- British Geological Survey, The Lyell Centre, Edinburgh, United Kingdom
Probabilistic volcanic hazard assessment (PVHA) has become the paradigm to quantify volcanic hazard over the last decades. Substantial aleatory and epistemic uncertainties in PVHA arise from complexity of physico-chemical processes, impossibility of their direct observation and, importantly, a severe scarcity of observables from past eruptions. One factor responsible for data scarcity is the infrequency of moderate/large eruptions; other factors include lack of discoverability and accessibility to volcanological data. Open-access databases can help alleviate data scarcity and have significantly contributed to long-term PVHA of eruption onset and size, while are less common for data required in other PVHA components (e.g., vent opening). Making datasets open is complicated by economical, technological, ethical and/or policy-related challenges. International synergies (e.g., Global Volcanism Program, WOVOdat, Global Volcano Model, EPOS) will be key to facilitate the creation and maintenance of open-access databases that support Next-Generation PVHA. Additionally, clarification of some misconceptions about PVHA can also help progress. Firstly, PVHA should be understood as an expansion of deterministic, scenario-based hazard assessments. Secondly, a successful PVHA should sometimes be evaluated by its ability to deliver useful and usable hazard-related messages that help mitigate volcanic risk. Thirdly, PVHA is not simply an end product but a driver for research: identifying the most relevant sources of epistemic uncertainty can guide future efforts to reduce the overall uncertainty. Broadening of the volcanological community expertise to statistics or engineering has already brought major breakthroughs in long-term PVHA. A vital next step is developing and maintaining more open-access datasets that support PVHA worldwide.
Introduction
Nearly 300,000 people died due to volcanic activity from 1600 to 2010 AD (Auker et al., 2013). Unfortunately, several other deadly eruptions have shocked the world since then. Some because of their lack of clear precursory evidence: Mayon, Philippines, in 2013 (Maeda et al., 2015). Others because of the magnitude of their destructive toll: Fuego, Guatemala, in June 2018 (Naismith et al., 2019) and Krakatau, Indonesia, in December 2018 (Grilli et al., 2019; Williams et al., 2019). One of the most infamous examples of volcano tragedy was the eruption of Mount Pelée, Martinique, in 1902, which claimed the life of around 30,000 people at St Pierre and Morne Rouge (Lacroix, 1904; Fisher et al., 1980). At the time of the eruption, it was assumed that “Mount Pelée would behave in 1902 as it had in 1851 – when a rain of ash […] did not harm those living under its shadow” (Reed, 2002). However, the timing, location, size and style of volcanic activity tend to change from eruption to eruption and within the same eruption. Thus, properly accounting for and quantifying this natural variability, or aleatory uncertainty, of volcanic eruptions and their associated hazardous phenomena is a basic requirement for volcanic hazard assessment (e.g., Woo, 1999; Connor et al., 2001; Marzocchi et al., 2004). Such assessments should also quantify the epistemic uncertainty related to incomplete knowledge (e.g., Marzocchi et al., 2004; Rougier and Beven, 2013; Tierz et al., 2016a). This can be achieved through probabilistic analyses of the onset, size and location of volcanic eruptions, as well as of the spatio-temporal intensity of hazardous phenomena such as pyroclastic density currents (PDCs) or lahars. Probabilistic volcanic hazard assessment (PVHA) started to develop several decades ago (e.g., Newhall, 1982; Barberi et al., 1990; Connor and Hill, 1995; Connor et al., 2001; Newhall and Hoblitt, 2002; Aspinall et al., 2003; Sparks, 2003) and has now become the standard method for robust, accurate and complete volcanic hazard assessment worldwide (e.g., Marzocchi et al., 2008, 2010; Bayarri et al., 2009; Sobradelo and Martí, 2010; Jenkins et al., 2012; Marzocchi and Bebbington, 2012; Bebbington, 2013, 2014; Del Negro et al., 2013; Hincks et al., 2014; Connor et al., 2015; Neri et al., 2015; Newhall and Pallister, 2015; Whelley et al., 2015; Bevilacqua et al., 2016; Biass et al., 2016; Mead and Magill, 2017; Tierz et al., 2017; Sandri et al., 2018).
Carrying out PVHA requires the collection and use of volcanological data (Figure 1) to select and parameterize probability distributions to calculate volcanic hazard, including the critical sources of uncertainty (e.g., Connor and Hill, 1995; Aspinall, 2006; Marzocchi et al., 2008, 2010; Marzocchi and Bebbington, 2012; Bebbington, 2013; Tierz et al., 2016a,b). While these tasks are very data demanding, the vast majority of volcanic systems in the world remain data scarce (e.g., Loughlin et al., 2015). Open data are crucial to help alleviate the widespread issue of data scarcity and, hence, support advancement of PVHA. For instance, open data can be used to borrow useful information from analogue volcanoes (i.e., volcanoes that have enough similar characteristics as to be considered partially exchangeable) and apply it to perform PVHA at a specific (data-scarce) target volcano (e.g., Marzocchi et al., 2004; Bebbington, 2014; Sheldrake, 2014; Ogburn et al., 2016a; Sheldrake et al., 2016; Newhall et al., 2017; Tierz et al., 2019). Some open-access volcanological databases that contain the necessary data to compute PVHA are currently available: Volcanoes of the world, Global Volcanism Program (Siebert et al., 2010; hereinafter GVP)1; WOVOdat (Newhall et al., 2017)2; Large Magnitude Explosive Volcanic Eruptions (Crosweller et al., 2012; hereinafter LaMEVE)3 and others. However, the majority of the volcanological community still heavily relies on non-open data to perform PVHA (e.g., Bevilacqua et al., 2016; Jaquet et al., 2017; Tierz et al., 2018). The predominant use of non-open data reduces the versatility and scope of PVHA methods because researchers worldwide may find it difficult to gain independent insights from the same datasets. Conversely, open-access datasets permit such complementary research to be developed (e.g., Deligne et al., 2010; Sheldrake and Caricchi, 2017; Papale, 2018; Rougier et al., 2018).
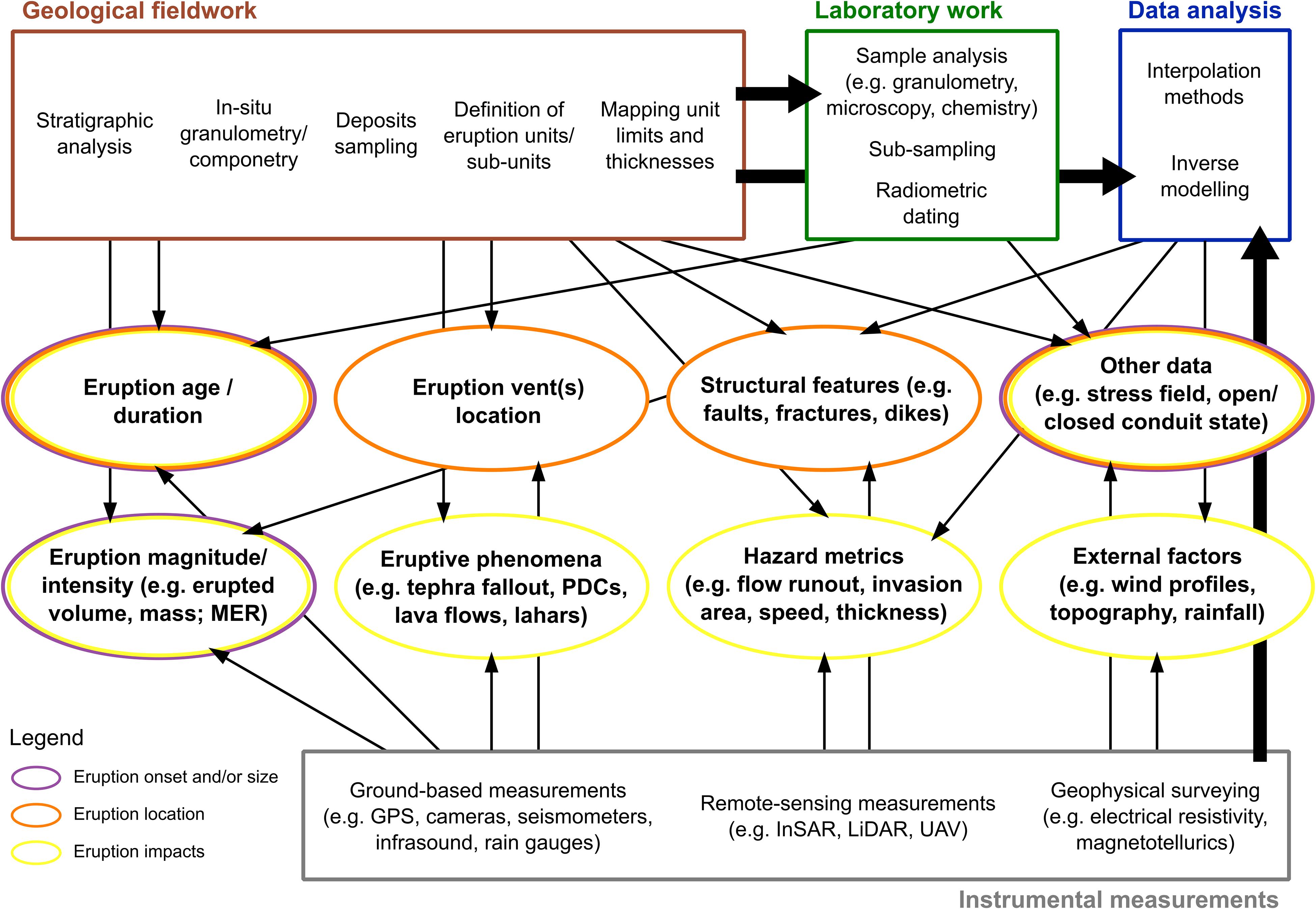
Figure 1. Schematic showing the main types of data (ellipses) that are required to carry out long-term PVHA and some intermediate methods or steps (rectangles) needed to obtain these data. Arrows indicate links between intermediate steps or with derived PVHA data types and variables (ellipses and text within parentheses). Data types are classified according to their use in different components of the hazard assessment: eruption onset and/or size, eruption location and eruption impacts. The majority of the data are generated, directly or indirectly, through geological fieldwork performed on the eruptive products of past volcanic eruptions. Since historical times, direct observations and instrumental measurements of both eruptive parameters as well as external factors (e.g., wind, rainfall, topography) have also become available. MER, Mass Eruption Rate; PDCs, Pyroclastic Density Currents; GPS, Global Positioning System; InSAR, Interferometric Synthetic Aperture Radar; LiDAR, Light Detection And Ranging; UAV, Unmanned Aerial Vehicle.
In this contribution, I explore the use of open and non-open data in long-term PVHA (i.e., volcanic hazard forecasts on the timescale of years to decades, Marzocchi and Bebbington, 2012) to evidence how the key issue of data scarcity manifests in the predominant and uneven use of non-open data across different components of the probabilistic assessment. Then, I discuss the importance of data scarcity and misconceptions around PVHA as current issues that collectively hinder further and wider development in the field. Finally, I suggest a few potential future directions in the view of an increased availability of open-access datasets to perform long-term PVHA at the individual volcano, regional and global scales.
Open and Non-Open Data in Long-Term PVHA
I have selected a collection of 100 peer-reviewed contributions to analyze long-term PVHA research since 2001 (see Supplementary Material). The collection covers, without over-representation, the spectrum of different research groups, volcanic systems, types of PVHA and hazardous phenomena. Although it is not necessarily statistically representative in terms of stratified random sampling, it nevertheless serves to illustrate certain research trends in PVHA over the last two decades. This collection, which I will henceforth denote as the “Sample” is analyzed (Figure 2; and Supplementary Material), focusing on the use of open, non-open and mixed (i.e., both open and non-open) datasets (see Definitions) to assess different components of the long-term forecast: (1) eruption onset and size; (2) eruption vent location; (3) eruption impacts (by hazardous phenomena); and (4) combinations of any of the three, which I term integrated PVHA. In the Sample, studies that use either non-open or mixed datasets dominate over those using open datasets to compute long-term PVHA (Figures 2A,B). According to the Sample, there have not been major changes in the use of open, non-open and mixed datasets over time (Figure 2C).
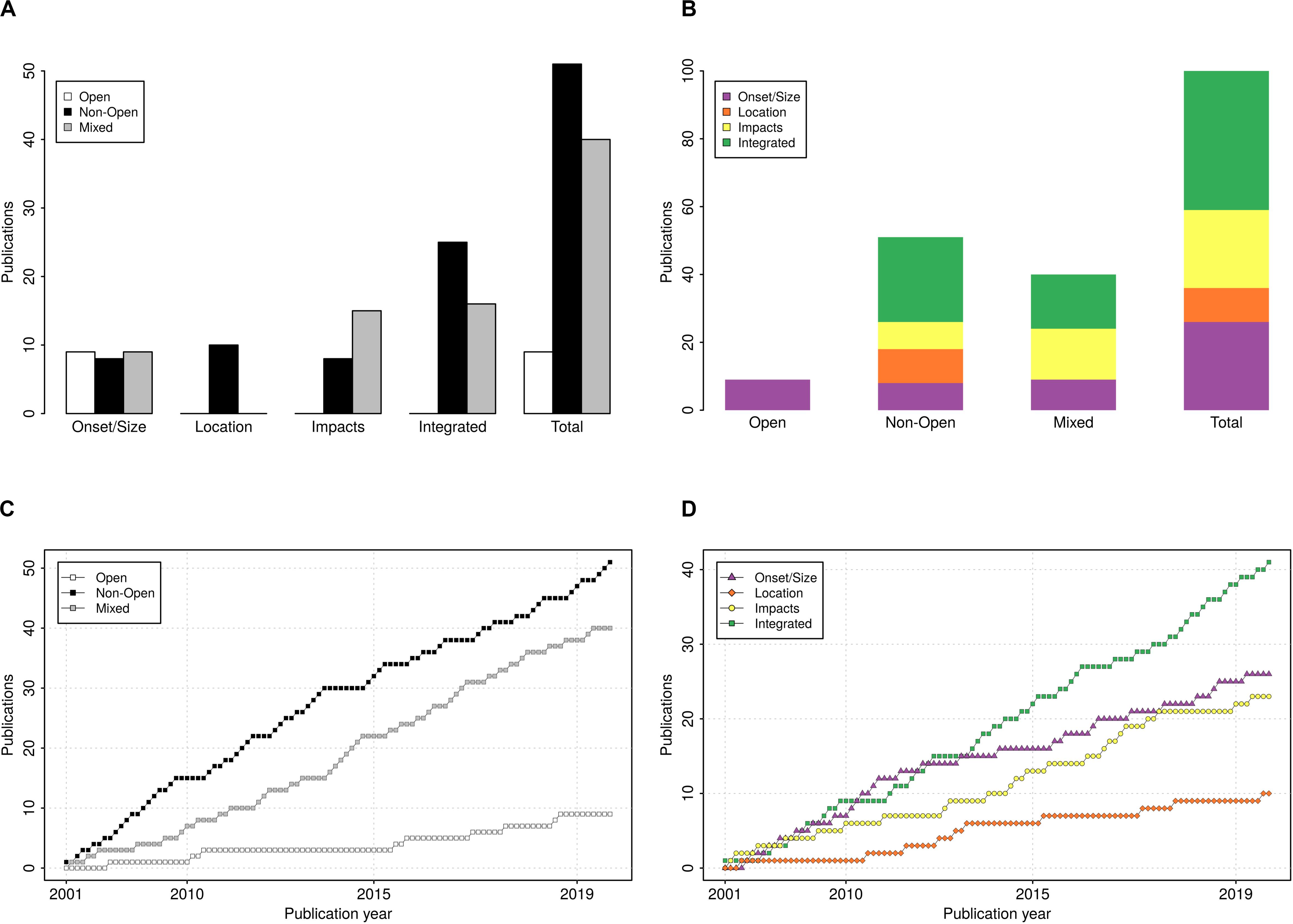
Figure 2. Summary of patterns observed on a collection of 100 publications on long-term PVHA published since 2001. In (A,B): total number of publications partitioned according to the use of open, non-open and mixed datasets (A); and the focus of the PVHA: eruption onset and/or size, eruption location, eruption impacts or integrated PVHA (B). In (C,D): cumulative number of publications according to the same partitions: type of data (C) and focus of the PVHA (D). Publications are sorted, in ascending order, by the year of publication and, for those with the same publication year, by alphabetical order. The x axis displays the resulting ordinal numbering (ID variable in the Supplementary Material). Labels on the x axis are placed on the first publication of the corresponding publication year.
Eruption Onset and Size
It is the only component of the hazard assessment where open data have been used in isolation (Figure 2B) and non-open and mixed data do not dominate (Figure 2A). Global databases of the timing and size of volcanic eruptions (principally GVP and LaMEVE) have been the key element required for the hazard analysts to conduct PVHA. The Sample suggests that eruption onset-size forecasts were more common during approximately the first decade of the 21st century (Figure 2D). This relative decrease could be partially explained by the incorporation of onset-size assessments into integrated PVHA over the second decade of the century (e.g., Sandri et al., 2012, 2014, 2018; Bartolini et al., 2015; Bevilacqua et al., 2017). Recent advances in PVHA of eruption onset and size have focused on alleviating issues around under-recording (Mead and Magill, 2014; Rougier et al., 2016; Sheldrake and Caricchi, 2017) and on the use of covariates to inform the statistical modeling: e.g., open/closed-conduit states (Bebbington, 2014; Whelley et al., 2015); rock geochemistry (Passarelli and Brodsky, 2012); volcano type or tectonic setting (Sheldrake, 2014; Sheldrake and Caricchi, 2017); or a combination of volcanological observations (Dzierma and Wehrmann, 2010, 2014). Moreover, advanced stochastic models to incorporate the epistemic uncertainty in the rates of eruption onsets, and model varied patterns of clustering and recurrent behavior of volcanic eruptions, have been proposed (e.g., Garcia-Aristizabal et al., 2012; Bebbington, 2013; Bevilacqua et al., 2016, 2018).
Eruption Vent Location
It has been exclusively performed using non-open data, according to the Sample (Figure 2A). This is strongly related to the fact that the detailed geological data (Figure 1) required to derive statistical models for the spatial occurrence of vent locations (e.g., Cappello et al., 2012; Connor et al., 2012; Selva et al., 2012b; Bevilacqua et al., 2015), are rarely available as open-access datasets. The data are available at specific volcanic systems but generalizations about the data-generating process for eruption location are hindered because of the lack of cross-volcano, open-access databases (NB. Le Corvec et al., 2013, could be considered as a “non-open archetype” for such databases). Some recent developments have looked at: (i) constraining the areal extent of vent locations by matching it to potential magma sources identified from geophysical imaging (Deng et al., 2017); or (ii) assessing changes in vent locations due to spatial migration of volcanism in geological time (Jaquet et al., 2017).
Eruption Impacts
Mixed datasets are more commonly used than non-open datasets (Figure 2A). This may be due to an increased accessibility to databases of some key elements for the PVHA such as wind profiles for tephra fallout (Kalnay et al., 1996; Dee et al., 2011) or digital elevation models for mass flows (e.g., Shuttle Radar Topography Mission)4. Recent advances are linked with: (1) the comprehensive quantification of inter- and intra-eruption-size aleatory variability of quantities such as the mass eruption rate, erupted mass or volume, column height, column-collapse height, etc., (e.g., Biass et al., 2016; Sandri et al., 2016; Tierz et al., 2016b); and (2) the recognition and quantification of diverse sources of epistemic uncertainty, which significantly affect the PVHA (e.g., Stefanescu et al., 2012a, b; Spiller et al., 2014; Tierz et al., 2016a). The parallel development of detailed, yet relatively fast, physical models and versatile statistical models has aided the quantification of the aforementioned uncertainties. Some examples include open-access numerical codes for granular flows (TITAN2D, Patra et al., 2005; LaharFlow)5 and uncertainty quantification techniques ranging from probabilistic graphical models (e.g., Sobradelo et al., 2014; Tonini et al., 2015; Tierz et al., 2017) to polynomial chaos methods (Dalbey et al., 2008; Tierz et al., 2018) and stochastic-process emulators (Bayarri et al., 2009; Spiller et al., 2014; Rutarindwa et al., 2019).
Integrated PVHA
According to the Sample, it has been the most common PVHA over the last years (Figure 2D) and it predominantly uses non-open data (Figure 2A). Notwithstanding, the range and scope of open-access databases for integrated PVHA seems to be increasing. Long-term programs like GVP have been joined by other efforts such as LaMEVE, FlowDat (Ogburn, 2012)6, DomeHaz (Ogburn et al., 2015)7, the Eruption Source Parameter database (Mastin et al., 2009)8, the Catalog of Icelandic Volcanoes9, etc. Recent advances have used some of these datasets to parameterize event trees in novel multi-volcano PVHA of tephra fallout (Jenkins et al., 2012) or PDCs (Sandri et al., 2018). Other advances, with potential applicability to integrated PVHA, have designed: (a) community cyberinfrastructure platforms that host or link to other open-access databases and provide online tools to support hazard assessment (Vhub, Palma et al., 2014; Volcanic Hazards Assessment Support System by G-EVER, Takarada, 2017)10,11; or (b) methods to identify objective sets of analogue volcanoes from global databases (VOLCANS, Tierz et al., 2019).
Discussion
In my view, further and wider development of long-term PVHA is hindered by data scarcity as well as by some misconceptions and criticism toward PVHA itself.
Data Scarcity in PVHA
Volcanological data (Figure 1) are the best possible source of information to quantify aleatory uncertainty in volcanic hazard (e.g., Newhall and Hoblitt, 2002; Marzocchi and Bebbington, 2012; Connor et al., 2015; Pallister et al., 2019). Data discovery can alter this quantification by changing the experimental concept (Marzocchi and Jordan, 2014) and this is especially acute at the scale of individual volcanoes and for infrequent, large-size eruptions. For instance, for some decades, the Campi Flegrei caldera (Italy) was known to have undergone two caldera-forming eruptions, one at ∼39 ka (the Campanian Ignimbrite eruption; Fedele et al., 2003) and another at ∼15 ka (the Yellow Neapolitan Tuff eruption; Deino et al., 2004). Recently, new evidence for a third caldera-forming eruption at ∼29 ka (the Masseria del Monte Tuff eruption) has been found (Albert et al., 2019). This discovery has drastically reduced the expected repose interval for caldera-forming eruptions at Campi Flegrei.
It is also important to distinguish between lack of data discoverability (Loughlin et al., 2015) and lack of data accessibility (see Definitions). At present, a number of volcanological datasets, typically collected at specific volcanic systems (e.g., Cioni et al., 2008; Thompson et al., 2015; Bevilacqua et al., 2018), are stored in non-open-access publications and, hence, are not freely available to the entire volcanological community. Open-access publications are becoming more customary, which increases the availability of open data to perform PVHA (e.g., Sobradelo et al., 2011; Biass et al., 2014; Jenkins et al., 2019), but there is still an urgent need to discover, assemble, digitize and store volcanological data in open-access databases (e.g., Geyer and Marti, 2008; Ogburn, 2012; Ogburn et al., 2015, 2016b; Newhall et al., 2017) that help develop Next-Generation PVHA. International synergies, such as GVP, the Global Volcano Model12, European Plate Observing System13, European Open Science Cloud14 or EarthCube15, can play key roles in facilitating the generation and maintenance of such databases, as well as in the transition toward open science in general, for which not only open data but open models and workflows would also be required.
There are significant and varied challenges that play a role in the release of open data. For instance, individual researchers or institutions may be reluctant to release their data until fundamental research has been successfully conducted using those data. Hence, a grace period of a few years (e.g., 2 years in WOVOdat)16 can provide the scope for initial interpretation and publication of results linked to the data. Other difficulties might be: economical and/or logistical (e.g., availability of long-term funding and tailored student supervision to help make existing data accessible), technological (e.g., software and hardware development) and/or legal and policy-related, ranging from intellectual property rights to protection of personal data. For instance, data-protection policies may be different across countries and/or change over time, within a given country or region (e.g., General Data Protection Regulation of May 2018 in Europe17). Particularly in volcanology, expert elicitation has become an important tool to extract data for PVHA (e.g., Aspinall, 2006; Selva et al., 2012a; Hincks et al., 2014; Thompson et al., 2015; Christophersen et al., 2018). The elicitation process has ethical and legal implications (e.g., Hemming et al., 2018), such as anonymity of the generated data, that need to be carefully considered while moving toward more open-data and open-science environments that facilitate PVHA worldwide.
Misconceptions Around PVHA
I focus here on three that I consider the most relevant. First, probabilistic approaches are sometimes criticized because of their overall complexity. However, it should be noted that PVHA is an expansion of deterministic approaches (Marzocchi and Bebbington, 2012). That is, a probabilistic hazard product can be converted into a “deterministic” one while the contrary does not hold. The versatility of PVHA should be an asset, not a detriment. For instance, a collection of hazard curves covering a spatial grid actually represents a set of probability and hazard maps that can be derived from the hazard curves by using thresholds in either the hazard-intensity measure (e.g., flow speed) or the probability of exceedance, respectively (e.g., Selva et al., 2014; Tonini et al., 2015; Tierz et al., 2018). The latter case is effectively equivalent to producing a scenario-based hazard map with known probability of exceedance. One key difference in deterministic, scenario-based hazard maps is that the associated probability of exceedance is not known (e.g., Esposti Ongaro et al., 2008; Charbonnier and Gertisser, 2012; Capra et al., 2015). Nevertheless, it is also important to acknowledge that probabilistic and deterministic approaches should not be seen as an “either-or choice” (e.g., Newhall and Pallister, 2015; Rouwet et al., 2017): both methods are reciprocally informative and beneficial for the goal of improving volcanic hazard assessments (e.g., Marzocchi et al., 2008; Marzocchi and Bebbington, 2012; Newhall and Pallister, 2015).
Secondly, validation of probabilistic hazard forecasts is necessary (Marzocchi and Bebbington, 2012; Marzocchi and Jordan, 2014; Connor et al., 2015) but not always there are enough data (Figure 1) available for such purpose (e.g., Marzocchi et al., 2004, 2008). In this context, a successful volcanic hazard assessment should be seen as one that efficiently delivers vital messages that help mitigation of volcanic risk (Pallister et al., 2019). Depending on the volcanological and socio-political context, the nature of this message can vary remarkably: from evidencing the possibility of far-reach PDCs during escalating phases of unrest (e.g., Mount Pinatubo, in June 1991: Newhall and Punongbayan, 1996; Newhall and Pallister, 2015) to raising awareness of the relevance of volcanic hazard at under-studied explosive volcanic systems with long repose intervals (e.g., Main Ethiopian Rift: Vye-Brown et al., 2016). In densely populated, heavily industrialized areas built on or surrounded by several volcanoes (e.g., the metropolitan area of Napoli, Italy, ∼3 M inhabitants), long-term PVHA represents a vital asset to deliver effective hazard-related messages. Considering Somma-Vesuvius and Campi Flegrei separately, long-term PDC hazard is most crucially controlled by different aspects at the two volcanoes. At Somma-Vesuvius, the size of the next eruption is a key factor controlling the volume and spatial reach of PDCs (Gurioli et al., 2010; Tierz et al., 2016a, b; Sandri et al., 2018) while vent-opening variability (Tadini et al., 2017) has a limited influence, partly due to major topographic controls on PDC propagation exerted by the stratocone and Mount Somma (Esposti Ongaro et al., 2008; Tierz et al., 2016a, 2018). At Campi Flegrei, eruption size has a more restricted effect on the volume and spatial reach of PDCs, for the most-likely explosive eruption sizes (Orsi et al., 2004, 2009; Neri et al., 2015; Tierz et al., 2016b). However, the location of the next eruptive vent (Selva et al., 2012b; Bevilacqua et al., 2015; Rivalta et al., 2019) has a paramount importance, given the complex intra-caldera topography and the smaller PDC invasion areas at Campi Flegrei (Tierz et al., 2016b; Bevilacqua et al., 2017; Sandri et al., 2018).
Finally, PVHA is often solely seen as an end product while it should also be considered a driver for research. If epistemic uncertainties are comprehensively quantified and ranked (e.g., Stefanescu et al., 2012a, b; Rougier and Beven, 2013; Spiller et al., 2014; Tierz et al., 2016a), then sensitivity of PVHA outputs can be explicitly explored (e.g., Tierz et al., 2016a; Bevilacqua et al., 2017; Sandri et al., 2018), and data collection can be aimed at reducing the overall uncertainty to improve volcanic hazard assessment (e.g., Tierz et al., 2016a; Trolese et al., 2019). The above example of PVHA of PDCs at the metropolitan area of Napoli exemplifies how research can focus on the most critical, volcano-specific aspects linked to PDC hazard: e.g., spatial probability of vent opening at Campi Flegrei and probability of eruption size at Somma-Vesuvius.
Future Directions
I propose four major steps to improve long-term PVHA in the context of an increased availability of open-access datasets:
1. Unraveling the links between global and local frequency-magnitude distributions: there is still debate about the portability of global frequency-magnitude distributions (Papale, 2018; Rougier et al., 2018) to regional (Sheldrake and Caricchi, 2017) and individual-volcano scales (Bebbington, 2014). Bayesian hierarchical models are a structured approach to accommodate end-member interpretations (Ogburn et al., 2016a) but different exchangeability assumptions between analogue volcanoes should be investigated to improve such models. Novel tools to identify objective sets of analogue volcanoes will facilitate such analyses (VOLCANS, Tierz et al., 2019);
2. Toward the use of data-generating processes to model vent locations: it would be preferable to use the data-generating process itself (i.e., magma transfer to the surface), instead of mostly focusing of past-vents data, to derive spatial PDFs of vent opening. Further developments in: (a) testing the spatial distributions of past vent locations against meaningful geological and geophysical data (Martin et al., 2004; Runge et al., 2016); and/or (b) applying hybrid physical-statistical approaches to simulate dike propagation trajectories and calculate spatial probabilities for future vent locations (Rivalta et al., 2019) are promising ways forward;
3. Increased granularity in intra-eruption forecasting: almost every PVHA of hazardous phenomena aggregates volcanic activity at the level of the single eruption (e.g., Jenkins et al., 2012; Becerril et al., 2014; Sandri et al., 2018) but very few incorporate the dynamic nature of intra-eruption phenomenology (e.g., Wolpert et al., 2018; Bebbington and Jenkins, 2019). More effort should be put in this direction and open-access, global datasets will be a fundamental resource for probabilistic models that incorporate this level of granularity in eruption dynamics (Cassidy et al., 2018);
4. From hazard summation to hazard interaction in multi-hazard assessments at volcanoes: the majority of volcanic multi-hazard assessments analyze hazardous phenomena separately and then combine or add their resulting hazard footprints (e.g., Sandri et al., 2014; Bartolini et al., 2015). There are very few examples where the hazard interaction is explicitly quantified (e.g., Volentik et al., 2009; Tierz et al., 2017). Probabilistic graphical models (Koller and Friedman, 2009) can help quantify such critical interactions and their construction could strongly benefit from techniques such as machine learning (Anantrasirichai et al., 2019), if enough open volcanological data (Figure 1) were available.
The volcanological community has been able to enlarge its collective expertise over the last decades by engaging with scientists from diverse fields such as statistics, engineering or computer science. This has already resulted in major breakthroughs in long-term PVHA (e.g., Barberi et al., 1990; Wadge et al., 1994; Connor and Hill, 1995; Newhall and Hoblitt, 2002; Bayarri et al., 2009; Marzocchi et al., 2010; Jenkins et al., 2012; Bebbington, 2014, 2015; Jaquet et al., 2017; Tierz et al., 2017; Sandri et al., 2018). A vital next step relates to creating, expanding and maintaining more open-access volcanological datasets, as well as open-source software and workflows (e.g., data processing), that can support long-term PVHA worldwide.
Definitions
Open Data
Any type of data that any user can access and re-use, entirely free of charge, through a device equipped with an Internet connection (NB. Data in the GVP database is considered as open data, even prior to its release as an open-access database in 2002, Siebert and Simkin, 2002). Please note that fully open data would also imply free availability of the software and workflows used to generate these data. A simplified approach, where open data refers only to data used to compute long-term PVHA (see Figure 1), was taken here for the sake of facilitating the analysis shown in Figure 2 and the Electronic Supplementary Material.
Non-open Data
Any type of data that does not comply with the definition given for open data. For example, all data found in subscription-based or pay-per-view articles is considered non-open. Please note that many authors may be willing to grant open access to their data upon publication. However, from the user point of view, data accessibility ultimately depends on the specific access policy associated with each published article.
Data Scarcity
General lack of data for any given volcano or group of volcanoes, independently of the reason behind this scarcity.
Data Discoverability
Quality of any kind of data for a given volcano or group of volcanoes of being able to be discovered through identification, description, measurement, sampling, etc.
Data Accessibility
Quality of any kind of data for a given volcano or group of volcanoes of being able to be accessed (and re-used) as open data. Please note that the concept of data accessibility may contain finer gradations (e.g., data in repositories, supplementary material files; maps drawn on an article; data available from author upon request, etc.), which would result in more data categories than the three distinguished in this manuscript: open, non-open and mixed. A simplified categorisation was chosen here for the sake of facilitating the analysis shown in Figure 2 and the Electronic Supplementary Material.
Data Availability
Quality of any kind of data for a given volcano or group of volcanoes of being available for its re-use as open data. In the context of the manuscript, synonym of data accessibility.
Author’s Note
New data used in this study are shown in the figures presented and available in the Supplementary Material. Figures were generated using Inkscape software (Harrington, 2004–2005) and R programming language (R Core Team, 2013) and color schema were based on www.ColorBrewer.org (Brewer, 2018).
Data Availability Statement
All datasets generated for this study are included in the article/Supplementary Material.
Author Contributions
PT conceived this perspective, collected and processed the data, analyzed the results, generated the figures, wrote the manuscript, and approved all its versions.
Funding
The research leading to these results has been supported by Global Geological Risk Platform of the British Geological Survey NC-ODA grant NE/R000069/1: Geoscience for Sustainable Futures.
Conflict of Interest
The authors declare that the research was conducted in the absence of any commercial or financial relationships that could be construed as a potential conflict of interest.
Acknowledgments
I would like to thank the Topic Editors: Elisa Trasatti, Fidel Costa, and Michelle Parks, for having invited me to submit a manuscript to their research topic. I am very grateful to Mark Bebbington, Chris Newhall, and an anonymous reviewer for their thorough revisions and insightful comments, which helped me improve the clarity and completeness of the manuscript, and to Fidel Costa and Valerio Acocella for their helpful feedback and editorial support. I wholeheartedly thank Susan Loughlin for encouraging me to write this perspective and for all her invaluable feedback; and her and Eliza Calder for the very insightful conversations we have shared about volcanoes and volcanic hazard and risk. Using the 1902 eruption at Mount Pelée as an example of the importance of quantifying aleatory uncertainty in PVHA was an idea envisaged by Elaine Spiller. I would like to thank Andrea Di Vico for helpful conversations. I profoundly thank Laura Sandri and Warner Marzocchi for allowing me to learn so much about statistics and volcanic hazard assessment from them. For having also contributed to increasing my understanding of volcanic systems and volcanic hazard assessment, I will try to summarize my warmest thanks to: Marceliano Lago, Carlos Galé, Teresa Ubide, Patricia Larrea, Joan Martí, Paolo Papale and all the NEMOH family, Antonio Costa, Roberto Sulpizio, Mauro Antonio Di Vito, Lucia Zaccarelli, Jacopo Selva, Abani Patra, Ramona Stefanescu, Greg Valentine, Sarah Ogburn, Sylvain Charbonnier, Henry Odbert, Mark Woodhouse, Jeremy Phillips, Ben Clarke, Elaine Spiller, and Bruce Pitman. Many other colleagues, reviewers and editors have played a crucial role in discussions that have made me improve my knowledge and perspectives on probabilistic volcanic hazard assessment and volcanoes in general. I would like to sincerely thank them all. Published with permission of the Executive Director of British Geological Survey (NERC-UKRI).
Supplementary Material
The Supplementary Material for this article can be found online at: https://www.frontiersin.org/articles/10.3389/feart.2020.00257/full#supplementary-material
Footnotes
- ^ http://www.volcano.si.edu
- ^ https://www.wovodat.org/
- ^ https://www.bgs.ac.uk/vogripa/view/controller.cfc?method=lameve
- ^ https://www2.jpl.nasa.gov/srtm/
- ^ https://www.laharflow.bristol.ac.uk/
- ^ https://vhub.org/resources/2076
- ^ https://vhub.org/resources/1742
- ^ https://www.bgs.ac.uk/research/volcanoes/esp/search.cfc?method=viewHome
- ^ http://icelandicvolcanos.is/
- ^ https://vhub.org/
- ^ http://volcano.g-ever1.org/
- ^ https://globalvolcanomodel.org/
- ^ https://epos-ip.org/
- ^ https://ec.europa.eu/research/openscience/index.cfm?pg=open-science-cloud
- ^ https://earthcube.org
- ^ https://wovodat.org/about/about.php
- ^ https://ec.europa.eu/info/law/law-topic/data-protection/data-protection-eu_en
References
Albert, P. G., Giaccio, B., Isaia, R., Costa, A., Niespolo, E. M., Nomade, S., et al. (2019). Evidence for a large-magnitude eruption from campi flegrei caldera (Italy) at 29 ka. Geology 47, 595–599. doi: 10.1130/g45805.1
Anantrasirichai, N., Biggs, J., Albino, F., and Bull, D. (2019). A deep learning approach to detecting volcano deformation from satellite imagery using synthetic datasets. Remote Sens. Environ. 230:111179. doi: 10.1016/j.rse.2019.04.032
Aspinall, W. P. (2006). “Structured elicitation of expert judgment for probabilistic hazard and risk assessment in volcanic eruptions,” in Statistics in Volcanology, eds H. M. Mader, S. G. Coles, C. B. Connor, and L. J. Connor (London: Geological Society), 15–30. doi: 10.1144/iavcei001.2
Aspinall, W. P., Woo, G., Voight, B., and Baxter, P. J. (2003). Evidence-based volcanology: application to eruption crises. J. Volcanol. Geotherm. Res. 128, 273–285. doi: 10.1016/s0377-0273(03)00260-9
Auker, M., Sparks, R., Siebert, L., Crosweller, H., and Ewert, J. (2013). A statistical analysis of the global historical volcanic fatalities record. J. Appl. Volcanol. 2:2.
Barberi, F., Macedonio, G., Pareschi, M. T., and Santacroce, R. (1990). Mapping the tephra fallout risk: an example from Vesuvius, Italy. Nature 344, 142–144. doi: 10.1038/344142a0
Bartolini, S., Bolós, X., Martí, J., Pedra, E. R., and Planagumà, L. (2015). Hazard assessment at the quaternary la garrotxa volcanic field (NE Iberia). Nat. Hazards 78, 1349–1367. doi: 10.1007/s11069-015-1774-y
Bayarri, M. J., Berger, J. O., Calder, E. S., Dalbey, K., Lunagomez, S., Patra, A. K., et al. (2009). Using statistical and computer models to quantify volcanic hazards. Technometrics 51, 402–413. doi: 10.1198/tech.2009.08018
Bebbington, M. S. (2013). Assessing probabilistic forecasts of volcanic eruption onsets. Bull. Volcanol. 75:783.
Bebbington, M. S. (2014). Long-term forecasting of volcanic explosivity. Geophys. J. Int. 197, 1500–1515. doi: 10.1093/gji/ggu078
Bebbington, M. S. (2015). Spatio-volumetric hazard estimation in the Auckland volcanic field. Bull. Volcanol. 77:39.
Becerril, L., Bartolini, S., Sobradelo, R., Martí, J., Morales, J. M., and Galindo, I. (2014). Long-term volcanic hazard assessment on El Hierro (Canary Islands). Nat. Hazards Earth Syst. Sci. 14, 1853–1870.
Bevilacqua, A., Bursik, M., Patra, A., Bruce Pitman, E., Yang, Q., Sangani, R., et al. (2018). Late Quaternary eruption record and probability of future volcanic eruptions in the Long Valley volcanic region (CA, USA). J. Geophys. Res. Solid Earth 123, 5466–5494. doi: 10.1029/2018jb015644
Bevilacqua, A., Flandoli, F., Neri, A., Isaia, R., and Vitale, S. (2016). Temporal models for the episodic volcanism of campi flegrei caldera (Italy) with uncertainty quantification. J. Geophys. Res. Solid Earth 121, 7821–7845. doi: 10.1002/2016jb013171
Bevilacqua, A., Isaia, R., Neri, A., Vitale, S., Aspinall, W. P., Bisson, M., et al. (2015). Quantifying volcanic hazard at Campi Flegrei caldera (Italy) with uncertainty assessment: 1. Vent opening maps. J. Geophys. Res. Solid Earth 120, 2309–2329. doi: 10.1002/2014jb011775
Bevilacqua, A., Neri, A., Bisson, M., Esposti Ongaro, T., Flandoli, F., Isaia, R., et al. (2017). The effects of vent location, event scale, and time forecasts on pyroclastic density current hazard maps at campi flegrei caldera (Italy). Front. Earth Sci. 5:72. doi: 10.3389/feart.2017.00072
Biass, S., Bonadonna, C., Connor, L., and Connor, C. (2016). TephraProb: a MATLAB package for probabilistic hazard assessments of tephra fallout. J. Appl. Volcanol. 5:10.
Biass, S., Scaini, C., Bonadonna, C., Folch, A., Smith, K., and Höskuldsson, A. (2014). A multi-scale risk assessment for tephra fallout and airborne concentration from multiple Icelandic volcanoes–Part 1: hazard assessment. Nat. Hazards Earth Syst. Sci. 14, 2265–2287. doi: 10.5194/nhess-14-2265-2014
Brewer, C. A. (2018). Available online at: http://www.colorbrewer.org (accessed November 15, 2019). doi: 10.5194/nhess-14-2265-2014
Cappello, A., Neri, M., Acocella, V., Gallo, G., Vicari, A., and Del Negro, C. (2012). Spatial vent opening probability map of Etna volcano (Sicily. Italy). Bull. Volcanol. 74, 2083–2094. doi: 10.1007/s00445-012-0647-4
Capra, L., Gavilanes-Ruiz, J. C., Bonasia, R., Saucedo-Giron, R., and Sulpizio, R. (2015). Re-assessing volcanic hazard zonation of Volcán de Colima, México. Nat. Hazards 76, 41–61. doi: 10.1007/s11069-014-1480-1
Cassidy, M., Manga, M., Cashman, K., and Bachmann, O. (2018). Controls on explosive-effusive volcanic eruption styles. Nat. Commun. 9, 1–16.
Charbonnier, S. J., and Gertisser, R. (2012). Evaluation of geophysical mass flow models using the 2006 block-and-ash flows of merapi volcano, java, indonesia: towards a short-term hazard assessment tool. J. Volcanol. Geotherm. Res. 231, 87–108. doi: 10.1016/j.jvolgeores.2012.02.015
Christophersen, A., Deligne, N. I., Hanea, A. M., Chardot, L., Fournier, N., and Aspinall, W. P. (2018). Bayesian Network modeling and expert elicitation for probabilistic eruption forecasting: pilot study for Whakaari/White Island, New Zealand. Front. Earth Sci. 6:211. doi: 10.3389/feart.2017.00211
Cioni, R., Bertagnini, A., Santacroce, R., and Andronico, D. (2008). Explosive activity and eruption scenarios at somma-vesuvius (Italy): towards a new classification scheme. J. Volcanol. Geotherm. Res. 178, 331–346. doi: 10.1016/j.jvolgeores.2008.04.024
Connor, C., Bebbington, M., and Marzocchi, W. (2015). “Probabilistic volcanic hazard assessment,” in The Encyclopedia Of Volcanoes, 2nd Edn, eds H. Sigurdsson, B. Houghton, S. McNutt, H. Rymer, and J. Stix (Cambridge: Academic Press), 897–910. doi: 10.1016/b978-0-12-385938-9.00051-1
Connor, C. B., and Hill, B. E. (1995). Three nonhomogeneous poisson models for the probability of basaltic volcanism: application to the yucca mountain region, nevada. J. Geophys. Res. Solid Earth 100, 10107–10125. doi: 10.1029/95jb01055
Connor, C. B., Hill, B. E., Winfrey, B., Franklin, N. M., and Femina, P. C. (2001). Estimation of volcanic hazards from tephra fallout. Nat. Hazards Rev. 2, 33–42. doi: 10.1061/(asce)1527-6988(2001)2:1(33)
Connor, L. J., Connor, C. B., Meliksetian, K., and Savov, I. (2012). Probabilistic approach to modeling lava flow inundation: a lava flow hazard assessment for a nuclear facility in Armenia. J. Appl. Volcanol. 1:3.
Crosweller, H. S., Arora, B., Brown, S. K., Cottrell, E., Deligne, N. I., Guerrero, N. O., et al. (2012). Global database on large magnitude explosive volcanic eruptions (LaMEVE). J. Appl. Volcanol. 1:4.
Dalbey, K., Patra, A. K., Pitman, E. B., Bursik, M. I., and Sheridan, M. F. (2008). Input uncertainty propagation methods and hazard mapping of geophysical mass flows. J. Geophys. Res. Solid Earth 113: B05203. doi: 10.1029/2006JB004471
Dee, D. P., Uppala, S. M., Simmons, A. J., Berrisford, P., Poli, P., Kobayashi, S., et al. (2011). The ERA-Interim reanalysis: configuration and performance of the data assimilation system. Q. J. R. Meteorol. Soc. 137, 553–597.
Deino, A. L., Orsi, G., de Vita, S., and Piochi, M. (2004). The age of the Neapolitan Yellow Tuff caldera-forming eruption (Campi Flegrei caldera–Italy) assessed by 40Ar/39Ar dating method. J. Volcanol. Geotherm. Res. 133, 157–170. doi: 10.1016/s0377-0273(03)00396-2
Del Negro, C., Cappello, A., Neri, M., Bilotta, G., Hérault, A., and Ganci, G. (2013). Lava flow hazards at mount etna: constraints imposed by eruptive history and numerical simulations. Sci. Rep. 3:3493.
Deligne, N. I., Coles, S. G., and Sparks, R. S. J. (2010). Recurrence rates of large explosive volcanic eruptions. J. Geophys. Res. Solid Earth 115:B06203. doi: 10.1029/2009jb006554
Deng, F., Connor, C. B., Malservisi, R., Connor, L. J., White, J. T., Germa, A., et al. (2017). A geophysical model for the origin of volcano vent clusters in a colorado plateau volcanic field. J. Geophys. Res. Solid Earth 122, 8910–8924. doi: 10.1002/2017jb014434
Dzierma, Y., and Wehrmann, H. (2010). Statistical eruption forecast for the chilean southern volcanic zone: typical frequencies of volcanic eruptions as baseline for possibly enhanced activity following the large 2010 concepción earthquake. Nat. Hazards Earth Syst. Sci. 10, 2093–2108. doi: 10.5194/nhess-10-2093-2010
Dzierma, Y., and Wehrmann, H. (2014). Probabilities of future VEI ≥ 2 eruptions at the Central American Volcanic Arc: a statistical perspective based on the past centuries’ eruption record. Int. J. Earth Sci. 103, 2029–2042. doi: 10.1007/s00531-012-0803-2
Esposti Ongaro, T., Neri, A., Menconi, G., De’Michieli Vitturi, M., Marianelli, P., Cavazzoni, C., et al. (2008). Transient 3D numerical simulations of column collapse and pyroclastic density current scenarios at Vesuvius. J. Volcanol. Geotherm. Res. 178, 378–396. doi: 10.1016/j.jvolgeores.2008.06.036
Fedele, F. G., Giaccio, B., Isaia, R., and Orsi, G. (2003). “The campanian ignimbrite eruption, heinrich event 4, and palaeolithic change in europe: a high-resolution investigation,” in Volcanism and the Earth’s Atmosphere, eds A. Rob and C. Oppenheimer (Hoboken: John Wiley & Sons), 301–325. doi: 10.1029/139gm20
Fisher, R. V., Smith, A. L., and Roobol, M. J. (1980). Destruction of St. Pierre, Martinique, by ash-cloud surges, May 8 and 20, 1902. Geology 8, 472–476.
Garcia-Aristizabal, A., Marzocchi, W., and Fujita, E. (2012). A Brownian model for recurrent volcanic eruptions: an application to Miyakejima volcano (Japan). Bull. Volcanol. 74, 545–558. doi: 10.1007/s00445-011-0542-4
Geyer, A., and Marti, J. (2008). The new worldwide collapse caldera database (CCDB): a tool for studying and understanding caldera processes. J. Volcanol. Geotherm. Res. 175, 334–354. doi: 10.1016/j.jvolgeores.2008.03.017
Grilli, S. T., Tappin, D. R., Carey, S., Watt, S. F. L., Ward, S. N., Grilli, A. R., et al. (2019). Modelling of the tsunami from the December 22, 2018 lateral collapse of Anak Krakatau volcano in the sunda straits. Indonesia. Sci. Rep. 9, 1–13.
Gurioli, L., Sulpizio, R., Cioni, R., Sbrana, A., Santacroce, R., Luperini, W., et al. (2010). Pyroclastic flow hazard assessment at Somma–Vesuvius based on the geological record. Bull. Volcanol. 72, 1021–1038. doi: 10.1007/s00445-010-0379-2
Harrington, B. (2004–2005). Inkscape. Available online at: http://www.inkscape.org/ (accessed November 15, 2019). doi: 10.1007/s00445-010-0379-2
Hemming, V., Burgman, M. A., Hanea, A. M., McBride, M. F., and Wintle, B. C. (2018). A practical guide to structured expert elicitation using the IDEA protocol. Methods Ecol. Evol. 9, 169–180. doi: 10.1111/2041-210x.12857
Hincks, T. K., Komorowski, J.-C., Sparks, S. R., and Aspinall, W. P. (2014). Retrospective analysis of uncertain eruption precursors at La Soufri re volcano, Guadeloupe, 1975–77: volcanic hazard assessment using a Bayesian Belief Network approach. J. Appl. Volcanol. 3:3. doi: 10.1111/2041-210x.12857
Jaquet, O., Lantuéjoul, C., and Goto, J. (2017). Probabilistic estimation of long-term volcanic hazard under evolving tectonic conditions in a 1 Ma timeframe. J. Volcanol. Geotherm. Res. 345, 58–66. doi: 10.1016/j.jvolgeores.2017.07.010
Jenkins, S., Magill, C., McAneney, J., and Blong, R. (2012). Regional ash fall hazard I: a probabilistic assessment methodology. Bull. Volcanol. 74, 1699–1712. doi: 10.1007/s00445-012-0627-8
Jenkins, S. F., Goldstein, H., Bebbington, M. S., Sparks, R. S. J., and Koyaguchi, T. (2019). Forecasting explosion repose intervals with a non-parametric bayesian survival model: application to sakura-jima volcano, Japan. J. Volcanol. Geotherm. Res. 381, 44–56. doi: 10.1016/j.jvolgeores.2019.04.008
Kalnay, E., Kanamitsu, M., Kistler, R., Collins, W., Deaven, D., Gandin, L., et al. (1996). The NCEP/NCAR 40-year reanalysis project. Bull. Am. Meteorol. Soc. 77, 437–472.
Koller, D., and Friedman, N. (2009). Probabilistic Graphical Models: Principles And Techniques. Cambridge, MA: MIT press.
Le Corvec, N., Spörli, K. B., Rowland, J., and Lindsay, J. (2013). Spatial distribution and alignments of volcanic centers: clues to the formation of monogenetic volcanic fields. Earth Sci. Rev. 124, 96–114. doi: 10.1016/j.earscirev.2013.05.005
Loughlin, S. C., Vye-Brown, C., Sparks, R. S. J., Brown, S. K., Barclay, J., Calder, E., et al. (2015). “An introduction to global volcanic hazard and risk,” in Global Volcanic Hazards And Risk, eds S. C. Loughlin, R. S. J. Sparks, S. K. Brown, S. F. Jenkins, and C. Vye-Brown (Cambridge: Cambridge University Press), 1–80. doi: 10.1017/cbo9781316276273.003
Maeda, Y., Kumagai, H., Lacson, R., Figueroa, M. S., Yamashina, T., Ohkura, T., et al. (2015). A phreatic explosion model inferred from a very long period seismic event at Mayon Volcano. Philippines. J. Geophys. Res. Solid Earth 120, 226–242. doi: 10.1002/2014jb011440
Martin, A. J., Umeda, K., Connor, C. B., Weller, J. N., Zhao, D., and Takahashi, M. (2004). Modeling long-term volcanic hazards through Bayesian inference: an example from the Tohoku volcanic arc, Japan. J. Geophys. Res. Solid Earth 109: B10208. doi: 10.1029/2004JB003201
Marzocchi, W., and Bebbington, M. S. (2012). Probabilistic eruption forecasting at short and long time scales. Bull. Volcanol. 74, 1777–1805. doi: 10.1007/s00445-012-0633-x
Marzocchi, W., and Jordan, T. H. (2014). Testing for ontological errors in probabilistic forecasting models of natural systems. Proc. Natl. Acad. Sci. U.S.A. 111, 11973–11978. doi: 10.1073/pnas.1410183111
Marzocchi, W., Sandri, L., Gasparini, P., Newhall, C., and Boschi, E. (2004). Quantifying probabilities of volcanic events: the example of volcanic hazard at mount vesuvius. J. Geophys. Res. Solid Earth 109:B11201. doi: 10.1029/2004JB003155
Marzocchi, W., Sandri, L., and Selva, J. (2008). BET_EF: a probabilistic tool for long-and short-term eruption forecasting. Bull. Volcanol. 70, 623–632. doi: 10.1007/s00445-007-0157-y
Marzocchi, W., Sandri, L., and Selva, J. (2010). BET_VH: a probabilistic tool for long-term volcanic hazard assessment. Bull. Volcanol. 72, 705–716. doi: 10.1007/s00445-010-0357-8
Mastin, L. G., Guffanti, M., Servranckx, R., Webley, P., Barsotti, S., Dean, K., et al. (2009). A multidisciplinary effort to assign realistic source parameters to models of volcanic ash-cloud transport and dispersion during eruptions. J. Volcanol. Geotherm. Res. 186, 10–21. doi: 10.1016/j.jvolgeores.2009.01.008
Mead, S., and Magill, C. (2014). Determining change points in data completeness for the Holocene eruption record. Bull. Volcanol. 76:874.
Mead, S. R., and Magill, C. R. (2017). Probabilistic hazard modelling of rain-triggered lahars. J. Appl. Volcanol. 6:8.
Naismith, A. K., Watson, I. M., Escobar-Wolf, R., Chigna, G., Thomas, H., Coppola, D., et al. (2019). Eruption frequency patterns through time for the current (1999–2018) activity cycle at Volcán de Fuego derived from remote sensing data: Evidence for an accelerating cycle of explosive paroxysms and potential implications of eruptive activity. J. Volcanol. Geotherm. Res. 371, 206–219. doi: 10.1016/j.jvolgeores.2019.01.001
Neri, A., Bevilacqua, A., Esposti Ongaro, T., Isaia, R., Aspinall, W. P., Bisson, M., et al. (2015). Quantifying volcanic hazard at Campi Flegrei caldera (Italy) with uncertainty assessment: 2. Pyroclastic density current invasion maps. J. Geophys. Res. Solid Earth 120, 2330–2349. doi: 10.1002/2014jb011776
Newhall, C., and Hoblitt, R. (2002). Constructing event trees for volcanic crises. Bull. Volcanol. 64, 3–20. doi: 10.1007/s004450100173
Newhall, C. G. (1982). A Method For Estimating Intermediate- And Long-Term Risks From Volcanic Activity, With An Example From Mount st. Helens. Washington, DC: U.S. Geological Society.
Newhall, C. G., Costa, F., Ratdomopurbo, A., Venezky, D. Y., Widiwijayanti, C., Win, N. T. Z., et al. (2017). WOVOdat–an online, growing library of worldwide volcanic unrest. J. Volcanol. Geotherm. Res. 345, 184–199. doi: 10.1016/j.jvolgeores.2017.08.003
Newhall, C. G., and Pallister, J. S. (2015). “Using multiple data sets to populate probabilistic volcanic event trees,” in Volcanic Hazards, Risks, Disasters, eds P. Papale, J. C. Eichelberger, S. Nakada, S. Loughlin, and H. Yepes (Amsterdam: Elsevier), 203–232. doi: 10.1016/b978-0-12-396453-3.00008-3
Newhall, C. G., and Punongbayan, R. S. (1996). Fire and Mud: Eruptions And Lahars Of Mount Pinatubo, Philippines. Seattle: University of Washington Press.
Ogburn, S. E. (2012). FlowDat: Mass Flow Database. Available online at: https://vhub.org/groups/massflowdatabase (accessed November 24, 2019).
Ogburn, S. E., Berger, J., Calder, E. S., Lopes, D., Patra, A., Pitman, E. B., et al. (2016a). Pooling strength amongst limited datasets using hierarchical Bayesian analysis, with application to pyroclastic density current mobility metrics. Stat. Volcanol. 2:1. doi: 10.5038/2163-338x.2.1
Ogburn, S. E., Harpel, C. J., Pesicek, J. D., and Wellik, J. (2016b). The Eruption Forecasting Information System: Volcanic Eruption Forecasting Using Databases. Washington, DC: American Geophysical Union.
Ogburn, S. E., Loughlin, S. C., and Calder, E. S. (2015). The association of lava dome growth with major explosive activity (VEI ≥ 4): DomeHaz, a global dataset. Bull. Volcanol. 77:40.
Orsi, G., Di Vito, M. A., and Isaia, R. (2004). Volcanic hazard assessment at the restless campi flegrei caldera. Bull. Volcanol. 66, 514–530. doi: 10.1007/s00445-003-0336-4
Orsi, G., Di Vito, M. A., Selva, J., and Marzocchi, W. (2009). Long-term forecast of eruption style and size at Campi Flegrei caldera (Italy). Earth Planet. Sci. Lett. 287, 265–276. doi: 10.1016/j.epsl.2009.08.013
Pallister, J., Papale, P., Eichelberger, J., Newhall, C., Mandeville, C., Nakada, S., et al. (2019). Volcano observatory best practices (VOBP) workshops-a summary of findings and best-practice recommendations. J. Appl. Volcanol. 8:2.
Palma, J. L., Courtland, L., Charbonnier, S., Tortini, R., and Valentine, G. A. (2014). Vhub: a knowledge management system to facilitate online collaborative volcano modeling and research. J. Appl. Volcanol. 3:2.
Passarelli, L., and Brodsky, E. E. (2012). The correlation between run-up and repose times of volcanic eruptions. Geophys. J. Int. 188, 1025–1045. doi: 10.1111/j.1365-246x.2011.05298.x
Patra, A. K., Bauer, A. C., Nichita, C. C., Pitman, E. B., Sheridan, M. F., Bursik, M., et al. (2005). Parallel adaptive numerical simulation of dry avalanches over natural terrain. J. Volcanol. Geotherm. Res. 139, 1–21. doi: 10.1016/j.jvolgeores.2004.06.014
Rivalta, E., Corbi, F., Passarelli, L., Acocella, V., Davis, T., and Di Vito, M. A. (2019). Stress inversions to forecast magma pathways and eruptive vent location. Sci. Adv. 5:eaau9784. doi: 10.1126/sciadv.aau9784
Rougier, J., Sparks, R. S. J., Cashman, K. V., and Brown, S. K. (2018). The global magnitude–frequency relationship for large explosive volcanic eruptions. Earth Planet. Sci. Lett. 482, 621–629. doi: 10.1016/j.epsl.2017.11.015
Rougier, J., Sparks, S. R., and Cashman, K. V. (2016). Global recording rates for large eruptions. J. Appl. Volcanol. 5:11.
Rougier, J. C., and Beven, K. J. (2013). “Model limitations: the sources and implications of epistemic uncertainty,” in Risk and Uncertainty Assessessment for Natural Hazards, eds J. C. Rougier, R. S. J. Sparks, and L. J. Hill (Cambridge: Cambridge University Press), 40–63. doi: 10.1017/cbo9781139047562.004
Rouwet, D., Constantinescu, R., and Sandri, L. (2017). “Deterministic versus probabilistic volcano monitoring: not ‘or’ but ‘and’. volcanic unrest,” in Advances in Volcanology, eds J. Gottsmann, J. Neuberg, and B. Scheu (Cham: Springer), 35–46. doi: 10.1007/11157_2017_8
Runge, M. G., Bebbington, M. S., Cronin, S. J., Lindsay, J. M., and Moufti, M. R. (2016). Integrating geological and geophysical data to improve probabilistic hazard forecasting of Arabian Shield volcanism. J. Volcanol. Geotherm. Res. 311, 41–59. doi: 10.1016/j.jvolgeores.2016.01.007
Rutarindwa, R., Spiller, E. T., Bevilacqua, A., Bursik, M. I., and Patra, A. K. (2019). Dynamic probabilistic hazard mapping in the long valley volcanic region CA: integrating vent opening maps and statistical surrogates of physical models of pyroclastic density currents. J. Geophys. Res. Solid Earth. 124, 9600–9621. doi: 10.1029/2019jb017352
Sandri, L., Costa, A., Selva, J., Tonini, R., Macedonio, G., Folch, A., et al. (2016). Beyond eruptive scenarios: assessing tephra fallout hazard from Neapolitan volcanoes. Sci. Rep. 6:24271.
Sandri, L., Jolly, G., Lindsay, J., Howe, T., and Marzocchi, W. (2012). Combining long-and short-term probabilistic volcanic hazard assessment with cost-benefit analysis to support decision making in a volcanic crisis from the Auckland Volcanic Field, New Zealand. Bull. Volcanol. 74, 705–723. doi: 10.1007/s00445-011-0556-y
Sandri, L., Thouret, J.-C., Constantinescu, R., Biass, S., and Tonini, R. (2014). Long-term multi-hazard assessment for El Misti volcano (Peru). Bull. Volcanol. 76, 1–26.
Sandri, L., Tierz, P., Costa, A., and Marzocchi, W. (2018). Probabilistic hazard from pyroclastic density currents in the neapolitan Area (Southern Italy). J. Geophys. Res. Solid Earth 123:890. doi: 10.1002/2017JB014890
Selva, J., Costa, A., Sandri, L., Macedonio, G., and Marzocchi, W. (2014). Probabilistic short-term volcanic hazard in phases of unrest: a case study for tephra fallout. J. Geophys. Res. Solid Earth 119, 8805–8826. doi: 10.1002/2014jb011252
Selva, J., Marzocchi, W., Papale, P., and Sandri, L. (2012a). Operational eruption forecasting at high-risk volcanoes: the case of Campi Flegrei, Naples. J. Appl. Volcanol. 1:5.
Selva, J., Orsi, G., Di Vito, M. A., Marzocchi, W., and Sandri, L. (2012b). Probability hazard map for future vent opening at the campi flegrei caldera, Italy. Bull. Volcanol. 74, 497–510. doi: 10.1007/s00445-011-0528-2
Sheldrake, T. (2014). Long-term forecasting of eruption hazards: a hierarchical approach to merge analogous eruptive histories. J. Volcanol. Geotherm. Res. 286, 15–23. doi: 10.1016/j.jvolgeores.2014.08.021
Sheldrake, T., and Caricchi, L. (2017). Regional variability in the frequency and magnitude of large explosive volcanic eruptions. Geology 45, 111–114. doi: 10.1130/g38372.1
Sheldrake, T. E., Sparks, R. S. J., Cashman, K. V., Wadge, G., and Aspinall, W. P. (2016). Similarities and differences in the historical records of lava dome-building volcanoes: Implications for understanding magmatic processes and eruption forecasting. Earth-Science Rev. 160, 240–263. doi: 10.1016/J.EARSCIREV.2016.07.013
Siebert, L., and Simkin, T. (2002). Volcanoes of the World: an Illustrated Catalog of Holocene Volcanoes and their Eruptions. Smithsonian Inst, Global Volcanism Prog Digital Inf Ser GVP-3. Available online at: http://www.volcano.si.edu (accessed May 8, 2018).
Siebert, L., Simkin, T., and Kimberly, P. (2010). Volcanoes of The World. Berkeley: University of California Press.
Sobradelo, R., Bartolini, S., and Martí, J. (2014). HASSET: a probability event tree tool to evaluate future volcanic scenarios using Bayesian inference. Bull. Volcanol. 76:770.
Sobradelo, R., and Martí, J. (2010). Bayesian event tree for long-term volcanic hazard assessment: application to Teide-Pico Viejo stratovolcanoes, Tenerife, Canary Islands. J. Geophys. Res. Solid Earth 115:B05206. doi: 10.1029/2009JB006566
Sobradelo, R., Martí, J., Mendoza-Rosas, A. T., and Gómez, G. (2011). Volcanic hazard assessment for the Canary Islands (Spain) using extreme value theory. Nat. Hazards Earth Syst. Sci. 11, 2741–2753. doi: 10.5194/nhess-11-2741-2011
Spiller, E. T., Bayarri, M. J., Berger, J. O., Calder, E. S., Patra, A. K., Pitman, E. B., et al. (2014). Automating emulator construction for geophysical hazard maps. SIAM/ASA J. Uncertain. Quantif. 2, 126–152. doi: 10.1137/120899285
Stefanescu, E. R., Bursik, M., Cordoba, G., Dalbey, K., Jones, M. D., Patra, A. K., et al. (2012a). Digital elevation model uncertainty and hazard analysis using a geophysical flow model. Proc. R. Soc. Math. Phys. Eng. Sci. 468, 1543–1563. doi: 10.1098/rspa.2011.0711
Stefanescu, E. R., Bursik, M., and Patra, A. K. (2012b). Effect of digital elevation model on Mohr-Coulomb geophysical flow model output. Nat. Hazards 62, 635–656. doi: 10.1007/s11069-012-0103-y
Tadini, A., Bevilacqua, A., Neri, A., Cioni, R., Aspinall, W. P., Bisson, M., et al. (2017). Assessing future vent opening locations at the Somma-Vesuvio volcanic complex: 2. Probability maps of the caldera for a future Plinian/sub-Plinian event with uncertainty quantification. J. Geophys. Res. Solid Earth 122, 4357–4376. doi: 10.1002/2016jb013860
Takarada, S. (2017). The volcanic hazards assessment support system for the online hazard assessment and risk mitigation of quaternary volcanoes in the world. Front. Earth Sci. 5:102. doi: 10.3389/feart.2017.00102
Thompson, M. A., Lindsay, J. M., Sandri, L., Biass, S., Bonadonna, C., Jolly, G., et al. (2015). Exploring the influence of vent location and eruption style on tephra fall hazard from the Okataina Volcanic Centre. New Zeal. Bull. Volcanol. 77:38.
Tierz, P., Loughlin, S. C., and Calder, E. S. (2019). VOLCANS: an objective, structured and reproducible method for identifying sets of analogue volcanoes. Bull. Volcanol. 81:76. doi: 10.1007/s00445-019-1336-3
Tierz, P., Sandri, L., Costa, A., Sulpizio, R., Zaccarelli, L., Vito, M. A. D., et al. (2016a). “Uncertainty assessment of pyroclastic density currents at mount vesuvius (italy) simulated through the energy cone model,” in Natural Hazard Uncertainty Assessment: Modeling and Decision Support, eds P. Webley, K. Riley, and M. P. Thompson (Hoboken: John Wiley & Sons), 125–145. doi: 10.1002/9781119028116.ch9
Tierz, P., Sandri, L., Costa, A., Zaccarelli, L., Di Vito, M. A., Sulpizio, R., et al. (2016b). Suitability of energy cone for probabilistic volcanic hazard assessment: validation tests at Somma-Vesuvius and campi flegrei (Italy). Bull. Volcanol. 78:1073. doi: 10.1007/s00445-016-1073-9
Tierz, P., Stefanescu, E. R., Sandri, L., Sulpizio, R., Valentine, G. A., Marzocchi, W., et al. (2018). Towards quantitative volcanic risk of pyroclastic density currents: probabilistic hazard curves and maps around Somma-Vesuvius (Italy). J. Geophys. Res. Solid Earth 123:383. doi: 10.1029/2017JB015383
Tierz, P., Woodhouse, M. J., Phillips, J. C., Sandri, L., Selva, J., Marzocchi, W., et al. (2017). A framework for probabilistic multi-hazard assessment of rain-triggered lahars using bayesian belief networks. Front. Earth Sci. 5:73. doi: 10.3389/feart.2017.00073
Tonini, R., Sandri, L., and Thompson, M. A. (2015). PyBetVH: a python tool for probabilistic volcanic hazard assessment and for generation of Bayesian hazard curves and maps. Comput. Geosci. 79, 38–46. doi: 10.1016/j.cageo.2015.02.017
Trolese, M., Cerminara, M., Ongaro, T. E., and Giordano, G. (2019). The footprint of column collapse regimes on pyroclastic flow temperatures and plume heights. Nat. Commun. 10:2476.
Volentik, A. C. M., Connor, C. B., Connor, L. J., and Bonadonna, C. (2009). “Aspects of volcanic hazard assessment for the bataan nuclear power plant, luzon Peninsula, Philippines,” in Volcanic and Tectonic Hazard Assessment for Nuclear Facilities, eds C. Connor, N. Chapman, and L. Connor (New York, NY: Cambridge University Press), 229–256. doi: 10.1017/cbo9780511635380.010
Vye-Brown, C., Sparks, R. S. J., Lewi, E., Mewa, G., Asrat, A., Loughlin, S. C., et al. (2016). Ethiopian volcanic hazards: a changing research landscape. Geol. Soc. Lond. Spec. Publ. 420, 355–365. doi: 10.1144/sp420.16
Wadge, G., Young, P. A. V., and McKendrick, I. J. (1994). Mapping lava flow hazards using computer simulation. J. Geophys. Res. Solid Earth 99, 489–504. doi: 10.1029/93jb01561
Whelley, P. L., Newhall, C. G., and Bradley, K. E. (2015). The frequency of explosive volcanic eruptions in Southeast Asia. Bull. Volcanol. 77:1.
Williams, R., Rowley, P., and Garthwaite, M. C. (2019). Reconstructing the Anak Krakatau flank collapse that caused the December 2018 Indonesian tsunami. Geology 47, 973–976. doi: 10.1130/g46517.1
Wolpert, R. L., Spiller, E. T., and Calder, E. S. (2018). Dynamic statistical models for pyroclastic density current generation at Soufrière Hills Volcano. Front. Earth Sci. 6:55. doi: 10.3389/feart.2018.00055
Keywords: probabilistic volcanic hazard assessment, uncertainty quantification, data scarcity, open data, global databases
Citation: Tierz P (2020) Long-Term Probabilistic Volcanic Hazard Assessment Using Open and Non-open Data: Observations and Current Issues. Front. Earth Sci. 8:257. doi: 10.3389/feart.2020.00257
Received: 06 December 2019; Accepted: 09 June 2020;
Published: 23 July 2020.
Edited by:
Fidel Costa, Nanyang Technological University, SingaporeReviewed by:
Mark Bebbington, Massey University, New ZealandChris Newhall, Mirisbiris Garden and Nature Center, Philippines
British Geological Survey © URKI 2020. This is an open-access article distributed under the terms of the Creative Commons Attribution License (CC BY). The use, distribution or reproduction in other forums is permitted, provided the original author(s) and the copyright owner(s) are credited and that the original publication in this journal is cited, in accordance with accepted academic practice. No use, distribution or reproduction is permitted which does not comply with these terms.
*Correspondence: Pablo Tierz, cGFibG9AYmdzLmFjLnVr
†ORCID: Pablo Tierz, orcid.org/0000-0001-8889-9900