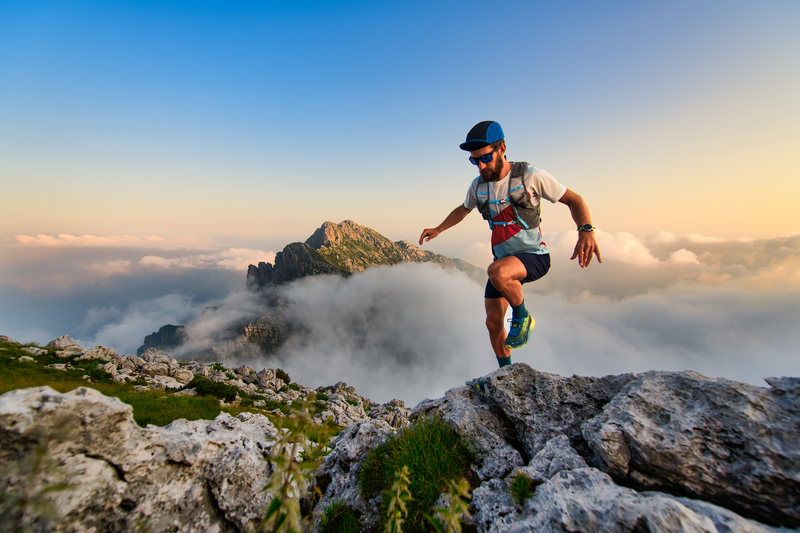
94% of researchers rate our articles as excellent or good
Learn more about the work of our research integrity team to safeguard the quality of each article we publish.
Find out more
REVIEW article
Front. Earth Sci. , 23 July 2019
Sec. Atmospheric Science
Volume 7 - 2019 | https://doi.org/10.3389/feart.2019.00152
This article is part of the Research Topic From the Satellite to the Earth's Surface: Studies Relevant to NASA’s Plankton, Aerosol, Cloud, Ocean Ecosystems (PACE) Mission View all 10 articles
NASA’s Plankton, Aerosol, Clouds, ocean Ecosystem (PACE) satellite mission is scheduled to launch in 2022, with the Ocean Color Instrument (OCI) on board. For the first time reflected sunlight from the Earth across a broad spectrum from the ultraviolet (UV: 350 nm) to the short wave infrared (SWIR: 2260 nm) will be measured from a single instrument at 1 km spatial resolution. While seven discrete bands will represent the SWIR, the spectrum from 350 to 890 nm will be continuously covered with a spectral resolution of 5 nm. OCI will thus combine in a single instrument (and at an enhanced spatial resolution for the UV) the heritage capabilities of the Moderate resolution Imaging Spectroradiometer (MODIS) and the Ozone Monitoring Instrument (OMI), while covering the oxygen A-band (O2A). Designed for ocean color and ocean biology retrievals, OCI also enables continuation of heritage satellite aerosol products and the development of new aerosol characterization from space. In particular the combination of MODIS and OMI characteristics allows deriving aerosol height, absorption and optical depth along with a measure of particle size distribution. This is achieved by using the traditional MODIS visible-to-SWIR wavelengths to constrain spectral aerosol optical depth and particle size. Extrapolating this information to the UV channels allows retrieval of aerosol absorption and layer height. A more direct method to derive aerosol layer height makes use of O2A absorption methods, despite the relative coarseness of the nominal 5 nm spectral resolution of OCI. Altogether the PACE mission with OCI will be an unprecedented opportunity for aerosol characterization that will continue climate data records from the past decades and propel aerosol science forward toward new opportunities.
Aerosols contribute the largest uncertainties in estimating climate forcing (Boucher et al., 2013). Reducing these uncertainties requires knowing global aerosol concentrations and distribution, and aerosol chemical, physical and optical properties. We also need to understand how aerosols affect other atmospheric constituents, especially clouds (Boucher et al., 2013). The process in which aerosols modify clouds to affect Earth’s energy budget is still poorly understood (Rosenfeld et al., 2014a; Seinfeld et al., 2016; Fan et al., 2016). Even more uncertain is how aerosol-cloud interaction affects precipitation onset and amount, influencing floods, droughts and the availability of fresh water (Andreae and Rosenfeld, 2008; Tao et al., 2012; Rosenfeld et al., 2014b).
The challenge to understanding these processes lies within the multiple pathways that aerosols, clouds and Earth’s energy balance intertwine (Koren et al., 2008; Stevens and Feingold, 2009; Morrison and Grabowski, 2011; Altaratz et al., 2014). Aerosols can act as cloud condensation nuclei (CCN) or as ice nuclei (IN), which means that variation on the concentrations or composition of aerosols can exert an influence on cloud evolution through a microphysical path (Koren et al., 2008; Altaratz et al., 2014). Observational and modeling studies have found a myriad of resulting changes in clouds linked to changes in aerosols via this microphysical path including changes to cloud albedo, cloud macrophysical properties, cloud “lifetime,” lightning and precipitation (Twomey, 1977; Albrecht, 1989; Kaufman and Nakajima, 1993; Rosenfeld and Woodley, 2001; Kaufman et al., 2005a; Khain et al., 2005; Koren et al., 2005, 2010, 2012; Rosenfeld et al., 2008; Li et al., 2011; Van den Heever et al., 2011; Yuan et al., 2011, 2012; Shi et al., 2014). Furthermore, because aerosols alter radiative absorption and scattering they can change atmospheric heating rates that will affect atmospheric stability and surface heat fluxes, which in turn affect cloud formation and evolution (Johnson et al., 2004; Koren et al., 2004, 2008; Feingold et al., 2005; Kaufman and Koren, 2006; Altaratz et al., 2014; Shi et al., 2014). We also know that aerosol-cloud interaction is a two-way street. Clouds can produce aerosols via nucleation in aqueous chemistry (Eck et al., 2014), and clouds can remove aerosols from the atmosphere through wet deposition (Chin et al., 2000). These intertwined processes occur in regimes that span 12 orders of magnitude from the microscale (aerosols ∼0.1 μm) to the synoptic scale (global ∼105 km). Mapping and understanding cloud, aerosol and energy balance processes across 12 orders of magnitude of spatial scale is required to fully understand today’s climate. The next challenge is to understand how these processes will evolve in a future changed climate.
In addition to their role in climate and hydrological processes, aerosols pose a serious global health threat. Particulate matter (PM) and aerosols that pollute ambient air are a major global cause of death and disease, having been found responsible for 3.2 million deaths per year as well as being the 9th leading risk factor for premature death globally (Lim et al., 2012). The relative toxicity of specific PM types — components having different size and chemical composition—is still poorly understood (Franklin et al., 2017). However, it has been shown that smaller (sub-micron) particles could be especially harmful since they can penetrate deeper into the lung (Franck et al., 2011). Aerosol particles can be lofted far from their sources and even transported across oceans to other continents (Kaufman et al., 2005b; Yu et al., 2012, 2013). Therefore, air pollution mitigation is a global problem because local air quality can be affected by sources far upwind. Some aerosols contain valuable minerals needed by terrestrial and marine plant life and, therefore, the transport and deposition of these aerosols can be an important source of micronutrients for otherwise nutrient-limited biomes (Jickells et al., 2005; Yu et al., 2015), or modify ocean chemistry and impact ocean biology (Ito et al., 2016).
An integrated approach is required to achieve sufficient understanding of the processes that aerosols affect. The approach requires modeling at all scales and observational data to inform and constrain these models. In situ data collection provides indispensable information about aerosol composition and detailed particle properties, but is limited to the surface layer or is brought aloft by infrequent aircraft or balloon sampling. Ground-based remote sensing observations such as the AERONET (Holben et al., 1998), other sun/sky radiometer networks, and lidars provide frequent measures of total column or vertically resolved aerosol properties However, these instrumented stations are typically limited geographically to continental land masses of wealthy countries, hence leaving the developing world and much of the world’s oceans undersampled (Knobelspiesse et al., 2004; Smirnov et al., 2009). Global statistics are beyond reach of these ground, aircraft or balloon observational systems, and models would be under-constrained if relying only on ground or suborbital observations. Only space-based remote sensing provides relatively frequent and consistent observations of the total atmospheric column.
Since the launch of Terra and the A-train constellation of satellites starting at the end of 1999, aerosol remote sensing reached a new era. The MODerate resolution Imaging Spectroradiometer (MODIS) and Multiangle Imaging SpectroRadiometer (MISR) on Terra, MODIS on Aqua, Ozone Monitoring Instrument (OMI) on Aura, Medium Resolution Imaging Spectrometer (MERIS), Sentinel-3 Ocean and Land Color Instrument (OLCI), Sentinel-2 Multi-Spectral Instrument (MSI), Polarization and Directionality of the Earth’s Reflectance (POLDER) on the Polarization and Anisotropy of Reflectances for Atmospheric Sciences coupled with Observations from a Lidar (PARASOL) and Cloud-Aerosol Lidar with Orthogonal Polarization (CALIOP) on Cloud-Aerosol Lidar and Infrared Pathfinder Satellite Observations; have combined to provide an unprecedented view of the global aerosol system. Focusing on passive remote sensing from radiometers, algorithms have extracted information from spectral measurements in the visible and shortwave infrared (SWIR) portions of the spectrum (i.e., MODIS), from spectral measurements in the ultraviolet (UV) portion of the spectrum (i.e., OMI and the earlier Total Ozone Mapping Spectrometer (TOMS) instrument from which OMI draws its heritage), and from multi-angular measurements in the visible and near-infrared (NIR) [i.e., MISR, Advanced Along Track Scanning Radiometer (AATSR) and POLDER]. Algorithms have also extracted information from multi-angular measurements of polarized reflectance in the visible (POLDER), but discussion of polarimetry is outside the scope of this review and will be covered in a companion paper by Remer et al. (2019), hereafter referred to as “Part 2.” The different information acquired by these different satellite sensors has enabled the community to produce reliable measures of total column ambient aerosol loading (aerosol optical depth - AOD) over a variety of land types and over the ocean (Boucher et al., 2013; Myhre et al., 2013), and even recently AOD in the column above clouds (Torres et al., 2012; Jethva et al., 2013, 2014a,b, 2016; Meyer and Platnick, 2015; Sayer et al., 2016). In addition, space-based measurements have produced information on aerosol microphysical properties: particle size parameter (Remer et al., 2005), single scattering albedo (SSA) (e.g., Jethva and Torres, 2011; Torres et al., 2013), particle non-sphericity (deviation from spherical shape, e.g., Kalashnikova and Kahn, 2006; Kalashnikova et al., 2013), and combinations of size, absorption and shape to categorize aerosol type (e.g., Kahn and Gaitley, 2015). Note, however, that, despite the unprecedented view from space, uncertainties remain because these retrievals are fundamentally underdetermined, contributing to large differences among satellite products in regional and seasonal patterns. Additionally, differences and uncertainties in calibration, sampling, cloud screening, treatment of the surface reflectivity, and aerosol retrieval algorithms contribute to overall retrieval uncertainty (e.g., Li et al., 2009; Kokhanovsky et al., 2010).
The information content of a single-look multispectral or hyperspectral sensor such as MODIS or OMI is limited, especially when neither spans the entire solar spectrum. MODIS does not measure in the UV, while OMI measures in the UV, but misses all wavelengths longer than 500 nm. In addition, OMI’s 13 km × 24 km footprint makes quantitative aerosol retrievals in all but the most cloud-free situations impossible. Both MODIS and OMI standard aerosol retrieval algorithms must rely on a series of assumptions in order to retrieve AOD and any other aerosol property. Multi-angle measurements of the same scene such as MISR or AATSR increase the information content because the instrument becomes sensitive to a wider array of aerosol properties with fewer assumptions. Even so, MISR and AATSR still are limited to determining “aerosol type,” a qualitative constraint based on a combination of size, absorption and shape. Aerosol type, while important for a wide range of applications, is not the same as determining quantitative particle properties such as size distribution, SSA or complex refractive index.
For retrieving these quantitative particle properties from space even more information is needed, such as with a multi-wavelength, multi-angle polarimeter of sufficient accuracy, wavelength range and resolution (Mishchenko et al., 2004; Cairns et al., 2010; Knobelspiesse et al., 2012; Kokhanovsky, 2015). Such instruments will be discussed more fully in Part 2 (Remer et al., 2019).
New contemporary algorithms applied to the traditional sensors are enhancing aerosol retrieval capabilities despite the inherent limitations. For example, an advanced type of algorithm only now making its way toward operations is a multi-pixel algorithm called the MAIAC (Lyapustin et al., 2011). MAIAC requires multiple days of views of the same scene in order to constrain surface reflectance for the aerosol retrieval. At the beginning of the MODIS era 18 years ago, holding onto a week’s worth of information at each pixel would have overwhelmed computer resources. In addition to computer resources, some algorithmic options require a data base containing, for example, spectral surface reflectance characteristics to be acquired for a particular sensor and then used in the operational forward processing stream. Thus, these algorithms generally are not optimal at launch and only achieve high accuracy and precision after the statistics of the data base have been acquired. The Deep Blue family of algorithms is an example of this type of algorithm (Hsu et al., 2004, 2013). For some purposes waiting for an algorithm to mature is entirely appropriate (e.g., accumulating long-term data records, offering constraint on aerosol and climate models). For other purposes such as near real-time hazards warning and other aerosol air quality applications, at-launch algorithms are highly desirable.
Single-view radiometers (e.g., MODIS and OMI) have provided decades of insight into the global aerosol system and new observational methods to constrain estimates of climate change (Boucher et al., 2013), study aerosol-cloud interaction (Koren et al., 2005, 2010, 2012), follow intercontinental transport of particles (Yu et al., 2012, 2013) and improve particulate air pollution forecasts (Al-Saadi et al., 2005). Enhancement to these heritage capabilities using single-view radiometry, even at launch, is possible, despite the limitations of the viewing geometry and lack of polarization information, but will require sensors that measure in additional wavelengths, cover a broader spectral range and/or at a finer spectral, spatial or temporal resolution.
This paper explores the potential for aerosol remote sensing and characterization in the PACE era, primarily from the OCI that has been designed to continue and even enhance heritage aerosol algorithms over both ocean and land. Section “Aerosol Remote Sensing for the PACE Mission” begins with a brief description of the PACE observatory, the two types of instruments on the observatory and the possibility for remote sensing of aerosols from the observatory. This is followed by discussion on the abilities and challenges of adapting current aerosol algorithms to OCI measurements in order to continue heritage aerosol time series beyond the lifetimes of today’s sensors and missions. Section “Advances Beyond Heritage. 1: Broad Spectral Range Retrievals From OCI” explores the potential of using OCI’s broad spectrum from the UV to the SWIR to retrieve an enhanced set of aerosol characterization products. These products include spectral AOD, a measure of particle size, SSA and aerosol layer height (ALH). Section “Advances Beyond Heritage, 2: Oxygen A-band Information Content on Aerosol Profiling” describes advances beyond heritage remote sensing by exploring the possibilities of retrieving ALH by capitalizing on OCI’s 5 nm spectral resolution through the oxygen A-band (O2A). In Section “Advances Beyond Heritage, 2: Oxygen A-band Information Content on Aerosol Profiling,” we also broach the possibility of improved aerosol profile retrievals from the combination of multiangle observations in the O2 A-band. Part 2 of this study (Remer et al., 2019) explores this in more detail. Finally in Section “Discussion and the Path Forward,” we discuss the results, identify the gaps that still exist before OCI’s full potential as an aerosol instrument can be realized, and provide suggestions for the path forward.
The algorithm concepts presented in this paper illustrate the potential for aerosol remote sensing from OCI. These concepts are not the official, operational, set of algorithms as these will require additional vetting. The focus of this paper is OCI. For further analysis of PACE aerosol remote sensing with multi-angle and polarimetry, we refer the reader to the companion paper in this same issue (Remer et al., 2019).
The PACE mission offers the aerosol community new opportunities for retrieving and characterizing aerosol from space. There are two levels of opportunity based on the two types of instruments the mission intends to fly. First there is OCI (Del Castillo et al., 2012) that will measure reflected sunlight from the Earth across the broad spectrum from the UV (350 nm) to the SWIR (2250 nm) all at nominally 1 km spatial resolution. The spectrum from 345 to 890 nm will be spectrally continuous with a spectral resolution of 5 nm, while the SWIR wavelengths will be measured in seven discrete bands. Opportunities from OCI will be based on the heritage of products already being produced from similar instruments such as MODIS and OMI. Table 1 compares the characteristics of MODIS, OMI and OCI. At the very least OCI will be able to continue the aerosol record begun from MODIS and OMI, providing opportunity to collocate and compare with similar aerosol algorithms applied to the Visible Infrared Imaging Reflectance Suite (VIIRS) and the constellation of geosynchronous satellites planned for the PACE time frame [Advanced Baseline Imager (ABI) on Geostationary Operational Environmental Satellite – Series R (GOES-R), etc.]. In addition to continuity, aerosol science from PACE OCI will advance beyond the capabilities provided by heritage instruments because OCI offers moderate spatial resolution in the UV region and fine spectral resolution through the oxygen-A band.
The second type of PACE instrument will be a pair of multi-wavelength, Mulit-Angle Polarimeters (MAPs). The PACE MAPs have the high potential to push beyond traditional limitations in characterizing aerosol retrievals with new capabilities that include retrievals of particle size distribution, complex refractive index, SSA, aerosol height distribution and simultaneous retrievals of aerosol properties with surface (ocean and land) reflectance (Waquet et al., 2009; Chowdhary et al., 2012; Xu et al., 2016, Xu F. et al., 2017; Gao et al., 2018; Stamnes et al., 2018).
Aerosol products for the PACE mission will be derived from OCI alone and from the MAPs alone. However, there is also opportunity to develop and use advanced algorithms that make use of all instruments in synergy. PACE aerosol products will at minimum, match aerosol products currently available from the OMI, MODIS and VIIRS sensors, and include the UV aerosol index (AI), spectral AOD over land and ocean, and fine mode fraction (FMF) over ocean. The FMF is a measure of the relative effect that this mode has on the reflectance at top-of-atmosphere as compared with the effect of the total aerosol of all sizes on the reflectance (Remer et al., 2005). Note that in the MODIS aerosol context the fine mode refers to a lognormal particle size distribution with effective radius less than 0.5 μm. Advances from this minimum set will depend on algorithm development as applied to OCI and the PACE polarimeters.
Fundamental to any aerosol retrieval is the need to identify scenes applicable for useful retrievals. This process, called masking, includes identifying and excluding scenes containing clouds, snow/ice, sun glint, and more from retrievals. In the PACE mission some masking will improve, others will be worse, while some will remain essentially the same as heritage aerosol algorithms. With the added information content for PACE, some retrievals that would have been masked for the heritage algorithms, may be able to proceed. For example, aerosol retrievals above clouds and otherwise unfavorable surfaces may be possible.
OCI lacks thermal infrared (IR) observations, which in terms of clouds, will lead to a degraded cloud masking capability compared to heritage MODIS and VIIRS datasets. However, compared to heritage OMI/TOMS-alone datasets, improved spatial resolution and expanded wavelengths into the SWIR will allow for significantly improved cloud masking. The OCI 1.38 μm channel is especially important for detecting thin cirrus clouds. In addition, the 1.38 μm channel may offer possibilities for “correction” rather than blunt masking by removing the net radiative effect of the cirrus in an adjustment of the measured top-of-atmosphere radiances, and then using those previously cirrus-affected pixels to derive information about the aerosol and/or surface beneath. Furthermore, the pair of SWIR channels centered at 2.135 and 2.25 μm will provide information on cloud thermodynamic phase unavailable from previous sensors (Coddington et al., 2017).
Masking is also typically required to remove the impact of the direct and diffuse solar radiation reflected by the sea surface and other sea surface features that can significantly enhance NIR and SWIR. Highly scattering non-aerosol targets on the ocean surface include whitecaps, foam and bubbles, sea ice, floating vegetation, high calcite waters, high sediment waters, optically shallow waters (e.g., with bright coral or sand) and regions with concentrated floating plastics (Frouin et al., 1996; Li et al., 2003; Marmorino and Smith, 2005; Balch et al., 2011; Dierssen et al., 2015; Fogarty et al., 2017; Garaba and Dierssen, 2018; Garaba et al., 2018; Perry et al., 2018). The most widespread of these is enhanced reflectance due to the production of whitecaps that occur over vast regions of the global ocean, particularly in the Southern Ocean (Albert et al., 2016). The heritage approach to mask whitecaps uses wind speed measurements to estimate the whitecap fraction, but such relationships are only climatological and do not predict instantaneous whitecap fields. Moreover, new measurements of whitecaps indicate that they have more spectral features across the visible to SWIR and new approaches are being tested to predict their contribution to at-sensor radiance without the reliance on climatological wind speeds (Dierssen, 2019). It is expected that improvements in assessments of wave conditions and whitecap fraction will help constrain the large uncertainty in emission fluxes for sea spray aerosols (Albert et al., 2010).
OCI, like OMI, is a hyperspectral resolution imager with continuous spectral coverage at 5 nm spectral resolution that spans a wavelength range from the UV into the NIR. From an aerosol retrieval perspective OCI is an improvement on OMI as its spatial resolution will nominally be 1 km instead of 13 km × 24 km, and its continuous spectral range extends to 885 nm, while OMI’s ends at 500 nm. However, OCI’s spectral resolution of its contiguous channels is 10 times coarser than OMI’s in the UV and visible.
The TOMS/OMI heritage products include both the qualitative UV AI (Herman and Celarier, 1997; Herman et al., 1997) and the quantitative suite of aerosol characteristics that include extinction AOD and SSA at 380 nm (Torres et al., 1998, 2002, 2005, 2007). The quantitative OMI aerosol retrieval fits two measured UV channels to the values of pre-computed reflectances stored in a Look Up Table (LUT). Aerosol type and layer height are constrained from ancillary information, leaving the algorithm to choose between different values of AOD and aerosol absorption (Torres et al., 1998, 2002, 2007). In the case of OMI-like aerosol algorithms applied to OCI inputs, we can expect a direct translation of the OMI technique to OCI measurements. There is already experience in porting the basic UV algorithm from its original application with TOMS observations to OMI. OCI’s coarser spectral resolution should not be a detrimental factor in applying the heritage algorithm, and because OCI has much finer spatial resolution than OMI, clouds should be less of a factor in the retrieval. This should significantly increase the overall availability of high quality retrievals, especially in cloudier conditions.
In addition, the wavelength pairs in the OCI oxygen A- and B- band spectral regions (∼690 and ∼765 nm) and in their adjacent continuous regions (∼680 and ∼780 nm) will allow the retrieval of the height of desert dust and carbonaceous aerosol layers using inversion schemes developed for the Earth Polychromatic Imaging Camera (EPIC) sensor (Xu X. et al., 2017; Xu et al., 2018b) (see Discussion and the Path Forward). The retrieved ALH will better constrain the OMI-like AOD/SSA retrieval resulting in more accurate products than current attainable from the OMI inversion. Thus, the OMI-like approach will be optimized on the account of OCI’s both fine spatial resolution and extended spectral coverage.
Multiple heritage aerosol algorithms are encompassed by the MODIS/VIIRS era. Of these, the most pertinent to the discussion that follows is the Dark Target (DT) over ocean retrieval (Tanré et al., 1997; Remer et al., 2005; Levy et al., 2013). In this algorithm measured top-of-atmosphere reflectances in six spectral bands (0.55, 0.66, 0.87, 1.24, 1.63, and 2.13 μm) are fit to calculated reflectances in a LUT. The table is constructed under the assumption that the aerosol size distribution can be represented by two lognormal distributions, one with effective radius larger than 0.5 μm (the coarse mode) and one with effective radius smaller than 0.5 μm (the fine mode). A successful match yields the spectral AOD and one or two size distribution parameters. Greater detail of this method will be given in Section “Advances Beyond Heritage. 1: Broad Spectral Range Retrievals From OCI” as this heritage algorithm forms the basis of Step 1 of the proto-algorithm developed for application to OCI inputs.
OCI’s hyperspectral range spans four of the MODIS bands used by the MODIS DT aerosol over ocean algorithm and further extends to include six discrete bands in the SWIR thereby spanning the range of wavelengths needed by any of the heritage aerosol algorithms, even those not specifically discussed in this paper. OCI spatial resolution of 1 km is less desirable than MODIS’ 0.5 km, and OCI is missing MODIS’ thermal IR channels used in cloud masking. Recent studies have shown that this could make a difference in maintaining continuity, but still achieve desired accuracy of key retrieved aerosol parameters (Levy et al., 2015). Thus, the OCI configuration of wavelengths, spatial resolution, swath width and temporal sampling is sufficient to produce AOD over land and ocean, from the UV to the SWIR, and the MODIS FMF over ocean to the accuracies and precisions of heritage MODIS products.
Even though the OCI configuration spans the same parameter space of heritage MODIS algorithms, a seamless continuation of the heritage product time series is not guaranteed. Experience in porting MODIS retrievals to VIIRS has revealed the subtleties of the transfer. Issues arise in translating band spectral response functions from old sensor to new sensor, changes in gas corrections resulting from differences in spectral response function and the creation of specific LUTs for the new sensor. Other issues are introduced by differences in spatial resolution and lack of auxiliary channels used for masking (e.g., clouds). Finally, calibration of radiances from one sensor to the next may differ, introducing offsets that may either be static from pre-launch differences and also time-dependent from on-orbit corrections.
Figure 1 illustrates some of these issues based on experience in porting the MODIS DT algorithm to VIIRS. The porting process consisted of (1) creating a LUT for VIIRS based on the VIIRS channels that paralleled the LUT used by the MODIS retrieval, (2) degrading the MODIS spatial resolution to match the VIIRS spatial resolution, and (3) applying a cloud mask to both MODIS and VIIRS inputs that used only wavelengths and spatial resolution common to both sensors. Then the MODIS algorithm could be applied to the (spatially) degraded MODIS inputs using the MODIS LUT. This resulted in a product labeled “MODIS-like on MODIS” (ML_M). “MODIS-like” is not the same as the operational Collection 6 MODIS algorithm (M_C6) because ML_M used different spatial resolution and different masking than M_C6. Figure 1A shows the seasonal mean AOD (March–April–May) of the MODIS-like algorithm applied to MODIS inputs, and Figure 1C shows the difference between the MODIS-like algorithm and the original operational true MODIS algorithm. Differences in algorithm applied to the same inputs are small, but regionally can reach magnitudes of ±0.05 AOD. These differences are attributed mostly to differences in sampling caused by masking differences.
Figure 1. (A,B) Global seasonal mean AOD at 550 nm (March–April–May) as derived from the MODIS-like retrieval algorithm applied to MODIS input radiances (A) and applied to VIIRS input radiances (B). (C) Difference between (A) and the corresponding seasonal mean AOD at 550 nm produced from the operational MODIS aerosol retrieval algorithm. (D) Difference between (B) and (A). Reprinted from Levy et al. (2015), under Creative Commons Attribution 3.0 License.
Figure 1B shows the seasonal mean AOD for the same MODIS-like algorithm applied to VIIRS inputs. Comparing Figures 1A,B demonstrates the consistency in the products after the porting. Figure 1D is the difference between applying exactly the same algorithm to the inputs of the two sensors. Here, differences in AOD can be as large as ±0.07 AOD over land, and systematically biased 0.02 to 0.10 over ocean. The take home message from Figure 1 is in comparing Figures 1C,D. Figure 1C shows the difference in retrieved AOD due to algorithmic difference (with same inputs) is relatively small, while Figure 1D shows relatively large differences in AOD due to different inputs (with same algorithm). Input reflectances, not the algorithm, are the major challenge to overcome in matching retrieved AOD data sets from different sensors. The differences in Figure 1D have been traced to fundamental biases in the input radiances of specific channels and could be eliminated with calibration that forces the instruments to be the same. Achieving continuity in the aerosol record between any two sensors, such as MODIS and VIIRS, requires a period of overlap during which differences can be identified and calibration adjusted, if necessary.
In Figure 1 we demonstrate differences in the results of applying the same algorithm to both MODIS and VIIRS input reflectances. Now we attempt to determine which of the two satellite sensors is returning the more accurate AOD product by comparing satellite retrievals against collocated ground-based AERONET measurements. Figure 2 shows the differences between satellite retrieval and AERONET AOD as a function of AERONET AOD. In Figure 2B, we can see the same positive bias of ML_V for ocean retrievals against AERONET as we saw ML_V against ML_M in Figure 1D. This suggests that the VIIRS radiances are the ones requiring calibration adjustment. We note that the MODIS and MODIS-like algorithms employ a different set of wavelengths in their retrievals over ocean and land. Calibration biases in particular wavelengths will not necessarily affect AOD retrievals the same over ocean and land.
Figure 2. Differences between satellite retrieval of AOD and collocated AERONET measurements of AOD from a global data set of satellite-AERONET collocations, sorted according to AERONET AOD and binned with equal number of collocations in each bin. Red dots indicate mean of each bin, boxes enclose one standard deviation in each bin, whiskers extend to two standard deviations and blue center line of each box denotes the median. (A,C) Is for the MODIS-like retrieval algorithm applied to MODIS input radiances. (B,D) Is for the same MODIS-like retrieval algorithm applied to VIIRS input radiances. (A,B) is for aerosol retrievals over ocean, and (C,D) is for retrievals over land. Reprinted from Levy et al. (2015), under Creative Commons Attribution 3.0 License.
If all reflectance measurements made by OCI are well-calibrated, we anticipate similar magnitude differences between heritage aerosol products and OCI aerosol products based on heritage algorithms as is illustrated in Figure 1C. These small differences, due to the coarser spatial resolution of OCI and its lack of IR channels that MODIS uses for masking, are unbiased and within the expected uncertainty of heritage products. However, as Figures 1D, 2 demonstrate, inexact instrument calibration, even within specified uncertainties of sensor characterization teams, has the potential to increase differences in retrieved AOD and create biases that are outside heritage expectations.
Irrespective of the challenges described above, OCI-alone can still advance aerosol characterization beyond heritage expectations by allowing for new algorithms that make use of the information content available from a broad spectrum spanning the UV to the SWIR. This is essentially merging the capabilities of OMI and MODIS into a single sensor with common geometry and spatial resolution, instantaneous viewing and the same calibration. This configuration will permit retrieval of aerosol loading (spectral AOD), a measure of particle size (FMF), a measure of aerosol absorption (SSA), and ALH. Thus, OCI should be able to retrieve four parameters to characterize aerosol, while OMI and MODIS heritage algorithms separately could each only retrieve two of the four parameters, while assuming values for the remaining two. Aerosol layer in this context is an optically effective scale height. Other satellite sensors past and present have also measured reflectances from the UV to the SWIR. These include the Thermal And Near infrared Sensor for Carbon Observation – Cloud and Aerosol Imager (TANSO-CAI) with four wavelengths (380, 674, 870, and 1620 nm) at 1 km spatial resolution (eoPortal directory: GOSAT) and the Tropospheric Ozone Monitoring Instrument; (TropOMI) with three spectral regions (270–495 nm, 710–775 nm, and 2305–2385 nm) at 7 km spatial resolution (European Space Agency [ESA], 2013). Data from TANSO-CAI and TropOMI may be able to provide a test bed for OCI algorithm development, although to date, there are no published studies describing full spectrum aerosol retrievals from these sensors.
Reflectances in the UV spectrum (e.g., those used in the OMI aerosol algorithms) are primarily sensitive to three aerosol characteristics: aerosol loading (AOD), aerosol absorption, and height of the aerosol layer. Unfortunately, heritage measurements in the UV provide only two pieces of independent information. Thus, retrievals are unconstrained, requiring assumptions to constrain one of the three aerosol parameters in order to retrieve the other two. The traditional tactic taken and applied to TOMS and OMI aerosol algorithms is to constrain aerosol height with assumptions based on climatology and then retrieve loading and absorption in the form of AOD and SSA. The retrievals, especially SSA, are also susceptible to assumptions of (i) surface reflectances, particularly in extremely oligotrophic oceans, and (ii) aerosol particle properties used by the retrieval, including particle size and most importantly the spectral signature of absorption through the UV and blue regions of the spectrum.
Meanwhile, aerosol retrievals based on the visible-NIR-SWIR spectrum (e.g., those applied in the MODIS and VIIRS aerosol algorithms) are primarily sensitive to only two aerosol characteristics: aerosol loading (AOD) and a measure of relative particle size based on spectral AOD. In addition to spectral AOD, particle size can be represented by a parameter denoted as FMF, which is usually thought of in terms of the ratio of fine mode AOD at 550 nm to total AOD at 550 nm. The retrieval in the visible-NIR-SWIR is less sensitive to ALH or aerosol absorption. Some MODIS algorithms that make use of the blue wavelengths retrieve and report SSA, but those products have not been validated.
Making use of the complementary capabilities of OMI and MODIS was an early goal of both science teams. Soon after the OMI-alone aerosol retrieval had been validated, attempts were made to join OMI’s sensitivity to absorption and layer height with MODIS’ sensitivity to loading and size. With both instruments being in the A-Train, and measuring less than 8-minutes apart, it was theoretically possible to create such a combined OMI-MODIS retrieval. However, the endeavor to merge Level 1 radiance data from the two instruments faced significant challenges in terms of non-uniform calibration, spatial resolution and viewing geometry. Instead, a retrieval was devised that used Level 2 retrieval products from each instrument. MODIS spectral AOD was extrapolated into the UV, thereby constraining the UV AOD and allowing OMI to return a constrained SSA and ALH that compared well to independent observations (Satheesh et al., 2009). This tactic was never implemented operationally, due to lack of investment to bring the idea to maturity. Recently Gassó and Torres (2016) provides additional demonstration of the concept.
The EPIC sensor on Deep Space Climate Observatory (DSCOVR) currently deployed on the L1 point (Marshak et al., 2018) merged measuring capabilities in the near UV, visible and NIR (including channels in the oxygen-A and B-bands) into a single instrument. A successful method to derive ALH from EPIC’s oxygen A- and B- channels was developed (Xu X. et al., 2017) and the results compared to independent observations over the oceans. Most recently, Xu et al. (2018b) also demonstrated the method over dark vegetated surfaces. The application of this inversion technique to OCI observations will go a long way into addressing the need of constraining the ALH in OMI-like near UV retrieval applications. We will further address O2A retrievals for OCI in section “Advances Beyond Heritage, 2: Oxygen A-band Information Content on Aerosol Profiling.”
Here we present a novel, OCI prototype algorithm developed specifically for a single instrument spanning the complete range from the UV to the SWIR and therefore moves beyond the Satheesh et al. (2009) technique. The algorithm requires a uniform calibration, spatial resolution and viewing geometry across the broad spectrum. The algorithm is based on the DT aerosol over ocean algorithm (Tanré et al., 1997; Remer et al., 2005; Levy et al., 2013) that was developed for MODIS, and has since been ported to VIIRS and other sensors. The prototype algorithm expands the DT algorithm to include UV channels that are used to retrieve SSA. This prototype algorithm is presented here for the first time.
The OCI prototype algorithm begins first by identifying applicable ocean scenes for retrieval and discarding non-applicable scenes with clouds/cirrus, ice, glint or suspended sediments in the water. The algorithm then ingests reflectances of the identified ocean scene in six wavelengths (550, 650, 880, 1240, 1640, and 2110 nm), as well as ancillary data in the form of total column ozone and surface wind speed. The water leaving radiance is assumed null at all wavelengths, except at 550 nm, where it is assumed equal to 0.005. The measured reflectances are compared with modeled top-of-atmosphere (TOA) reflectances, and the best fit between satellite-measured and modeled TOA spectral reflectances is used to determine the aerosol characteristics and aerosol loading (AOD) of the scene. Modeled TOA reflectances are computed for a combination of one fine mode model (out of four) and one coarse mode model (out of five). In all nine models aerosols are assumed to be spherical particles with minimal absorption. The latter assumption is justified by the fact that the algorithm at these wavelengths is not sensitive to particle absorption. Thus the DT retrieval returns the AOD, a choice of a fine mode and a coarse mode, each defined with a specific size distribution, and also the relative weighting between fine and coarse modes, resulting in a bimodal size distribution.
The first step, as described above, is unchanged from the traditional DT algorithm first developed for MODIS. To the first order, because of the selected wavelengths, the DT algorithm is sensitive to aerosol loading and to particle size distribution but insensitive to absorption variation (Tanré et al., 1996, 1997). The nine DT aerosol models represent particles with minimal absorption with imaginary parts of the refractive index spanning 0.001–0.0035 (Remer et al., 2005). The choice of the imaginary part of the refractive index in these wavelengths was based on ground-based measurements, evaluated later by total column sky radiometer retrievals (Tanré et al., 1997; Dubovik et al., 2002). However, because the primary sensitivity of the retrieval using wavelengths of 0.55 μm and longer is to aerosol loading and size distribution, the effect of uncertainties in assumed absorption properties is to introduce manageable uncertainty in the retrieval of the primary aerosol characteristics. The traditional DT algorithm was not able to distinguish between particles of the same size, but different absorptions, nor able to retrieve a quantitative measure of absorption.
The creation of a second step, an appendage to the traditional DT algorithm that uses the returns from the first step, provides quantitative measures of aerosol absorption from measured UV reflectances. The other inputs are OCI-measured reflectances at 354 and 388 nm. In this second step we use expanded LUTs of the nine traditional models to include the two new UV wavelengths, and in addition allow the imaginary part of the refractive index to vary between four distinct values in these two new wavelengths. Size distribution and the real part of the refractive index are spectrally extrapolated to the UV from the original values assigned to each of the nine models for visible and SWIR wavelengths, and are kept constant as those models’ imaginary parts of the refractive index are allowed to vary between four different values. These four imaginary parts of the refractive index represent four different models representative of different degrees of aerosol absorption. These are referred to as “absorption models,” even though one is purposely non-absorbing. The range of the imaginary part of the refractive index varies from 0.000 to 0.011. Thus the prototype algorithm LUT consists of 36 models: nine original DT models, each expanded into four possible absorption models in the UV spectrum.
The LUT of step 2 consists of top-of-atmosphere reflectances at 354 and 388 nm for a variety of geometries, a variety of AODs at 0.55 μm extrapolated to the UV wavelengths using the extinction optical properties of each of the 36 models and for four ALHs.: 1.5, 3, 6, and 10 km. Thus for a specific geometry and a specific AOD at 0.55 μm determined from step 1, there are computed reflectances from 144 possible situations (36 models × 4 layer heights). Note that the expansion into multiple absorption properties and layer heights for each size distribution only affects the computed top-of-atmosphere reflectance in the two UV wavelengths. There is no change in the LUT values of the original six wavelength bands of the first step. As such, step 1 and step 2 are only linked because the outputs of step 1 become the inputs to step 2. Step 1 already has determined the size distribution that best fits the observations, choosing one fine mode and one coarse mode from the nine possible models and combining them into a bimodal distribution with a retrieved reflectance weighting parameter (FMF). This retrieved bimodal size distribution and the retrieved spectral AOD are the outputs from step 1 that are input into step 2. These inputs from step 1 constrain the step 2 retrieval in the UV into just 16 possibilities (4 absorption levels × 4 layer heights) out of the original 144.
In the traditional UV aerosol retrievals (Torres et al., 1998) the measured reflectance in the UV wavelengths is sensitive to four aerosol properties: loading (AOD), absorption, layer height and aerosol type (size distribution). The retrieval then assumes two of the four properties and uses the spectral dependence of the UV reflectances to determine the remaining two parameters. For example, the retrieval might assume aerosol type and layer height to retrieve AOD and absorption (Torres et al., 1998). The spectral dependence of the UV reflectances is key in pulling out two pieces of information in the retrieval. Here in the OCI proto-algorithm, we do not need to assume two properties because Step 1 provides that information, and we use the spectral dependence of the UV reflectances to determine both absorption and layer height from the 16 possibilities in the LUT. The proto-algorithm returns the interpolated layer height and interpolates the SSA from the values of the two absorption models closest to the observations.
Figure 3 demonstrates this prototype algorithm applied to custom granules of merged MODIS and OMI Level 1 radiances.
Figure 3. Examples of the proto-type OCI algorithm described in the text applied to custom-built collocated MODIS-OMI granules where the OMI reflectances are mapped to the MODIS granule at the MODIS spatial resolution, although the OMI reflectances maintain their original geometric meta data. The (left) column shows the true color MODIS granules. (Center) Shows the standard MODIS AOD at 550 nm retrievals and the (right) column shows the resulting single scattering albedo at 388 nm using the OMI UV reflectances. (Top) Row is for a smoke case over the ocean (23 October 2007, 21:40 UTC). (Bottom) Row is for a dust case over ocean (13 March 2006, 15:25 UTC).
We tested the algorithm through a series of experiments in which we simulate TOA spectral reflectance from forward calculations using the aerosol models described above, and then retrieve from these calculated reflectances using the proto-algorithm. Doing such an experiment for a range of AOD at 550 nm (0–3.0) and doubling the normalized water leaving radiance to 0.010 from the UV to 0.66 μm, we found the algorithm retrieved the correct input parameters in 100% of the cases when AOD > 0.30, but only 56% of the cases when the AOD was less. This suggests that we can expect little sensitivity to ocean color as long as AOD at 550 nm exceeds about 0.30. When AOD is less than this threshold, preliminary results obtained from theoretical studies suggest that absorbing aerosol can still be identified and separated from non-absorbing marine aerosol, even if the absorption cannot be quantified. Identifying absorbing aerosol depends on spectral signatures in the UV region that differ from spectral signatures of chlorophyll in Case I (open ocean) waters.
One of the expected products from this prototype algorithm is an optically effective aerosol layer scale height. Retrieving this parameter should be possible, given that the AOD and aerosol model are sufficiently constrained by Step 1 of the algorithm. However, this algorithm will not be the only means of obtaining height from PACE instruments. As will be shown in Section “Advances Beyond Heritage, 2: Oxygen A-band Information Content on Aerosol Profiling,” there is sufficient information in the OCI channels that cover the O2A to constrain aerosol height independently. In addition one of the MAPs on PACE will be measuring the polarized signal in the UV and deep blue wavelengths. ALH can also be retrieved from the polarized signal in these wavelengths (Wu et al., 2016). Thus, there will be at least three independent methods for characterizing ALH from PACE. Not only will this redundancy confirm the OCI broad spectrum retrieval of layer height, but in doing so, it will also increase confidence in the retrievals of the other parameters produced by the proto-algorithm.
Validation of the prototype retrieval demonstrated in Figure 3 is based on collocations with SKYNET retrievals. SKYNET is a ground-based network of autonomous sun/sky radiometers, like AERONET, except SKYNET also makes sky scans in the UV, and retrieves SSA in the UV. This provides a direct comparison with the satellite retrieval’s primary product without spectral extrapolation to the visible. Collocations with SKYNET retrievals are rare, and so far validation of the prototype algorithm has been inconclusive. The reason SKYNET was selected for validation and not AERONET is that originally AERONET instruments did not perform a sky scan at 380 nm and therefore no absorption retrieval was produced in the UV. Subsequently some AERONET stations do produce absorption products in the UV, but the same problems encountered with SKYNET collocations would also apply to AERONET.
Part of the difficulty in proving this retrieval with the merged MODIS-OMI data sets is the original problem of possible inconsistent calibration, spatial resolution and geometry. The work done so far demonstrates feasibility and identifies/quantifies sensitivities. The exact retrieval algorithm to be applied to actual OCI observations may take advantage of advanced computer power and two decades of experience and need not follow the MODIS or OMI heritage procedures, as was presented here. Although any OCI aerosol algorithm will make use of the same information content and be challenged by the similar uncertainties.
OCI offers two new capabilities that permit advancing aerosol retrievals beyond heritage. In Section “Advances Beyond Heritage. 1: Broad Spectral Range Retrievals from OCI” we explored the first of these capabilities: the broad spectrum from the UV through the SWIR at a common 1 km spatial resolution and in a single instrument. In Section “Advances Beyond Heritage, 2: Oxygen A-band Information Content on Aerosol Profiling” we explore the second of these capabilities: the continuous 5 nm spectral resolution through the O2A (758–778 nm) region. This capability through the O2A can be mined from the single-view OCI measurements to retrieve ALH, but if it can be measured from a multi-angle instrument, such as the PACE MAPs, information on aerosol layering in the atmosphere increases. Both of these situations will be addressed in this section.
Radiance measurements in the O2A are sensitive to the vertical distribution of atmospheric scatterers, which in principle allows for the retrieval of aerosol height from future instruments with hyperspectral capability. OCI has continuous spectral measurements through the O2A and, while much coarser than an ideal (quasi-line-by-line) hyperspectral coverage such as by Orbiting Carbon Observatory (OCO)-2/3 sensors (Crisp et al., 2004), it may have sufficient spectral resolution to achieve similar results. We therefore address here aerosol height estimation via spectral analysis of O2 absorption in the visible-NIR spectral region. The O2A method to be shown in this section is governed by spectral gas absorption, and not the interplay of Rayleigh and aerosol scattering used by radiometric and polarimetric UV and blue-channel visible measurements (Torres et al., 1998; Wu et al., 2016). The spectral gas absorption method is less affected by confounding factors that create ambiguities in the scattering methods (Kalashnikova et al., 2011; Wu et al., 2016). For example, in the prototype algorithm described in Section “Advances Beyond Heritage. 1: Broad Spectral Range Retrievals from OCI,” retrieval of ALH can be confounded by unknown AOD, absorption or particle size distribution. Determination of the height of a layer of scattering particles using spectral analysis of an absorbing gas is grounded in differential optical absorption spectroscopy (DOAS) (Hebestreit et al., 1999). DOAS is normally used to infer the unknown amount of absorbing gas of known cross-section across a known path. If, as is the case for atmospheric O2, where both the amount and cross-section of the gas are accurately known, one can infer the effective path through the gas. This in turn becomes a means of locating the layer of the aerosol scattering contributions in the atmospheric column.
The ability to use oxygen absorption for the retrieval of aerosol height from passive sensors has been described extensively in the literature (Gabella et al., 1999; Min and Harrison, 2004; Corradini and Cervino, 2006; Pelletier et al., 2008; Dubuisson et al., 2009; Kokhanovsky and Rozanov, 2010; Frankenberg et al., 2012; Sanghavi et al., 2012; Ding et al., 2016; Xu X. et al., 2017, Xu et al., 2018a,b, Zeng et al., 2018; and others). Therein, the need for the highest possible spectral resolution has been clearly demonstrated. Indeed, the better the spectral resolution, the more samples of weak to strong absorption there are, yielding better vertical resolution in the scattering layer height estimate, and possibly its thickness as well. In other words, a coarse aerosol profiling becomes possible using a passive sensor system. However, compared with active (lidar) approaches to aerosol profiling, the complications of passive DOAS-based methods, including multiple scatterings and surface reflections, increase retrieval uncertainty. To ensure detectability, the aerosol loadings should therefore be significant. Previous theoretical and observational studies (Corradini and Cervino, 2006; Dubuisson et al., 2009; Kokhanovsky and Rozanov, 2010) have suggested an AOD at 550 nm in excess of ≈0.3 is necessary to infer height to within a few hundred meters. Our present analysis shows that AOD in the O2A bands > ≈ 0.1 (equivalent to ≈0.15 at 550 nm for an Angstrom exponent of about unity) may suffice over a dark surface such as water.
OCI, at a nominal resolution of 5 nm, will have 3 or 4 spectral bands in the O2A, but only at a single viewing angle. One of the proposed PACE MAPs, Spectro-Polarimeter for Planetary Exploration-1 (SPEXone) (Hasekamp et al., 2019) has hyperspectral radiometry at 2 nm resolution up to 770 nm for five viewing angles. However, SPEXone would provide only one piece of O2A information per pixel, namely, the ratio of a continuum channel and an in-band channel, but will be available at five viewing angles. One of the questions that we explore here is whether observing at different view angles is essentially equivalent to sensing of different penetration depths in the atmosphere above the layer, assuming a plane-parallel atmosphere? In other words will SPEXone or another multi-angle instrument enhance OCI’s ability to use DOAS to profile aerosol layers, or will the information content from both sensors essentially over lap? However, first we demonstrate that OCI alone has sufficient spectral resolution to discriminate between different ALHs for less aerosol loading than literature suggests. Then we will proceed with a more formal analysis of DoF for both OCI and a MAP modeled on SPEXone. The point of that exercise is to see if OCI can profile aerosol layers on its own, in addition to discriminating layer top height, and then see if merged OCI and MAP O2A observations can improve the aerosol profiling offered by OCI alone.
To begin we examine whether or not OCI will be able to discriminate ALH. The upper-left panel of Figure 4 illustrates the O2A spectrum displayed as a DOAS ratio for a single surface reflection (where surface albedo is immaterial). That is, spectral radiance normalized by the continuum value (λ = 754 nm), in this case, for nadir viewing and a solar zenith angle of 60°. Notional bandpasses for a (mono-view) hyperspectral sensor with 5 nm resolution are also shown (top) along with that of a plausible in-band MAP channel (bottom).
Figure 4. (Upper left) Synthetic spectrum of the O2 A-band at 0.05 nm resolution, normalized in DOAS-style to continuum level, for a typical airmass of 3 (e.g., sun at 60°, nadir view). An anticipated spectral coverage by the OCI hyperspectral sensor (4 channels, each 5 nm wide) is indicated above in orange, and a reasonable assumption for the single MAP channel is indicated below in blue. (Upper right) Evolution of DOAS ratios with 2% error for an airmass of 3 for the 4 hyperspectral OCI channels needed to completely cover the A-band as a function of where the 1st one starts. One good, two bad, and one average scenarios in terms of ratio separations are color-coded in green, red and orange, and the latter values are used in the lower left-hand panel. (Lower left) Reflected DOAS ratios for optically thin [AOD(O2A) = 0.1] boundary layer and lofted aerosol layer in the 0–2 km (solid) and 3–5 km (dashed) zones, respectively, assuming the sun is 60° from zenith and nadir viewing geometry. (Lower right) Bidirectional reflection function (BRF) for the MAP signal as a function of view angle. Even at typical radiometric noise levels, assumed to be 3%, the two aerosol layer elevations are easier to distinguish here than in lower-left, using instrument error magnitude as unit of signal distance. Reprinted by permission from Springer Nature: Davis and Kalashnikova (2019).
When this study was performed, the rational hypothesis is that we will have no control over how OCI will sample the O2A spectral region.1 Our operative assumption for this study is that it will be a randomly positioned overlay of adjacent 5-nm-wide channels. The upper-right panel of Figure 4 therefore shows how the oxygen DOAS ratio spectrally averaged in the upper-left panel varies as a function of where the first hyperspectral channel starts. We see that there are cases when the three values required to cover the A-band are quite widely separated; there are also cases are when two of the three values nearly coincide, thus leaving effectively only two effective O2 optical depths to use. We proceed with the simulation below using an in-between sampling where two of the three available DOAS ratios are relatively close.
In the lower-left panel of Figure 4, we used the most likely spectral sampling of the A-band by the OCI (λstart = 756.5 nm) to compute signals for two ALH s, along with the anticipated measurement error bars. These synthetic reflected spectra are for optically thin (single-scattering, AOD at the O2A band = 0.1) aerosol layers in 0–2 and 3–5 km altitude ranges. Notice the lesser absorptance (larger in-band radiance) for the elevated layer. Instrumental uncertainties at the 2% level are shown for the two DOAS signals, noting that such radiance ratios are typically more precisely known than are the individual radiances where absolute radiometric error is typically 3% or more. This is because some part of radiometry error (e.g., calibration drift) is the same across (in this case, spectral) pixels. The difference between the two spectra is clearly outside the anticipated measurement error, and we can conclude that there is sensitivity to ALH, at least at this coarse level of discrimination.
This impact of aerosol height on the measured signal will naturally be stronger as the AOD and SSA increase, with the usual tradeoff between the two aerosol optical properties (scattering and absorption). In short, if AOD and SSA are reasonably well quantified from other spectral regions/channels of OCI and/or MAP instruments, we anticipate workable retrieval accuracy for ALH from the O2A, at least sufficient to discriminate between low altitude (background and/or near sources) and high altitude (long-range transported) aerosol scenarios.
To rigorously quantify the retrievability of parameterized aerosol profile using O2A spectroscopy, supplemented or not with simultaneous multi-angle observations, we use Rodgers (2000) Bayesian formalism. Specifically, we use the non-dimensional DoF for retrieved parameter x, Ax = 1–σx2/σa,x2, where x can be either aerosol layer top pressure ptop or its pressure thickness Δp. We use σa,x to denote the “a priori” uncertainty expressed as a standard deviation that quantifies our uncertainty about the parameter before making the radiance measurements and performing the retrieval; similarly, σx denotes the “a posteriori” (post-observation/retrieval) uncertainty on the targeted parameter. The DoF varies, by definition, between 0 and 1. If Ax approaches unity, then we have considerably reduced the uncertainty on x thanks to the observation and retrieval; conversely, if Ax approaches 0, then we have gained very little new information and the uncertainty remains almost as large as the prior value. We have assumed σa,pt = 250 hPa (layer top is anywhere between, say, ≈1 to ≈6 km in altitude), and σa,Δp = 150 hPa (translates to ≈1.2 km in physical layer thickness near the surface, and ≈2.5 km at 6 km altitude). Measurement error on the DOAS ratios used in the retrieval was set at ±1.5% and error assigned to the non-retrieved (actually, otherwise-retrieved) aerosol optical thickness τa was set to ±0.025, while τa itself was set to 0.1 in accord with the simple black-surface/single-scattering forward model f(x) for any given “state vector” x = (ptop,Δp). The vector-valued function f(x) contains all the DOAS ratios across the OCI spectral channels that cover the significantly absorbing parts of the O2A (cf. Figure 4, lower-left panel), as predicted by the simplified (black-surface/single-scattering) radiative transfer model. In a separate simulation discussed in Section “Aerosol Layer Height Retrievals Using EPIC/DSCOVR’s Oxygen A- and B-band Channels,” f(x) predicts DOAS ratios for both the OCI and the MAP, assuming five angles are used in the principal plane (0o, ±30o, ±60o). In this case, the forward model f(x) is thus a function of n = 2 variables, plus another aerosol parameter (AOD) assumed known but within known uncertainty; f(x) maps these aerosol properties to DOAS ratios, formally in a vector space with either m = 3 dimensions (one for each of OCI’s A-band spectral channels). For details about how to derive σx and Ax from the above information, we refer to Rodgers (2000). For details about the adopted radiative transfer model, we refer to Davis and Kalashnikova (2019), who also summarize the required elements of optimal estimation theory from Rodgers (2000).
Figure 5 shows Ax for x = ptop (upper panels) and x = Δp (lower panels). On the left, the retrieval uses only OCI spectral data. In all cases, Ax is plotted as a function of the aerosol layer’s top pressure (horizontally) and pressure thickness (vertically). We note that there is some dependence on the latter but none on the former. We also note that much information is gained from the spectroscopy about ptop. Specifically, we have Aa,pt in the interval [0.922, 0.928], hence σa,pt in the range of 67–70 hPa. In turn, this yields an error in aerosol layer top altitude of ≈100 m for low altitude aerosol scenarios to ≈150 m for lofted layers, which is somewhat better or comparable to accuracy estimates in the above-cited literature. In contrast, Figure 5’s lower left-hand panel shows that very little information is gained about Δp since its DoF is in a very low range of 0.0690–0.0735.
Figure 5. (Upper left) Degree of freedom Apt (denoted A/d11/n) associated with aerosol layer top pressure ptop (which has index i = 1 in the state vector/bx/n) as retrieved by OCI only with a posterior uncertainty of σpt based on a prior uncertainty of σa,pt = 250 hPa; DOAS ratio measurement uncertainty is assumed to be 1.5% and aerosol optical depth is taken to be 0.1 at O2A wavelengths, with ±0.025 error. (Lower left) Same as upper-left, but for AΔp (denoted A/d22/n) associated with aerosol layer pressure thickness Δp (which has index i = 2 in the state vector/bx/n), using σa,Δp = 150 hPa. (Upper right) As for (upper left) but with additional information from collocated multi-angle observations. (Lower right) As for (upper right), but for Δp rather than ptop. Details explained in Davis and Kalashnikova (2019).
The result of these sensitivity studies shows that the top pressure, hence altitude, of an aerosol layer can be retrieved using O2A spectroscopy at the relatively modest spectral resolution (5 nm) of the OCI sensor that is planned to launch on PACE. The often-stated lower limit of ≈0.3 in AOD at 550 nm may be relaxed to ≈0.1, as long as the underlying surface is very dark, to achieve an uncertainty of ≈0.15 km on aerosol layer height.
Now the question is whether or not DOAS applied to a multi-angle instrument improves on OCI’s abilities.
Returning to Figure 4, the lower right-hand panel shows DOAS ratios for multi-angle measurements for the boundary layer (0–2 km) aerosol and its lofted (3–5 km) counterpart for a sensor with just one in-band channel, as indicated in the upper-left panel. Viewing angle is sampled in the MISR pattern (Diner et al., 1998): nadir, ±26.1°, ±45.6°, ±60°, and ±70.5°, with “ + ” meaning “fore” and “–” meaning “aft” looks that correspond respectively to forward and backward scattering in the northern hemisphere for in MISR’s descending sun-synchronous orbit. Again, the anticipated DOAS ratio measurement error of 2% is indicated, demonstrating that, here too, there is sensitivity to ALH, in addition to the one conveyed by OCI spectroscopy.
Moving on to Figure 5 where DoFs are displayed, we used a separate simulation where f(x) predicts DOAS ratios for both the OCI and the MAP, assuming five angles are used in the principal plane (0°, ±30°, ±60°). In this case, the forward model f(x) is still a function of n = 2 aerosol profile variables, plus AOD (assumed known but with known uncertainty). In this set-up, f(x) is a formal measurement vector with m = 8 dimensions (OCI’s and MAP’s A-band observations).
What happens to the above DoF assessment if the spectrometer is supplemented by a multi-angle radiometer with non-polarized O2A band channels? We assume an in-band channel as shown at the bottom of the upper left-hand panel of Figure 4, and an out-of-band/continuum channel that can combined into a DOAS ratio for each of the sampled viewing angles. The outcome is displayed in the right two panels of Figure 5 for five angles (nadir, ±30°, ±60°). We note the similarity with the left-hand panels but the ranges for the DoFs are different. In the upper panels, the range of Apt increases from [0.922, 0.928] to [0.930, 0.950], which corresponds to a modest reduction of σa,pt by ≈10%. In contrast, AΔp increases quite dramatically from [0.0690, 0.0735] to [0.09, 0.19] and, moreover, the trend of AΔp with Δp has changed direction. This range, however, is still not enough to build a credible retrieval of Δp with any confidence. Rather, we can interpret it as a gain in information that can be used to constrain somewhat better the uncertainty on Δp that, in the retrieval of its top pressure ptop, should be treated as a non-retrieved (hence necessarily prescribed but uncertain) property of the aerosol layer under consideration.
The bottom line of this theoretical exploration is that PACE will be able to discriminate different altitudes of ALH, but will not be able to profile that height, even by combining OCI with multi-angle measurements.
Oxygen A-band techniques have already been adapted to other sensors currently taking data, and results validated. For example, Xu X. et al. (2017) have retrieved ALHs over ocean surfaces using radiances measured in the oxygen A- and B-bands from the EPIC sensor on the DSCOVR satellite. EPIC measures earth-reflected solar radiances in ten narrow bands. Two of these bands are located in the oxygen A- and B-bands centered at 764 and 688 nm with bandwidth of 0.8 and 1.0 nm, respectively. Two corresponding continuum bands are placed at 680 and 780 nm. The ALH retrieval uses DOAS ratios as described above providing one piece of information by comparing an in-band value with the continuum in both the oxygen A- and B-bands, Figure 6A shows the results plotted against collocated CALIOP-measured ALH s over ocean. Most recently, Xu et al. (2018b) extended the retrieval algorithm for smoke ALH over vegetated land surfaces (Figure 6B). Assuming that CALIOP-derived aerosol extinction profiles represent an accurate representation of ALH the retrieval accuracy from EPIC’s oxygen bands was 0.45 km over ocean and 0.58 km over vegetated land surfaces. Although OCI has a spectral resolution (∼ 5 nm) coarser than the spectral width of EPIC’s oxygen A and B channels, theoretical studies show that OCI can resolve oxygen absorption signals and infer ALH. The addition of SPEXone, one of the PACE MAPs with finer spectral resolution through the O2A region, may improve the situation, but the study we have done suggests limited enhancement to OCI’s basic sensitivity from the MAP. Furthermore, SPEXone’s narrow swath width will not cover the entire OCI domain. Therefore, the ability to infer ALH from OCI-alone will be important to constrain retrievals of other aerosol parameters from the PACE broad swath instruments, including retrievals of aerosol absorption information as described in Section 3, microphysical retrievals from the other PACE polarimeter without O2A capability and will also benefit atmospheric correction for OCI, especially through the shortwave end of its spectrum.
Figure 6. Scatterplots of EPIC retrieved aerosol layer height (ALH) versus CALIOP extinction-weighted ALH. (A) For a dust plume over the Atlantic Ocean on 17 – 18 April 2016. Adapted from Xu X. et al. (2017) with permission from John Wiley and Sons. (B) For smoke plumes over Canada and Northeastern United States on 25 – 26 August 2017. Adapted from Xu et al. (2018a) with permission under Creative Commons Attribution 4.0 License. Also plotted on each panel are the one-to-one line (solid), ±0.5 km (dashed) and ±1.0 km (dotted) envelopes, the number of samples (N), bias, root-mean-square error (RMSE), and coefficient of determination (R2). On (B), color of each scatter indicates EPIC retrieved 680-nm AOD values, and error bars of each scatter indicates standard deviations for ALH values of the collocated EPIC and CALIOP pixels.
PACE offers multi-tiered opportunities for aerosol remote sensing. At the most basic level, OCI will allow continuation of heritage aerosol retrieval algorithms developed for and applied to MODIS, VIIRS and OMI. Continuation aerosol algorithms include the MODIS/VIIRS DT and Deep Blue family of algorithms and products, the OMI UV AI, the OMI UV-derived aerosol products, and new algorithms such as MODIS MAIAC. There are no foreseen barriers to porting these algorithms directly to OCI and we anticipate comparable validation results over both ocean and land surfaces. However, experience in porting aerosol retrievals from MODIS to VIIRS, or OMI to EPIC, suggest points of difficulty that will need to be addressed. Shifts in wavelengths will require new gas corrections and re-calculation of any empirical spectral relationships in the algorithm. Algorithms that depend on acquisition of statistics of surface characteristics will require time to acquire and build these new LUTs. Absence of thermal IR channels may affect cloud masking and introduce differences from heritage sensor results. Furthermore, consistency of sensor characterization will be a significant issue. Homogenizing calibration from existing sensors to new sensors, such as OCI will be necessary in order to maintain consistency across aerosol Climate Data Records. We recommend planning for collocations between OCI and other satellite sensors in the overall mission strategy. All of these recommendations are supported by first-hand experience in the transfer from MODIS to VIIRS, or in OMI to EPIC.
In addition, OCI alone offers a step up from current sensors in terms of its broad spectrum and 5 nm spectral resolution. These additional abilities show promise of obtaining new information on aerosol absorption and ALH. OCI is equivalent to merging MODIS and OMI into a single instrument and extending OMI’s spectral range into the SWIR, co-registered and with common calibration and common moderate spatial resolution. For the first time we will have UV channels available for aerosol retrievals at the same resolution as the visible-SWIR channels. Note that OMI’s spatial resolution is 13 × 24 km, and the Ozone Mapping and Profiling Suite (OMPS) is 50 km, whereas OCI’s spatial resolution is 1 km. Using the broad-spectrum retrieval we will be able to characterize aerosol absorption, layer height, AOD and a measure of particle size across the broad OCI swath, over ocean. Meanwhile, OCI’s spectral resolution of 5 nm is sufficient to resolve the signal through the oxygen-A band, and will permit independent retrievals of ALH. Independent retrievals of aerosol height from the UV and oxygen-A band offer an opportunity for redundancy. However, these advances are only possible when aerosol loading is sufficiently high, so that the aerosol signal overwhelms ocean variability. This is more important to the UV to SWIR retrieval than it is when applying O2A algorithms. We also see that oxygen-A band retrievals can be applied to multi-angle measurements that increase confidence in the OCI-alone retrieval of ALH, but still do not allow for profiling of aerosol layers.
A potential limitation to applying the UV proto-algorithm to the aerosol over global oceans is the requirement of moderate to high aerosol loading (AOD at 550 nm > 0.30). We need this requirement to be assured that the atmosphere dominates the OCI-measured signal in the UV and is not affected by variable conditions in the ocean. In testing, we found that the retrieval correctly identified input aerosol absorption 100% of the time despite perturbation to ocean spectral reflectance when AOD > 0.30, but only 56% of the time when the AOD was less. The fraction of retrievals that meet this threshold and would be available for retrieval is small, globally. According to the first 5–7 years of MODIS aerosol product over ocean, only about 10% of the 1 degree grid squares met this requirement (Remer et al., 2008). However in certain regions and seasons, namely March through May in the north Pacific, March through August in the north tropical Atlantic, and December through August in the north Indian Ocean, the seasonal regional mean AOD approaches or exceeds 0.25, suggesting a significant number of retrievals would be possible. Even in the vast areal extent of the ocean where seasonal mean AOD is near background levels, an occasional aerosol event from volcano or wildfire would likely exceed the threshold and be retrievable. These belts of persistent moderate to high aerosol loading are key regions where better characterization of aerosol absorption properties and height will constrain estimates of aerosol effects on regional circulations, cloud processes and the hydrological system (Samset et al., 2018).
Heritage and new aerosol algorithms can be developed from PACE sensor measurements for both ocean and land surfaces, and in some cases over clouds, as well. The PACE mission will be able to produce global aerosol products. However, because of the high interest in the mission’s ocean objectives, we comment now specifically on the aerosol over ocean retrieval. Heritage aerosol algorithms have served us well and “heritage plus” algorithms will continue to serve us well when applied to OCI measurements over open ocean in Case 1 waters. However, in coastal regions, where traditional assumptions of the ocean spectral signal breaks down, backscattering from suspended hydrosol particles (e.g., phytoplankton and sediment) in unanticipated spectral ranges can be misinterpreted as aerosols, leading to overestimation of AOD at low aerosol loading and interfering with new retrievals making use of the UV and hyperspectral capabilities. Furthermore, adjacency effects between water and shore also add to the complexity in near-shore regions (Bulgarelli et al., 2014). These greater challenges in complex situations require a new instrument, and that is the MAP. While not completely immune from these complex situations, the MAP increases information content, which can be exploited to better separate atmospheric from oceanic and land signals. The MAP is the subject of a companion paper in this same issue (Remer et al., 2019).
There exists strong synergy between atmosphere and ocean retrievals, for PACE. Products derived from each sensor and from each discipline will provide essential information to each other’s algorithms, constraining aerosol characteristics and ocean properties for each other, either in near real time, statistically over time, or for reprocessing. The aerosol loading limitations of the above described methods prevents their direct use for atmospheric correction over most of the world’s oceans, but does not prevent exploration of more sophisticated methods that might retrieve aerosol and ocean properties together. Simultaneous retrievals of aerosol and ocean properties, or aerosol and land properties are possible. We did not deliberate on the aerosol/land retrievals, but we did consider the possibilities of aerosol/ocean retrievals. These possibilities are discussed more fully in other papers appearing in this special issue (Chowdhary et al., 2019; Frouin et al., 2019; Remer et al., 2019).
Currently there is a severe lack of appropriate space or field data to use as a test bed for algorithm development. Hyperspectral data to simulate OCI observations can be provided by spaceborne HICO or AVIRIS, but neither cover the full spectral range of OCI, and both sensors tend to target scenes when and where aerosol loading is minimal. High altitude hyperspectral imagery that extends into the UV, and is complemented by bands in the SWIR, similar to the OCI design will be essential for preparation of the PACE mission. Such imagery should target aerosol events and various cloudy scenes, over land and ocean, as well as oceanic targets. These images will aid PACE cloud, atmospheric correction and Inherent Optical Property algorithm development, as well as aerosol algorithms. We note that maintaining calibration of such an instrument, especially in the deep blue and UV part of the spectrum is currently challenging, with commonly used sensors such as AVIRIS declaring known issues in this spectral range. Not having reliable full spectrum test data is a serious technical gap in preparing for the PACE mission. Furthermore, such instruments are rarely deployed with airborne MAPs. Existing data in cloud free conditions for with both types of instruments are extremely rare (Gao et al., 2018). We note that AVIRIS, CPL and the Airborne Multiangle SpectroPolarimetric Imager (AirMSPI: a MAP) will fly together during the FIREX-AQ campaign, which should begin to build the necessary test bed for algorithm development, but it is only a start from what will actually be needed.
The PACE mission will be an unprecedented opportunity for aerosol characterization. The combination of the broad-spectrum hyperspectral radiometer and multi-angle polarimeters, used independently or synergistically, will be able to continue heritage global aerosol remote sensing while opening up new opportunities for aerosol characterization on several levels. In addition, the PACE mission unites aerosol, cloud and ocean communities as they work towards meeting intertwined technical and research challenges. We anticipate PACE leading a surge of new understanding that crosses traditional atmosphere-ocean boundaries, leading to new science and societal benefits.
This work represents part of the overall consensus statement agreed to by the first NASA PACE Science Team and includes contributions from the Principal Investigators of the Atmospheric Correction subgroup of that Science Team, along with their associates involved with the aerosol characterization focus of that subgroup. All authors of this manuscript were part of the discussions of that subgroup and Science Team from 2014 to 2018. In addition, LR was the Deputy Lead of the Science Team and Leader of the subgroup. She organized this particular manuscript and consolidated the input from all authors. In addition she led the effort in creating the prototype broad-spectrum algorithm. SM, RL, and OT all contributed directly in obtaining the results of the heritage and broad-spectrum algorithms and writing of those sections. AD along with OK led the O2A work presented herein. KK and JC were active participants in commenting on early versions of this manuscript and helped to clarify several sections. ZA, EB, BC, OC, HD, DD, BF, RF, B-CG, AI, JM, AO, FX, and P-WZ were active participants during the years of discussion that resulted in this manuscript, and each has made contributions, edited, or commented on this manuscript.
This work was supported by the NASA Grants under the solicitation NNH13ZDA001N-PACEST. In addition, LR, SM, RL, and OT acknowledge funding from the NASA grant NNX15AD15G. AD acknowledge funding from the NASA grant NN105525. P-WZ acknowledges funding from the NASA grant 80NSSC18K0345.
AI was employed by Science Application International Inc.
The remaining authors declare that the research was conducted in the absence of any commercial or financial relationships that could be construed as a potential conflict of interest.
Portions of this work were carried out by OK, AD, and DD at the Jet Propulsion Laboratory, California Institute of Technology, under a contract with the National Aeronautics and Space Administration. JPL work was supported by the PACE science team grant, under Paula Bontempi.
AATSR, Advanced Along Track Scanning Radiometer; ABI, Advanced Baseline Imager; AERONET, Aerosol Robotics Network; AI, Aerosol Index; AirMSPI, Airborne Multiangle SpectroPolarimetric Imager; ALH, aerosol layer height; AOD, aerosol optical depth; AVIRIS Airborne Visible InfraRed Imaging Spectrometer; BRF, bidirectional reflection function; CALIOP, Cloud-Aerosol Lidar with Orthogonal Polarization; CALIPSO, Cloud-Aerosol Lidar and Infrared Pathfinder Satellite Observations; CCN, cloud condensation nuclei; CPL, Cloud Physics Lidar; DOAS, differential optical absorption spectroscopy; DoF, degrees of freedom; DSCOVR, Deep Space Climate Observatory; DT, Dark Target; EPIC, Earth Polychromatic Imaging Camera; FMF, fine mode fraction; GOES, Geostationary Operational Environmental Satellite; HICO, Hyperspectral Imager for Coastal Ocean; IN, ice nuclei; IR, InfraRed; LUT, Look Up Table; M_C6, MODIS Collection 6; MAIAC, Multi-Angle Implementation of Atmospheric Correction; MAP, multi-angle imaging polarimeters; MERIS, Medium Resolution Imaging Spectrometer; MISR, Multi-angle Imaging SpectroRadiometer; ML_M, MODIS-Like applied to MODIS inputs; ML_V, MODIS-Like applied to VIIRS inputs; MODIS, Moderate Resolution Imaging Spectroradiometer; MSI, Multi-Spectral Instrument; NASA, National Aeronautics Space Administration; NIR, Near InfraRed; O2A, oxygen A-band; OCI, Ocean Color Instrument; OCO, Orbiting Carbon Observatory; OLCI, Ocean and Land Color Instrument; OMI, Ozone Monitoring Instrument; OMPS, Ozone Mapping and Profiling Suite; PACE, Plankton, Aerosols, Clouds, Ocean Ecosystems; PARASOL, Polarization and Anisotropy of Reflectances for Atmospheric Sciences coupled with Observations from a Lidar; PM, particulate matter; POLDER, Polarization and Directionality of the Earth’s Reflectance; RMSE, Root Mean Square Error; SLSTR, Sea and Land Surface Temperature Radiometer; SPEXone, Spectro-Polarimeter for Planetary Exploration-1; SSA, single scattering albedo; SWIR, Short Wave Infra Red; TANSO-CAI, Thermal And Near infrared Sensor for Carbon Observation - Cloud and Aerosol Imager; TOA, top of atmosphere; TOMS, Total Ozone Mapping Spectrometer; TropOMI, Tropospheric Ozone Monitoring Instrument; UV, ultraviolet; VIIRS, Visible Infrared Imaging Radiometer Suite.
Albert, M. F., Anguelova, M. D., Manders, A. M., Schaap, M., and De Leeuw, G. (2016). Parameterization of oceanic whitecap fraction based on satellite observations. Atmos. Chem. Phys. 16, 13725–13751. doi: 10.5194/acp-16-13725-2016
Albert, M. F. M. A., Schaap, M., de Leeuw, G., and Builtjes, P. J. H. (2010). Progress in the determination of the sea spray source function using satellite data. J. Integr. Environ. Sci. 7, 159–166. doi: 10.1080/1943815100362146
Albrecht, B. A. (1989). Aerosols, cloud microphysics and fractional cloudiness. Science 245, 1227–1230. doi: 10.1126/science.245.4923.1227
Al-Saadi, J., Szykman, J., Pierce, R. B., Kittaka, C., Neil, D., Chu, D. A., et al. (2005). Improving national air quality forecasts with satellite aerosol observations. Bull. Am. Meteor. Soc. 86, 1249–1262. doi: 10.1175/BAMS-86-9-1249
Altaratz, O., Koren, I., Remer, L., and Hirsh, E. (2014). Review: cloud invigoration by aerosols – coupling between microphysics and dynamics. Atmos. Res. 140-141, 38–60. doi: 10.1016/j.atmosres.2014.01.009
Andreae, M. O., and Rosenfeld, D. (2008). Aerosol-cloud-precipitation interactions. Part 1. The nature and sources of cloud-active aerosols. Earth Sci. Rev. 89, 13–41. doi: 10.1016/j.earscirev.2008.03.001
Balch, W. M., Drapeau, D. T., Bowler, B. C., Lyczskowski, E., Booth, E. S., and Alley, D. (2011). The contribution of coccolithophores to the optical and inorganic carbon budgets during the southern ocean gas exchange experiment: new evidence in support of the “Great Calcite Belt” hypothesis. J. Geophys. Res. 116:C00F06.
Boucher, O., Randall, P., Artaxo, C., Bretherton, G., Feingold, P., Forster, V.-M., et al. (2013). “Clouds and aerosols,” in Proceedings of the Climate Change 2013: The Physical Science Basis. Contribution of Working Group I to the Fifth Assessment Report of the Intergovernmental Panel on Climate Change, eds T. F. Stocker, D. Qin, G.-K. Plattner, M. Tignor, S. K. Allen, J. Boschung, et al. (Cambridge: Cambridge University Press).
Bulgarelli, B., Kiselev, V., and Zibordi, G. (2014). Simulation and analysis of adjacency effects in coastal waters: a case study. Appl. Opt. 53, 1523–1545. doi: 10.1364/AO.53.001523
Cairns, B., Mishchenko, M. I., Maring, H., Fafaul, B., and Pszcolka, S. (2010). “Accurate monitoring of terrestrial aerosols and total solar irradiance: the NASA Glory mission,” in Proceedings of the SPIE Sensors, Systems, and Next-Generation Satellites XIV, (Toulouse).
Chin, M., Rood, R. B., Lin, S.-J., Mller, J.-F., and Thompson, A. M. (2000). Atmospheric sulfur cycle simulated in the global model GOCART: model description and global properties. J. Geophys. Res. 105, 24671–24687. doi: 10.1029/2000jd900384
Chowdhary, J.,Waquet, F., Knobelspiesse, K., Ottaviani, M., Redemann, J., et al. (2012). Sensitivity of multiangle, multispectral polarimetric remote sensing over open oceans to water-leaving radiance: analyses of RSP data acquired during the MILAGRO campaign. Rem. Sens. Envir. 118, 284–308. doi: 10.1016/j.rse.2011.11.003
Chowdhary, J.,Boss, E., Dierssen, H., Frouin, R., Ibrahim, A., et al. (2019). Radiative transfer in atmosphere-ocean systems. Remote. Sens. Environ. doi: 10.1016/j.rse.2011.11.003
Coddington, O. M., Vukicevic, T., Schmidt, K. S., and Platnick, S. (2017). Characterizing the information content of cloud thermodynamic phase retrievals from the notional PACE OCI shortwave reflectance measurements. J. Geophys. Res. Atmos. 122, 8079–8100. doi: 10.1002/2017JD026493
Corradini, S., and Cervino, M. (2006). Aerosol extinction coefficient profile retrieval in the oxygen A-band considering multiple scattering atmosphere. Test case: SCIAMACHY nadir simulated measurements. J. Quant. Spectrosc. Radiat. Transf. 97, 354–380. doi: 10.1016/j.jqsrt.2005.05.061
Crisp, D., Atlas, R. M., Bréon, F.-M., Brown, L. R., Burrows, J. P., Ciais, P., et al. (2004). The orbiting carbon observatory (OCO) mission. Adv. Space Res. 34, 700–709.
Davis, A. B., and Kalashnikova, O. V. (2019). “Aerosol layer height over water via O2 A-band observations from space: a tutorial,” in Springer Series in Light Scattering, vol. 3: Radiative Transfer and Light Scattering, ed. A. A. Kokhanovsky (Heidelberg: Springer), 133–166. doi: 10.1007/978-3-030-03445-0_4
Del Castillo, A., and the Pace Science Definition Team (2012). The Pre-ACE Aerosols, Clouds and Ocean Ecosystems Mission Science Definition Team Report. Available at: https://pace.oceansciences.org/docs/pace_sdt_report_final.pdf (accessed June 13, 2019).
Dierssen, H. M. (2019). Spectral characterization of whitecaps reveals new hyperspectral methods for estimating whitecap and foam in ocean color imagery. Front. Earth Sci. Atmos. Sci. 7.
Dierssen, H. M., Chlus, A., and Russell, B. (2015). Hyperspectral discrimination of floating mats of seagrass wrack and the macroalgae Sargassum in coastal waters of Greater Florida Bay using airborne remote sensing. Remote Sens. Environ. 167, 247–258. doi: 10.1016/j.rse.2015.01.027
Diner, D. J., Beckert, J. C., Reilly, T. H., Bruegge, C. J., Conel, J. E., Kahn, R. A., et al. (1998). Multi-angle imaging spectroradiometer (MISR) instrument description and experiment overview. IEEE Trans. Geosci. Remote Sens. 36, 1072–1087. doi: 10.1109/36.700992
Ding, S., Wang, J., and Xu, X. (2016). Polarimetric remote sensing in oxygen A and B bands: sensitivity study and information content analysis for vertical profile of aerosols. Atmos. Meas. Tech. 9, 2077–2092. doi: 10.5194/amt-9-2077-2016
Dubovik, O., Holben, B. N., Lapyonok, T., Sinyuk, A., Mishchenko, M. I., Yang, P., et al. (2002). Non-spherical aerosol retrieval method employing light scattering by spheroids. Geophys. Res. Lett. 29, 54-1, 54-4. doi: 10.1029/2001GL014506
Dubuisson, P., Frouin, R., Duforêt, L., Dessailly, D., Voss, K. J., and Antoine, D. (2009). Estimating the altitude of aerosol plumes over the ocean from reflectance ratio measurements in the O2 A-band. Remote Sens. Environ. 113, 1899–1911. doi: 10.1016/j.rse.2009.04.018
Eck, T. F., Holben, B. N., Reid, J. S., Arola, A., Ferrare, R. A., Hostetler, C. A., et al. (2014). Observations of rapid aerosol optical depth enhancements in the vicinity of polluted cumulus clouds. Atmos. Chem. Phys. 14, 11633–11656. doi: 10.5194/acp-14-11633-2014
European Space Agency [ESA] (2013). Sentinel-5 Precursor. Copernicus Low Earth Orbit Atmosphere Mission. Available at: http://esamultimedia.esa.int/docs/S5-prec_Data_Sheet.pdf (accessed June 13, 2019).
Fan, J., Wang, Y., Rosenfeld, D., and Liu, X. (2016). Review of aerosol-cloud interactions: mechanisms, significance and challenges. J. Atmos. Sci. 73,4221–4252. doi: 10.1175/JAS-D-16-0037.1
Feingold, G., Jiang, H. L., and Harrington, J. Y. (2005). On smoke suppression of clouds in Amazonia. Geophys. Res. Lett. 32:L02804. doi: 10.1029/2004GL021369
Fogarty, M. C., Fewings, M. R., Paget, A. C., and Dierssen, H. M. (2017). The Influence of a sandy substrate, seagrass, or highly turbid water on albedo and surface heat flux. J. Geophys. Res. Oceans 123, 53–73. doi: 10.1016/j.scitotenv.2017.06.249
Franck, U., Odeh, S., Wiedensohler, A., Wehner, B., and Herbarth, O. (2011). The effect of particle size on cardiovascular disorders – the smaller the worse. Sci. Total Environ. 409, 4217–4221. doi: 10.1016/j.scitotenv.2011.05.049
Frankenberg, C., Hasekamp, O., O’Dell, C., Sanghavi, S., Butz, A., and Worden, J. (2012). Aerosol information content analysis of multi- angle high spectral resolution measurements and its benefit for high accuracy greenhouse gas retrievals. Atmos. Meas. Tech. 5, 1809–1821. doi: 10.5194/amt-5-1809-2012
Franklin, M. O., Kalashnikova, V., and Garay, M. J. (2017). Size-resolved particulate matter concentrations derived from 4.4 km-resolution size-fractionated multi-angle imaging spectroradiometer (MISR) aerosol optical depth over Southern California. Remote Sens. Environ. 196, 312–323. doi: 10.1016/j.rse.2017.05.002
Frouin, R., Schwindling, M., and Deschamps, P.-Y. (1996). Spectral reflectance of sea foam in the visible and near-infrared: in situ measurements and remote sensing implications. J. Geophys. Res. Oceans 101, 14361–14371. doi: 10.1029/96jc00629
Frouin, R. J., Franz, B. A., Ibrahim, A., Knobelspiesse, K., Ahmad, Z., Cairns, B., et al. (2019). Atmospheric correction of satellite ocean-color imagery during the PACE Era. Front. Earth Sci. doi: 10.3389/feart.2019.00145
Gabella, M., Kisselev, V., and Perona, G. (1999). Retrieval of aerosol profile variations from reflected radiation in the oxygen absorption A band. Appl. Opt. 38, 3190–3195.
Gao, B.-C., and Davis, C. O. (1997). Development of a line-by-line-based atmosphere removal algorithm for airborne and spaceborne imaging spectrometers. SPIE Proc. 3118, 132–141.
Gao, M., Zhai, P.-W., Franz, B., Hu, Y., Knobelspiesse, K., Werdell, P. J., et al. (2018). Retrieval of aerosol properties and water-leaving reflectance from multi-angular polarimetric measurements over coastal waters. Opt. Exp. 26, 8968–8989. doi: 10.1364/OE.26.008968
Garaba, S., Aitken, J., Slat, B., Dierssen, H. M., Lebreton, L., Zielinski, O., et al. (2018). Sensing ocean plastics with an airborne hyperspectral shortwave IR imager. Env. Sci. Technol. 52, 11699–11707. doi: 10.1021/acs.est.8b02855
Garaba, S. P., and Dierssen, H. M. (2018). An airborne remote sensing case study of synthetic hydrocarbon detection using short wave infrared absorption features identified from marine-harvested macro-and microplastics. Remote Sens. Environ. 205, 224–235. doi: 10.1016/j.rse.2017.11.023
Gassó, S., and Torres, O. (2016). The role of cloud contamination, aerosol layer height and aerosol model in the assessment of the OMI near-UV retrievals over the ocean. Atmos. Meas. Tech. 9, 3031–3052. doi: 10.5194/amt-9-3031-2016
Hasekamp, O. P., Fu, G., Rusli, S. P., Wu, L., Di Noia, A., de Brugh, J., et al. (2019). Aerosol measurements by SPEXone on the NASA PACE mission: expected retrieval capabilities. J. Quant. Spectrosc. Radiat. Transf. 227, 170–184. doi: 10.1016/j.jqsrt.2019.02.006
Hebestreit, K., Stutz, J., Rosen, D., Matveiv, V., Peleg, M., Luria, M., et al. (1999). DOAS measurements of tropospheric bromine oxide in mid-latitudes. Science 283, 55–57. doi: 10.1126/science.283.5398.55
Herman, J. R., and Celarier, E. A. (1997). Earth surface reflectivity climatology at 340 nm to 380 nm from TOMS data. J. Geophys. Res. 102, 28,003–28,011.
Herman, J. R., Bhartia, P. K., Torres, O., Hsu, C., Seftor, C., and Celarier, E. (1997). Global distribution of UV-absorbing aerosols from Nimbus 7/TOMS data. J. Geophys. Res. Atmos. 102, 16911–16922. doi: 10.1029/96JD03680
Holben, B. N., Eck, T., Slutsker, I., Tanré, D., Buis, J., Setzer, A., et al. (1998). Aeronet: a federated instrument network and data archive for aerosol characterization. Rem. Sens. Environ. 66, 1–16. doi: 10.1016/s0034-4257(98)00031-5
Hsu, N. C., Jeong, M.-J., Bettenhausen, C., Sayer, A. M., Hansell, R., Seftor, C. S., et al. (2013). Enhanced deep blue aerosol retrieval algorithm: the second generation. J. Geophys. Res. Atmos. 118, 9296–9315. doi: 10.1002/jgrd.50712
Hsu, N. C., Tsay, S. C., King, M. D., and Herman, J. R. (2004). Aerosol properties over bright-reflecting source regions. IEEE Trans. Geosci. Rem. Sens. 42,557–569. doi: 10.1109/TGRS.2004.824067
Ito, T., Nenes, A., Johnson, M. S., Meskhidze, N., and Deutsch, C. (2016). Acceleration of oxygen decline in the tropical Pacific over the past decades by aerosol pollutants. Nat. Geosci. 9, 443. doi: 10.1038/ngeo2717
Jethva, H., and Torres, O. (2011). Satellite-based evidence of wavelength-dependent aerosol absorption in biomass burning smoke inferred from ozone monitoring instrument. Atmos. Chem. Phys. 11, 10541–10551. doi: 10.5194/acp-11-10541-2011
Jethva, H., Torres, O., and Ahn, C. (2014a). Global assessment of OMI aerosol single-scattering albedo using ground-based AERONET inversion. J. Geophys. Res. Atmos. 119, 9020–9040. doi: 10.1002/2014JD021672
Jethva, H., Torres, O., Waquet, F., Chand, D., and Hu, Y. (2014b). How do A-train sensors intercompare in the retrieval of above-cloud aerosol optical depth? A case study-based assessment. Geophys. Res. Lett. 41, 186–192. doi: 10.1002/2013GL058405
Jethva, H., Torres, O., Remer, L., and Bhartia, P. (2013). A color ratio method for simultaneous retrieval of aerosol and cloud optical thickness of above-cloud absorbing aerosols from passive sensors: application to MODIS measurements. IEEE Trans. Geosci. Rem. Sens. 51, 3862–3870. doi: 10.1109/TGRS.2012.2230008
Jethva, H., Torres, O., Remer, L., Redemann, J., and Livingston, J. (2016). Validating MODIS above-cloud aerosol optical depth retrieved from “color ratio” algorithm using direct measurements made by NASA’s airborne AATS and 4STAR sensors. Atmos. Meas. Tech 9, 5053–5062. doi: 10.5194/amt-9-5053-2016
Jickells, T. D., An, Z. S., and Andersen, K. K. (2005). Global iron connections between desert dust, ocean biogeochemistry, and climate. Science 308, 67–71. doi: 10.1126/science.1105959
Johnson, B. T., Shine, K. P., and Forster, F. M. (2004). The semi-direct aerosol effect: impact of absorbing aerosols on marine stratocumulus. Q. J. R. Meteorol. Soc. 130, 1407–1422. doi: 10.1256/qj.03.61
Kahn, R. A., and Gaitley, B. J. (2015). An analysis of global aerosol type as retrieved by MISR. J. Geophys. Res. Atmos. 120, ,4248–4281. doi: 10.1002/2015JD02332
Kalashnikova, O. V., Garay, M. J., Davis, A. B., Diner, D. J., and Martonchik, J. V. (2011). Sensitivity of multi-angle photo-polarimetry to vertical layering and mixing of absorbing aerosols: quantifying measurement uncertainties. J. Quat. Spectrosc. Radiat. Trans. 112, 2149–2163. doi: 10.1016/j.jqsrt.2011.05.010
Kalashnikova, O. V., Garay, M. J., Martonchik, J. V., and Diner, D. J. (2013). MISR dark water aerosol retrievals: operational algorithm sensitivity to particle non-sphericity. Atmos. Meas. Tech. 6, 2131–2154. doi: 10.5194/amt-6-2131-2013
Kalashnikova, O. V., and Kahn, R. (2006). Ability of multiangle remote sensing observations to identify and distinguish mineral dust types: part 2. Sensitivity over dark water. J. Geophys. Res. 111, D11207. doi: 10.1029/2005JD00675
Kaufman, Y. J., and Koren, I. (2006). Smoke and pollution aerosol effect on cloud cover. Science 313, 655–658. doi: 10.1126/science.1126232
Kaufman, Y. J., Koren, I., Remer, L. A., Rosenfeld, D., and Rudich, Y. (2005a). The effect of smoke, dust and pollution aerosol on shallow cloud development over the atlantic ocean. Proc. Nat. Acad. Sci. U.S.A. 102, 11207–11212. doi: 10.1073/pnas.0505191102
Kaufman, Y. J., Koren, I., Remer, L. A., Tanré, D., Ginoux, P., and Fan, S. (2005b). Dust transport and deposition observed from the Terra-MODIS spacecraft over the Atlantic Ocean. J. Geophys. Res. 110:D10S12. doi: 10.1029/2003JD004436
Kaufman, Y. J., and Nakajima, T. (1993). Effect of Amazon smoke on cloud microphysics and albedo. J. Appl. Meteor. 32, 729–744. doi: 10.1175/1520-0450(1993)032<0729:eoasoc>2.0.co;2
Khain, A., Rosenfeld, D., and Pokrovsky, A. (2005). Aerosol impact on the dynamics and microphysics of deep convective clouds. Q. J. R. Meteorol. Soc. 131, 2639–2663. doi: 10.1256/qj.04.62
Knobelspiesse, K., Cairns, B., Mishchenko, M., Chowdhary, J., Tsigaridis, K., van Diedenhoven, B., et al. (2012). Analysis of fine-mode aerosol retrieval capabilities by different passive remote sensing instrument designs. Opt. Exp. 20, 21457–21484. doi: 10.1364/OE.20.021457
Knobelspiesse, K. D., Pietras, C., Fargion, G. S., Wang, M., Frouin, R., Miller, M. A., et al. (2004). Maritime aerosol optical thickness measured by handheld sun photometers. Remote Sens. Environ. 93, 87–106. doi: 10.1016/j.rse.2004.06.018
Kokhanovsky, A. A. (2015). The modern aerosol retrieval algorithms based on the simultaneous measurements of the intensity and polarization of reflected solar light: a review. Front. Environ. Sci. 3:4. doi: 10.3389/fenvs.2015.00004
Kokhanovsky, A. A., Deuzé, J. L., Diner, D. J., Dubovik, O., Ducos, F., Emde, C., et al. (2010). The inter-comparison of major satellite aerosol retrieval algorithms using simulated intensity and polarization characteristics of reflected light. Atmos. Meas. Tech. 3, 909–932. doi: 10.5194/amt-3-909-2010
Kokhanovsky, A. A., and Rozanov, V. V. (2010). The determination of dust cloud altitudes from a satellite using hyperspectral measurements in the gaseous absorption band. Int. J. Remote Sens. 31, 2729–2744. doi: 10.1080/01431160903085644
Koren, I., Altaratz, O., Remer, L. A., Feingold, G., Martins, J. V., and Heiblum, R. H. (2012). Aerosol-induced intensification of rain from the tropics to the mid-latitudes. Nat. Geosci. 5, 118–122. doi: 10.1038/ngeo1364
Koren, I., Kaufman, Y. J., Remer, L. A., and Martins, J. V. (2004). Measurement of the effect of amazon smoke on inhibition of cloud formation. Science 303, 1342–1345. doi: 10.1126/science.1089424
Koren, I., Kaufman, Y. J., Rosenfeld, D., Remer, L. A., and Rudich, Y. (2005). Aerosol invigoration and restructuring of Atlantic convective clouds. Geophys. Res. Lett. 32:LI4828. doi: 10.1029/2005GL023187
Koren, I., Martins, J. V., Remer, L. A., and Afargan, H. (2008). Smoke invigoration versus inhibition of clouds over the amazon. Science 321, 946–949. doi: 10.1126/science.1159185
Koren, I., Remer, L. A., Altaratz, O., Martins, J. V., and Davidi, A. (2010). Aerosol-induced changes of convective cloud anvils produce strong climate warming. Atmos. Chem. Phys. 10, 5001–5010. doi: 10.5194/acp-10-5001-2010
Levy, R. C., Mattoo, S., Munchak, L. A., Remer, L. A., Sayer, A. M., Patadia, F., et al. (2013). The Collection 6 MODIS aerosol products over land and ocean. Atmos. Meas. Tech. 6, 2989–3034. doi: 10.5194/amt-6-2989-2013
Levy, R. C., Munchak, L. A., Mattoo, S., Patadia, F., Remer, L. A., and Holz, R. E. (2015). Towards a long-term global aerosol optical depth record: applying a consistent aerosol retrieval algorithm to MODIS and VIIRS-observed reflectance. Atmos. Meas. Tech. 8, 4083–4110. doi: 10.5194/amt-8-4083-2015
Li, R.-R., Kaufman, Y. J., Gao, B.-C., and Davis, C. O. (2003). Remote sensing of suspended sediments and shallow coastal waters. IEEE Trans. Geosci. Remote Sens. 41, 559–566. doi: 10.1007/s10661-017-5905-7
Li, Z., Niu, F., Fan, J., Liu, Y., Rosenfeld, D., and Ding, Y. (2011). Long-term impacts of aerosols on the vertical development of clouds and precipitation. Nat. Geosci. 4, 888–894. doi: 10.1038/ngeo1313
Li, Z., Zhao, X., Kahn, R., Mishchenko, M., Remer, L., Lee, K.-H., et al. (2009). Uncertainties in satellite remote sensing of aerosols and impact on monitoring its long-term trend: a review and perspective. Ann. Geophys. 27, 2755–2770. doi: 10.5194/angeo-27-2755-2009
Lim, S. S., Vos, T., and Flaxman, A. D. (2012). A comparative risk assessment of burden of disease and injury attributable to 67 risk factors and risk factor clusters in 21 regions, 1990-2010: a systematic analysis for the Global Burden of Disease Study 2010. Lancet 380, 2224–2260. doi: 10.1016/S0140-6736(12)61766-8
Lyapustin, A., Wang, Y., Laszlo, I., Kahn, R., Korkin, S., Remer, L., et al. (2011). Multiangle implementation of atmospheric correction (MAIAC): 2. Aerosol algorithm. J. Geophys. Res. 116:D03211. doi: 10.1029/2010JD014986
Marmorino, G. O., and Smith, G. B. (2005). Bright and dark ocean whitecaps observed in the infrared. Geophys. Res. Lett. 32:L11604. doi: 10.1029/2005GL023176
Marshak, A., Herman, J., Adam, S., Karin, B., Carn, S., Cede, A., et al. (2018). Earth observations from DSCOVR EPIC Instrument. Bull. Amer. Meteor. Soc. 99, 1829–1850. doi: 10.1175/BAMS-D-17-0223.18
Meyer, K. G., and Platnick, S. E. (2015). Simultaneously inferring above-cloud absorbing aerosol optical thickness and underlying liquid phase cloud optical and microphysical properties using MODIS. J. Geophys. Res. Atmos 120,5524–5547. doi: 10.1002/2015JD023128
Min, Q., and Harrison, L. C. (2004). Retrieval of atmospheric optical depth profiles from downward-looking high-resolution O2 A-band measurements: optically thin conditions. J. Atmos. Sci. 61, 2469–2477. doi: 10.1175/1520-0469(2004)061<2469:roaodp>2.0.co;2
Mishchenko, M. I., Cairnsa, B., Hansen, J. E., Travis, L. D., Burgc, R., Kaufman, Y. J., et al. (2004). Monitoring of aerosol forcing of climate from space: analysis of measurement requirements. J. Quant. Spectrosc. Radiat. Transf. 88, 149–161. doi: 10.1016/j.jqsrt.2004.03.030
Morrison, H., and Grabowski, W. W. (2011). Cloud-system resolving model simulations of aerosol indirect effects on tropical deep convection and its thermodynamic environment. Atmos. Chem. Phys. 11, 10503–10523. doi: 10.5194/acp-11-10503-2011
Myhre, G., Bréon, F.-M., Collins, W., Fuglestvedt, J., Huang, J., Koch, D., et al. (2013). “Anthropogenic and natural radiative forcing,” in Proceedings of the Climate Change 2013: The Physical Science Basis. Contribution of Working Group I to the Fifth Assessment Report of the Intergovernmental Panel on Climate Change, eds T. F. Stocker, D. Qin, G.-K. Plattner, M. Tignor, S. K. Allen, J. Boschung, et al. (Cambridge: Cambridge University Press).
Pelletier, B., Frouin, R., and Dubuisson, P. (2008). “Retrieval of the aerosol vertical distribution from atmospheric radiance,” in Proceedings of the SPIE, (Noumea), 71501R.
Perry, R. A., Vaudrey, J. M., and Dierssen, H. M. (2018). Long range transport and carbon and nitrogen dynamics of floating seagrass wracks in Greater Florida Bay. Estuar. Coast. Shelf Sci. 209, 7–17. doi: 10.1016/j.ecss.2018.05.006
Remer, L. A., Kaufman, Y. J., Tanré, D., Mattoo, S., Chu, D. A., Martins, J. V., et al. (2005). The MODIS aerosol algorithm, products, and validation. Atmos. J. Sci. 62, 947–973.
Remer, L. A., Kleidman, R. G., Levy, R. C., Kaufman, Y. J., Tanré, D., Mattoo, S., et al. (2008). Global aerosol climatology from the MODIS satellite sensors. J. Geophys. Res.: Atmos. 113. doi: 10.1029/2007JD009661
Remer, L. A., Knobelspiesse, K., Zhai, P.-W., Xu, F., Kalashnikova, O. V., Chowdhary, J., et al. (2019). Retrieving aerosol characteristics from the PACE mission, Part 2: multi-angle and polarimetry. Front. Earth Sci. doi: 10.3389/fenvs.2019.00094
Rodgers, C. D. (2000). Inverse Methods for Atmospheric Sounding: Theory and Practice. Singapore: World Scientific.
Rosenfeld, D., Lohmann, U., Raga, G. B., O’Dowd, C. D., Kulmala, M., Fuzziet, S., et al. (2008). Flood or drought: how do aerosols affect precipitation? Science 321, 1309–1313. doi: 10.1126/science.1160606
Rosenfeld, D., Sherwood, S., Wood, R., and Donner, L. (2014b). Climate effects of aerosol-cloud interactions. Science 343, 379–380. doi: 10.1126/science.1247490
Rosenfeld, D., Andreae, A., and Asmi, A. (2014a). Global observations of aerosol-cloud-precipitation-climate interactions. Rev. Geophys. 52, 750–808. doi: 10.1002/2013RG000441
Samset, B. H., Stjern, C. W., and Andrews, E. (2018). Aerosol absorption: progress towards global and regional constraints. Curr. Clim. Change Rep. 4:65. doi: 10.1007/s40641-018-0091-4
Sanghavi, S., Martonchik, J. V., Landgraf, J., and Platt, U. (2012). Retrieval of the optical depth and vertical distribution of particulate scatterers in the atmosphere using OA- and B-band SCIAMACHY observations over Kanpur: a case study. Atmos. Meas. Tech. 5, 1099–1119. doi: 10.5194/amt-5-1099-2012
Satheesh, S. K., Torres, O., Remer, L. A., Suresh Babu, S., Vinoj, V., Eck, T. F., et al. (2009). Improved assessment of aerosol absorption using OMI-MODIS joint retrieval. J. Geophys. Res. 114:D05209. doi: 10.1029/2008JD011024
Sayer, A. M., Hsu, N. C., Bettenhausen, C., Lee, J., Redemann, J., Schmid, B., et al. (2016). Extending “Deep Blue” aerosol retrieval coverage to cases of absorbing aerosols above clouds: sensitivity analysis and first case studies. J. Geophys. Res. Atmos. 121, 4830–4854. doi: 10.1002/2015JD024729
Seinfeld, J. H., Bretherton, C., Carslaw, K. S., Coe, H., DeMott, P. J., Dunlea, E. J., et al. (2016). Improving our fundamental understanding of the role of aerosol- cloud interactions in the climate system. Proc. Nat. Acad. Sci. U.S.A. 113, 5781–5790. doi: 10.1073/pnas.1514043113
Shi, J. J., Matsui, T., Tao, W.-K., Peters-Lidard, C., Chin, M., Tan, Q., et al. (2014). Implementation of an aerosol-cloud microphysics-radiation coupling into the NASA Unified WRF: simulation results for the 6-7 August 2006 AMMA special observing period. Quart. J. R. Meteor. Soc. 140, 2158–2175. doi: 10.1002/qj.2286
Smirnov, A., Holben, B. N., Slutsker, I., Giles, D. M., McClain, C. R., Eck, T. F., et al. (2009). Maritime aerosol network as a component of aerosol robotic network. J. Geophys. Res. Atmos. 114:D06204.
Stamnes, S., Hostetler, C., Ferrare, R., Burton, S., Liu, X., Hair, J., et al. (2018). Simultaneous polarimetric retrievals of microphysical aerosol and ocean color parameters from the “MAPP” algorithm with comparison to high spectral resolution lidar aerosol and ocean products. Appl. Opt. 57, 2394–2413. doi: 10.1364/AO.57.002394
Stevens, B., and Feingold, G. (2009). Untangling aerosol effects on clouds and precipitation in a buffered system. Nature 461, 607–613. doi: 10.1038/nature08281
Tanré, D., Herman, M., and Kaufman, Y. J. (1996). Information on aerosol size distribution contained in solar reflected spectral radiances. Geophys. J. Res. 101, 43–19.
Tanré, D., Kaufman, Y. J., Herman, M., and Mattoo, S. (1997). Remote sensing of aerosol properties over oceans using the MODIS/EOS spectral radiances. J. Geophys. Res. 102, 971–916.
Tao, W. K., Chen, J.-P., Li, Z., Wang, C., and Zhang, C. (2012). Impact of aerosols on convective clouds and precipitation. Rev. Geophys. 50:RG2001. doi: 10.1029/2011RG000369
Torres, O., Ahn, C., and Chen, Z. (2013). Improvements to the OMI near-UV aerosol algorithm using A-train CALIOP and AIRS observations. Atmos. Meas. Tech. 6, 3257–3270. doi: 10.5194/amt-6-3257-2013
Torres, O., Bhartia, P. K., Herman, J. R., Ahmad, Z., and Gleason, J. (1998). Derivation of aerosol properties from satellite measurements of backscattered ultraviolet radiation: theoretical basis. Geophys. J. Res. 103, 99–17.
Torres, O., Bhartia, P. K., Herman, J. R., Sinyuk, A., and Holben, B. (2002). A long term record of aerosol optical thickness from TOMS observations and comparison to AERONET measurements. Atmos. J. Sci. 59, 398–413. doi: 10.1175/1520-0469(2002)059<0398:altroa>2.0.co;2
Torres, O., Bhartia, P. K., Syniuk, A., and Welton, E. (2005). TOMS Measurements of aerosol absorption from space: comparison to SAFARI 2000 ground based observations. Geophys. J. Res. 110, D10S18. doi: 10.10129/2004JD004611
Torres, O., Jethva, H., and Bhartia, P. K. (2012). Retrieval of aerosol optical depth above clouds from omi observations: sensitivity analysis and case studies. J. Atmos. Sci. 69, 1037–1053. doi: 10.1175/JAS-D-11-0130.1
Torres, O., Tanskanen, A., Veihelman, B., Ahn, C., Braak, R., Bhartia, P. K., et al. (2007). Aerosols and surface UV products from OMI observations: an overview. Geophys. J. Res. 112, D24S47. doi: 10.1029/2007JD008809
Twomey, S. (1977). Influence of pollution on shortwave albedo of clouds. Atmos. J. Sci. 34, 1149–1152. doi: 10.1175/1520-0469(1977)034<1149:tiopot>2.0.co;2
Van den Heever, S. C., Stephens, G. L., and Wood, N. B. (2011). Aerosol indirect effects on tropical convection characteristics under conditions of radiative convective equilibrium. Atmos. J. Sci. 68, 699–718. doi: 10.1175/2010JAS3603.1
Waquet, F., Cairns, B., Knobelspiese, K., Chowdhary, J., Travis, L. D., Schmid, B., et al. (2009). Polarimetric remote sensing of aerosols over land. Geophys. J. Res. 114:D01206. doi: 10.1029/2008JD010619
Wu, L., Hasekamp, O., van Diedenhoven, B., Cairns, B., Yorks, J. E., and Chowdhary, J. (2016). Passive remote sensing of aerosol layer height using near-UV multi-angle polarization measurements. Geophys. Res. Lett. 43, 8783–8790. doi: 10.1002/2016GL069848
Xu, F., Dubovik, O., Zhai, P.-W., Diner, D. J., Kalashnikova, O. V., Seidel, F. C., et al. (2016). Joint retrieval of aerosol and water-leaving radiance from multispectral, multiangular and polarimetric measurements over ocean. Atmos. Meas. Tech. 9, 2877–2907. doi: 10.5194/amt-9-2877-2016
Xu, F., van Harten, G., Diner, D. J., Kalashnikova, O. V., Seidel, F. C., Bruegge, C. J., et al. (2017). Coupled retrieval of aerosol properties and land surface reflection using the airborne multiangle spectropolarimetric imager (AirMSPI). J. Geophys. Res. Atmos. 122, 7004–7026. doi: 10.1002/2017JD026776
Xu, X., Wang, J., Wang, Y., Zeng, J., Torres, O., Yang, Y., et al. (2017). Passive remote sensing of altitude and optical depth of dust plumes using the oxygen A and B bands: first results from EPIC/DSCOVR at Lagrange-1 point. Geophys. Res. Lett. 44, 7544–7554. doi: 10.1002/2017GL073939
Xu, X., Wang, J., Wang, Y., Zeng, J., Torres, O., Reid, J. S., et al. (2018b). Detecting layer height of smoke aerosols over vegetated land and water surfaces via oxygen absorption bands: hourly results from EPIC/DSCOVR satellite in deep space. Atmos. Meas. Tech.
Xu, X., Wang, J., Wang, Y., and Kokhanovsky, A. (2018a). “Passive remote sensing of aerosol height,” in Remote Sensing of Aerosols, Clouds, and Precipitation, ed. T. Islam (Amsterdam: Elsevier), 1–22. doi: 10.1016/b978-0-12-810437-8.00001-3
Yu, H., Chin, M., Yuan, T. L., Bian, H., Remer, L. A., Prospero, J. M., et al. (2015). The fertilizing role of African dust in the Amazon rainforest: a first multiyear assessment based on data from cloud-aerosol lidar and infrared pathfinder satellite observations. Geophys. Res. Lett. 42, 1984–1991. doi: 10.1002/2015GL063040
Yu, H., Remer, L. A., Chin, M., Bian, H., Tan, Q., Yuan, T., et al. (2012). Aerosols from overseas rival domestic emissions over North America. Science 337, 566–569. doi: 10.1126/science.1217576
Yu, H., Remer, L. A., Kahn, R. A., Chin, M., and Zhang, Y. (2013). Satellite perspective of aerosol intercontinental transport: from qualitative tracking to quantitative characterization. Atmos. Res. 124, 73–100. doi: 10.1016/j.atmosres.2012.12.013
Yuan, T., Remer, L., and Yu, H. (2011). Microphysical, macrophysical and radiative signatures of volcanic aerosols in trade wind cumulus observed by the A-Train. Atmos. Chem. Phys. 11, 7119–7132. doi: 10.5194/acp-11-7119-2011
Yuan, T., Remer, L. A., Bian, H., Ziemke, J. R., Albrecht, R., Pickering, K. E., et al. (2012). Aerosol indirect effect on tropospheric ozone via lightning. J. Geophys. Res. 117, D18212. doi: 10.1029/2012JD017723
Keywords: aerosol, oxygen A-band, hyperspectral, PACE, remote sensing, UV
Citation: Remer LA, Davis AB, Mattoo S, Levy RC, Kalashnikova OV, Coddington O, Chowdhary J, Knobelspiesse K, Xu X, Ahmad Z, Boss E, Cairns B, Dierssen HM, Diner DJ, Franz B, Frouin R, Gao B-C, Ibrahim A, Martins JV, Omar AH, Torres O, Xu F and Zhai P-W (2019) Retrieving Aerosol Characteristics From the PACE Mission, Part 1: Ocean Color Instrument. Front. Earth Sci. 7:152. doi: 10.3389/feart.2019.00152
Received: 30 November 2018; Accepted: 03 June 2019;
Published: 23 July 2019.
Edited by:
David Antoine, Curtin University, AustraliaReviewed by:
Howard R. Gordon, University of Miami, United StatesCopyright © 2019 Remer, Davis, Mattoo, Levy, Kalashnikova, Coddington, Chowdhary, Knobelspiesse, Xu, Ahmad, Boss, Cairns, Dierssen, Diner, Franz, Frouin, Gao, Ibrahim, Martins, Omar, Torres, Xu and Zhai. This is an open-access article distributed under the terms of the Creative Commons Attribution License (CC BY). The use, distribution or reproduction in other forums is permitted, provided the original author(s) and the copyright owner(s) are credited and that the original publication in this journal is cited, in accordance with accepted academic practice. No use, distribution or reproduction is permitted which does not comply with these terms.
*Correspondence: Lorraine A. Remer, cmVtZXJAdW1iYy5lZHU=
Disclaimer: All claims expressed in this article are solely those of the authors and do not necessarily represent those of their affiliated organizations, or those of the publisher, the editors and the reviewers. Any product that may be evaluated in this article or claim that may be made by its manufacturer is not guaranteed or endorsed by the publisher.
Research integrity at Frontiers
Learn more about the work of our research integrity team to safeguard the quality of each article we publish.