ground-based or airborne measurements to produce regional active layer and permafrost maps (Hubbard et al., 2013; Pastick et al., 2015). Similar as active layer thickness, a variety of methods including geospatial analysis and machine-learning data-driven approaches have been applied to upscale in-situ soil organic carbon content and soil moisture measurements to provide grid-cell mean estimates at a large footprint for direct comparison with satellite or model-based products (e.g., Mishra and Riley, 2015; Clewley et al., 2017).
The above spatial analysis and datasets provide critically needed information on permafrost distribution for global model parameterization and validation (Mishra et al., 2017). Previous studies have demonstrated the importance of considering sub-grid scale variability in land cover and surface properties in large-scale global models to accurately simulate water, energy and carbon exchange in permafrost landscapes (Liston, 2004; Gouttevin et al., 2012). However, how to represent those local effects to facilitate upscaling of model simulated water, energy and carbon fluxes remains a challenge (Muster et al., 2012). Potential improvements may include statistical representation of spatial distribution and temporal changes in the surface variables related to permafrost properties. For example, Zhang et al. (2014) used statistical functions derived from field measurements to characterize the spatial variability of surface ground and soil conditions and incorporated this information into a process model to simulate the frequency of permafrost occurrence at very high spatial (<1 km) resolution. Much better accuracy in model simulated ground temperatures in Norway was achieved when using a gamma distribution to represent sub-grid variability in snow distribution than using grid-cell average snow depth (Gisnås et al., 2016). Yi et al. (2018b) also found the model better represented the statistical distribution of active layer thickness when using a logistic distribution consistent with the spatial distribution of surface wetness derived from the P-band radar data to represent sub-grid variability of organic layer thickness. In addition, the zonation approach can combine diverse datasets over different scales and identify the large-scale zones that have distinct properties, which can be used to characterize the spatial heterogeneity in complex permafrost environments. For example, Wainwright et al. (2015) employed a nested polygon geomorphic zonation approach to characterize the distributions of environmental properties in Arctic tundra and assess their impact on ecosystem carbon fluxes. The combination of zonation approach and emerging technologies such as machine learning approach can generate spatially-explicit datasets from a diverse range of observations supporting the model needs of parameterization and initialization, and may have great potential in permafrost research (Hubbard et al., 2018; Hubbard et al., 2020).
Research Priorities and Recommendations
We have reviewed the state of knowledge for Tibetan Plateau permafrost, soil freeze/thaw state, and summarized recent progress in using satellite remote sensing data and models to monitor Tibetan Plateau permafrost. This exercise has revealed important gaps in capabilities for monitoring and modeling the current state of Tibetan Plateau permafrost as well as projecting its future trajectory. Here we recommend the following research priorities to address these gaps.
Satellite-based optical and infrared remote sensing provide high spatial resolution land surface conditions. These techniques have great potential for producing Tibetan Plateau classification maps and determining correlations between these classifications and permafrost distributions, especially in regional-scale applications. Particularly, high-resolution imagery (<10 m scale) can be used to capture the high degree of spatial heterogeneity in the Tibetan Plateau and further infer the related permafrost states, such as using high-resolution imaging of swamps, wetlands and small watersheds to monitor changes in the hydrologic state that may be coupled with local permafrost degradation.
Microwave remote sensing has shown great potential in surface freeze/thaw and permafrost monitoring due to its strong sensitivity to soil water content and phase changes, its ability to penetrate clouds, and providing all-weather monitoring of the land surface. However, current microwave remote sensing methods are limited to detections in the upper soil layer within a few centimeters of the surface. Deeper soil remote sensing requires lower frequency surveys (such as P-band SAR) and multi-frequency observations (such as joint L- and P-band SAR) to enable soil vertical profile retrievals. These sensors and corresponding algorithms should be developed. InSAR have been widely used to map regional subsidence and active layer thickness in the Tibetan Plateau, but more systematic subsidence mapping is still needed, with particular emphasis on the permafrost transition zone that is vulnerable to abrupt thaw and degradation. Improved approaches and regional data such as accurate DEM and soil moisture information are also needed to support InSAR active layer retrieval.
The apparent scarcity of local-scale observations greatly limits our process understanding in the Tibetan Plateau permafrost area. Therefore, we also call for the expansion of the in-situ sampling network, particularly in the western Tibetan Plateau, along the transition zones between discontinuous and sporadic permafrost zones, and along elevation gradients (Figure 1). Additional information on deep soil temperature, ground ice content, more consistent soil moisture and dielectric profiles, improved mapping of soil texture and soil organic content are required for improving remote sensing algorithms and regional models. Geophysical measurements especially airborne electromagnetic method can obtain information on deep ground structure and unfrozen water content, and provide more spatially extensive measurements than point-scale observations, and should be considered when expanding the local-scale observational network in the Tibetan Plateau.
Integrating local-scale observations, remote sensing data with process-based models will be an effective way to monitor regional permafrost and project its future state. However, a number of factors limit such applications in Tibetan Plateau permafrost research, including but not limiting to large uncertainties in current satellite products for the Tibetan Plateau area, mismatch between model input data needs and information provided by current satellite sensors, insufficient accounting for spatial heterogeneity impact on permafrost distribution. Better approaches to combine diverse datasets at different spatial and temporal scales with models are needed to address the above challenges, and should be developed. In addition, current model scheme and parameterization also require improvements to better reproduce the unique soil freeze/thaw characteristics in the Tibetan Plateau region.
Author Contributions
YY initiated the study. HJ, GZ, and YY wrote the paper. Other co-authors contributed to the discussion and provided feedback.
Funding
YY acknowledges support by the NASA Terrestrial Ecology program under the Arctic Boreal Vulnerability Experiment (ABoVE, NNH18ZDA001N-TE). It was also supported by the Strategic Priority Research Program of Chinese Academy of Sciences (Grant No. XDA20060401), National Natural Science Foundation of China (41771112), and the Swedish Research Council (VR 2014–5320). A portion of this research was carried out at the Jet Propulsion Laboratory, California Institute of Technology, under contract with NASA. ©2020. All rights reserved.
Conflict of Interest
The authors declare that the research was conducted in the absence of any commercial or financial relationships that could be construed as a potential conflict of interest.
References
Aalto, J., Karjalainen, O., Hjort, J., and Luoto, M. (2018). Statistical forecasting of current and future circum-Arctic ground temperatures and active layer thickness. Geophys. Res. Lett. 45, 4889–4898. doi:10.1029/2018gl078007
Adnan, M., Ahsan, M., Rehman, A., and Nazir, M. (2017). Estimating evapotranspiration using machine learning techniques. Int. J. Adv. Comput. Sci. Appl. 8, 108–113. doi:10.14569/ijacsa.2017.080915
Anisimov, O. A., Shiklomanov, N. I., and Nelson, F. E. (2002). Variability of seasonal thaw depth in permafrost regions: a stochastic modeling approach. Ecol. Model. 153, 217–227. doi:10.1016/s0304-3800(02)00016-9
Arocena, J., Hall, K., and Zhu, L. P. S. J. O. S. S. (2012). Soil formation in high elevation and permafrost areas in the Qinghai plateau (China). Spanish J. Soil Sci. 2, 34–49. doi:10.3232/SJSS.2012.V2.N2.02
Bao, H., Koike, T., Yang, K., Wang, L., Shrestha, M., and Lawford, P. (2016). Development of an enthalpy-based frozen soil model and its validation in a cold region in China. J. Geophys. Res. Atmos. 121, 5259–5280. doi:10.1002/2015jd024451
Bi, H., Ma, J., Zheng, W., and Zeng, J. (2016). Comparison of soil moisture in GLDAS model simulations and in situ observations over the Tibetan Plateau. J. Geophys. Res. Atmos. 121, 2658–2678. doi:10.1002/2015jd024131
Bibi, S., Wang, L., Li, X., Zhou, J., Chen, D., and Yao, T. (2018). Climatic and associated cryospheric, biospheric, and hydrological changes on the Tibetan Plateau: a review. Int. J. Climatol. 38, e1–e17. doi:10.1002/joc.5411
Brown, J., Ferrians, O. J., Heginbottom, J. A., and Melnikov, E. S. (1997). Circum arctic map of permafrost and ground ice conditions. Middleton, WI: Center for Integrated Data Analytics Wisconsin Science Center.
Brown, J., Hinkel, K. M., and Nelson, F. E. (2000). The circumpolar active layer monitoring (calm) program: research designs and initial results. Polar Geogr. 24, 166–258. doi:10.1080/10889370009377698
Cao, B., Zhang, T., Wu, Q., Sheng, Y., Zhao, L., and Zou, D. (2019). Brief communication: evaluation and inter-comparisons of Qinghai-Tibet Plateau permafrost maps based on a new inventory of field evidence. Cryosphere 13, 511–519. doi:10.5194/tc-13-511-2019
Chen, D., Xu, B., Yao, T., Guo, Z., Cui, P., Chen, F., et al. (2015a). Assessment of past, present and future environmental changes on the Tibetan Plateau. Chin. Sci. Bull. 60, 3025–3035. doi:10.1360/n972015-00849
Chen, H., Nan, Z., Zhao, L., Ding, Y., Chen, J., and Pang, Q. (2015b). Noah modelling of the permafrost distribution and characteristics in the west Kunlun area, qinghai-tibet plateau, China. Permafr. Periglac. Process. 26, 160–174. doi:10.1002/ppp.1841
Chen, R. H., Tabatabaeenejad, A., and Moghaddam, M. (2019). Retrieval of permafrost active layer properties using time-series P-band radar observations. IEEE Trans. Geosci. Rem. Sens. 57, 6037–6054. doi:10.1109/tgrs.2019.2903935
Chen, Y., Yang, K., Qin, J., Cui, Q., Lu, H., La, Z., et al. (2017). Evaluation of SMAP, SMOS, and AMSR2 soil moisture retrievals against observations from two networks on the Tibetan Plateau. J. Geophys. Res. Atmos. 122, 5780–5792. doi:10.1002/2016jd026388
Chen, Y., Yang, K., Tang, W., Qin, J., and Zhao, L. (2012). Parameterizing soil organic carbon’s impacts on soil porosity and thermal parameters for Eastern Tibet grasslands. Sci. China Earth Sci. 55, 1001–1011. doi:10.1007/s11430-012-4433-0
Cheng, G.,, and Wu, T. (2007). Responses of permafrost to climate change and their environmental significance, Qinghai-Tibet Plateau. J. Geophys. Res. 112. doi:10.1029/2006jf000631
Cheng, G., Zhao, L., Li, R., Wu, X., Sheng, Y., Hu, G., et al. (2019). Characteristic, changes and impacts of permafrost on Qinghai-Tibet Plateau. Chin. Sci. Bull. 64, 2783–2795. (in Chinese). doi:10.136./tb-2019-0191
Clewley, D., Whitcomb, J. B., Akbar, R., Silva, A. R., Berg, A., Adams, J. R., et al. (2017). A method for upscaling in situ soil moisture measurements to satellite footprint scale using random forests. IEEE J. Sel. Top. Appl. Earth Observations Remote Sensing 10, 2663–2673. doi:10.1109/jstars.2017.2690220
Dafflon, B., Hubbard, S., Ulrich, C., Peterson, J., Wu, Y., Wainwright, H., et al. (2016). Geophysical estimation of shallow permafrost distribution and properties in an ice-wedge polygon-dominated Arctic tundra region. Geophysics 81, WA247–WA263. doi:10.1190/geo2015-0175.1
Dai, L., Che, T., Ding, Y., and Hao, X. (2017). Evaluation of snow cover and snow depth on the Qinghai-Tibetan Plateau derived from passive microwave remote sensing. Cryosphere 11, 1933–1948. doi:10.5194/tc-11-1933-2017
Dai, L., Che, T., Xie, H., and Wu, X. (2018). Estimation of snow depth over the Qinghai-Tibetan plateau based on AMSR-E and MODIS data. Rem. Sens. 10. doi:10.3390/rs10121989
Dai, Y., Xin, Q., Wei, N., Zhang, Y., Shangguan, W., Yuan, H., et al. (2019). A global high‐resolution data set of soil hydraulic and thermal properties for land surface modeling. J. Adv. Model. Earth Syst. 11, 2996–3023. doi:10.1029/2019ms001784
Daout, S., Doin, M. P., Peltzer, G., Socquet, A., and Lasserre, C. (2017). Large‐scale InSAR monitoring of permafrost freeze‐thaw cycles on the Tibetan Plateau. Geophys. Res. Lett. 44, 901–909. doi:10.1002/2016gl070781
Dente, L., Ferrazzoli, P., Su, Z., Van Der Velde, R., and Guerriero, L. (2014). Combined use of active and passive microwave satellite data to constrain a discrete scattering model. Rem. Sens. Environ. 155, 222–238. doi:10.1016/j.rse.2014.08.031
Drusch, D. M., Walvoord, M., Minsley, B., Rover, J., and Singha, K. (2019). Investigating lake-area dynamics across a permafrost-thaw spectrum using airborne electromagnetic surveys and remote sensing time-series data in Yukon Flats, Alaska. Environ. Res. Lett. 14, 025001. doi:10.1088/1748-9326/aaf06f
Du, J., Kimball, J., and Moghaddam, M. (2015). Theoretical modeling and analysis of L- and P-band radar backscatter sensitivity to soil active layer dielectric variations. Rem. Sens. 7, 9450–9472. doi:10.3390/rs70709450
Duan, A. M., and Wu, G. X. (2005). Role of the Tibetan Plateau thermal forcing in the summer climate patterns over subtropical Asia. Clim. Dynam. 24, 793–807. doi:10.1007/s00382-004-0488-8
Gao, B., Yang, D., Qin, Y., Wang, Y., Li, H., Zhang, Y., et al. (2018). Change in frozen soils and its effect on regional hydrology, upper Heihe basin, northeastern Qinghai-Tibetan Plateau. Cryosphere 12. doi:10.5194/tc-12-657-2018
Gisnås, K., Westermann, S., Schuler, T. V., Melvold, K., and Etzelmüller, B. (2016). Small-scale variation of snow in a regional permafrost model. Cryosphere 10, 1201–1215. doi:10.5194/tc-10-1201-2016
Gouttevin, I., Krinner, G., Ciais, P., Polcher, J., and Legout, C. (2012). Multi-scale validation of a new soil freezing scheme for a land-surface model with physically-based hydrology. Cryosphere 6, 407–430. doi:10.5194/tc-6-407-2012
Gruber, S., Fleiner, R., Guegan, E., Panday, P., Schmid, M.-O., Stumm, D., et al. (2017). Review article: inferring permafrost and permafrost thaw in the mountains of the Hindu Kush Himalaya region. Cryosphere 11, 81–99. doi:10.5194/tc-11-81-2017
Guo, D., Sun, J., Yang, K., Pepin, N., and Xu, Y. (2019). Revisiting recent elevation‐dependent warming on the Tibetan plateau using satellite‐based data sets. J. Geophys. Res. Atmos. 124, 8511–8521. doi:10.1029/2019jd030666
Guo, D.,, and Wang, H. (2013). Simulation of permafrost and seasonally frozen ground conditions on the Tibetan Plateau, 1981–2010. J. Geophys. Res. Atmos. 118, 5216–5230. doi:10.1002/jgrd.50457
Guo, D., Wang, H., and Wang, A. (2017). Sensitivity of historical simulation of the permafrost to different atmospheric forcing data sets from 1979 to 2009. J. Geophys. Res. Atmos. 122 (12), 269–212. doi:10.1002/2017jd027477
Han, L., Tsunekawa, A., and Tsubo, M. (2011). Radar remote sensing of springtime near-surface soil thaw events at mid-latitudes. Int. J. Rem. Sens. 32, 8555–8574. doi:10.1080/01431161.2010.542203
Han, M., Yang, K., Qin, J., Jin, R., Ma, Y., Wen, J., et al. (2015). An algorithm based on the standard deviation of passive microwave brightness temperatures for monitoring soil surface freeze/thaw state on the Tibetan plateau. IEEE Trans. Geosci. Rem. Sens. 53, 2775–2783. doi:10.1109/tgrs.2014.2364823
Hauck, C., Guglielmin, M., Isaksen, K., and Vonder Mühll, D. (2001). Applicability of frequency-domain and time-domain electromagnetic methods for mountain permafrost studies. Permafr. Periglac. Process. 12, 39–52. doi:10.1002/ppp.383
He, J., Yang, K., Tang, W., Lu, H., Qin, J., Chen, Y., et al. (2020). The first high-resolution meteorological forcing dataset for land process studies over China. Scientific Data 7, 1–11. doi:10.1038/s41597-020-0369-y
Hinzman, L. D., Deal, C. J., Mcguire, A. D., Mernild, S. H., Polyakov, I. V., and Walsh, J. E. (2013). Trajectory of the Arctic as an integrated system. Ecol. Appl. 23, 1837–1868. doi:10.1890/11-1498.1
Holmes, T. R. H., De Jeu, R. a. M., Owe, M., and Dolman, A. J. (2009). Land surface temperature from Ka band (37 GHz) passive microwave observations. J. Geophys. Res. Atmos. 114. doi:10.1029/2008jd010257
Huang, L., Luo, J., Lin, Z., Niu, F., and Liu, L. (2020). Using deep learning to map retrogressive thaw slumps in the Beiluhe region (Tibetan Plateau) from CubeSat images. Rem. Sens. Environ. 237, 111534. doi:10.1016/j.rse.2019.111534
Hubbard, S. S., Gangodagamage, C., Dafflon, B., Wainwright, H., Peterson, J., Gusmeroli, A., et al. (2013). Quantifying and relating land-surface and subsurface variability in permafrost environments using LiDAR and surface geophysical datasets. Hydrogeol. J. 21, 149–169. doi:10.1007/s10040-012-0939-y
Hubbard, S. S., Varadharajan, C., Wu, Y., Wainwright, H., and Dwivedi, D. (2020). Emerging technologies and radical collaboration to advance predictive understanding of watershed hydrobiogeochemistry. Hydrol. Process. 34, 3175–3182. doi:10.1002/hyp.13807
Hubbard, S. S., Williams, K. H., Agarwal, D., Banfield, J., Beller, H., Bouskill, N., et al. (2018). The east river, Colorado, watershed: a mountainous community testbed for improving predictive understanding of multiscale hydrological-biogeochemical dynamics. Vadose Zone J. 17, 180061. doi:10.2136/vzj2018.03.0061
Immerzeel, W. W., Van Beek, L. P. H., and Bierkens, M. F. P. (2010). Climate change will affect the Asian water towers. Science 328, 1382–1385. doi:10.1126/science.1183188
Jia, Y., Kim, J.-W., Shum, C., Lu, Z., Ding, X., Zhang, L., et al. (2017). Characterization of active layer thickening rate over the northern qinghai-Tibetan plateau permafrost region using ALOS interferometric synthetic aperture radar data, 2007–2009. Rem. Sens. 9, 84. doi:10.3390/rs9010084
Jiang, H., Yi, Y., Zhang, W., Yang, K., and Chen, D. (2020). Sensitivity of soil freeze/thaw dynamics to environmental conditions at different spatial scales in the central Tibetan Plateau. Sci. Total Environ. 734, 139261. doi:10.1016/j.scitotenv.2020.139261
Jiang, H., Zhang, W., Yi, Y., Yang, K., Li, G., and Wang, G. (2018). The impacts of soil freeze/thaw dynamics on soil water transfer and spring phenology in the Tibetan Plateau. Arctic Antarct. Alpine Res. 50, e1439155. doi:10.1080/15230430.2018.1439155
Jin, H.-j., Yu, Q.-h., Wang, S.-l., and Lü, L.-z. (2008). Changes in permafrost environments along the Qinghai-Tibet engineering corridor induced by anthropogenic activities and climate warming. Cold Reg. Sci. Technol. 53, 317–333. doi:10.1016/j.coldregions.2007.07.005
Jin, H., Luo, D., Wang, S., Lü, L., and Wu, J. (2011). Spatiotemporal variability of permafrost degradation on the Qinghai-Tibet Plateau. Sci. Cold Arid Regions 3, 281–305. doi:10.3724/sp.j.1226.2011.00281
Jin, R., Li, X., and Che, T. (2009). A decision tree algorithm for surface soil freeze/thaw classification over China using SSM/I brightness temperature. Rem. Sens. Environ. 113, 2651–2660. doi:10.1016/j.rse.2009.08.003
Jin, R., and Li, X. (2009). Improving the estimation of hydrothermal state variables in the active layer of frozen ground by assimilating in situ observations and SSM/I data. Sci. China Earth Sci. 52, 1732. doi:10.1007/s11430-009-0174-0
Jones, B. M., Baughman, C. A., Romanovsky, V. E., Parsekian, A. D., Babcock, E. L., Stephani, E., et al. (2016). Presence of rapidly degrading permafrost plateaus in south-central Alaska. Cryosphere 10, 2673–2692. doi:10.5194/tc-10-2673-2016
Jorgenson, M. T.,, and Grosse, G. (2016). Remote sensing of landscape change in permafrost regions. Permafr. Periglac. Process. 27, 324–338. doi:10.1002/ppp.1914
Kang, S., Xu, Y., You, Q., Flügel, W.-A., Pepin, N., and Yao, T. (2010). Review of climate and cryospheric change in the Tibetan Plateau. Environ. Res. Lett. 5, 015101. doi:10.1088/1748-9326/5/1/015101
Kou, X., Jiang, L., Yan, S., Zhao, T., Lu, H., and Cui, H. (2017). Detection of land surface freeze-thaw status on the Tibetan Plateau using passive microwave and thermal infrared remote sensing data. Rem. Sens. Environ. 199, 291–301. doi:10.1016/j.rse.2017.06.035
Lan, C., Zhang, Y., Bohn, T. J., Zhao, L., Li, J., Liu, Q., et al. (2015). Frozen soil degradation and its effects on surface hydrology in the northern Tibetan Plateau. J. Geophys. Res. Atmos. 120, 8276–8298. doi:10.1002/2015jd023193
Léger, E., Dafflon, B., Robert, Y., Ulrich, C., Peterson, J. E., Biraud, S. C., et al. (2019). A distributed temperature profiling method for assessing spatial variability in ground temperatures in a discontinuous permafrost region of Alaska. Cryosphere 13, 2853–2867. doi:10.5194/tc-13-2853-2019
Li, C., Lu, H., Leung, L. R., Yang, K., Li, H., Wang, W., et al. (2019). Improving land surface temperature simulation in CoLM over the Tibetan plateau through fractional vegetation cover derived from a remotely sensed clumping index and model‐simulated leaf area index. J. Geophys. Res. Atmos. 124, 2620–2642. doi:10.1029/2018jd028640
Li, X., Cheng, G., Jin, H., Kang, E., Che, T., Jin, R., et al. (2008). Cryospheric change in China. Global Planet. Change 62, 210–218. doi:10.1016/j.gloplacha.2008.02.001
Li, X., Jin, R., Pan, X., Zhang, T., and Guo, J. (2012a). Changes in the near-surface soil freeze-thaw cycle on the Qinghai-Tibetan Plateau. Int. J. Appl. Earth Obs. Geoinf. 17, 33–42. doi:10.1016/j.jag.2011.12.002
Li, X.,, and Koike, T. (2003). Frozen soil parameterization in SiB2 and its validation with GAME-Tibet observations. Cold Reg. Sci. Technol. 36, 165–182. doi:10.1016/s0165-232x(03)00009-0
Li, X., Zhang, L., Weihermüller, L., Jiang, L., and Vereecken, H. (2013). Measurement and simulation of topographic effects on passive microwave remote sensing over mountain areas: a case study from the Tibetan Plateau. IEEE Trans. Geosci. Remote Sens. 52, 1489–1501. doi:10.1109/tgrs.2013.2251887
Li, Y., Sun, R., and Liu, S. (2015a). Vegetation physiological parameter setting in the Simple Biosphere model 2 (SiB2) for alpine meadows in the upper reaches of Heihe river. Sci. China Earth Sci. 58, 755–769. doi:10.1007/s11430-014-4909-1
Li, Z., Tang, P., Zhou, J., Tian, B., Chen, Q., and Fu, S. (2014). Permafrost environment monitoring on the Qinghai-Tibet Plateau using time series ASAR images. Int J Digit Earth 8, 840–860. doi:10.1080/17538947.2014.923943
Li, Z., Zhao, L., and Fu, Z. (2012b). Estimating net radiation flux in the Tibetan Plateau by assimilating MODIS LST products with an ensemble Kalman filter and particle filter. Int. J. Appl. Earth Obs. Geoinf. 19, 1–11. doi:10.1016/j.jag.2012.04.003
Li, Z., Zhao, R., Hu, J., Wen, L., Feng, G., Zhang, Z., et al. (2015b). InSAR analysis of surface deformation over permafrost to estimate active layer thickness based on one-dimensional heat transfer model of soils. Sci. Rep. 5, 15542. doi:10.1038/srep15542
Liljedahl, A. K., Boike, J., Daanen, R. P., Fedorov, A. N., Frost, G. V., Grosse, G., et al. (2016). Pan-Arctic ice-wedge degradation in warming permafrost and its influence on tundra hydrology. Nat. Geosci. 9, 312. doi:10.1038/ngeo2674
Lin, P., Wei, J., Yang, Z. L., Zhang, Y., and Zhang, K. (2016). Snow data assimilation‐constrained land initialization improves seasonal temperature prediction. Geophys. Res. Lett. 43 (11), 423–411. doi:10.1002/2016gl070966
Liston, G. E. (2004). Representing subgrid snow cover heterogeneities in regional and global models. J. Clim. 17, 1381–1397. doi:10.1175/1520-0442(2004)017<1381:rsschi>2.0.co;2
Liu, L., Schaefer, K., Zhang, T., and Wahr, J. (2012). Estimating 1992–2000 average active layer thickness on the Alaskan North Slope from remotely sensed surface subsidence. J. Geophys. Res. 117. doi:10.1029/2011jf002041
Liu, Y., Zhao, L., and Li, R. (2013). Simulation of the soil water-thermal features within the active layerin Tanggula region, Tibetan plateau, by using SHAW model. J. Glaciol. Geocryol. 35, 280–290.
Luo, D., Jin, H., Lin, L., He, R.-X., Yang, S.-Z., and Chang, X.-L. (2012). New progress on permafrost temperature and thickness in the source area of the Huanghe River. Sci. Geogr. Sin. 32, 898–904. doi:10.13249/j.cnki.sgs.2012.07.898
Luo, D., Jin, H., Lü, L., and Wu, Q. (2014). Spatiotemporal characteristics of freezing and thawing of the active layer in the source areas of the Yellow River (SAYR). Chin. Sci. Bull. 59, 3034–3045. doi:10.1007/s11434-014-0189-6
Luo, D., Jin, H., Marchenko, S. S., and Romanovsky, V. E. (2018). Difference between near-surface air, land surface and ground surface temperatures and their influences on the frozen ground on the Qinghai-Tibet Plateau. Geoderma 312, 74–85. doi:10.1016/j.geoderma.2017.09.037
Luo, D., Liu, L., Jin, H., Wang, X., and Chen, F. (2020). Characteristics of ground surface temperature at chalaping in the source area of the Yellow River, northeastern Tibetan plateau. Agric. For. Meteorol. 281, 107819. doi:10.1016/j.agrformet.2019.107819
Ma, Y., Zhang, Y., Zubrzycki, S., Guo, Y., and Farhan, S. B. (2015). Hillslope-scale variability in seasonal frost depth and soil water content investigated by GPR on the southern margin of the sporadic permafrost zone on the Tibetan plateau. Permafr. Periglac. Process. 26, 321–334. doi:10.1002/ppp.1844
Mikucki, J. A., Auken, E., Tulaczyk, S., Virginia, R. A., Schamper, C., Sorensen, K. I., et al. (2015). Deep groundwater and potential subsurface habitats beneath an Antarctic dry valley. Nat. Commun. 6, 6831. doi:10.1038/ncomms7831
Miller, C. E., Griffith, P. C., Goetz, S. J., Hoy, E. E., Pinto, N., Mccubbin, I. B., et al. (2019). An overview of ABoVE airborne campaign data acquisitions and science opportunities. Environ. Res. Lett. 14, 080201. doi:10.1088/1748-9326/ab0d44
Minsley, B. J., Abraham, J. D., Smith, B. D., Cannia, J. C., Voss, C. I., Jorgenson, M. T., et al. (2012). Airborne electromagnetic imaging of discontinuous permafrost. Geophys. Res. Lett. 39. doi:10.1029/2011gl050079
Mishra, U., Drewniak, B., Jastrow, J. D., Matamala, R. M., and Vitharana, U. W. A. (2017). Spatial representation of organic carbon and active-layer thickness of high latitude soils in CMIP5 earth system models. Geoderma 300, 55–63. doi:10.1016/j.geoderma.2016.04.017
Mishra, U.,, and Riley, W. J. (2015). Scaling impacts on environmental controls and spatial heterogeneity of soil organic carbon stocks. Biogeosci. Discuss. 12, 1721–1751. doi:10.5194/bgd-12-1721-2015
Muster, S., Langer, M., Heim, B., Westermann, S., and Boike, J. (2012). Subpixel heterogeneity of ice-wedge polygonal tundra: a multi-scale analysis of land cover and evapotranspiration in the Lena River Delta, Siberia. Tellus B 64, 17301. doi:10.3402/tellusb.v64i0.17301
Naderpour, R.,, and Schwank, M. (2018). Snow wetness retrieved from L-band radiometry. Rem. Sens. 10, 359. doi:10.3390/rs10030359
National Research Council (2014). Opportunities to use remote sensing in understanding permafrost and related ecological characteristics: report of a workshop. Washington, DC: The National Academies Press.
Niu, F., Yin, G., Luo, J., Lin, Z., and Liu, M. (2018). Permafrost distribution along the qinghai-tibet engineering corridor, China using high-resolution statistical mapping and modeling integrated with remote sensing and GIS. Rem. Sens. 10 (2), 215. doi:10.3390/rs10020215
Obu, J., Westermann, S., Bartsch, A., Berdnikov, N., Christiansen, H. H., Dashtseren, A., et al. (2019). Northern Hemisphere permafrost map based on TTOP modelling for 2000–2016 at 1 km2 scale. Earth Sci. Rev. 193, 299–316. doi:10.1016/j.earscirev.2019.04.023
Pan, Y., Lyu, S., Li, S., Gao, Y., Meng, X., Ao, Y., et al. (2017). Simulating the role of gravel in freeze-thaw process on the Qinghai-Tibet Plateau. Theor. Appl. Climatol. 127, 1011–1022. doi:10.1007/s00704-015-1684-7
Panda, S. K., Prakash, A., Solie, D. N., Romanovsky, V. E., and Jorgenson, M. T. (2010). Remote sensing and field‐based mapping of permafrost distribution along the Alaska Highway corridor, interior Alaska. Permafr. Periglac. Process. 21, 271–281. doi:10.1002/ppp.686
Park, H., Kim, Y., and Kimball, J. S. (2016). Widespread permafrost vulnerability and soil active layer increases over the high northern latitudes inferred from satellite remote sensing and process model assessments. Rem. Sens. Environ. 175, 349–358. doi:10.1016/j.rse.2015.12.046
Pastick, N. J., Jorgenson, M. T., Wylie, B. K., Nield, S. J., Johnson, K. D., and Finley, A. O. (2015). Distribution of near-surface permafrost in Alaska: estimates of present and future conditions. Rem. Sens. Environ. 168, 301–315. doi:10.1016/j.rse.2015.07.019
Peng, X., Zhang, T., Frauenfeld, O. W., Wang, K., Cao, B., Zhong, X., et al. (2017). Response of seasonal soil freeze depth to climate change across China. Cryosphere 11, 1059–1073. doi:10.5194/tc-11-1059-2017
Pepin, N., Deng, H., Zhang, H., Zhang, F., Kang, S., and Yao, T. (2019). An examination of temperature trends at high elevations across the Tibetan plateau: the use of MODIS LST to understand patterns of elevation‐dependent warming. J. Geophys. Res. Atmos. 124, 5738–5756. doi:10.1029/2018jd029798
Qin, Y., Wu, T., Zhao, L., Wu, X., Li, R., Xie, C., et al. (2017). Numerical modeling of the active layer thickness and permafrost thermal state across Qinghai-Tibetan plateau. J. Geophys. Res. Atmos. 122 (11), 604–611. doi:10.1002/2017jd026858
Ran, Y., Li, X., and Cheng, G. (2018). Climate warming over the past half century has led to thermal degradation of permafrost on the Qinghai-Tibet Plateau. Cryosphere 12, 595–608. doi:10.5194/tc-12-595-2018
Rautiainen, K., Parkkinen, T., Lemmetyinen, J., Schwank, M., Wiesmann, A., Ikonen, J., et al. SMOS prototype algorithm for detecting autumn soil freezing. Rem. Sens. Environ. 180, 346–360. doi:10.1016/j.rse.2016.01.012
Rey, D. M., Walvoord, M., Minsley, B., Rover, J., and Singha, K. (2019). Investigating lake-area dynamics across a permafrost-thaw spectrum using airborne electromagnetic surveys and remote sensing time-series data in Yukon Flats, Alaska. Environ. Res. Lett. 14, 025001. doi:10.1088/1748-9326/aaf06f
Riseborough, D., Shiklomanov, N., Etzelmüller, B., Gruber, S., and Marchenko, S. (2008). Recent advances in permafrost modelling. Permafr. Periglac. Process. 19, 137–156. doi:10.1002/ppp.615
Romanovsky, V. E., Drozdov, D. S., Oberman, N. G., Malkova, G. V., Kholodov, A. L., Marchenko, S. S., et al. (2010). Thermal state of permafrost in Russia. Permafr. Periglac. Process. 21, 136–155. doi:10.1002/ppp.683
Schaefer, K., Liu, L., Parsekian, A., Jafarov, E., Chen, A., Zhang, T., et al. (2015). Remotely sensed active layer thickness (ReSALT) at Barrow, Alaska using interferometric synthetic aperture radar. Rem. Sens. 7, 3735–3759. doi:10.3390/rs70403735
Scherler, M., Hauck, C., Hoelzle, M., Stähli, M., and Völksch, I. (2010). Meltwater infiltration into the frozen active layer at an alpine permafrost site. Permafr. Periglac. Process. 21, 325–334. doi:10.1002/ppp.694
Shamir, E.,, and Georgakakos, K. P. (2014). MODIS land surface temperature as an index of surface air temperature for operational snowpack estimation. Rem. Sens. Environ. 152, 83–98. doi:10.1016/j.rse.2014.06.001
Su, B., Rao, R., Li, Z., Song, L., and Yue, J. (2020). Detecting permafrost in plateau and mountainous areas by airborne transient electromagnetic sensing. Electronics 9 (8), 1229. doi:10.3390/electronics9081229
Su, F., Duan, X., Chen, D., Hao, Z., and Cuo, L. (2013). Evaluation of the global climate models in the CMIP5 over the Tibetan Plateau. J. Clim. 26, 3187–3208. doi:10.1175/jcli-d-12-00321.1
Sun, Z., Zhao, L., Hu, G., Qiao, Y., Du, E., Zou, D., et al. (2019). Modeling permafrost changes on the Qinghai-Tibetan plateau from 1966 to 2100: a case study from two boreholes along the Qinghai-Tibet engineering corridor. Permafr. Periglac. Process. 31, 156–171. doi:10.1002/ppp.2022
Tang, G., Long, D., Hong, Y., Gao, J., and Wan, W. (2018). Documentation of multifactorial relationships between precipitation and topography of the Tibetan Plateau using spaceborne precipitation radars. Rem. Sens. Environ. 208, 82–96. doi:10.1016/j.rse.2018.02.007
Tran, A. P., Dafflon, B., Bisht, G., and Hubbard, S. S. (2018). Spatial and temporal variations of thaw layer thickness and its controlling factors identified using time-lapse electrical resistivity tomography and hydro-thermal modeling. J. Hydrol. 561, 751–763. doi:10.1016/j.jhydrol.2018.04.028
Tran, A. P., Dafflon, B., and Hubbard, S. S. (2017). Coupled land surface-subsurface hydrogeophysical inverse modeling to estimate soil organic carbon content and explore associated hydrological and thermal dynamics in the Arctic tundra. Cryosphere 11, 2089–2109. doi:10.5194/tc-11-2089-2017
Ulaby, F. T., Long, D. G., Blackwell, W. J., Elachi, C., Fung, A. K., Ruf, C., et al. (2014). Microwave radar and radiometric remote sensing. Ann Arbor, MI: University of Michigan Press.
Ulaby, F. T., Moore, R. K., and Fung, A. K. (1982). Microwave remote sensing: active and passive. Reading, MA: Addison-Wesley.1064.
Van Der Velde, R., and Su, Z. (2009). Dynamics in land-surface conditions on the Tibetan plateau observed by advanced synthetic aperture radar (ASAR). Hydrol. Sci. J. 54, 1079–1093. doi:10.1623/hysj.54.6.1079
Wainwright, H. M., Dafflon, B., Smith, L. J., Hahn, M. S., Curtis, J. B., Wu, Y., et al. (2015). Identifying multiscale zonation and assessing the relative importance of polygon geomorphology on carbon fluxes in an Arctic tundra ecosystem. J. Geophys. Res. Biogeosci. 120, 788–808. doi:10.1002/2014jg002799
Wang, A., Zeng, X., and Guo, D. (2016a). Estimates of global surface hydrology and heat fluxes from the community land model (CLM4.5) with four atmospheric forcing datasets. J. Hydrometeorol. 17, 2493–2510. doi:10.1175/jhm-d-16-0041.1
Wang, B.,, and French, H. M. (1995). Permafrost on the Tibet plateau, China. Quat. Sci. Rev. 14, 255–274. doi:10.1016/0277-3791(95)00006-b
Wang, C., Zhang, Z., Zhang, H., Zhang, B., Tang, Y., and Wu, Q. (2018). Active layer thickness retrieval of qinghai-tibet permafrost using the TerraSAR-X InSAR technique. IEEE J. Sel. Top. Appl. Earth Observations Remote Sensing 11, 4403–4413. doi:10.1109/jstars.2018.2873219
Wang, G., Liu, G., Li, C., and Yang, Y. (2012a). The variability of soil thermal and hydrological dynamics with vegetation cover in a permafrost region. Agric. For. Meteorol. 162–163, 44–57. doi:10.1016/j.agrformet.2012.04.006
Wang, G., Liu, G., and Li, G. (2012b). Effects of changes in alpine grassland vegetation cover on hillslope hydrological processes in a permafrost watershed. J. Hydrol. 444-445, 22–33. doi:10.1016/j.jhydrol.2012.03.033
Wang, J., Li, H., Hao, X., Huang, X., Hou, J., Che, T., et al. (2014). Remote sensing for snow hydrology in China: challenges and perspectives. J. Appl. Remote Sens. 8 (1), 084687. doi:10.1117/1.jrs.8.084687
Wang, L., Sun, L., Shrestha, M., Li, X., Liu, W., Zhou, J., et al. (2016b). Improving snow process modeling with satellite‐based estimation of near‐surface‐air‐temperature lapse rate. J. Geophys. Res. Atmos. 121 (20), 12005–12030. doi:10.1002/2016jd025506
Wang, L., Zhou, J., Qi, J., Sun, L., Yang, K., Tian, L., et al. (2017). Development of a land surface model with coupled snow and frozen soil physics. Water Resour. Res. 53, 5085–5103. doi:10.1002/2017wr020451
Wang, T., Yang, D., Fang, B., Yang, W., Qin, Y., and Wang, Y. (2019a). Data-driven mapping of the spatial distribution and potential changes of frozen ground over the Tibetan Plateau. Sci. Total Environ. 649, 515–525. doi:10.1016/j.scitotenv.2018.08.369
Wang, W., Yang, K., Zhao, L., Zheng, Z., Lu, H., Mamtimin, A., et al. (2020). Characterizing surface albedo of shallow fresh snow and its importance for snow ablation on the interior of the Tibetan Plateau. J. Hydrometeorol. 21 (4), 815–827. doi:10.1175/jhm-d-19-0193.1
Wang, Y., Chen, J., and Yang, D. (2019b). Bayesian assimilation of multiscale precipitation data and sparse ground gauge observations in mountainous areas. J. Hydrometeorol. 20, 1473–1494. doi:10.1175/jhm-d-18-0218.1
Westermann, S., Elberling, B., Højlund Pedersen, S., Stendel, M., Hansen, B. U., and Liston, G. E. (2015). Future permafrost conditions along environmental gradients in Zackenberg, Greenland. Cryosphere 9, 719–735. doi:10.5194/tc-9-719-2015
Wu, Q., Hou, Y., Yun, H., and Liu, Y. (2015). Changes in active-layer thickness and near-surface permafrost between 2002 and 2012 in alpine ecosystems, Qinghai-Xizang (Tibet) Plateau, China. Global Planet. Change 124, 149–155. doi:10.1016/j.gloplacha.2014.09.002
Wu, Q., Sheng, Y., Yu, Q., Chen, J., and Ma, W. (2020). Engineering in the rugged permafrost terrain on the roof of the world under a warming climate. Permafr. Periglac. Process. 31 (3), 417–428. doi:10.1002/ppp.2059
Wu, Q., Zhang, T., and Liu, Y. (2010). Permafrost temperatures and thickness on the qinghai-tibet plateau. Global Planet. Change 72, 32–38. doi:10.1016/j.gloplacha.2010.03.001
Wu, Q.,, and Zhang, T. (2008). Recent permafrost warming on the Qinghai-Tibetan plateau. J. Geophys. Res. 113. doi:10.1029/2007jd009539
Wu, X., Nan, Z., Zhao, S., Zhao, L., and Cheng, G. (2018). Spatial modeling of permafrost distribution and properties on the Qinghai-Tibet Plateau. Permafr. Periglac. Process. 29, 86–99. doi:10.1002/ppp.1971
Xiao, Y., Zhao, L., Dai, Y., Li, R., Pang, Q., and Yao, J. (2013). Representing permafrost properties in CoLM for the Qinghai-Xizang (Tibetan) plateau. Cold Reg. Sci. Technol. 87, 68–77. doi:10.1016/j.coldregions.2012.12.004
Yan, L.,, and Liu, X. (2014). Has climatic warming over the Tibetan Plateau paused or continued in recent years. J. Earth Ocean Atmos. Sci. 1, 13–28.
Yang, D., Gao, B., Jiao, Y., Lei, H., Zhang, Y., Yang, H., et al. (2015). A distributed scheme developed for eco-hydrological modeling in the upper Heihe River. Sci. China Earth Sci. 58, 36–45. doi:10.1007/s11430-014-5029-7
Yang, K., Chen, Y., He, J., Zhao, L., Lu, H., Qin, J., et al. (2020). Development of a daily soil moisture product for the period of 2002–2011 in Mainland China. Sci. China Earth Sci., 63, 1113–1125. doi:10.1007/s11430-019-9588-5
Yang, K., Qin, J., Zhao, L., Chen, Y., Tang, W., Han, M., et al. (2013a). A multiscale soil moisture and freeze-thaw monitoring network on the third Pole. Bull. Am. Meteorol. Soc. 94, 1907–1916. doi:10.1175/bams-d-12-00203.1
Yang, K., Watanabe, T., Koike, T., Li, X., Fujii, H., Tamagawa, K., Ma, Y., and Ishikawa, H. (2007a). Auto‐calibration system developed to assimilate AMSR‐E data into a land surface model for estimating soil moisture and the surface energy budget. J. Meteorol. Soc. Jpn. Ser. II 85, 229–242.
Yang, K., Wu, H., Qin, J., Lin, C., Tang, W., and Chen, Y. (2014). Recent climate changes over the Tibetan Plateau and their impacts on energy and water cycle: a review. Global Planet. Change 112, 79–91. doi:10.1016/j.gloplacha.2013.12.001
Yang, M., Nelson, F. E., Shiklomanov, N. I., Guo, D., and Wan, G. (2010). Permafrost degradation and its environmental effects on the Tibetan Plateau: a review of recent research. Earth Sci. Rev. 103, 31–44. doi:10.1016/j.earscirev.2010.07.002
Yang, Y., Chen, R.-S., Ye, B.-S., Song, Y.-X., Liu, J.-F., Han, C.-T., et al. (2013b). Heat and water transfer processes on the typical underlying surfaces of frozen soil in cold regions (I): model comparison. J. Glaciol. Geocryol. 35, 1545–1554. [in Chinese with English abstract]. doi:10.7522/j.issn.1000-0240.2013.0171
Yao, T., Thompson, L., Yang, W., Yu, W., Gao, Y., Guo, X., et al. (2012). Different glacier status with atmospheric circulations in Tibetan Plateau and surroundings. Nat. Clim. Change 2 (9), 663–667. doi:10.1038/nclimate1580.
Yi, S., He, Y., Guo, X., Chen, J., Wu, Q., Qin, Y., et al. (2018a). The physical properties of coarse-fragment soils and their effects on permafrost dynamics: a case study on the central Qinghai-Tibetan Plateau. Cryosphere 12, 3067–3083. doi:10.5194/tc-12-3067-2018
Yi, Y., Kimball, J. S., Chen, R. H., Moghaddam, M., Reichle, R. H., Mishra, U., et al. (2018b). Characterizing permafrost active layer dynamics and sensitivity to landscape spatial heterogeneity in Alaska. Cryosphere 12, 145–161. doi:10.5194/tc-12-145-2018
Yi, Y., Kimball, J. S., Rawlins, M. A., Moghaddam, M., and Euskirchen, E. S. (2015). The role of snow cover affecting boreal-arctic soil freeze-thaw and carbon dynamics. Biogeosciences 12, 5811–5829. doi:10.5194/bg-12-5811-2015
Yue, T., Zhao, N., Fan, Z., Li, J., Chen, C., Lu, Y., et al. (2016). CMIP5 downscaling and its uncertainty in China. Global Planet. Change 146, 30–37. doi:10.1016/j.gloplacha.2016.09.003
Zhang, T. (2005). Influence of the seasonal snow cover on the ground thermal regime: an overview. Rev. Geophys. 43. doi:10.1029/2004rg000157
Zhang, Y.-F., Hoar, T. J., Yang, Z.-L., Anderson, J. L., Toure, A. M., and Rodell, M. (2014). Assimilation of MODIS snow cover through the data assimilation research testbed and the community land model version 4. J. Geophys. Res. Atmos. 119, 7091–7103. doi:10.1002/2013jd021329
Zhang, Y. L., Li, X., Cheng, G. D., Jin, H. J., Yang, D. W., Flerchinger, G. N., et al. (2018). Influences of topographic shadows on the thermal and hydrological processes in a cold region mountainous watershed in northwest China. J. Adv. Model. Earth Syst. 10, 1439–1457. doi:10.1029/2017ms001264
Zhao, L., Ping, C.-L., Yang, D., Cheng, G., Ding, Y., and Liu, S. (2004). Changes of climate and seasonally frozen ground over the past 30 years in Qinghai-Xizang (Tibetan) Plateau, China. Global Planet. Change 43, 19–31. doi:10.1016/j.gloplacha.2004.02.003
Zhao, L., Wu, Q., Marchenko, S. S., Sharkhuu, N., and Lewkowicz, A. G. (2010). Thermal state of permafrost and active layer in central asia during the international polar year. Permafr. Periglac. Process. 21, 198–207. doi:10.1002/ppp.688
Zhao, L., Yang, K., Qin, J., Chen, Y., Tang, W., Lu, H., et al. (2014). The scale-dependence of SMOS soil moisture accuracy and its improvement through land data assimilation in the central Tibetan Plateau. Rem. Sens. Environ. 152, 345–355. doi:10.1016/j.rse.2014.07.005
Zhao, L., Zou, D., Hu, G., Du, E., Pang, Q., Xiao, Y., et al. (2020). Changing climate and the permafrost environment on the qinghai–tibet (xizang) plateau. Permafr. Periglac. Process. 31 (3), 396–405. doi:10.1002/ppp.2056
Zhao, S.-P., Nan, Z.-T., Huang, Y.-B., and Zhao, L. (2017a). The application and evaluation of simple permafrost distribution models on the qinghai-tibet plateau. Permafr. Periglac. Process. 28, 391–404. doi:10.1002/ppp.1939
Zhao, T., Shi, J., Hu, T., Zhao, L., Zou, D., Wang, T., et al. (2017b). Estimation of high-resolution near-surface freeze/thaw state by the integration of microwave and thermal infrared remote sensing data on the Tibetan Plateau. Earth and Space Science 4, 472–484. doi:10.1002/2017ea000277
Zhao, T., Zhang, L., Jiang, L., Zhao, S., Chai, L., and Jin, R. (2011). A new soil freeze/thaw discriminant algorithm using AMSR-E passive microwave imagery. Hydrol. Process. 25, 1704–1716. doi:10.1002/hyp.7930
Zhao, W. L., Gentine, P., Reichstein, M., Zhang, Y., Zhou, S., Wen, Y., et al. (2019). Physics‐constrained machine learning of evapotranspiration. Geophys. Res. Lett. 46 (24), 14496–14507. doi:10.1029/2019GL085291
Zheng, D., Li, X., Wang, X., Wang, Z., Wen, J., Van Der Velde, R., et al. (2019a). Sampling depth of L-band radiometer measurements of soil moisture and freeze-thaw dynamics on the Tibetan Plateau. Rem. Sens. Environ. 226, 16–25. doi:10.1016/j.rse.2019.03.029
Zheng, G., Yang, Y., Yang, D., Dafflon, B., Lei, H., and Yang, H. (2019b). Satellite-based simulation of soil freezing/thawing processes in the northeast Tibetan Plateau. Rem. Sens. Environ. 231, 111269. doi:10.1016/j.rse.2019.111269
Zheng, G., Yang, Y., Yang, D., Dafflon, B., Yi, Y., Zhang, S., et al. (2020). Remote sensing spatiotemporal patterns of frozen soil and the environmental controls over the Tibetan Plateau during 2002–2016. Rem. Sens. Environ. 247, 111927. doi:10.1016/j.rse.2020.111927
Zhou, J., Kinzelbach, W., Cheng, G., Zhang, W., He, X., and Ye, B. (2013). Monitoring and modeling the influence of snow pack and organic soil on a permafrost active layer, Qinghai-Tibetan Plateau of China. Cold Reg. Sci. Technol. 90-91, 38–52. doi:10.1016/j.coldregions.2013.03.003
Zhou, J., Wang, G., Li, X., Yang, Y., and Pan, X. (2008). Data assimilation algorithm apply to energy-water balance analysis of the high cold ecosystem at Qinghai-Tibet plain, Northwest China. Adv. Earth Sci. 23, 965–973.
Zhu, F., Cuo, L., Zhang, Y., Luo, J.-J., Lettenmaier, D. P., Lin, Y., et al. (2017). Spatiotemporal variations of annual shallow soil temperature on the Tibetan Plateau during 1983–2013. Clim. Dynam. 51, 2209–2227. doi:10.1007/s00382-017-4008-z
Zhu, W., Lű, A., and Jia, S. (2013). Estimation of daily maximum and minimum air temperature using MODIS land surface temperature products. Rem. Sens. Environ. 130, 62–73. doi:10.1016/j.rse.2012.10.034
Zou, D., Zhao, L., Sheng, Y., Chen, J., Hu, G., Wu, T., et al. (2017). A new map of permafrost distribution on the Tibetan Plateau. Cryosphere 11, 2527–2542. doi:10.5194/tc-11-2527-2017
Keywords: process models, satellite remote sensing, freeze/thaw, permafrost, Tibetan plateau
Citation: Jiang H, Zheng G, Yi Y, Chen D, Zhang W, Yang K and Miller CE (2020) Progress and Challenges in Studying Regional Permafrost in the Tibetan Plateau Using Satellite Remote Sensing and Models. Front. Earth Sci. 8:560403. doi: 10.3389/feart.2020.560403
Received: 08 May 2020; Accepted: 28 October 2020;
Published: 04 December 2020.
Edited by:
Tobias Conradt, Potsdam Institute for Climate Impact Research (PIK), GermanyReviewed by:
Dongliang Luo, Chinese Academy of Sciences (CAS), ChinaGuo Donglin, Institute of Atmospheric Physics (CAS), China
Rinat Manasypov, Tomsk State University, Russia
Susan Hubbard, Lawrence Berkeley National Laboratory, United States
Copyright © 2020 Jiang, Zheng, Yi, Chen, Zhang, Yang and Miller. This is an open-access article distributed under the terms of the Creative Commons Attribution License (CC BY). The use, distribution or reproduction in other forums is permitted, provided the original author(s) and the copyright owner(s) are credited and that the original publication in this journal is cited, in accordance with accepted academic practice. No use, distribution or reproduction is permitted which does not comply with these terms.
*Correspondence: Yonghong Yi, eW9uZ2hvbmcueWlAanBsLm5hc2EuZ292