- 1Department of Natural Resources and the Environment, University of New Hampshire, Durham, NH, United States
- 2Department of Earth and Environmental Science, University of Pennsylvania, Philadelphia, PA, United States
- 3U.S. Geological Survey, Montpelier, VT, United States
Concentration-discharge relationships are a key tool for understanding the sources and transport of material from watersheds to fluvial networks. Storm events in particular provide insight into variability in the sources of solutes and sediment within watersheds, and the hydrologic pathways that connect hillslope to stream channel. Here we examine high-frequency sensor-based specific conductance and turbidity data from multiple storm events across two watersheds (Quebrada Sonadora and Rio Icacos) with different lithology in the Luquillo Mountains of Puerto Rico, a forested tropical ecosystem. Our analyses include Hurricane Maria, a category 5 hurricane. To analyze hysteresis, we used a recently developed set of metrics to describe and quantify storm events including the hysteresis index (HI), which describes the directionality of hysteresis loops, and the flushing index (FI), which can be used to infer whether the mobilization of material is source or transport limited. We also examine the role of antecedent discharge to predict hysteretic behavior during storms. Overall, specific conductance and turbidity showed contrasting responses to storms. The hysteretic behavior of specific conductance was similar across sites, displaying clockwise hysteresis and a negative FI indicating proximal sources of solutes and consistent source limitation. In contrast, the directionality of turbidity hysteresis was significantly different between watersheds, although both had strong flushing behavior indicative of transport limitation. Overall, models that included antecedent discharge did not perform any better than models with peak discharge alone, suggesting that the magnitude and trajectory of an individual event was the strongest driver of material flux and hysteretic behavior. Hurricane Maria produced unique hysteresis metrics within both watersheds, indicating a distinctive response to this major hydrological event. The similarity in response of specific conductance to storms suggests that solute sources and pathways are similar in the two watersheds. The divergence in behavior for turbidity suggests that sources and pathways of particulate matter vary between the two watersheds. The use of high-frequency sensor data allows the quantification of storm events while index-based metrics of hysteresis allow for the direct comparison of complex storm events across a heterogeneous landscape and variable flow conditions.
Introduction
Concentration-discharge (C-Q) relationships provide fundamental insight into the mobilization and export of solutes and sediment from watersheds (Chorover et al., 2017). C-Q relationships offer an integrated signal representing the combined effects of sources, flow paths, biogeochemical cycles, and watershed state-factors such as lithology, soil type, and climate on stream solute and sediment concentrations. Because storm events are responsible for a disproportionate amount of material transfer compared to base flow (Inamdar et al., 2006; Fellman et al., 2009), they are particularly important in estimation of annual fluxes. Developing general models of solute and sediment behavior across a range of flow conditions would thus provide insights into watershed function as well as valuable guidance for sampling regimes that will most efficiently capture the impacts of large events. The study of C-Q relationships from individual events is challenging as individual events can be highly complex. Difficulty in interpreting C-Q patterns from individual storm events results from their spatial and temporal variability, their inherently ephemeral nature, and the challenges associated with traditional sampling approaches (e.g., grab samples) during short-term pulse events.
Recent advancements in the development of in situ sensors offer the opportunity to collect temporally high-resolution data that can be used to describe storm events including those in remote locations. High-frequency data are particularly useful for understanding the hysteretic behavior of storm events. Solutes and sediments often display “hysteresis-loops” and the width, magnitude, and direction of these loops provide insight into how reservoirs of solutes and sediments are stored within the hillslope and exported to fluvial networks (Gellis, 2013; Koenig et al., 2017; Vaughan et al., 2017; Olshansky et al., 2018; Rose et al., 2018). Hysteresis occurs when concentrations at a given discharge differ on the rising and falling limbs of the hydrograph. Clockwise hysteresis is interpreted to reflect proximal and rapidly mobilized sources; whereas counterclockwise hysteresis reflects sources that are either proximal to the stream channel with slow travel times or those that are distal to the stream channel. The slope of the line describing C-Q relationships can be positive where the delivery of material is considered transport limited (flushing behavior), negative where the export of material is considered source limited (dilution behavior), or chemostatic where concentrations are independent of discharge (Evans and Davies, 1998; Godsey et al., 2009). Storm events can display complex hysteresis due to variability in the spatial and temporal patterns of precipitation and runoff during a single event (Williams, 1989), which can make comparison among events difficult. Also contributing to this variability and intra-storm complexity is antecedent moisture condition. For example, storms under dry conditions can mobilize solutes rapidly and promote clockwise hysteresis (Ávila et al., 1992; Biron et al., 1999). These events tend to deplete reservoirs of solutes and sediment from both the hillslope and stream channel, causing weaker C-Q relationships during the subsequent storm (McDowell and Asbury, 1994). On the other hand, high antecedent moisture increases connectivity, which expands the contributing area for sediment and solutes to more distal parts of the catchment, promoting counterclockwise hysteresis (Seeger et al., 2004). High-frequency sensor-based data can help provide detailed insights into the complexity of storm events.
Novel quantitative approaches are also providing new ways to describe hysteresis loops. As opposed to simply relying on the slope of the regression line in log(C)–log(Q) space, studies are increasingly incorporating an indexed-based approach to quantify event-based hysteretic behavior (Lawler et al., 2006; Lloyd et al., 2016; Zuecco et al., 2016; Vaughan et al., 2017). One of the strengths of an indexed approach is that it can be used to quantify C-Q relationships at multiple time-points during an individual storm event. This allows for a robust quantification of the storm’s central tendency (e.g., mean width of hysteresis loop) and variability, while facilitating the comparison of events across space and time. One such approach is the hysteresis index (HI) proposed by Lloyd et al. (2016) and modified by Vaughan et al. (2017). HI uses normalized concentration and discharge values (Lawler et al., 2006; Lloyd et al., 2016) to enumerate differences on the rising and falling limbs of the hydrograph. HI values range between −1 and 1, with negative and positive values representing counterclockwise and clockwise hysteresis, respectively. The magnitude of HI (i.e., width of loop) thus describes the difference in concentration between the rising and falling limb at points of equal discharge. Vaughan et al. (2017) further enhanced these index-based analyses by combining HI with a “flushing index” (FI), which compares normalized concentrations at the beginning of the storm and at peak discharge, thereby providing further description of solute and sediment behavior and delivery to the stream channel. Here we use the term “normalized slope” (NS) to describe FI and to avoid confusion with other FI terminology common in the stream biogeochemistry literature (e.g., McKnight et al., 2001) and to better capture the fact that this metric can be used to describe both flushing and diluting behavior. Normalized slope values also range between −1 and 1 with positive values representing a flushing response and negative values representing a dilution response.
In this study we used high-frequency data collected with specific conductance and turbidity sensors to better understand how variation in watershed characteristics across a heterogeneous tropical landscape affects the mobilization of material during storm events. One framework within which to consider spatial variation is the Critical Zone (CZ). The CZ is defined as the layer of the Earth’s surface from the lowest extent of freely circulating groundwater to the top of vegetative canopy (National Research Council [NRC], 2001). As such, the CZ incorporates lithology, regolith and soil, all terrestrial biota, and topography, providing a predictive framework for understanding the relationship between watershed structure and function (e.g., Wymore et al., 2017). Watersheds across the Luquillo Mountains, Puerto Rico, provide an ideal laboratory to examine how the structure of the CZ affects the export of material in a tropical landscape as affected by variations in lithology, forest composition, and topography while experiencing a similar climatic regime. It is this CZ template, especially lithology, that influences where material is stored and how flow paths are distributed both laterally and vertically. We analyzed storm-generated hysteresis loops using the indices described by Lloyd et al. (2016) and Vaughan et al. (2017) in an effort to understand where solutes and sediment are stored within our study watersheds and the role of storms in mobilizing and exporting this material. We analyzed data from 46 unique hydrological events including a major category 5 hurricane.
We hypothesized that each of our study watersheds would have unique hysteretic behavior in response to storms and that these responses would be associated with lithology and would be similar to their long-term C-Q relationships. In the Luquillo Mountains, lithology exerts control on the long-term C-Q relationships of multiple solutes (McDowell and Asbury, 1994; Shanley et al., 2011; Stallard and Murphy, 2012; Gellis, 2013; Wymore et al., 2017) primarily by affecting weathering rates, hydrological flow paths and spatial variation in reservoirs of solutes and sediment (Shanley et al., 2011; Wymore et al., 2017). For example, in the volcaniclastic terrain of the Luquillo Mountains apatite weathering is the primary control on phosphate C-Q behavior, while biotic processes driven by nutrient limitation (Chadwick and Asner, 2016) influence the solute’s C-Q behavior in the granitic watershed (Wymore et al., 2017). We also expected that hysteresis metrics (HI and NS) would correlate with the magnitude of the storm (measured as peak Q) and that antecedent discharge would add predictive power to these models (Gellis, 2013). Quantitative and predictive frameworks that describe C-Q relationships across tropical watersheds are important because the tropics are understudied compared to temperate watersheds, despite the fact that they provide a disproportionate contribution to the global flux of solutes and sediment to the oceans (Hilton et al., 2008; Gellis, 2013; Schlesinger and Bernhardt, 2013). Understanding C-Q relationships and the associated drivers provides key information to resource managers who can benefit from predictive frameworks that describe how watersheds respond to disturbance events.
Materials and Methods
Site Description
Study watersheds were located in the tropical Luquillo Mountains of northeastern Puerto Rico (Figure 1). The Luquillo Mountains are warm and wet, with a mean annual temperature of 20°C and mean annual precipitation varying between 2500 and 4500 mm as a function of elevation (Garcia-Martino et al., 1996; McDowell et al., 2012; Murphy et al., 2017). Our two study watersheds, Quebrada Sonadora (QS) and Rio Icacos (RI), vary in lithology (volcaniclastic rocks at QS and quartz diorite at RI), but have similar vegetation, elevation, and slope. A primary difference besides geology is the considerably higher rainfall and annual runoff at RI (McDowell and Asbury, 1994; Table 1).
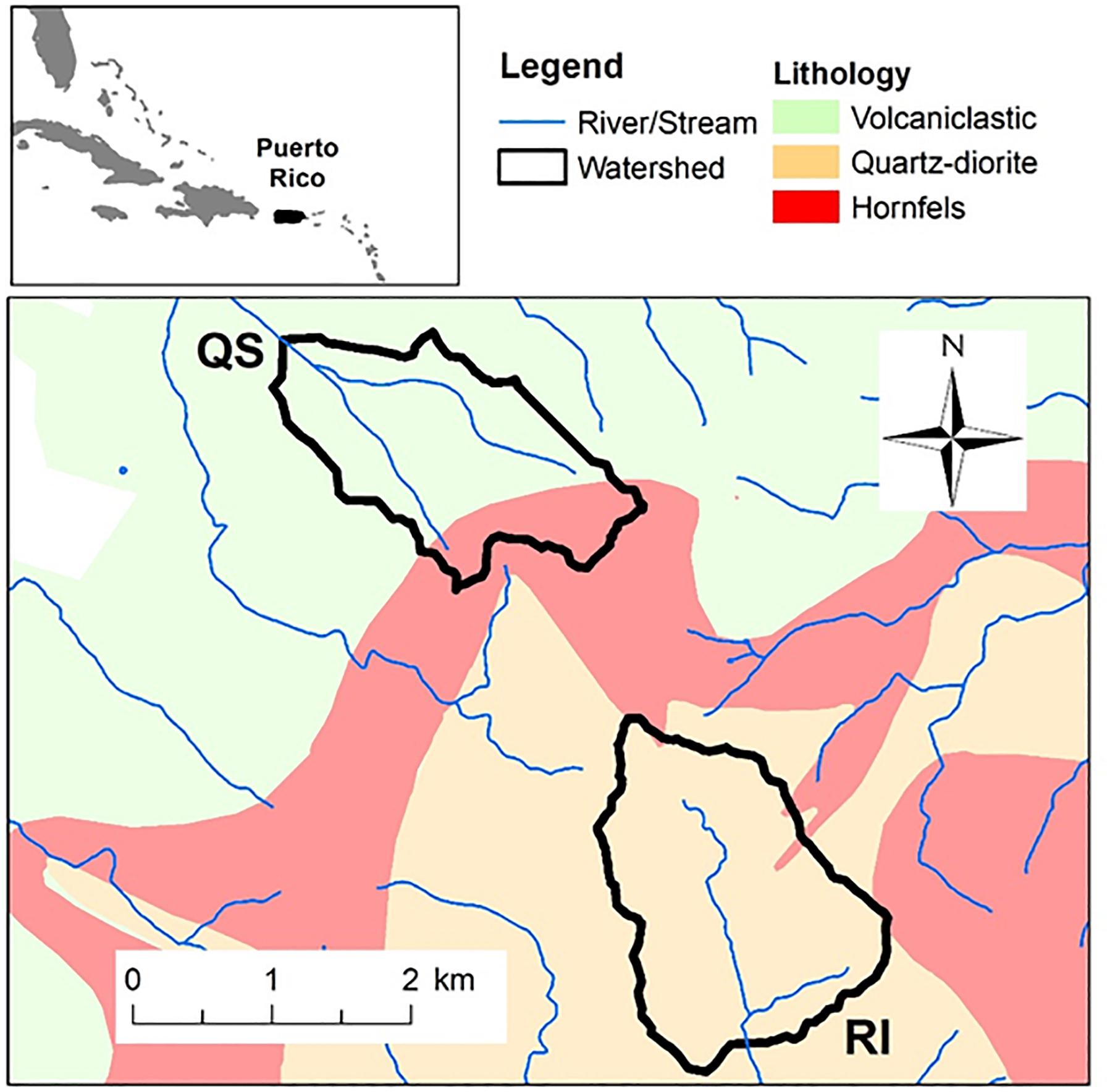
Figure 1. Map of the Luquillo Mountains, Puerto Rico, and the drainages of Quebrada Sonadora (QS) and Rio Icacos (RI) outlined in bold. Colors represents differences in lithology.
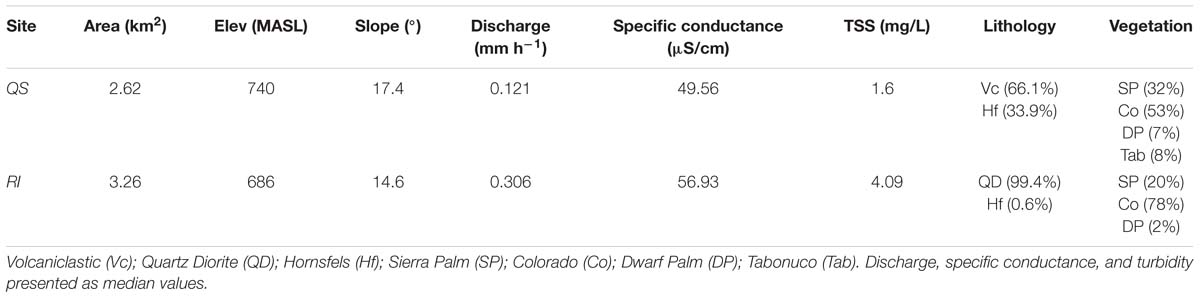
Table 1. Watershed characteristics and background hydrologic and stream chemistry metrics for Quebrada Sonadora (QS) and Rio Icacos (RI).
Hurricane Maria (initially a category 5 hurricane) made landfall in Puerto Rico on September 19th, 2017. Hurricane Maria made a direct hit on the southeast corner of the island moving northwest across the island and over the Luquillo Mountains. The peak discharge associated with Hurricane Maria was 5- to 6.5-fold greater than the mean peak discharges of all other storms assessed in this study (Table 2). As such, data from Hurricane Maria were treated as an anomaly and not included in calculations of central tendencies or regression analyses (see section “Statistical Analyses”). We do, however, directly compare the hysteretic metrics and behavior of this major Atlantic hurricane to that of the mean storm response experienced by both watersheds (Table 2). We do not treat Hurricane Irma, another major Atlantic hurricane which grazed past Puerto Rico 1 week prior to Hurricane Maria, as an anomalous extreme event because the storm’s runoff and calculated hysteresis metrics were within the range of the other assessed storm events. Irma’s HI and NS values are thus included in calculations of central tendencies.
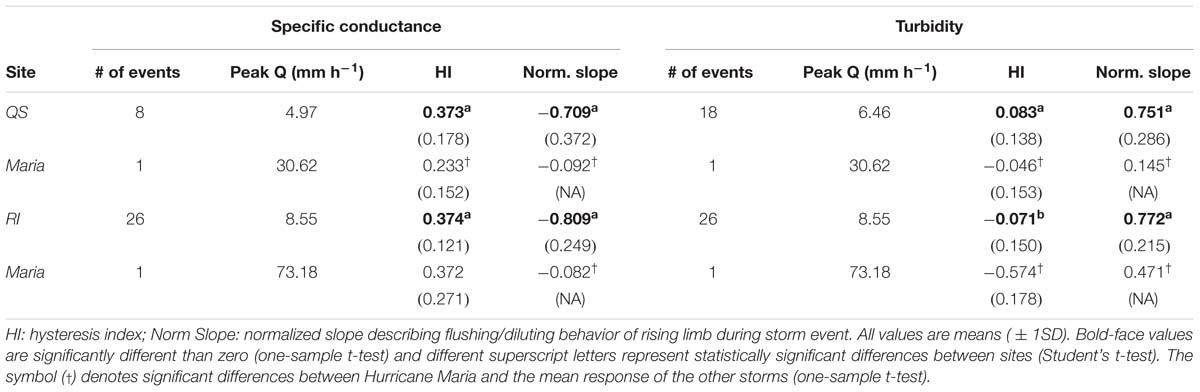
Table 2. Summary statistics of storm events across Quebrada Sonadora (QS) and Rio Icacos (RI) and during Hurricane Maria.
In situ Sensors
High-frequency specific conductance and turbidity data were collected using in situ sensors that were installed in 2014. In these Luquillo streams electrical conductivity is primarily driven by chloride, bicarbonate, magnesium, calcium, and sodium due to weathering and atmospheric inputs of sea salt. (McDowell and Asbury, 1994). Specific conductance was measured with an independent Onset HOBO conductance probe or a Campbell Scientific CS527 conductivity probe connected to a Campbell CR 1000 datalogger. Turbidity was measured with a Turner Designs Cyclops 7 turbidity probe at 850 nm (Turner Designs, Sunnyvale, CA, United States). The Campbell conductance and Turner turbidity probes were controlled and read by a Campbell Scientific CR1000 datalogger and data were transmitted in near real time to a database with CUASHI Observational Data Model (ODM2). Onset HOBO data were manually downloaded from the probe and uploaded to ODM2 on a web interface. QA/QC was performed on the data in ODM2 by staff at the University of New Hampshire. These measures primarily included eliminating spikes and making linear drift corrections for fouling.
Runoff and Storm Identification
Instantaneous stream discharge was provided by the USGS National Water Information System (U.S. Geological Survey, 2018) for Rio Icacos (RI: station 50075000) using their 15-min record and a Campbell stage logger at QS. At QS the rating curve was established using historic USGS data. and stage height measured at 15-min intervals. Discharge was measured in cubic feet per second and then converted to runoff. Discharge records, stored in ODM2, were interrogated for high flow events using ODM2 Admin with the Django Object-Relational Mapper (ORM). To identify the start of a storm event, we used a discharge threshold criterion of 3.5 and 6.3 mm h−1 at QS and RI, respectively. These threshold criteria represent approximately 30- and 20-fold increases in runoff relative to mean baseflow. The beginning of the storm was then identified as an inflection point that distinguished the start of the event from antecedent conditions. The end of the storm was identified as the point on the falling limb where discharge approached baseflow conditions or when another hydrological event commenced. Using these criteria, we assessed 18 events in QS and 26 storms in RI with peak storm discharge ranging from 3.93 to 21.25 mm h−1 in QS and 6.29–27.15 mm h−1 in RI. Hurricane Maria had a peak discharge of 30.62 mm h−1 in QS and 73.18 mm h−1 in RI.
Hysteresis Indices
Detailed methods describing the calculation of the HI and normalized slope (i.e., flushing index) can be found in Lloyd et al. (2016) and Vaughan et al. (2017) but are also briefly described here.
The HI as proposed by Lloyd et al. (2016) uses normalized concentration and discharge data (Eqs 1A,B):
where Qi and Ci are discharge and concentration, respectively, of time interval i; Qmin and Cmin are minimum storm values; and Qmax and Cmax are maximum storm values. Normalization of the data is key to facilitate comparison of storm-based metrics across events, as all events are assessed on the same scale (Lloyd et al., 2016). Because real-time measurements do not always occur at 2% intervals of discharge, we used piecewise linear regression to determine normalized solute concentrations (Cinorm) at each 2% interval of normalized discharge (Qinorm) on both the rising and falling limbs of the storm.
To describe each storm event, HI was calculated at each 2% discharge interval (Vaughan et al., 2017). Values derived from this index range between −1 and 1. Negative values describe counterclockwise hysteresis; positive values describe clockwise hysteresis. A value of zero indicates no hysteresis (Lloyd et al., 2016).
where CRL and CFL represent normalized concentration values on the rising and falling limbs, respectively. A mean HI and standard deviation for each storm was then calculated for all 50 pairs of values.
We also calculated the NS following Butturini et al. (2008) and Vaughan et al. (2017). This index uses normalized concentration and discharge data at the beginning of the storm and at peak discharge to describe a flushing or dilution effect on the rising limb of the hydrograph (Eq. 3):
where CQpeaknorm is the normalized concentration at peak discharge and Cinitialnorm is the normalized concentration at the beginning of the storm. Normalized slope values also range from −1 to 1. Negative values describe a diluting effect on the rising limb, while positive values indicate a flushing effect (Vaughan et al., 2017).
Long-Term C-Q Patterns
To compare storm hysteretic behavior to long-term C-Q patterns, we used data collected by the US Geological Survey (USGS) and the Luquillo Long-Term Ecological Research (LTER) and Critical Zone Observatory (CZO) programs. The long-term QS data record incorporates ∼1,500 specific conductance and total suspended sediment (TSS) data points at approximately weekly intervals across 30 years (1983–2013). Discharge data for QS were obtained from the USGS (2018; station 50063440). Long-term RI data include ∼850 specific conductance and 970 TSS samples collected at weekly intervals through much of the same period of record (1983–2014). TSS correlates strongly with turbidity (Gippel, 1995; Lewis, 1996) and thus we used it to compare long-term records with our event-based turbidity data. Both data sets include intensive sampling of individual storms as reported earlier by Stallard and Murphy (2012) and incorporated here into our long-term record. Discharge data for RI were obtained from the USGS (station 50075000). Specific conductance was measured in the field using a handheld probe. TSS was measured by filtering 0.5–2 L of water through pre-combusted Whatman GF/F glass fiber filters and weighed at the International Institute of Tropical Forestry in Rio Piedras, Puerto Rico or at the USGS Caribbean-Florida Water Science Center (Stallard and Murphy, 2012). We used log-log analyses [log(C)–log(Q)] to determine the long-term C-Q behavior for both specific conductance and TSS within each watershed (e.g., Johnson et al., 1969; Godsey et al., 2009).
Statistical Analysis
We used a one-sample t-test to determine if mean HI and mean NS values for each site were statistically different than zero. In these analyses, zero represents no predictable directionality of the hysteresis loop (HI; clockwise/counterclockwise) and no predictable flushing or dilution behavior (NS). We then used Student’s t-test to compare HI and NS between sites. We used simple linear regression to determine if there was a relationship between peak storm discharge and peak specific conductance and turbidity, mean HI, and NS. We used multiple linear regression to examine whether adding antecedent discharge explained additional variation in the response variables compared to peak storm Q alone. Accordingly, our multiple regression models examined average Q over the antecedent 6, 12, and 24 h prior to the onset of the storm. We chose these time intervals to be able to compare with results from McDowell and Asbury (1994). We then examined model performance with stepwise backward elimination regression comparing Akaike Information Criterion (AIC). To evaluate the response to Hurricane Maria we used a one-sample t-test. In this series of analyses, we set μ to the HI or NS value recorded during the hurricane event and tested whether the mean response of the other storms was significantly different. All analyses were performed in R using the base functions except for the stepwise regression which used the MASS package (Venables and Ripley, 2002).
Results
Specific Conductance
Contrary to our hypothesis, the directionality and flushing behavior of specific conductance were highly congruent across our two study watersheds (Figures 2, 3 and Table 2) with nearly identical mean HI values (0.373–0.374; Table 2). In both watersheds, mean HI for specific conductance was positive and significantly different than zero indicating clockwise hysteresis (QS: p = 0.003; RI: p < 0.0001). Specific conductance normalized slopes were also not significantly different between watersheds (p = 0.468; Table 2). In both watersheds, NS values were strongly negative and significantly different than zero (QS: p = 0.0002; RI: p < 0.0001). These results reflect a diluting relationship between discharge and specific conductance during storms and indicate a significant source limitation of the solutes that contribute to specific conductance.
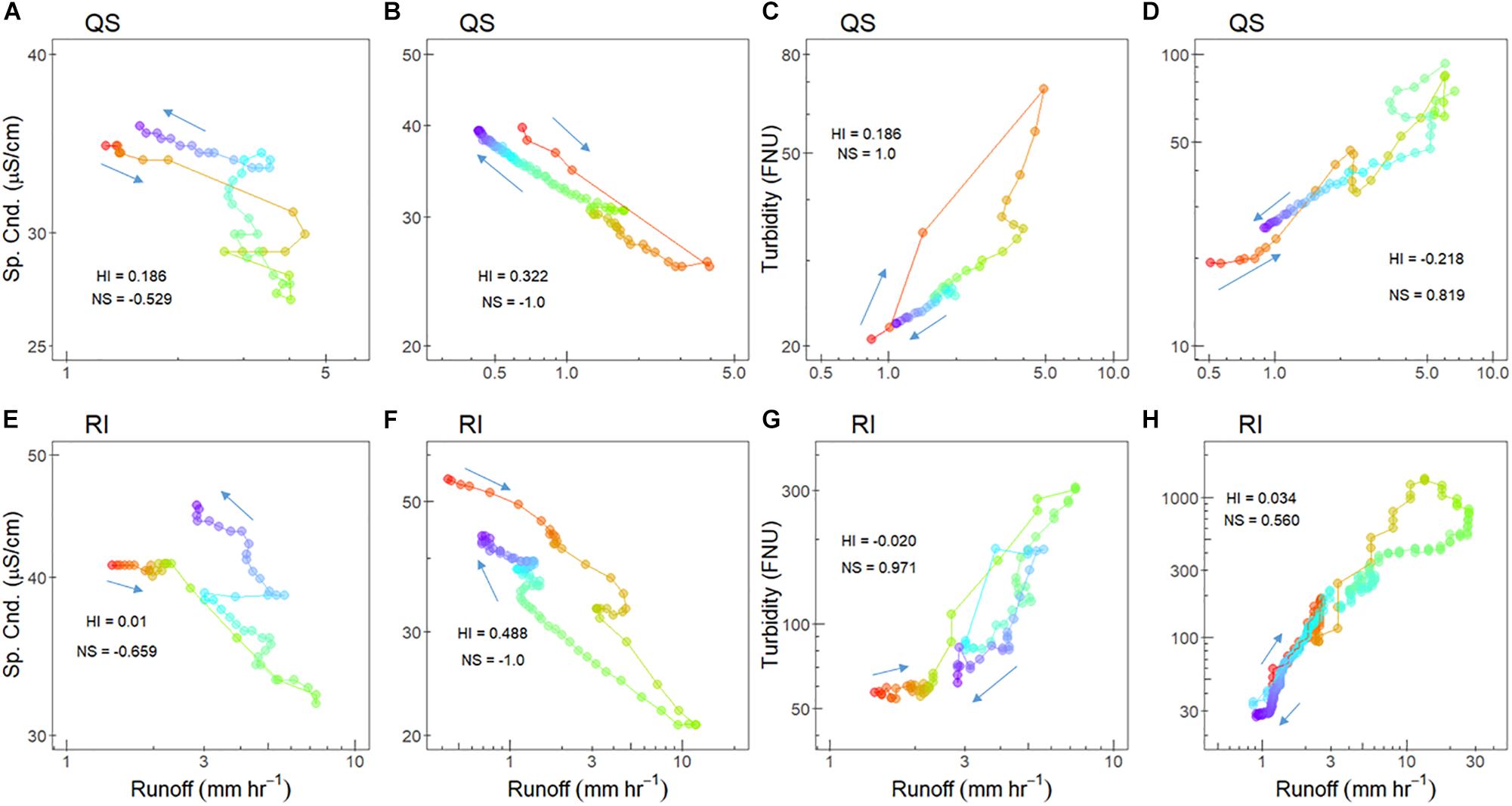
Figure 2. Typical specific conductance (Sp. Cnd.) and turbidity responses to increased streamflow during individual storm events. Red/orange colors represent the beginning of the storm and blue/purple colors represent the end of the storm. Arrows also provide beginning and ending points of each event. The top row of (A–D) shows the response of specific conductance and turbidity at Quebrada Sonadora (QS) and the bottom row of (E–H) shows the response of specific conductance and turbidity at Rio Icacos (RI). Hysteresis index (HI), and normalized slope (NS) values are presented for each storm. Specific conductance generally dilutes in response to increased flow whereas turbidity exhibits flushing behavior. Note that both axes are log-scaled.
Turbidity
The response of turbidity to storm events contrasted to the response of specific conductance (Figures 2, 3 and Table 2). As hypothesized, turbidity HI was significantly different between watersheds (p < 0.0001; Table 2). In QS, turbidity HI was positive and significantly greater than zero (p = 0.004) showing clockwise hysteresis. Turbidity HI in RI was negative and significantly less than zero showing counterclockwise hysteresis (p < 0.0001). Both watersheds had turbidity normalized slopes that were positive and significantly greater than zero (p < 0.0001 for both), with no statistical difference between watersheds. The positive normalized slopes indicate that the mobilization of sediment in both QS and RI was transport limited.
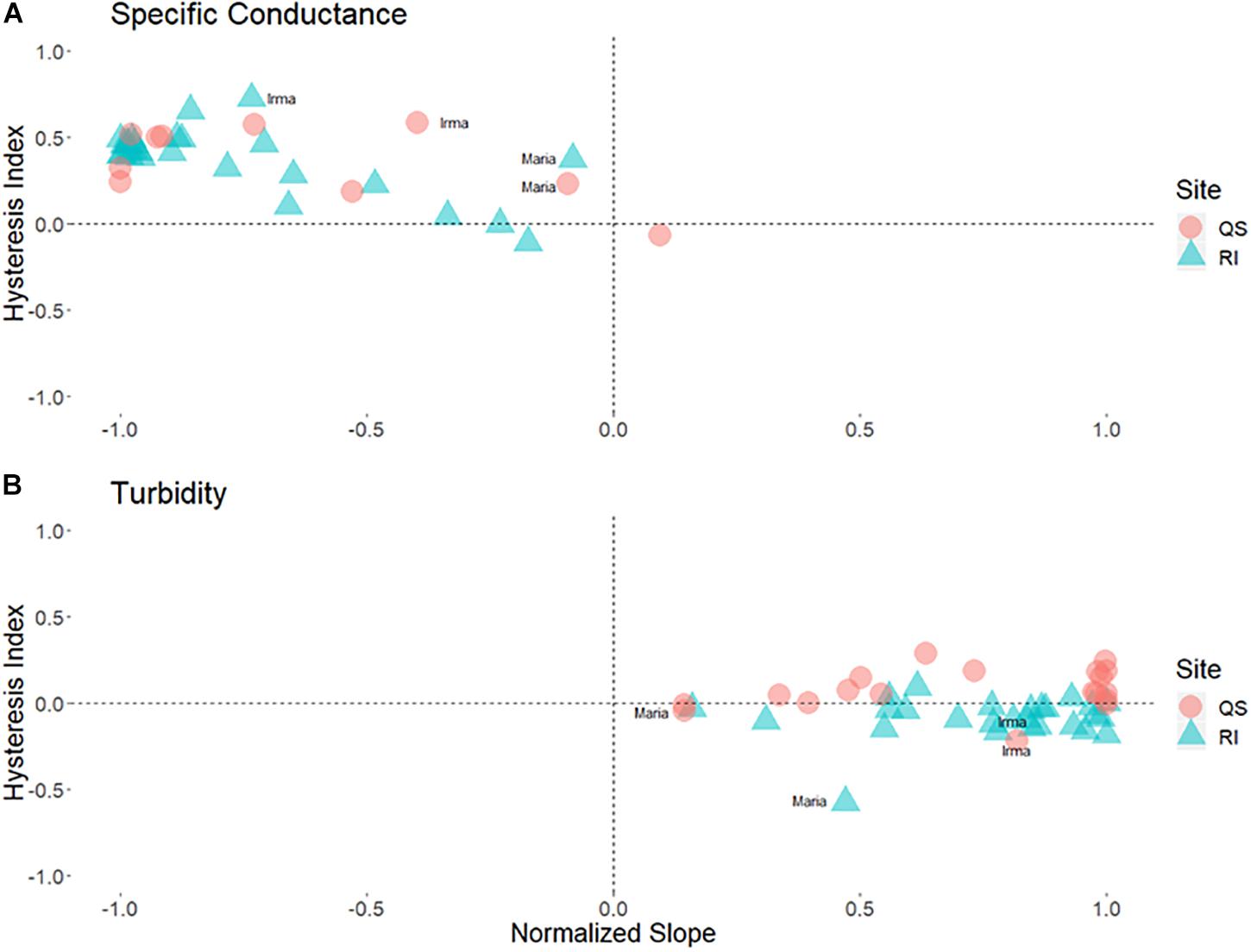
Figure 3. Plots of (A) specific conductance and (B) turbidity storm HI versus storm normalized slope at both Quebrada Sonadora (QS) and Rio Icacos (RI). Upper-left hand quadrants represent a clockwise diluting response; upper right-hand quadrants represent a clockwise flushing response; lower left-hand quadrants represent counterclockwise diluting response; and lower right-hand quadrants represent a counterclockwise flushing response. The responses due to Hurricane Irma and Hurricane Maria are also labeled.
Regression Models and Model Performance
The explanatory power of peak and antecedent discharge on hysteresis behavior (i.e., peak specific conductance and turbidity, mean HI, and NS) was inconsistent across sites, metrics and models (Table 3). Evaluation of model performance via AIC indicated, however, that when significant relationships were detected, peak Q alone was consistently the best model to predict minimum and maximum concentrations of specific conductance and turbidity, and metrics of hysteresis. While consideration of antecedent Q added explanatory power to some models, the multi-parameter models performed no better than simple linear regression models of peak Q alone.
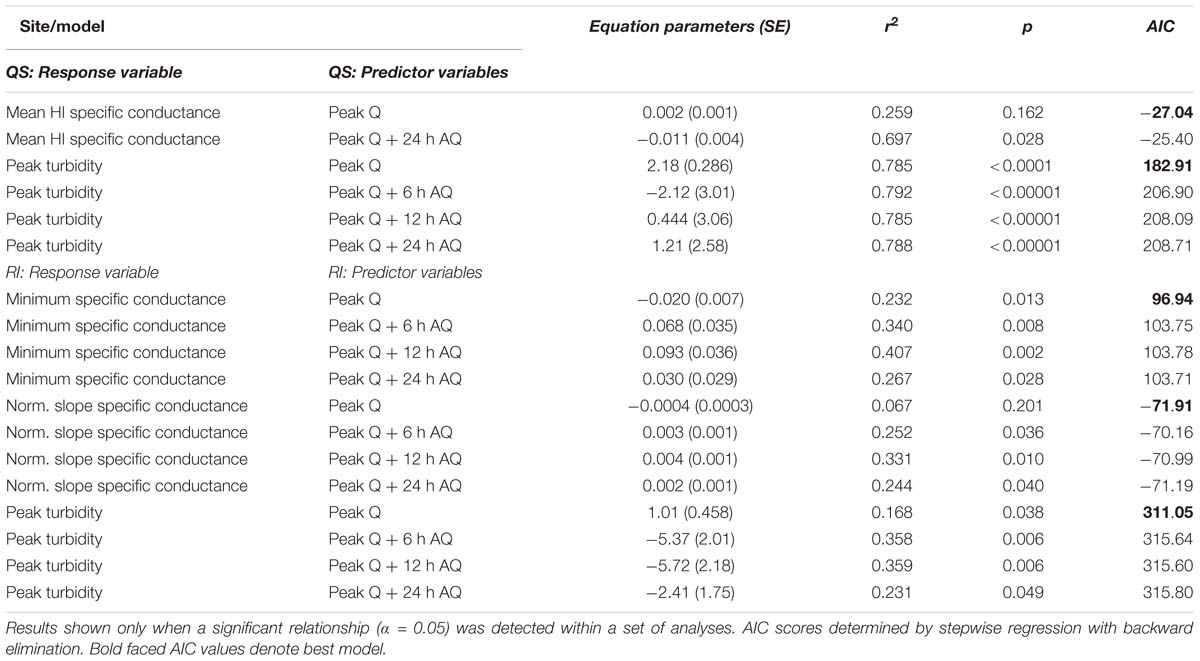
Table 3. Simple and multiple linear regression results of storm and hysteresis metrics with peak and antecedent discharge (AQ) averaged over 6, 12, and 24 h at Quebrada Sonadora (QS) and Rio Icacos (RI).
As hypothesized, peak discharge correlated significantly with peak turbidity in both watersheds for the individual storms sampled with sensors (Table 3). Contrary to our expectations, the relationship between peak turbidity and peak discharge was much stronger in QS (r2 = 0.785) than in RI (r2 = 0.168), even though the larger weekly data set shows that TSS is better predicted by Q in RI than in QS (see section “Long-Term C-Q Patterns”). In contrast, minimum specific conductance was correlated with peak discharge only in RI. The lack of a relationship in QS may be the result of a smaller sample size than in RI.
Hurricane Maria
Hurricane Maria generated unique hysteretic patterns (Figure 4 and Table 2). In QS, specific conductance HI showed clockwise hysteresis, but average HI was significantly less than the mean of the other analyzed storms (p = 0.046) indicating smaller differences in concentration on the rising and falling limbs of the storm. Normalized slope of specific conductance in QS during Maria was also significantly different from the other storms (p < 0.001) and much closer to zero (Figure 4A). Patterns of turbidity during Maria in QS also differed significantly from other storms with a slightly negative HI (p < 0.001). Although the normalized slope for turbidity in QS during Maria remained positive (Figure 4B), there was a significant decrease in the slope (p < 0.001) of approximately five-fold.
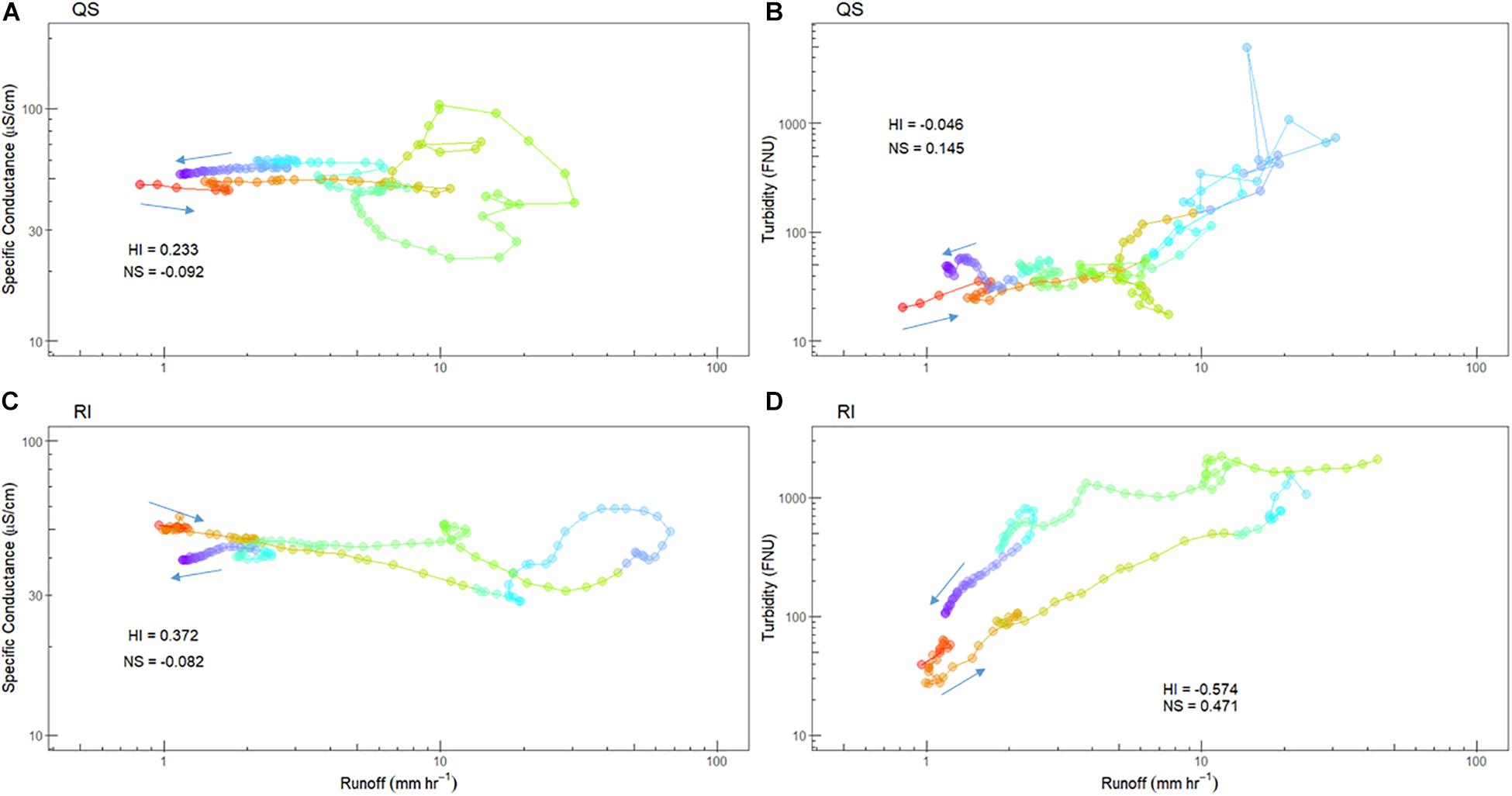
Figure 4. The response of specific conductance and turbidity to Hurricane Maria in Quebrada Sonadora (QS: A,B) and Rio Icacos (RI: C,D). Red/orange colors represent the beginning of the storm and blue/purple colors represent the end of the storm. Arrows also provide beginning and ending points of each event. HI and normalized slope (NS) values are presented for each storm. Note that both axes are log-scaled.
In RI during Maria, specific conductance HI was positive and nearly identical to the other storms (p = 0.48; Table 2), but the normalized slope was significantly less negative than the other storms (p < 0.001) and approached zero indicating less pronounced diluting behavior (Figure 4C). Turbidity HI was significantly more negative than other storms (p < 0.001) in RI demonstrating strong counterclockwise hysteretic behavior. And similar to turbidity in QS, normalized slope in RI during Maria remained positive (Figure 4D) but was significantly less (1.64x) than the other storms (p < 0.001; Table 2).
Long-Term C-Q Patterns
As hypothesized, storm hysteresis flushing and diluting behavior (i.e., positive and negative FI values, respectively) matched long-term C-Q patterns with respect to source versus transport limitation as the primary control mechanism. In both watersheds, specific conductance demonstrated strong diluting behavior across a range of flow conditions (Figures 5A,C) indicating source limitation. Discharge explained 41 and 50% of the variation in specific conductance in QS and RI, respectively. In contrast, concentrations of TSS became enriched (flushing) with increases in discharge in both watersheds indicating transport limitation (Figures 5B,D). However, the relationship between TSS and discharge was much weaker in QS (r2 = 0.13) compared to RI (r2 = 0.55).
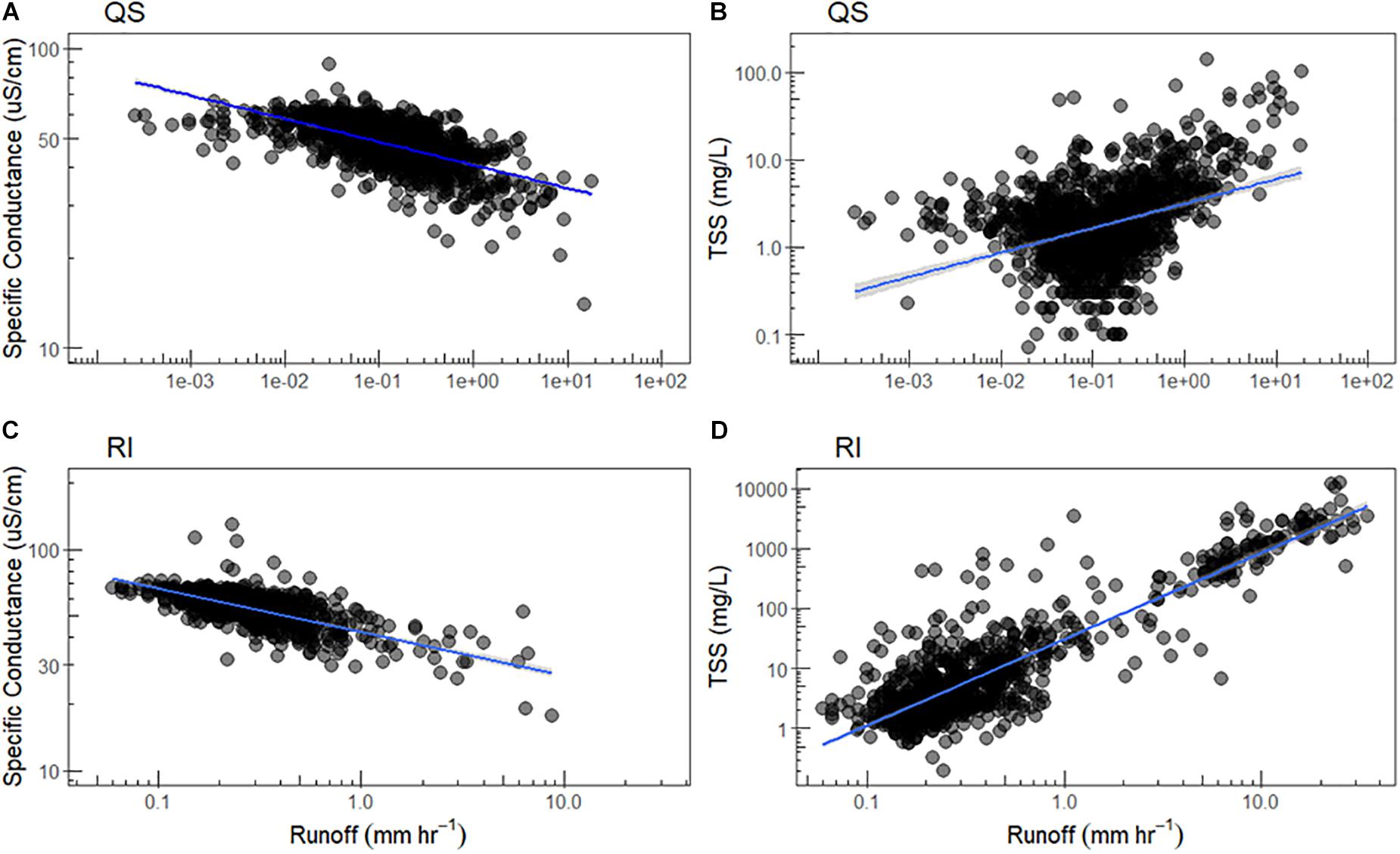
Figure 5. Long-term concentration-discharge (C-Q) response of specific conductance and total suspended sediment (TSS) in Quebrada Sonadora (QS: A,B) and Rio Icacos (RI: C,D). Note that both axes are log-scaled.
Discussion
The application of high-frequency sensor data in tandem with indexed-based metrics allowed us to describe and quantify hysteresis and effectively compare storm events. Storm-based C-Q relationships often show complex hysteretic behavior including non-linear and figure-eight patterns where concentrations on the rising and falling limbs intersect multiple times. Index-based approaches such as the HI (Lloyd et al., 2016; Vaughan et al., 2017) can be used to describe an event at multiple time points capturing intra-storm complexities, and objectively integrate the complexity. With the normalization of the data (Lawler et al., 2006; Lloyd et al., 2016), storm events are placed onto a similar scale supporting the direct comparison of the response of solute and sediment across space and time. Storms in the Luquillo Mountains reveal a high degree of complexity making the use of these metrics appropriate for this landscape (e.g., Figures 2A,D,G).
Our analysis showed that the mobilization and export of sediments are largely decoupled from that of solutes during storm events (Figure 3) and that solutes are generally source limited, while sediment is generally transport limited. Across both study watersheds, specific conductance showed a consistent response to changes in discharge during storm events. The directionality as measured by HI (clockwise) and strong negative normalized slopes of specific conductance were virtually identical in the two sites. These patterns suggest that the primary source of solutes in both watersheds is located near the stream channel and is rapidly mobilized and depleted during storm events due to near-surface flow paths. Higher concentrations on the rising limb likely reflect the storage of solutes in riparian zones and the contribution of marine aerosols transported inland via the predominant east-west storm tracks (McDowell et al., 1990). Contrary to our expectations, we did not see any evidence that the hysteretic responses of specific conductance during storm events is the result of different lithographic templates. While lithology is known to influence flow paths including length, depth, and reaction time (e.g., McDowell et al., 1992), as well as porosity and reactive minerals in the weathering zone, lithology might be responsible for some differences in solute concentration among sites across the Luquillo Mountains (Bluth and Kump, 1994; McDowell and Asbury, 1994; Wymore et al., 2017). Lithology does not, however, appear to play a role in determining hysteretic behavior of those solutes as measured by specific conductance. This finding is consistent with a recent study that did not find a relationship between the structure of the Critical Zone and the C-Q behavior of multiple solutes in streams of the Luquillo Mountains (Wymore et al., 2017).
In contrast to specific conductance, turbidity showed less pronounced patterns of hysteresis with respect to directionality. Although turbidity had a strong flushing response to increases in discharge (positive NS values), we computed several near-zero HI values. HI values closer to zero signify minimal hysteresis but may also result from complex concentration dynamics in multi-peaked storms. The lack of differentiation between concentrations on the rising and falling limbs of the storms indicates reservoirs of sediment both proximal and distal to the stream channel. This conclusion contrasts with many reports (Smith and Dragovich, 2009; Aich et al., 2014; Rose et al., 2018) including from the Luquillo Mountains and RI (Gellis, 2013; Clark et al., 2017). In an assessment of sensor-collected turbidity data during rewetting events following a major drought in RI, Clark et al. (2017) reported a clockwise C-Q response for turbidity (Figure 6). Our HI analysis on the other hand, indicates that the turbidity C-Q relationship generally lacks directionality across events, including a reanalysis of the data used in Clark et al. (2017; see Figure 6). We do not believe this is an artefact of the index-based approach as computed HI values were consistent with the clear hysteresis of specific conductance. The lack of directionality, and supply of seemingly inexhaustible sources of sediment, may be the result of RI’s extremely high weathering rates (White et al., 1998). The minimal hysteresis that we have observed challenges the notion that the majority of sediment mobilization occurs within the stream channel (McDowell and Asbury, 1994) and that the increase in energy during the rising limb of the hydrograph is the primary mechanism resulting in hysteresis.
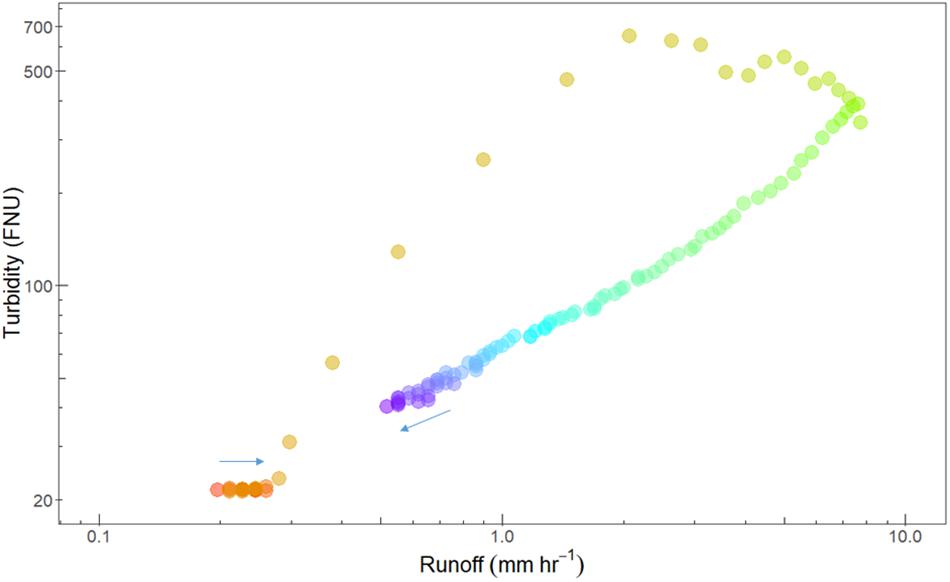
Figure 6. The relationship between turbidity and runoff after a major rewetting event in Rio Icacos (September 2015) showing a clockwise hysteresis loop. Red/orange colors represent the beginning of the storm and blue/purple colors represent the end of the storm. Arrows also provide beginning and ending points of the storm event. Note that both axes are log-scaled. Figure redrawn from USGS sensor data available from Clark et al. (2017). When these data are normalized to peak flow the resultant mean HI value for the event is 0.098. HI values closer to zero indicate less pronounced differences in turbidity between the rising and falling limb of the hydrograph. See discussion text for additional insight.
We point to two mechanisms that account for these opposing results and interpretations. First, peak turbidity in RI is not consistently associated with peak flow as visually apparent in Figures 2H, 6, for example, and from the relatively weak correlation coefficient describing the relationship between peak turbidity and peak Q. After peak turbidity is reached, there are distinct periods of dilution behavior as the system continues toward peak flow. We suggest that this offset of peak turbidity coincides with peak storm intensity (Clark et al., 2017) rather than peak stream discharge, and initial flushing behavior is the direct result of sediment mobilization from the hillslope rather than flow in the stream channel. Second, the additional source of sediment that must be activated to maintain concentrations during the falling limb is the result of slopewash (Larsen et al., 1999) and particle movement via subsurface seepage. Recent evidence demonstrates the mobilization of particles can occur within the subsurface (Herndon et al., 2018; Kim et al., 2018), a finding that challenges previously held models that particles in the soil profile are assumed to be difficult to transport. Our field observations show that seepage of groundwater or shallow interflow can occur at many points along the stream banks within the RI watershed. Although we would expect both seepage and particle mobilization within the clay-rich RI soils to occur at very slow rates, it has been documented throughout the Luquillo Mountains that earthworms are an important agent of change within the subsurface responsible for increasing soil porosity (Larsen et al., 2012). Changes within the subsurface due to the burrowing behavior of earthworms provide the needed porosity and macropore space for seepage and saturated overland flow that will introduce novel sources of particles. These watershed-scale phenomena, in addition to in-stream processes above the point of sampling, may help to explain the uninterrupted supply of sediment during the recession limb. We conclude that after normalizing the data to peak flow, the processes that mobilize particles during early and late stages of storm events are more evident and their effects result in a lack of strong directionality and hysteresis. This series of observations points to interactions between biological, geological and hydrological mechanisms that can drive watershed exports from the Critical Zone.
We also explored relationships between peak storm discharge and the role of antecedent discharge to influence patterns of hysteresis and found inconsistent patterns between watersheds. Peak turbidity in both QS and RI correlated significantly with peak discharge but this pattern was much stronger in QS than in RI, contrary to expectations derived from the long-term TSS C-Q patterns. These contrasting responses to flow between turbidity and TSS likely reflect both methodological differences and differences in particle size between the two watersheds. TSS is a filtration-based method reflecting only those particles captured typically on a 0.7 μm glass-fiber filter (e.g., Clark et al., 2017). In contrast, optical and sensor-based assessments of turbidity reflect a bulk measurement of all particles and sediment. Volcaniclastic watersheds, such as QS, commonly contain larger particles in the stream bed compared to other lithologies; however, these particles are typically of a more uniform size distribution (Phillips and Jerolmack, 2016). Quartz-diorite and sand-dominated watersheds like RI on the other hand, contain particles of smaller size in the stream bed, yet reflect a more heterogeneous mixture of particle sizes (e.g., Larsen, 1997; Pike et al., 2010). This difference in stream beds is directly related to differences in soil texture between the two lithologies. In the quartz diorite, soils are consistently coarser across the riparian to upland catena than they are in the volcaniclastic lithology, which is exceedingly clay-rich (McDowell et al., 1992) and delivers little sand or gravel to the stream bed compared to the diorite, as reflected in stream bed pebble counts in the two lithologies (Potter et al., 2010). The greater diversity of particle size in the stream bed of RI likely contributes to the high degree of unexplained variation in our analysis. The relative uniformity in particle size in QS is likely why the inclusion of antecedent discharge in our regression models did not add any additional explanatory power to our predictions of hysteresis and peak turbidity. In RI, however, antecedent discharge added explanatory power to our models, suggesting that it may capture the complex manner in which a heterogeneous particle load changes in response to variation in discharge. The negative parameter estimates associated with regression models that include antecedent Q provides some support for a flushing effect of previous storms on bed sediment that can decrease the supply of mobilizable particles ahead of the subsequent storm, a mechanism previously proposed for RI (Gellis, 2013). However, because models that considered antecedent Q did not outperform models of peak Q alone, such results must be interpreted with caution. Although previous Luquillo-based studies also found that antecedent Q provided additional explanatory power in predicting sediment and solute concentrations (McDowell and Asbury, 1994; Gellis, 2013), our analyses suggest that the primary mechanisms controlling the magnitude of material flux and hysteresis metrics are the magnitude and intensity of the individual storm events.
Overall, the response of solute and sediment export to Hurricane Maria differed from the other storms we assessed, including Hurricane Irma. All hysteresis metrics, except RI’s specific conductance HI value, were significantly different from the average storm response. Many of Hurricane Maria’s metrics also moved closer to zero, suggesting a homogenizing effect of this major hurricane on hysteresis. For example, specific conductance HI in QS decreased approximately 38% indicating less differentiation between concentrations of solutes on the rising and falling limb of the hurricane’s hydrograph. Similarly, specific conductance normalized slope in QS went from steeply negative (−0.709) to nearly zero (−0.092) indicating a more chemostatic response during the storm (Figure 4A), with a similar pattern observed in RI (Figure 4C). These significantly different values in normalized slope values for specific conductance likely reflect the entrainment of sea salt during Hurricane Maria so that the typically observed dilution response was muted. Typically storm events have a strong dilution response in the Luquillo Mountains including for concentrations of sea salt (Shanley et al., 2011). Specific conductance values in QS during Hurricane Maria however, reached as high as 104 μS/cm which is a 2.6x increase above where other more typical storms begin their dilution response. And the intra-storm variability in specific conductance during Hurricane Maria far exceeded other evaluated storms including Hurricane Irma (Figure 7). The magnitude of Maria appears to be an exception with respect to delivery of sea salts ultimately resulting in a chemostatic response for specific conductance. RI’s mean turbidity HI during the hurricane also became considerably more negative, indicative of strong counterclockwise hysteresis. This unique pattern in RI reflects a significant secondary pulse and mobilization of particles distal to the stream channel that may not occur during storms of smaller magnitude. Possible sources of this large secondary pulse include bank collapse (Simon et al., 2000; Rinaldi et al., 2004), landslides (Larsen and Torres-Sanchez, 1992) and in-channel sand activation, all of which require the additional energy supplied by a storm of such magnitude. Changes in hysteresis due to Hurricane Maria indicate that hurricanes and other large storms may surpass a threshold that activates these unique watershed-scale processes and where the associated flood wave travels faster than the sediment wave (Marcus, 1989; Gellis, 2013), collectively resulting in strong counterclockwise hysteresis.
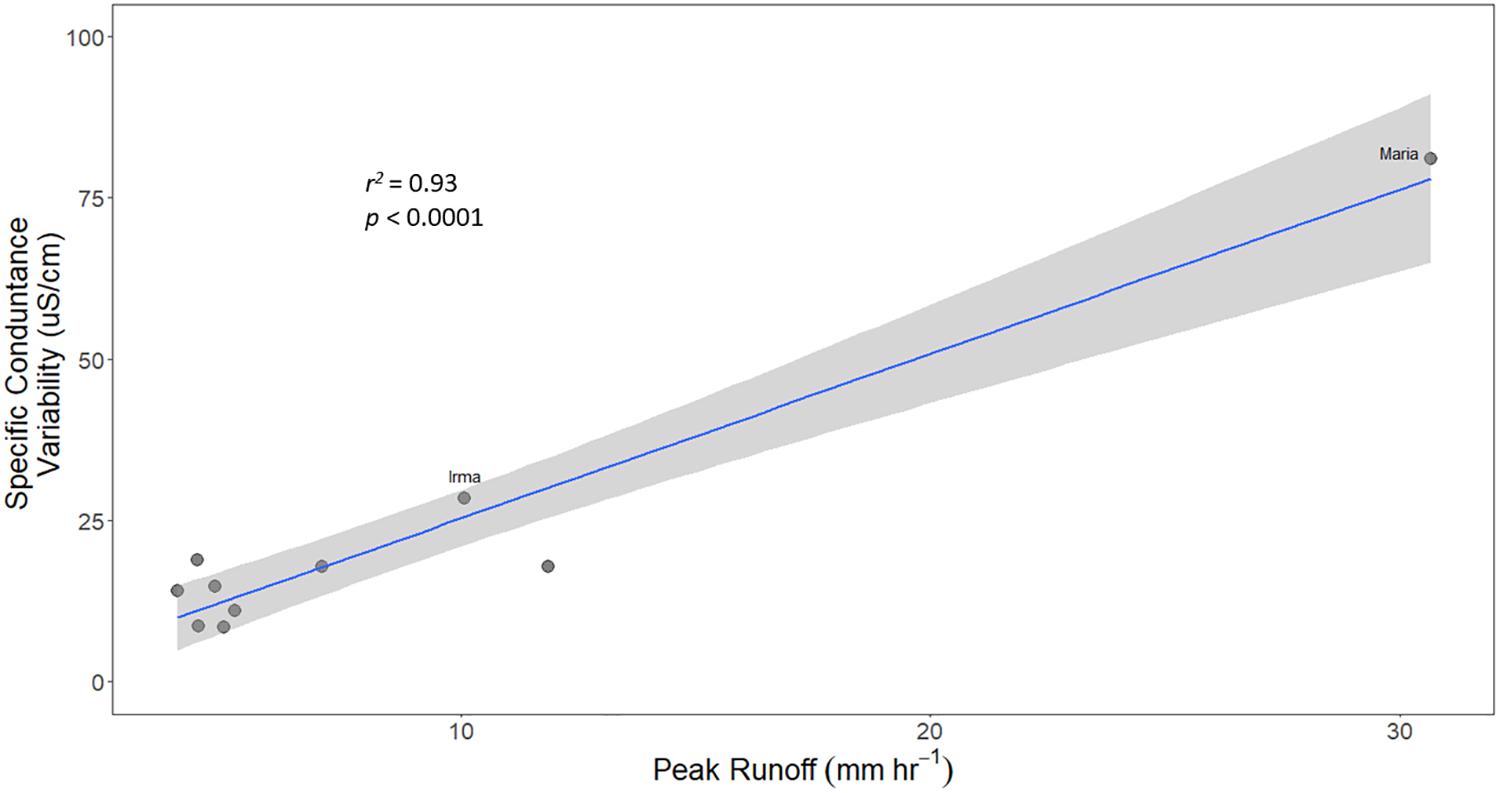
Figure 7. Linear regression of intra-storm variability in specific conductance (highest value-lowest value) and peak storm runoff at Quebrada Sonadora (QS). The points associated with Hurricane Irma and Hurricane Maria are labeled.
Few studies have been able to capture within-storm hysteretic dynamics of an extreme event the magnitude of Hurricane Maria. Previous studies have incorporated less frequent ISCO-based sampling (Dhillon and Inamdar, 2013) while others have assessed the falling limb of the hydrograph (Shanley et al., 2011; Rue et al., 2017). To our knowledge, this study represents one of the first to describe and quantify solute and sediment hysteretic behavior during an extreme event using automated high-frequency in situ sensors capturing both the rising and falling limbs of the hydrograph. While we might hypothesize an increase in exports resulting from the observed switch from dilution to chemostasis for specific conductance and the effect of landslides (in addition to the overall erosive nature of such events; Larsen and Torres-Sanchez, 1992), measured fluxes due to cyclonic events reveal inconsistent patterns. For example, dissolved organic carbon and particulate organic carbon (POC) from Hurricane Irene accounted for 56 and 19%, respectively, of annual exports from a temperate forested watershed (Dhillon and Inamdar, 2013), whereas in the western and tropical Pacific, cyclones can mobilize 77–92% of the total POC load (Hilton et al., 2008) and deliver approximately 32% of the suspended sediment load (Darby et al., 2016). In contrast, suspended sediment loads due to Hurricane Hugo, which made landfall in Puerto Rico in September 1989, were lower than expected due to debris dams which created local backwater effects, reducing stream velocity and decreasing sediment loads (Gellis, 1993). Hurricane Maria, however, may have surpassed critical thresholds above which exports become highly concentrated.
Previous efforts to understand material export during storms, especially in remote locations, have been hampered due to the difficulties associated with event-based sampling. High-frequency in situ sensors provide novel insights into storm-based C-Q dynamics including major hydrological events. Understanding the complexities within and among storms is critical because of the disproportionate effects that storms have on material export (Hilton et al., 2008; Raymond and Saiers, 2010; Inamdar et al., 2017) and the substantial contribution of tropical mountainous landscapes to global exports from land to ocean. Using recently developed index-based approaches, we demonstrate that solutes are generally source limited while sediment is generally transport limited in the Luquillo Mountains. We also provide evidence that turbidity may not have a hysteretic response to changes in flow due to the combined effects of precipitation, seepage and saturation overland flow. Insights from these hysteresis metrics suggest the presence of previously unaccounted for sources of sediment and processes of sediment mobilization that can influence material export from a tropical Critical Zone.
Author Contributions
JS and WM installed and operated the sensors networks. AW and ML designed and performed the analyses. AW, JS, and WM interpreted results. AW wrote the initial draft. All authors contributed to revisions.
Funding
Funding for this project was provided by the Luquillo Critical Zone Observatory (EAR-0722476 and 1331841), the Luquillo Long-Term Ecological Research program (DEB 0620919, 1239764, and 1546686), the StreamPulse project (EF- 1442444), and a University of New Hampshire CoRE (Collaborative Research Excellence) grant to the Watershed Informatics group. Partial funding was provided by the New Hampshire Agricultural Experiment Station. This is Scientific Contribution Number 2803. This work was supported by the U.S. Department of Agriculture National Institute of Food and Agriculture (McIntire-Stennis) Project (1006760).
Conflict of Interest Statement
The authors declare that the research was conducted in the absence of any commercial or financial relationships that could be construed as a potential conflict of interest.
Acknowledgments
The authors wish to acknowledge the Water Quality Analysis Lab at the University of New Hampshire and field work by Carla Lopez Lloreda. The authors also acknowledge reviews by Jenn Hoyle Fair and two the reviewers. Any use of trade, firm, or product names is for descriptive purposes only and does not imply endorsement by the U.S. Government. Data are available via Hydroshare: https://doi.org/10.4211/hs.f8420c1447fe440eb93e656b2db0b64d. Discharge data are available from the USGS National Water Information System (2018).
References
Aich, V., Zimmermann, A., and Elsenbeer, H. (2014). Quantification and interpretation of suspended-sediment discharge hysteresis patterns: how much data do we need? Catena 122, 120–129. doi: 10.1016/j.catena.2014.06.020
Ávila, A., Piñol, J., Rodà, F., and Neal, C. (1992). Storm solute behaviour in a montane Mediterranean forested catchment. J. Hydrol. 140, 143–161. doi: 10.1016/0022-1694(92)90238-q
Biron, P. M., Roy, A. G., Courschesne, F., Hendershot, W. H., Côté, B., and Fyles, J. (1999). The effects of antecedent moisture conditions on the relationship of hydrology to hydrochemistry in a small forested watershed. Hydrol. Process. 13, 1541–1555. doi: 10.1002/(sici)1099-1085(19990815)13:11<1541::aid-hyp832>3.3.co;2-a
Bluth, G. J. S., and Kump, L. R. (1994). Lithologic and climatologic controls of river chemistry. Geochim. Cosmochim. Acta 58, 2341–2359. doi: 10.1016/0016-7037(94)90015-9
Butturini, A., Alvarez, M., Bernal, S., Vazquez, E., and Sabater, F. (2008). Diversity and temporal sequences of forms of DOC and NO3 – discharge responses in an intermittent stream: predictable or random succession? J. Geophys. Res. Biogeo. 113:G03016. doi: 10.1029/2008JG000721
Chadwick, K. D., and Asner, G. P. (2016). Tropical soil nutrient distributions a determined by biotic and hillslope processes. Biogeochemistry 127, 273–289. doi: 10.1007/s10533-015-0179-z
Chorover, J., Derry, L. A., and McDowell, W. H. (2017). Concentration-discharge relations in the critical zone: implications for resolving critical zone structure, function and evolution. Water Resour. Res. 53, 8654–8659. doi: 10.1002/2017wr021111
Clark, K. E., Shanley, J. B., Scholl, M. A., Perdrial, N., Perdrial, J. N., Plante, A. F., et al. (2017). Tropical river suspended sediment and solute dynamics in storms during an extreme drought. Water Resour. Res. 53, 3695–3712. doi: 10.1002/2016WR019737
Darby, S. E., Hackney, C. R., Leyland, J., Kummu, M., Lauri, H., Parsons, D. R., et al. (2016). Fluvial sediment supply to a mega-delta reduced by shifting tropical-cyclone activity. Nature 539, 276–279. doi: 10.1038/nature19809
Dhillon, G. S., and Inamdar, S. (2013). Extreme storms and changes in particulate and dissolved organic carbon in runoff: entering uncharted waters? Geophys. Res. Lett. 40, 1322–1327. doi: 10.1002/grl.50306
Evans, C., and Davies, T. D. (1998). Causes of concentration/discharge hysteresis and its potential as a tool for analysis of episode hydrochemistry. Water Resour. Res. 34, 129–137. doi: 10.1029/97wr01881
Fellman, J. B., Hood, E., Edwards, R. T., and D’Amore, D. V. (2009). Changes in the concentration, biodegradability, and fluorescent properties of dissolved organic matter during stormflows in coastal temperate watersheds. J. Geophys. Res. Biogeo. 114:G01021. doi: 10.1029/2008JG000790
Garcia-Martino, A. R., Warner, G. S., Scatena, F. N., and Civco, D. L. (1996). Rainfall, runoff and elevation relationships in the Luquillo Mountains of Puerto Rico. Caribb. J. Sci 32, 413–424.
Gellis, A. C. (1993). The effects of hurricane hugo on suspended-sediment loads, Lago Loiza Basin, Puerto Rico. Earth Surf. Process. Landforms. 18, 505–517. doi: 10.1002/esp.3290180604
Gellis, A. C. (2013). Factors influencing storm-generated suspended-sediment concentrations and loads in four basins of contrasting land use, humid-tropical Puerto Rico. Catena 104, 39–57. doi: 10.1016/j.catena.2012.10.018
Gippel, C. J. (1995). Potential of turbidity monitoring for measuring the transport of suspended solids in streams. Hydrol. Process. 9, 87–97. doi: 10.1002/hyp.3360090108
Godsey, S. E., Kirchner, J. W., and Clow, D. W. (2009). Concentration-discharge relationships reflect chemostatic characteristics of US catchments. Hydrol. Process. 23, 1844–1864. doi: 10.1002/hyp.7315
Herndon, E. M., Steinhoefel, G., Dere, A. L. D., and Sullivan, P. L. (2018). Perennial flow through convergent hillslopes explains chemodynamic behavior in a shale headwater catchment. Chem. Geol. 493, 413–425. doi: 10.1016/j.chemgeo.2018.06.019
Hilton, R. G., Galy, A., Hovius, N., Chen, M.-C., Horng, M.-J., and Chen, H. (2008). Tropical-cyclone-driven erosion of the terrestrial-biosphere from mountains. Nat. Geosci. 1, 759–762. doi: 10.1038/ngeo333
Inamdar, S., Shanely, J. B., and McDowell, W. H. (2017). Aquatic Ecosystems in a Changing Climate EOS, Transactions of the American Geophysical Union. Reston: USGS.
Inamdar, S. P., O’Leary, N., Mitchell, M. J., and Riley, J. T. (2006). The impact of storm events on solute exports from a glaciated forested watershed in western New York. USA. Hydrol. Process. 20, 3423–3439. doi: 10.1002/hyp.6141
Johnson, N. M., Likens, G. E., Bormann, F. H., Fisher, D. W., and Pierce, R. S. (1969). A working model for the variation in stream water chemistry at the hubbard brook experimental forest, New Hampshire. Water Resour. Res. 5, 1353–1363. doi: 10.1029/wr005i006p01353
Kim, H., Gu, X., and Brantley, S. L. (2018). Particle fluxes in groundwater change subsurface share rock chemistry over geologic time. Earth Planet. Sci. Lett. 500, 180–191. doi: 10.1016/j.epsl.2018.07.031
Koenig, L. E., Shattuck, M. D., Snyder, L. E., Potter, J. D., and McDowell, W. H. (2017). Deconstructing the effects of flow on DOC, nitrate, and major ion interactions using a high-frequency aquatic sensor network. Water Resour. Res. 53, 10655–10673. doi: 10.1002/2017WR020739
Larsen, M. C. (1997). Tropical Geomorphology and Geomorphicwork–A Study of Geomorphic Processes and Sediment and Water Budgets in Montane Humid-Tropical Forested and Developed Watersheds, Puerto Rico. Ph.D. Dissertation, University of Colorado, Boulder.
Larsen, M. C., Liu, Z., and Zou, X. (2012). “Effects of earthworms on slopewash, surface runoff, and fine litter transport on a humid tropical forested hillslope, Luquillo Experimental Forest, Puerto Rico,” in Water Quality and Landscape Processes of Four Watersheds in Eastern Puerto Rico: U.S. Geological Survey Professional Paper 1789, eds S. F. Murphy and R. F. Stallard (Reston, VA: U.S. Geol. Surv.), 179–198. doi: 10.3133/pp1789g
Larsen, M. C., and Torres-Sanchez, A. J. (1992). Landslides triggered by Hurricane Hugo in eastern Puerto Rico, September 1989. Caribb. J. Sci. 28, 113–125.
Larsen, M. C., Torres-Sanchez, A. J., and Conception, I. M. (1999). Slopewash, surface runoff and fine-litter transport in forest and landslide scars in humid-tropical steeplands, Luquillo Experimental Forest, Puerto Rico. Earth Surf. Process. Landforms. 24, 481–502. doi: 10.1002/(sici)1096-9837(199906)24:6<481::aid-esp967>3.3.co;2-7
Lawler, D. M., Petts, G. E., Foster, I. D. L., and Harper, S. (2006). Turbidity dynamics during spring storm events in an urban headwater river system: the Upper Tame, West Midlands, UK. Sci. Total Environ. 360, 109–126. doi: 10.1016/j.scitotenv.2005.08.032
Lewis, J. (1996). Turbidity-controlled suspended sediment sampling for runoff-event load estimation. Water Resour. Res. 32, 2299–2310. doi: 10.1029/96WR00991
Lloyd, C. E. M., Freer, J. E., Johnes, P. J., and Collins, A. L. (2016). Testing an improved index for analysing storm discharge–concentration hysteresis. Hydrol. Earth Sys. Sci. 20, 625–632. doi: 10.5194/hess-20-625-2016
Marcus, W. A. (1989). Lag-time routing of suspended-sediment concentrations during unsteady flow. Bull. Geol. Soc. Am. 101, 644–651. doi: 10.1130/0016-7606(1989)101<0644:ltross>2.3.co;2
McDowell, W. H., and Asbury, C. E. (1994). Export of carbon, nitrogen, and major ions from three tropical montane watersheds. Limnol. Oceanogr. 39, 111–125. doi: 10.4319/lo.1994.39.1.0111
McDowell, W. H., Bowden, W. B., and Asbury, C. E. (1992). Riparian nitrogen dynamics in two geomorphologically distinct tropical rain forest watersheds - subsurface solute patterns. Biogeochemistry 18, 53–75. doi: 10.1007/bf00002703
McDowell, W. H., Gines-Sanchez, C., Asbury, C. E., and Ramos Perez, C. R. (1990). Influence of sea salt aerosols and long range transport on precipitation chemistry at El Verde. Puerto Rico. Atmos. Environ. 24, 2813–2821. doi: 10.1016/0960-1686(90)90168-m
McDowell, W. H., Scatena, F. N., Waide, R. B., Brokaw, N., Camilo, G. R., Covich, A. P., et al. (2012). “Geographic and ecological setting of the Luquillo Mountains,” in A Caribbean Forest Tapestry: The Multidimensional Nature of Disturbance and Response, eds N. Brokaw, et al. (New York, NY: Oxford Univ. Press), 87–90.
McKnight, D. M., Boyer, E. W., Westerhoff, P. K., Doran, P. T., Kulbe, T., and Anderson, D. T. (2001). Spectrofluorometric characterization of dissolved organic matter for indication of precursor organic material and aromaticity. Limnol. Oceanogr. 46, 38–48. doi: 10.4319/lo.2001.46.1.0038
Murphy, S. F., Stallard, R. F., Scholl, M. A., González, G., and Torres-Sánchez, A. J. (2017). Reassessing rainfall in the Luquillo Mountains, Puerto Rico: local and global ecohydrological implications. PLoS One 12:e0180987. doi: 10.1371/journal.pone.0180987
National Research Council [NRC] (2001). Basic Research Opportunities in Earth Science. Washington, D. C: Natl. Acad. Press.
Olshansky, Y., White, A. M., Moravec, B. G., McIntosh, J., and Chorover, J. (2018). Subsurface pore water contributes to stream concentration-discharge relations across a snowmelt hydrograph. Front. Earth Sci. 6:181. doi: 10.3389/feart.2018.00181
Phillips, C. B., and Jerolmack, D. J. (2016). Self-organization of river channels as a critical filter on climate signals. Science 352:6286. doi: 10.1126/science.aad3348
Pike, A. S., Scatena, F. N., and Wohl, E. E. (2010). Lithological and fluvial control on the geomorphology of tropical montane stream channels in Puerto Rico. Earth Surf. Process. Landforms. 35, 1402–1417. doi: 10.1002/esp.1978
Potter, J. D., McDowell, W. H., Merriam, J. L., Peterson, B. J., and Thomas, S. M. (2010). Denitrification and total nitrate uptake in streams of a tropical landscape. Ecol. Appl. 20, 2104–2115. doi: 10.1890/09-1110.1
Raymond, P. A., and Saiers, J. E. (2010). Event controlled DOC export from forested watersheds. Biogeochemistry 100, 197–209. doi: 10.1007/s10533-010-9416-7
Rinaldi, M., Casagli, N., Dapporto, S., and Gargini, A. (2004). Monitoring and modeling of pore water pressure changes and riverbank stability during flow events. Earth Surf. Process. Landforms. 29, 237–254. doi: 10.1002/esp.1042
Rose, L. A., Karwan, D. L., and Godsey, S. E. (2018). Concentration-discharge relationships describe solute and sediment mobilization, reaction, and transport at event and longer timescales. Hydrol. Process. 32, 2829–2844. doi: 10.1002/hyp.13235
Rue, G. P., Rock, N. D., Gabor, R. S., Pitlick, J., Tfaily, M., and McKnight, D. M. (2017). Concentration-discharge relationships during an extreme event: contrasting behavior of solutes and changes to chemical quality of dissolved organic material in the Boulder Creek watershed during the September 2013 flood. Water. Resour. Res. 53, 5276–5297. doi: 10.1002/2016wr019708
Schlesinger, W. H., and Bernhardt, E. S. (2013). Biogeochemistry, 3rd. Edn. New York, NY: Academic Press, 688.
Seeger, M., Errea, M. P., Beguerıa, S., Arnáez, J., Martı, C., and Garcıa-Ruiz, J. M. (2004). Catchment soil moisture and rainfall characteristics as determinant factors for discharge/suspended sediment hysteretic loops in a small headwater catchment in the Spanish Pyrenees. J. Hydrol. 288, 299–311. doi: 10.1016/j.jhydrol.2003.10.012
Shanley, J. B., McDowell, W. H., and Stallard, R. F. (2011). Long-term patterns and short-term dynamics of stream solutes and suspended sediment in a rapidly weathering tropical watershed. Water Resour. Res. 47:W07515. doi: 10.1029/2010WR009788
Simon, A., Curini, A., Darby, S., and Langendoen, E. J. (2000). Bank and near-bank processes in an incised channel. Geomorphology 35, 193–217. doi: 10.1016/s0169-555x(00)00036-2
Smith, H. G., and Dragovich, D. (2009). Interpreting sediment delivery processes using suspended sediment-discharge hysteresis patterns from nested upland catchments, south-eastern Australia. Hydrol. Process. 23, 2415–2426. doi: 10.1002/hyp.7357
Stallard, R. F., and Murphy, S. F. (2012). “Water quality and mass transport in four watersheds in Puerto Rico,” in Water Quality and Landscape Processes of Four Watersheds in Eastern Puerto Rico: U.S. Geological Survey Professional Paper 1789, eds S. F. Murphy and R. F. Stallard (Reston, VA: U.S. Geol. Surv.), 113–152. doi: 10.3133/pp1789e
U.S. Geological Survey (2018). National Water Information System—Web interface. Reston, VA: U.S. Geol. Surv.
Vaughan, M. C. H., Bowden, W. B., Shanley, J. B., Vermilyea, A., Sleeper, R., Gold, A. J., et al. (2017). High-frequency dissolved organic carbon and nitrate measurements reveal differences in storm hysteresis and loading in relation to land cover and seasonality. Water Resour. Res. 53, 5345–5363. doi: 10.1002/2017wr020491
Venables, W. N., and Ripley, B. D. (2002). Modern Applied Statistics with S-Plus, 4th Edn. New York, NY: Springer.
White, A. F., Blum, A. E., Schulz, M. S., Vivit, D. V., Stonestrom, D. A., Larsen, M., et al. (1998). Chemical weathering in a tropical watershed, Luquillo Mountains, Puerto Rico: I. long-term versus short-term weathering fluxes. Geochim. Cosmochim. Acta 62, 209–226. doi: 10.1016/s0016-7037(97)00335-9
Williams, G. P. (1989). Sediment concentration versus water discharge during single hydrologic events. J. Hydrol. 111, 89–106. doi: 10.1016/0022-1694(89)90254-0
Wymore, A. S., Brereton, R. L., Ibarra, D. E., Maher, K., and McDowell, W. H. (2017). Critical zone structure controls concentration-discharge relationships and solute generation in forested tropical montane watersheds. Water Resour. Res. 53, 6279–6295. doi: 10.1002/2016WR020016
Keywords: Luquillo, hysteresis, storm events, sensors, specific conductance, turbidity, hurricanes
Citation: Wymore AS, Leon MC, Shanley JB and McDowell WH (2019) Hysteretic Response of Solutes and Turbidity at the Event Scale Across Forested Tropical Montane Watersheds. Front. Earth Sci. 7:126. doi: 10.3389/feart.2019.00126
Received: 01 February 2019; Accepted: 10 May 2019;
Published: 31 May 2019.
Edited by:
Nicole West, Central Michigan University, United StatesReviewed by:
Ronny Lauerwald, Free University of Brussels, BelgiumElizabeth Herndon, Kent State University, United States
Copyright © 2019 Wymore, Leon, Shanley and McDowell. This is an open-access article distributed under the terms of the Creative Commons Attribution License (CC BY). The use, distribution or reproduction in other forums is permitted, provided the original author(s) and the copyright owner(s) are credited and that the original publication in this journal is cited, in accordance with accepted academic practice. No use, distribution or reproduction is permitted which does not comply with these terms.
*Correspondence: Adam S. Wymore, adam.wymore@unh.edu