- 1European Plate Observing System, ERIC-Istituto Nazionale di Geofisica e Vulcanologia, Rome, Italy
- 2Istituto Nazionale di Geofisica e Vulcanologia, Seismology and Tectonophysics, Rome, Italy
FAIR principles have become reference criteria for promoting and evaluating openness of scientific data and for improving datasets Findability, Accessibility, Interoperability, and Reusability. This also applies to Research Infrastructures (RIs) in the solid Earth domain committed to provide access to seismological data, ground deformations inferred from terrestrial, and satellite observations, geological maps, and laboratory experiments. Such RIs have been indeed committed for a long time, well before the appearance of FAIR principles, to engage scientific communities involved in data collection, standardization, and quality control as well as in implementing metadata and services for qualification, storage and accessibility. By addressing open science and managing scientific data, they are working to adopt FAIR principles, thus having the onerous task of turning these principles into practices. In this work we argue that although FAIR principles have the merit of creating a common background of knowledge to engage communities in providing data in a standard way thus easing interoperability and data sharing, in order to make the adoption of FAIR principles less onerous there is an urgent need of clear models, reference architectures and technical guidelines which can support RI implementers in the realization of FAIR data provision systems. We therefore discuss the state of the art of FAIR principles ecosystem and open new perspectives by discussing a four-stages roadmap that reorganizes FAIR principles in a way that better fits to the approach of RI implementers, and a FAIR adoption process that relates FAIR principles to technologies for their implementation.
Introduction
FAIR (Findable, Accessible, Interoperable, Reusable) data principles (Wilkinson et al., 2016) are gaining consensus within scientific communities, fostered by the participation in designing pan-European initiatives such as the European Open Science Cloud (EOSC) (EOSC, 2018), where they are used as driving concepts to support data interoperability among standardized repositories compliant to a shared set of requirements (Mons et al., 2017).
FAIR principles were discussed and launched at a FORCE111 workshop in 2014, enabling the publication of a first paper with their detailed definition (Wilkinson et al., 2016).
EOSC initiative subsequently emphasized how these principles just provide guidelines, without including any technical requirement nor explicitly suggesting technologies for their adoption (Mons et al., 2017), which may lead to ambiguities regarding their implementation; this led proponents already involved in their definition to establish a FAIR Metrics group for measuring FAIRness of data (Wilkinson et al., 2018). Such an approach, however, does not consider the impact of FAIRness on the full research data lifecycle and on the related development activities (Boeckhout et al., 2018).
On the same page, initiatives have been undertaken to provide support for FAIR principles adoption: FAIRsharing2 provides a platform for enhancing the discoverability of resources even outside of a community (Sansone et al., 2019); GO-FAIR3 offers supporting materials and tools (Schultes et al., 2018); Enabling FAIR data project4 promotes FAIR principles implementation with the document “Commitment Statement to Enabling FAIR Data in the Earth, Space, and Environmental Sciences” (E. F. D. Community, 2018). In parallel, further work is carried out by the Research Data Alliance (RDA5) “FAIR Data Maturity Model WG Case Statement” Interest Group for establishing core criteria to assess the implementation level of FAIR data principles (FAIR Data Maturity Model WG, 2019).
In this framework, Environmental Research Infrastructures (ENVRI) have set up a joint 4 years program, ENVRI-FAIR (Petzold and Glaves, 2018; Petzold et al., 2020), with the overarching goal of promoting findability, accessibility, interoperability, and reusability of digital assets through the development of FAIR compliant common policies and technical solutions following expectations of the authorities promoting FAIRness (Cocco et al., 2019).
Similarly, several scientific communities are attempting to fill existing gaps between FAIR principles and viable practices for FAIRness by exploring pilot implementations as in life science case (Wilkinson et al., 2017) that propose standards-based reference technologies. Furthermore, an action plan for FAIR adoption has been presented by the European Commission Expert Group with the “Turning FAIR data into reality” report (Collins et al., 2018).
Relying on the experience matured in the framework of the European Plate Observing System (EPOS) (www.epos-eu.org) RI (Bailo et al., 2016), we argue that the aforementioned search for technological solutions need to be further elaborated. Already existing RIs, in particular those involved in the EOSC initiative (EOSC, 2018), would incredibly benefit from a clear and technically consistent roadmap to make technologies already in place better adhere to FAIR principles.
Perspectives on Adoption of Fair Principles
Perspective view and expertise related to technical implementation of the FAIR principles matured in the context of EPOS RI, which engages 10 different scientific communities in the solid Earth domain and integrates more than 250 research infrastructures and data providers.
On the basis of experience and know-how in the EPOS community of practitioners, experts, and engineers, a common approach was observed, which is described by the re-organization of FAIR principles into a four-stages roadmap. Such roadmap is part of a more general FAIR adoption process that may enable potentially any RI in the solid Earth domain and beyond to build FAIR Research Infrastructures. List of abbreviations to ease readability of the text is reported in the Supplementary Material.
Four-Stages Roadmap Approach
The driving concept is that FAIR principles are not easily understood by every domain scientist, data practitioner and IT specialist (here summarized as RI implementers as they all contribute to the technical construction of an RI), because such professionals have a specific mindset when it comes to building a system for data stewardship in the scientific domain.
The roadmap hence reflects the pragmatic approach undertaken by RI implementers and considers four different stages of development (Figure 1A), each of them referencing detailed FAIR principles [as described in (Wilkinson et al., 2016)]: (a) data stage, (b) metadata stage, (c) access stage, and (d) use stage.
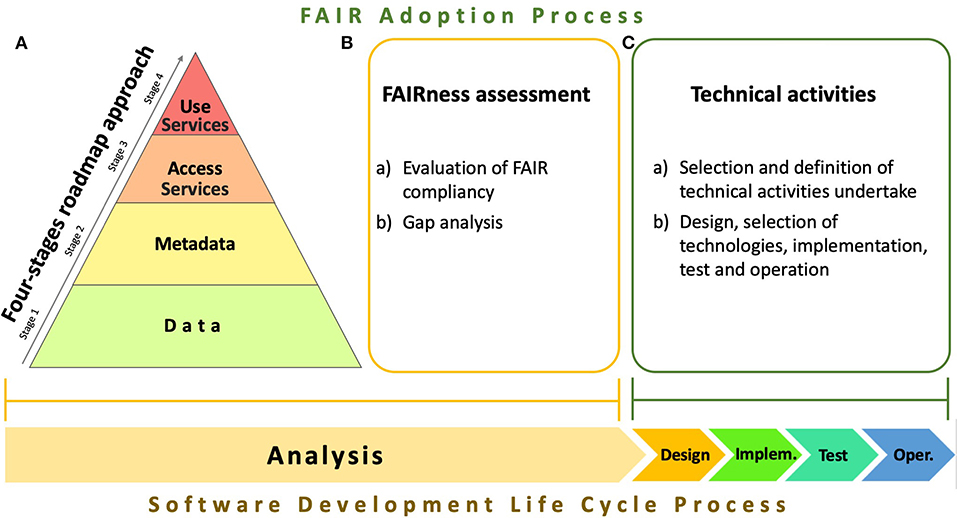
Figure 1. FAIR adoption and Software Development Life Cycle (SLDC) processes are shown in parallel and correspondences between the two are highlighted. FAIR adoption is a three-step process: the first step (A) is represented by the four-stages roadmap (Bailo, 2019), which reorganizes detailed FAIR principles (Wilkinson et al., 2016) according to the mindset of RI implementers; the second step (B) is devoted to the evaluation of re-organized FAIR principles at each stage, and to a gap analysis to make emerge the priority by which FAIR principles at each stage need to be addressed; the third step (C) is devoted, on the basis of the gap analysis, to the selection of technical activities to undertake, and then to actual implementation. Software Development Life Cycle, described in section FAIR adoption process, is here compared to the FAIR adoption process. What emerges is that step one and two of the FAIR adoption process are carried out in the Analysis Phase in the SDLC; indeed, FAIR principles are elicited as requirement and gap analysis in order to understand which ones will be implemented at each “round” of implementation is performed. Phases from design to operation are executed in the “technical activities” step of the FAIR adoption process.
In the following paragraphs, references to detailed FAIR principles will be done by using letters of the acronym F1, F2, etc. for Findability detailed principles, A1, A1.1, etc. for Accessibility detailed principles and so on, as defined in Box 2 of Wilkinson et al. (2016).
The first concern of RI implementers is indeed the data, which constitutes the main business in the scientific domain and whose collection process is often hard, effort-consuming and resulting from ingenious and complex experimental techniques.
The first stage (S1) therefore considers datasets, data products, research objects and any other resource to which we refer to as data in this context. Wherever data come from—laboratory or field experiment, selection or collection, monitoring sensors, whether organized in networks, or standalone, etc.—they usually need to be harmonized when provided in heterogeneous formats in order to ensure interoperability. As a consequence, data providers in a certain domain should agree on community standards (R1.3), broadly applicable language and formats for representation of knowledge (I1, I2, I3); data should then be uniquely and globally identified using persistent identifier (PID) (F1), and indexed in a searchable resource (F4), taking also into account licensing aspects to guarantee intellectual property rights and appropriate credit (R1.1).
Once data is properly collected and managed, RI implementers are concerned with attaching information to facilitate findability encompassing searchability and contextualization. The second stage (S2) thus concerns metadata implementation, which allows proper description of data—if rich enough (F2, I3)—and supports provenance recording (R1.2) and license information (R1.1). Usage of standards (R1.3), based on existing vocabularies (I2) and formal languages (I1) also facilitates the process. Similarly to S1, also metadata needs to be referenced by a unique persistent identifier (F1, F3) and to be indexed in searchable resources (F4). Metadata are supposed to be persistent and accessible even if data no longer exist (A2).
Well-described and contextualized data need to be accessible. Hence, the third stage (S3) deals with the provision of services that allow both human and machine access to data and metadata. They should include search functionalities (F4) and enable access (e.g., download) also through persistent identifiers (F1). Such services need to use standardized communication protocols (A1.1) and must also support authentication and authorization mechanisms if applicable (A1.2).
Access to data is not, however, the end of the scientific process that aims at producing meaningful and interpretable data. This is implicitly assumed by the FAIR principles that include reusability (and lately reproducibility), but also apparent in several Data Lifecycle models where other steps related to the usage of data are envisaged (Ball, 2012). Such steps are concerned with all functionalities that go beyond data access, for instance data analysis and processing.
FAIR RIs and data stewardship systems should then address a fourth stage (S4) concerned with services that make use of data (S1) and metadata (S2) FAIRly accessed (S3) and produce new data products as output. Such a stage guarantees that analysis performed on FAIR data still produce FAIR data as output. A common use case is indeed represented by services that use machine-readable and machine-actionable data to perform data analysis, visualization and processing, and produce what is often referred to as “data products” also in other environmental RIs.
FAIR Adoption Process
RI implementers setting up or upgrading an existing RI usually follow a system development life-cycle (SDLC) process (Blanchard, 2004). In the current work, an SDLC inspired by the waterfall development model was used, which encompasses the following steps: (a) analysis, including use cases and requirements collection; (b) design, including architecture design, and identification of architectural components matching requirements, (c) implementation, through software developments and adoption of suitable technologies, (d) test, (e) operation and maintenance.
Although FAIR principles claim to be technically non-prescriptive (Mons et al., 2017), they need to intersect and be part of SDLC since its early phases, leading to three main questions:
i where FAIR principles have to be considered in the SDLC
ii what FAIR detailed principles should be implemented first, and what would be a correct sequence and time-line
iii how FAIR principles should be technically addressed
FAIR principles define what the system should provide and how it should be provided, they can therefore be considered as requirements in the SDLC and should be taken into account during the analysis phase.
However, how to manage contexts where a Research Infrastructure already exists and needs to be upgraded to be compliant to FAIR principles is still an open question. This is indeed a common status for many RIs, for instance all those in the ENVRI-FAIR initiative.
The reorganization of the FAIR detailed principles into a four-stages roadmap together with potential technical activities to implement them at each of the stages of the roadmap (Table 1A) provide a perspective to answer to this latter question and also to questions (ii) and (iii).
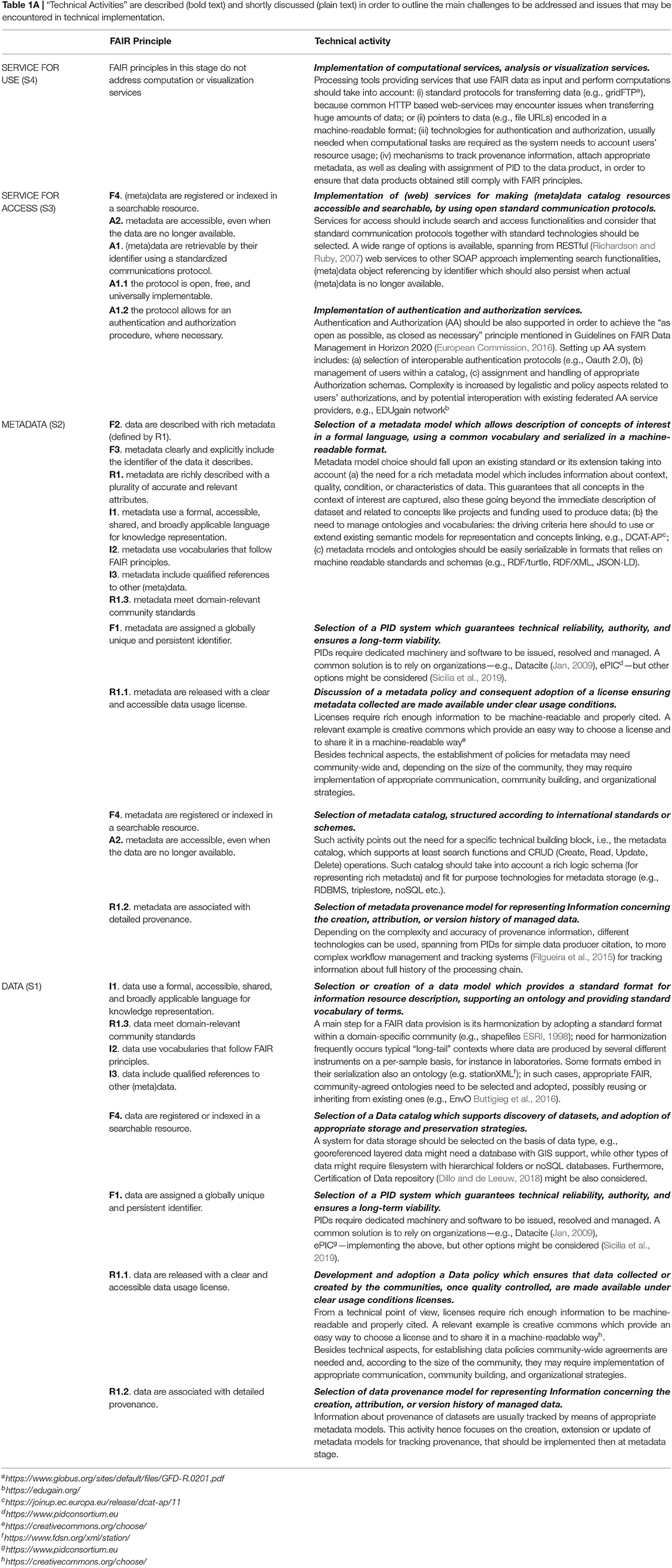
Table 1. At each stage of the roadmap starting from the bottom (Data Sheet 1) to the top stage (Services For Use, S4), specific FAIR principles (Wilkinson et al., 2016) related to each layer, together with corresponding technical activities, are listed.
Although the four-stages roadmap makes it easier for RI implementers to understand FAIR principles within a framework they are familiar with, a clear process is required for going from the roadmap and principles to actual technical implementations fulfilling FAIR principles.
A three-step FAIR adoption process can be used, and each step is detailed as follows:
1. Per-Stage Implementation Approach. For Research Infrastructures that are built from scratch, the pyramidal roadmap is likely to be followed in a sequential way starting from the bottom data layer (S1) to the top services for usage layer (S4), with a potential exception for processing services producing FAIR data products in output using FAIR dataset as input, as discussed in the next section. Indeed it wouldn't make sense to consider, for example, services for accessing data (S3) when data is not defined, stored, and not described by a (rich) metadata standard. For existing RIs that need to be enhanced to reach an higher level of FAIRness, the four layers can be considered also in a non-sequential way, as the work can be carried out only where needed. So, for instance, if a minimal level of FAIRness exist at data stage (e.g., dataset are at least stored in an accessible repository) and at metadata stage (e.g., simple metadata available for stored datasets), it is then possible to only improve services for accessing such assets (S3).
2. FAIRness Assessment. In order to enhance a specific stage, its compliance to the re-organized detailed FAIR principles needs to be evaluated and a gap-analysis performed to define technical activities addressing criticalities emerged during the evaluation. Currently, several initiatives are devoted to evaluating compliancy to FAIR principles6, 7, 8 (Wilkinson et al., 2019); as consensus about one or more evaluation methods is reached within the scientific community, they might be adopted as canonical evaluations.
3. Technical Activities Definition. On the basis of the FAIRness evaluation results and the emerged gaps, the actual technical activities needed to fulfill a specific FAIR principle can finally be defined and executed in the domain specific context by following steps from design (b) to operation (e) of the SLDC.
In this three-step FAIR adoption process the SDLC is not confined to the technical implementation step (3), as its analysis phase encompasses also the Definition of the stages to implement (1) and FAIRness evaluation (2) steps, which is where FAIR detailed principles are defined and elicited as requirements (Figure 1).
In the following paragraph we describe the technical activities required for implementing FAIR requirements at each stage.
Technical Implementation
FAIR principles, in their definition, implicitly refer to the adoption of certain technological solutions, for instance web services or PIDs. Existing technical-related resources (Collins et al., 2018) usually mention these elements as best practices, avoiding any mandatory guideline. However, a clear process for the implementation of FAIR principles, like the three-step FAIR adoption process described in the previous section, requires an explicit definition of technical activities.
By technical activities in this context we refer to: (a) tasks with a clear technical outcome (e.g., community agreements about data standards, R1.3); (b) activities for construction of architectural building blocks of a system, including software or hardware, needed to provide features defined by FAIR requirements (e.g., metadata catalog building block for rich metadata storage - F2); (c) activities aiming at defining conceptual models and paradigms (e.g., adoption or extension of existing ontologies for knowledge representation—I1). We do not refer nor propose any specific software or tool, since they would change as technology evolves.
Table 1A provides a compact overview at each stage (column 1) of technical activities (column 3) RI implementers need to undertake in order to fulfill specific FAIR principles (column 2). Such table, by establish a correspondence between specific FAIR principles and technical activities at each stage, represents a pragmatic approach implementing the three-step FAIR adoption process.
Fair Adoption Example
In this section we describe the FAIR adoption process—including the four-stages roadmap—described in the previous section, in the context of the EPOS pan-European Research Infrastructure.
European Plate Observing System
The European Plate Observing System (EPOS) is building a pan-European research infrastructure for solid-Earth sciences. Its Preparatory Phase (PP), funded under the European Commission's FP7 Work Programme, ran from 2010 to 2014 and reached the ambitious goal of creating the conditions for the integration of existing and future national and international research infrastructures (RIs) in Europe with the final goal of improving access to data, products, and services. The technical architecture (Jeffery et al., 2018) was built following a co-design approach, with a continuous interaction among communities stakeholders, data practitioners, scientists, and engineers who shared their skills and experiences.
EPOS also undertook an Implementation Phase from 2014 to September 2019, where the implementation of a pre-operational Integrated Core Services (ICS) system and of interoperable Thematic Core Services (TCS) were carried out, and the technical architecture confirmed.
Such an architecture is composed by three fundamental elements:
• Thematic Core Services (TCS), representing datasets and services provided by the domain specific communities. At such community level, EPOS has promoted and stimulated the harmonization of data management, access methods and policies, as well as services (e.g., processing, visualization) and resource provisioning by: (1) Fostering the creation of new European-wide thematic hubs; and (2) Supporting existing organizations (e.g., ORFEUS29 for seismology).
• Integrated Core Services-Centralized (ICS-C), representing the novel system which integrates resources provided by the TCS. Interoperability between ICS and TCS was implemented by activities also envisaged by the four-stages roadmap in the FAIR adoption process, i.e., metadata related activities.
• Integrated Core Services-Distributed (ICS-D), which constitute the distributed part of the ICS, devoted to computational or visualization tasks, designed as services offered by e-Infrastructure providers and resource providers that—under clear procurement policies or SLAs—make resources available (e.g., HPC, HTC, data storage and data transport).
FAIR Implementation in EPOS
As many RI in the environmental domain, EPOS relies on already existing data providers, and as such contains peculiarities that need to be remarked, before addressing actual implementation activities (Table 1A).
The first remark is that technical implementation of FAIR principles in EPOS required consistent non-technical efforts, i.e., keeping the full community together by adopting community building actions, communicating results, manage legalistic, and governance aspects. The actual magnitude of these efforts needs to be taken into account as it influences the technical work, and make the entire FAIR adoption process difficult to fit to the timelines envisaged by some policy makers, for instance at EOSC level9. The FAIR adoption process in EPOS started years ago, is cyclically being refined and the experience suggests that this will be an iterative activity still going on in the medium term in the future.
Secondly, in EPOS the process of creating the conditions for interoperability among existing RIs has actually started in 2010, long before the FAIR principles were made explicit by FORCE11 (Wilkinson et al., 2016). This entails two considerations: (a) EPOS architecture was FAIR-compliant since its conception because some of its driving aspects are key concepts for FAIR principles as well, i.e., metadata and ontologies, identifiers, technological interoperability; (b) the methodology was applied to an existing research infrastructure, and in the wider Environmental RIs landscape this is often the case in the experience of the authors.
The third remark is that the evaluation step in the FAIR adoption process was carried out on the basis of IT expertise and skills of EPOS RI implementers, being rigorous methods to evaluate and making the gap analysis not yet available before 2016 and currently still under discussion (Wilkinson et al., 2019). Importantly, such FAIR evaluation methods developed at different levels and in different continents, are taking advantage of domain specific RIs like EPOS and RIs clusters like ENVRI-FAIR, when it comes to have an harmonized application, test, and feedback of the proposed evaluation methods and questions, as in the case of the work being carried out in ENVRI-FAIR WP5 and WP7.
Details about technological activities carried out in EPOS are reported in Table 1B.
Discussion
Perspective on FAIR Principles
Nowadays, FAIR principles are largely accepted by a wide stakeholders range, all over Europe (and beyond); the acronym well-reflects the concept of “establishing equally a common technical background” for all those involved in data provision; also, it has the merit of making technical concepts like “interoperability” understandable by non-technical audience; FAIR concepts discussions are cross-disciplinary and applicable also to non-technical domains [see “FAIR policies” discussions (Boeckhout et al., 2018)]; finally, being it a driving concept in European initiatives like EOSC, it has rapidly become a reference for all those RIs whose sustainability relies on European funding.
However, the road from principles to real FAIR RIs is still long: at now many initiatives focus primarily on the establishment of criteria for assessing “FAIRness” of data stewardship systems and then on complementary guidelines (Collins et al., 2018) or support actions (GO-FAIR) working on best practices and not yet attempting to formalize technical references architectures.
The current work aims at making a step forward on the “principles-to-reality” track, by emphasizing the need for technical guidelines and by proposing a four-stages roadmap related to technological solutions that ease real implementation.
The roadmap in Figure 1, rather than prescribing a sequential temporal approach, aims at capturing the mindset and approach of RI implementers. The roadmap also demonstrates that an approach that follows sequentially the letters of the FAIR acronym (i.e., implementing first technologies for Findability, secondly technologies for Accessibility, and so on), is not technically viable and does not fit with development practices within RIs.
The roadmap is part of a FAIR adoption process which also include an SDLC and reflects the real workflow RI implementers use in the actual technical work. The SDLC discussed here is simple but efficient and commonly used; others may be used to meet more advanced implementational techniques, and the FAIR adoption process would still work as an implementation guideline method.
Importantly, the FAIR adoption process can be applied also to existing RIs, which in the current FAIR landscape (for instance in the EOSC) represent the majority.
With the conceptual solutions proposed (Table 1A) and their actual technical implementation in EPOS (Table 1B), the authors want to foster efforts in providing clear technical guidelines for building or upgrading existing RIs data systems. In perspective, an agreed common view of the architectural technical building blocks may also facilitate the establishment of metrics for FAIR assessment of systems, not only of the data they steward.
Future Work
The fourth stage, which deals with services taking FAIR data as input to produce FAIR data products in output, paves the way for future work related to the extension of FAIR principles also to processing services, which seem not to have a habitat yet in the FAIR environment (definition, guidelines, etc.) although they are a key element for re-usability based on interoperability. Additional consideration on provenance aspects and on what building blocks are needed to produce FAIR provenance records should be done.
In the EPOS case study we observed that the common approach to RI system development influences RI architectures that turn out to be very similar across the EPOS Thematic Communities, in terms of system components. Hence, additional effort in the definition of FAIR reference architectures would make any RI, especially those providing access to similar resources, benefit of a pool of reference architectural building blocks and technical solutions that would ease implementation of FAIR compliant systems.
Author Contributions
DB developed the main idea and wrote the manuscript. RP provided support in manuscript writing and contributed to the definition of technological solutions. MS, RR, and VV participated to EPOS team technical work and discussions that paved the way for roadmap elaboration, produced figures, and tables and reviewed the text. MC overviewed the manuscript and as EPOS coordinator, managed strategic FAIR RI activities in the EPOS framework.
Funding
This research has been supported by EPOS-IP EU project (No. 676564). VRE4EIC Grant agreement ID: 676247. ENVRI-FAIR Grant agreement ID 824068.
Conflict of Interest
The authors declare that the research was conducted in the absence of any commercial or financial relationships that could be construed as a potential conflict of interest.
Acknowledgments
We would like to thank the EPOSWP6–WP7 Technical Team.
Supplementary Material
The Supplementary Material for this article can be found online at: https://www.frontiersin.org/articles/10.3389/feart.2020.00003/full#supplementary-material
Footnotes
4. ^http://www.copdess.org/enabling-fair-data-project/
5. ^https://www.rd-alliance.org/
6. ^http://blog.ukdataservice.ac.uk/fair-data-assessment-tool/
7. ^https://www.go-fair.org/2017/12/11/metrics-evaluation-fairness/ [http://aims.fao.org/activity/blog/put-fair-principles-practice-and-enjoy-your-data]
8. ^https://www.biorxiv.org/content/biorxiv/early/2018/09/25/418376.full.pdf
9. ^https://op.europa.eu/en/publication-detail/-/publication/78ae5276-ae8e-11e9-9d01-01aa75ed71a1
References
Bailo, D. (2019). Four-Stages FAIR Roadmap - FAIR “Pyramid,”. Zenodo. Available online at: http://doi.org/10.5281/zenodo.3299353
Bailo, D., and Jeffery, K. G. (2014). EPOS: a novel use of CERIF for data-intensive science. Proc. Comput. Sci. 33, 3–10. doi: 10.1016/j.procs.2014.06.002
Bailo, D., Jeffery, K. G., Spinuso, A., and Fiameni, G. (2016). “Interoperability oriented architecture: the approach of EPOS for solid Earth e-infrastructures,” in Proceedings of the 2015 IEEE 11th International Conference on e-Science, (Munich) 529–534. doi: 10.1109/eScience.2015.22
Ball, A. (2012). Review of Data Management Lifecycle Models, 14. Available online at: http://opus.bath.ac.uk/28587/1/redm1rep120110ab10.pdf. (accessed January 23, 2020).
Boeckhout, M., Zielhuis, G. A., and Bredenoord, A. L. (2018). The FAIR guiding principles for data stewardship: fair enough? Eur. J. Hum. Genet. 26, 931–936. doi: 10.1038/s41431-018-0160-0
Buttigieg, P. L., Pafilis, E., Lewis, S. E., Schildhauer, M. P., Walls, R. L., and Mungall, C. J. (2016). The environment ontology in 2016: bridging domains with increased scope, semantic density, and interoperation. J. Biomed. Semant. 7, 1–12. doi: 10.1186/s13326-016-0097-6
Cocco, M., Bailo, D., Paciello, R., Freda, C., and Jeffery, K. (2019). The FAIRness horizon: from principles to practice, the EPOS experience in solid Earth science. Geophys. Res. Abstr. 21:13137. Available online at: https://ui.adsabs.harvard.edu/abs/2019EGUGA.2113137C. (accessed January 23, 2020).
Collins, S., Genova, F., Harrower, N., Hodson, S., Jones, S., Laaksonen, L., et al. (2018). Turning FAIR Data Into Reality: Interim Report From the European Commission Expert Group on FAIR Data. Interim Rep. from Eur. Comm. Expert Gr. FAIR Data.
Dillo, I., and de Leeuw, L. (2018). Coretrustseal. VOEB-Mitteilungen. 71, 162–170. doi: 10.31263/voebm.v71i1.1981
E. F. D. Community (2018). Commitment Statement to Enabling FAIR Data in the Earth, Space, and Environmental Sciences.
ESRI (1998). Environmental systems research institute, ESRI shapefile technical description. Comput. Stat. 16, 370–371.
European Commission (2016). H2020 Programme Guidelines on FAIR Data Management in Horizon 2020. Available online at: https://www.unive.it/pag/fileadmin/user_upload/SBA/documenti/BDA/H2020_Guidelines_on_FAIR_Data_Management_Version3.0_26_luglio_2016.pdf (accessed February 7, 2018).
FAIR Data Maturity Model WG (2019). Available online at: https://www.rd-alliance.org/groups/fair-data-maturity-model-wg (accessed June 29, 2019).
Filgueira, R., Krause, A., Atkinson, M., Klampanos, I., Spinuso, A., and Sanchez-Exposito, S. (2015). “Dispel4py: an agile framework for data-intensive eScience,” in Proc. - 11th IEEE Int. Conf. EScience, EScience, (Munich) 454–464. doi: 10.1109/eScience.2015.40
Gurtner, W., and Estey, L. (2007). Rinex-the Receiver Independent Exchange Format-Version 3.00. Bolulder, CO: Astronomical Institute, University of Bern and UNAVCO.
Jan, B. (2009). “DataCite - a global registration agency for research data,” in 4th Int. Conf. Coop. Promot. Inf. Resour. Sci. Technol. COINFO. (Beijing) 257–261.
Jeffery, K., Harrison, M., Atakan, K., Bailo, D., Card, C., Michalek, J., et al. (2018). D7. 2 Second (final) Report on EPOS-ICS Architecture, 1–30.
Mons, B., Neylon, C., Velterop, J., Dumontier, M., Da Silva Santos, L. O. B., and Wilkinson, M. D. (2017). Cloudy, increasingly FAIR; revisiting the FAIR data guiding principles for the European Open Science Cloud. Inf. Serv. Use 37, 49–56. doi: 10.3233/ISU-170824
Petzold, A., and Glaves, H. (2018). ENVRI-FAIR - the next step towards FAIRer environmental research. Am. Geophys. Union Fall Meet. 2019:15684.
Petzold, G., Asmi, H. M., Bailo, A., Bundke, D., Vermeulen, U., Schaap, A, et al. (2020). ENVRI - FAIR - Interoperable Environmental FAIR Data Services for Society, Innovation Research. Zenodo, doi: 10.1109/eScience.2019.00038
Sansone, S. A., McQuilton, P., Rocca-Serra, P., Gonzalez-Beltran, A., Izzo, M., Lister, A. L., et al. (2019). FAIRsharing as a community approach to standards, repositories and policies. Nat. Biotechnol. 37, 358–367. doi: 10.1038/s41587-019-0080-8
Schultes, E., Strawn, G., and Mons, B. (2018). Ready, set, go fair: accelerating convergence to an internet of fair data and services. CEUR Workshop Proc. 2277, 19–23. Available online at: http://ceur-ws.org/Vol-2277/paper07.pdf. (accessed January 23, 2020).
Sicilia, M. A., García-Barriocanal, E., Sánchez-Alonso, S., and Cuadrado, J. J. (2019). “Decentralized persistent identifiers: a basic model for immutable handlers,” in Procedia Comput. Science, eds M.-A. Sicilia, E. Simons, A. Clements, P. de Castro, and J. Bergström (Umeå University), 123–130. doi: 10.1016/j.procs.2019.01.087
Trani, L., Atkinson, M., Bailo, D., Paciello, R., and Filgueira, R. (2018). Establishing core concepts for information-powered collaborations. Futur. Gener. Comput. Syst. 89, 421–436. doi: 10.1016/j.future.2018.07.005
Wilkinson, M. D., Dumontier, M., Ij.Aalbersberg, J., Appleton, G., Axton, M., Baak, A., et al. (2016). The FAIR guiding principles for scientific data management and stewardship. Sci. Data. 3:160018. doi: 10.1038/sdata.2016.18
Wilkinson, M. D., Dumontier, M., Sansone, S., da Silva Santos, L. O. B., Prieto, M., Batista, D., et al. (2019). Evaluating FAIR maturity through a scalable, automated, community-governed framework. Sci. Data 6:174. doi: 10.1038/s41597-019-0184-5
Wilkinson, M. D., Sansone, S. A., Schultes, E., Doorn, P., Da Silva Santos, L. O. B., and Dumontier, M. (2018). Comment: a design framework and exemplar metrics for FAIRness. Sci. Data 5:180118. doi: 10.1038/sdata.2018.118
Keywords: research infrastructure, FAIR principles, solid Earth science, multidisciplinary data, system interoperability
Citation: Bailo D, Paciello R, Sbarra M, Rabissoni R, Vinciarelli V and Cocco M (2020) Perspectives on the Implementation of FAIR Principles in Solid Earth Research Infrastructures. Front. Earth Sci. 8:3. doi: 10.3389/feart.2020.00003
Received: 10 October 2019; Accepted: 10 January 2020;
Published: 31 January 2020.
Edited by:
Susanne Buiter, Geological Survey of Norway, NorwayReviewed by:
Juliane Dannberg, University of Florida, United StatesMatthias Rosenau, German Research Centre for Geosciences, Helmholtz Centre Potsdam, Germany
Copyright © 2020 Bailo, Paciello, Sbarra, Rabissoni, Vinciarelli and Cocco. This is an open-access article distributed under the terms of the Creative Commons Attribution License (CC BY). The use, distribution or reproduction in other forums is permitted, provided the original author(s) and the copyright owner(s) are credited and that the original publication in this journal is cited, in accordance with accepted academic practice. No use, distribution or reproduction is permitted which does not comply with these terms.
*Correspondence: Daniele Bailo, daniele.bailo@ingv.it; bailo.daniele@gmail.com