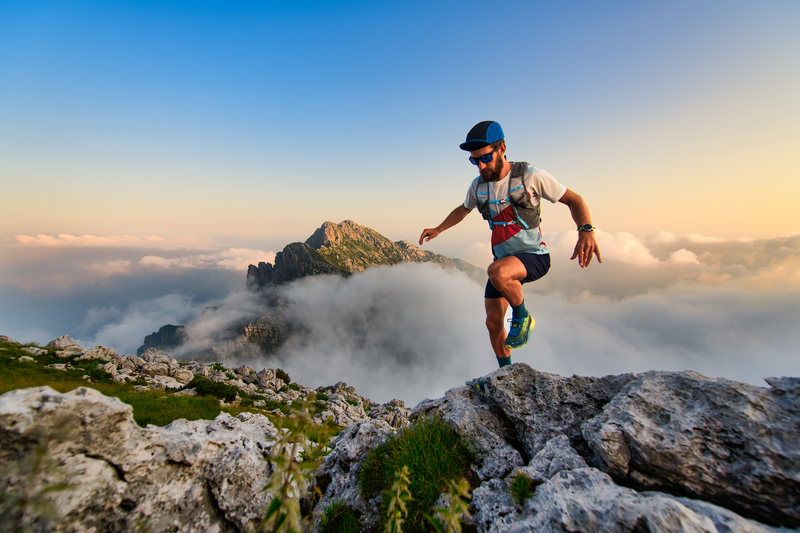
95% of researchers rate our articles as excellent or good
Learn more about the work of our research integrity team to safeguard the quality of each article we publish.
Find out more
MINI REVIEW article
Front. Earth Sci. , 07 March 2019
Sec. Geohazards and Georisks
Volume 7 - 2019 | https://doi.org/10.3389/feart.2019.00044
This article is part of the Research Topic Citizen Science: Reducing Risk and Building Resilience to Natural Hazards View all 14 articles
Pluvial flooding can have devastating effects, both in terms of loss of life and damage. Predicting pluvial floods is difficult and many cities do not have a hydrodynamic model or an early warning system in place. Citizen science and crowdsourcing have the potential for contributing to early warning systems (EWS) and can also provide data for validating flood forecasting models. Although there are increasing applications of citizen science and crowdsourcing in fluvial hydrology, less is known about activities related to pluvial flooding. Hence the aim of this paper is to review current activities in citizen science and crowdsourcing with respect to applications of pluvial flooding. Based on a search in Scopus, the papers were first filtered for relevant content and then classified into four main themes. The first two themes were divided into (i) applications relevant during a flood event, which includes automated street flooding detection using crowdsourced photographs and sensors, analysis of social media, and online and mobile applications for flood reporting; and (ii) applications related to post-flood events. The use of citizen science and crowdsourcing for model development and validation is the third theme while the development of integrated systems is theme four. All four main areas of research have the potential to contribute to EWS and build community resilience. Moreover, developments in one will benefit others, e.g., further developments in flood reporting applications and automated flood detection systems will yield data useful for model validation.
Over the period 1995–2015, 90% of disasters globally have been weather-related, e.g., due to floods, storms, and extreme temperatures (CRED and UNISDR, 2015). Of these events, 47% were due to flooding, which affected 2.3 billion people globally. Flooding can be caused by different factors (Doornkamp, 1998; Falconer et al., 2009; Hammond et al., 2015). Fluvial flooding occurs when river levels rise to due to heavy rainfall, snowmelt, rain on snow, dam collapse or sudden ice melting due to volcanic activity or ice dam breaching in a partially frozen river in winter. When water levels exceed the river banks, the surrounding areas are flooded. Groundwater flooding can occur due to groundwater rise, which can cause large-scale flooding. Coastal flooding is caused by storm surges due to large storm events, e.g., hurricanes or cyclones, rising sea levels due to climate change or from tsunamis. Pluvial flooding occurs when overland flow and ponding are generated by rainfall before it enters a drainage or sewer system, or a water course, or because the capacity of the network is full, flooding streets and nearby structures. Pluvial flooding is often only a few centimeters in depth but can cause considerable damage to houses and other assets. It occurs more frequently in urban areas because of higher concentration of impervious surfaces (Maddox, 2014). This is in contrast to surface water flooding, which refers to combined pluvial and fluvial flooding in urban areas, and flash flooding, which is generally associated with high-intensity rainfall arising from a watercourse (Falconer et al., 2009), both of which can lead to asset damage and loss of life.
With climate change, the frequency and severity of extreme events and hence weather-related natural disasters will most likely increase (IPCC, 2014), affecting greater numbers of people through flooding. Early warning systems (EWS) are one area of response where more efforts could be deployed, particularly in areas with vulnerable populations (CRED and UNISDR, 2015). Cools et al. (2016) recommend the need to engage local communities, both in the design of EWS but also in data collection, where the information can be used to calibrate and validate flood forecasting models or to refine the thresholds of the early warning alerts issued. The involvement of citizens in data collection for environmental monitoring or scientific research is becoming increasingly common, and is often referred to as citizen science or crowdsourcing. Citizen science is the involvement of citizens in the scientific process, where the degree of involvement can range from tasks such as data collection to full involvement in research design (Bonney et al., 2009). Crowdsourcing is the outsourcing of tasks to a crowd that would otherwise be too large to accomplish by a single organization (Howe, 2006). However, crowdsourcing can also be used in the context of tasks undertaken by citizens for any purpose including data collection. Citizen science has a strong tradition in the fields of conservation and biodiversity (See et al., 2016) yet more recently, citizen science and crowdsourcing are being used within many different domains in the geosciences (Zheng et al., 2018). Many of the citizen science and crowdsourcing applications in hydrology are focused on water quality (Jollymore et al., 2017) or river flooding (Assumpção et al., 2018). Yet an area where citizen science and crowdsourcing might be very helpful is in applications related to pluvial flooding, where citizens could report the occurrence of flooding in real-time, improving the spatial and temporal availability of information for disaster response and management. The aim of this paper is to establish the current situation with regard to citizen science, crowdsourcing and pluvial flooding, and to reflect on future directions in this field.
In the literature, pluvial flooding is not always explicitly referred to using this terminology. Hence to find an initial set of papers, the Scopus facility was searched using the following expression:
Although this will not include every paper written on this topic or the non-English literature, these results should represent an adequate sample for determining the latest trends in this field. The search yielded 129 papers, of which 31 were removed due to irrelevance, e.g., papers with sentences such as “the flood of data” or a topic that mentions flooding but is not directly related.
The papers were then classified based on the type of flooding addressed, i.e., pluvial, fluvial, groundwater or coastal flooding, multiple flood types (e.g., surface water flooding) or unspecified, to isolate those papers dealing primarily with pluvial flooding or those applicable to any type of flooding. These remaining papers (around 50) were then categorized into a set of main themes. It was clear that some papers presented applications during flood events while others were related to post-flood event analysis. Another set was related to the validation of models or providing information to forecasting models. The remaining papers fell into a group called integrated systems, which dealt with bringing information together from different sources. These became the four themes. Within the main themes, further sub-themes were extracted based on the methodologies employed and the data used.
Table 1 summarizes the themes and sub-themes with indicative references; more detail is provided below.
Table 1. The main themes in the literature regarding citizen science, crowdsourcing and pluvial flooding (or with potential for application to pluvial flooding), with indicative references.
One clear area of research is in automated flood detection from two sources. Crowdsourced photographs are the first source, where Witherow et al. (2018a,b) present a workflow for determining if streets are flooded from pairs of crowdsourced photographs that show flooded and non-flooded scenes. Despite problems related to differences in resolution, lighting and the presence of unwanted vehicles in the photographs, the results are promising. Overall accuracies in detecting the area of inundation ranged from 72 to 95% depending on the location. A second area of research is in the use of sensors that automatically detect flooded roads. Wu and Wang (2014) describe how sensors installed on the side of roads in Oregon trigger alerts when they become submerged and reach a threshold, sending messages to a central system and to drivers in the area. Automatic detection systems have also been developed in the Philippines. De Guzman et al. (2016) designed and tested a flood detection system that uses Arduinos and ultrasonic sensors to automatically detect floods on road surfaces along with cameras for monitoring the situation. A similar system has been developed by Amagsila et al. (2018), but it additionally includes a mobile app for drivers to receive flood warnings and make flood reports in places where Arduino sensors are not located. Although promising, the system does not yet appear to be operational.
Another prominent area of research is in the analysis of data from social media, primarily Twitter. Twitter is a passive source of crowdsourced information and has been analyzed to create flood maps in real-time (Arthur et al., 2018), to detect, cluster and map flood events or to categorize different types of flood-related information (Kiatpanont et al., 2016; Pandey and Natarajan, 2016; Albahari and Schultz, 2017; Feng and Sester, 2018; Lin et al., 2018; Bhuvaneswari and Valliyammai, 2019). Twitter has also been used in a more active manner in the PetaJakarta application (Holderness and Turpin, 2015; Ogie and Forehead, 2018; Ogie et al., 2018; Yang et al., 2018). Citizens in Jakarta are very active on Twitter, e.g., 2.4% of global tweets in 2012 originated from Jakarta (Holderness and Turpin, 2015). Hence a system was set up to identify tweets related to flooding (‘unconfirmed reports), where the system then contacted the same individuals via Twitter for more information in order to become “confirmed” reports of flooding. One type of tweet sent to the system was about flood conditions, which could include the height of the water, either in cm or m, or as a description, e.g., knee height, with a photograph. From this, maps of affected areas have been produced in real-time. However, there are uncertainties in the accuracy of the tweet locations in relation to the actual flooding as well as the need to better align the outputs with the needs of the local authorities. Similarly, during the 2015 rains in Chennai, Twitter and Facebook were used to disseminate information to the public and as a source of communication for those stranded or trying to locate missing family and friends (Yadav and Rahman, 2016).
The development of applications that allow for online reporting or reporting of floods using a mobile application is another area of active research. Ushahidi was developed in Kenya as a website for crowdsourcing and sharing of information (Okolloh, 2009); in this context it has been used to report floods in Australia (Koswatte et al., 2015, 2016, 2018) and to develop a crisis map of the Czechia during the 2013 floods (Pánek et al., 2017). Other online reporting systems include the Flooded Streets application for reporting street flooding in Chennai during the 2015 floods (Naik, 2016), the CrowdMonitor application, which assigns data gathering tasks to citizens during an emergency of different kinds, including any kind of flooding (Ludwig et al., 2015) and flood reporting via the Philippines Flood Hazard Maps application (Victorino et al., 2016). The latter application is also supported by the Flood Patrol mobile app (Victorino and Estuar, 2014). In addition to online reporting, a number of papers outline different mobile apps for flood reporting (Liu et al., 2011; Le Coz et al., 2016; Middelhoff et al., 2017; Minn et al., 2017; Sahay et al., 2017; Hendricks et al., 2018; Wang et al., 2018; Yabut et al., 2018). Le Coz et al. (2016) provide an overview of different mobile apps developed in Argentina, France, and New Zealand while the other papers outline individual apps. Most of the papers deal with the development of a bespoke app for flood reporting, e.g., in the Philippines, India, United States, and Vietnam. However, some of the apps presented were more generic, i.e., for reporting different types of disaster (Liu et al., 2011), for tasking the crowd with specific data collection activities in the context of a flood emergency (Middelhoff et al., 2017) or an app for infrastructure surveying of ditches, culverts, and drains (Hendricks et al., 2018), i.e., for flood preparedness.
Three papers specifically addressed data collection by citizens after a large event to aid in the assessment of damage. For a large hurricane event in 2014, Kim et al. (2015) used Ushahidi to crowdsource damage due to fallen trees and from storm surge or flooding in Hawaii. Molinari et al. (2014) developed their own system called Poli-RISPOSTA to collect flood damage information following flood events in Italy in support of the EU Floods Directive. Schnebele et al. (2014) developed a methodology to generate a road damage map for New York City after Hurricane Sandy. Authoritative data were merged with videos from YouTube and Twitter data to fill in gaps in information, both spatially and temporally, demonstrating the effectiveness of such an approach.
Early warning systems need a sensor network or a model in order to issue alerts. Crowdsourcing can provide a source of validation for these models. Four studies have used crowdsourcing to validate hydrodynamic models in Shanghai (Yu et al., 2016), two in the United States in New Jersey (Blumberg et al., 2015) and Virginia (Loftis et al., 2017) and one in the city of Newcastle in the United Kingdom (Kutija et al., 2014). However, the source of crowdsourced information differed in the studies. In Yu et al. (2016), flood reports from citizens were collected using an emergency reporting portal while crowdsourced flood extents were captured using a mobile app in Loftis et al. (2017). In both the studies by Blumberg et al. (2015) and Kutija et al. (2014), the public was approached for the information post-event, i.e., for photographs and flood reports through an email and questionnaire, respectively. The results showed satisfactory performance in all four models. For example, in Yu et al. (2016), the results showed that the model is capable of predicting the broad pattern of flooded areas across the city as well as the flood timing while the results by Blumberg et al. (2015) were satisfactory when comparing watermarks, inundation depths and overall flood extent from the two sources.
In addition to flood models, improved nowcasting, which uses multiple sources of data including reports from social media and data from amateur weather stations, can also provide much needed inputs to pluvial EWS. Lewis and Silkstone (2017) analyzed three severe thunderstorms in northern England and used social media, photographs and video to verify impacts such as surface water flooding and hail in real-time. Data from amateur weather stations allowed United Kingdom Met Office staff to track the progression of a storm that was not possible using the official observing network alone. A final study in this area of research is by Smith and Rodriguez (2017), who examined how crowdsourced data from call centers could be used in combination with radar and rainfall data to help understand the characteristics of flooding (flash, pluvial, and coastal surge) in New York city. Such data could potentially be used to build data-driven models for early warning purposes.
The final area in which research is taking place is in the development of systems that can integrate data from different sources, including crowdsourced data, for flood risk management. The first is an example from Brazil where de Assis et al. (2018) present a middleware architecture that can integrate any type of sensor using open standards with unstructured crowdsourced data from citizen reports, demonstrated on four flood events. Although there may be other national or regional examples, these were not found in the literature search.
The other examples are more global in nature. For example, Leyh (2018) presented the design of an interface to the OpenStreetMap (OSM) application to provide a standardized data collection system for surface water features, which can cover different types of flooding. This could encourage volunteers to use OSM as a platform for flood reporting that could be accessed by any city in the world for early warning purposes. The cloud-based system developed by Wan et al. (2014) has similarities to the previous application, i.e., building an infrastructure (called CyberFlood) around an existing database, in this case the Global Flood Inventory, to access the data for visualization, model validation, management of flood risks and awareness raising. The interface also has an online reporting module where users can upload flood observations, thereby enhancing the data from the Global Flood Inventory.
This paper has summarized the state-of-the-art in citizen science, crowdsourcing and pluvial flooding based on a review of the academic literature. Most papers are from 2014 and later, indicating a relatively new and emerging area of research. The topic with the largest number of papers was on the use of Twitter and other social media for flooding applications. These are not necessarily related to pluvial flooding alone but can be used to detect flood events of any nature and even create flood maps in real-time. An example is PetaJakarta, which has taken advantage of the willingness of Jakarta residents to use Twitter and turned it into an active source of flood reports. Applications such as these will be much more effective than passive data mining of Twitter, and should see transference to other cities in the future.
Despite the fact that the use of sensors for automatic flood detection was only described in a small number of papers, this is clearly an area of future growth. As sensors become cheaper and with new open standards for sensor networks now available, more cities plagued by frequent pluvial flooding may adopt this type of technological solution in the development of EWS, once it becomes more developed. Moreover, with advances in computer vision, applications of automatic flood detection from photographs is an area where there is much potential. Yet automation alone is not the answer and the role of citizens is still critical. For example, they can provide verification of flooding when alerts are received or make flood reports where sensors are not available, increasing both the spatial and temporal resolution of the information. Examples can be found in the EU-funded WeSenseIt, Scent, Ground Truth 2.0, and FloodCitiSense projects, which have or are providing information to EWS with information about flooding conditions, either in real-time or in post-flood analysis. Numerous developments in online platforms and mobile applications for flood reporting clearly indicate the value of citizens in flood risk management and building of resilience, particularly in terms of awareness raising and engagement in a larger observation network. More examples of these types of applications will, undoubtedly, appear in the future.
There is clearly great potential for using data collected by citizens for model development and validation as evidenced by the papers on this topic. The amount of data collected in the different modeling examples was actually not that large yet can still provide an effective form of validation. As more web- and mobile-based flood reporting systems are developed, more data will become available for this task. The development of more integrated systems may also facilitate this process as citizen observations become one data stream of many in a flood forecasting and EWS. Moreover, building interfaces to existing systems such as OSM and the Global Flood Inventory are promising developments. OSM already has a vast number of contributors and could provide standardized flood reporting that could be accessed by any city or used for model validation. However, the quality of data from citizen science and crowdsourcing has been raised as an issue of concern more generally; see e.g., Flanagin and Metzger (2008) and Lukyanenko et al. (2016). These concerns are being addressed through numerous methods now available for ensuring quality (Goodchild and Li, 2012; Kosmala et al., 2016; Fonte et al., 2017) while training and regular feedback have been shown to improve data quality and volunteer retention (Kosmala et al., 2016).
Teasing out pluvial flooding from the papers was difficult in some instances as some papers could include surface water flooding or other flooding types. In other cases, applications were more generic but could be applied to pluvial flooding. Despite these limitations, the four main themes were clearly in evidence, all of which should see continued research and development in the future.
LS did the literature search, classified the papers by theme, and wrote the manuscript.
This paper has been supported by the ENSUF FloodCitiSense project (#860918), funded in Austria by the Austrian Agency for the Promotion of Science (FFG).
The author declares that the research was conducted in the absence of any commercial or financial relationships that could be construed as a potential conflict of interest.
Albahari, A., and Schultz, C. H. (2017). A qualitative analysis of the spontaneous volunteer response to the 2013 Sudan floods: changing the paradigm. Prehosp. Disaster Med. 32, 240–248. doi: 10.1017/S1049023X17000164
Amagsila, G. A., Cabuhat, M. E., Tigbayan, J. E., Uy, E., and Ramirez, E. (2018). “A framework for mobile application of flood alert monitoring system for vehicle users using Arduino device,” in Proceedings of the 9th International Conference on Humanoid, Nanotechnology, Information Technology, Communication and Control, Environment and Management HNICEM 2017 (Manila: IEEE), 1–6. doi: 10.1109/HNICEM.2017.8269431
Arthur, R., Boulton, C. A., Shotton, H., and Williams, H. T. P. (2018). Social sensing of floods in the UK. PLoS One 13:e0189327. doi: 10.1371/journal.pone.0189327
Assumpção, T. H., Popescu, I., Jonoski, A., and Solomatine, D. P. (2018). Citizen observations contributing to flood modelling: Opportunities and challenges. Hydrol. Earth Syst. Sci. 22, 1473–1489. doi: 10.5194/hess-22-1473-2018
Bhuvaneswari, A., and Valliyammai, C. (2019). Social IoT-enabled emergency event detection framework using geo-tagged microblogs and crowdsourced photographs. Adv. Intell. Syst. Comput. 814, 151–162. doi: 10.1007/978-981-13-1501-5_13
Blumberg, A. F., Georgas, N., Yin, L., Herrington, T. O., and Orton, P. M. (2015). Street-scale modeling of storm surge inundation along the New Jersey Hudson river waterfront. J. Atmos. Ocean. Technol. 32, 1486–1497. doi: 10.1175/JTECH-D-14-00213.1
Bonney, R., Cooper, C. B., Dickinson, J., Kelling, S., Phillips, T., Rosenberg, K. V., et al. (2009). Citizen science: a developing tool for expanding science knowledge and scientific literacy. Bioscience 59, 977–984. doi: 10.1525/bio.2009.59.11.9
Cools, J., Innocenti, D., and O’Brien, S. (2016). Lessons from flood early warning systems. Environ. Sci. Policy 58, 117–122. doi: 10.1016/j.envsci.2016.01.006
CRED and UNISDR (2015). The Human Cost of Weather Related Disasters 1995-2015. Available at: https://www.preventionweb.net/files/46796_cop21weatherdisastersreport2015.pdf
de Assis, L. F. F. G., Horita, F. E. A., de Freitas, E. P., Ueyama, J., and de Albuquerque, J. P. (2018). A service-oriented middleware for integrated management of crowdsourced and sensor data streams in disaster management. Sensors 18:1689. doi: 10.3390/s18061689
De Guzman, E., Cuadra, V. S., Grace de Luna, A., and Villanueva, C. (2016). “Flood detector system using Arduino,” in Proceedings of the 37th IRES International Conference, Bangkok.
Doornkamp, J. C. (1998). Coastal flooding, global warming and environmental management. J. Environ. Manag. 52, 327–333. doi: 10.1006/jema.1998.0188
Falconer, R. H., Cobby, D., Smyth, P., Astle, G., Dent, J., and Golding, B. (2009). Pluvial flooding: new approaches in flood warning, mapping and risk management. J. Flood Risk Manag. 2, 198–208. doi: 10.1111/j.1753-318X.2009.01034.x
Feng, Y., and Sester, M. (2018). Extraction of pluvial flood relevant volunteered geographic information (VGI) by deep learning from user generated texts and photos. ISPRS Int. J. Geo Inf. 7:39. doi: 10.3390/ijgi7020039
Flanagin, A., and Metzger, M. (2008). The credibility of volunteered geographic information. Geo J. 72, 137–148. doi: 10.1007/s10708-008-9188-y
Fonte, C. C., Antoniou, V., Bastin, L., Estima, J., Arsanjani, J. J., Laso-Bayas, J.-C., et al. (2017). “Assessing VGI data quality,” in Mapping and the Citizen Sensor, eds G. M. Foody, L. See, S. Fritz, C. C. Fonte, P. Mooney, A.-M. Olteanu-Raimond, et al. (London: Ubiquity Press), 137–164.
Goodchild, M. F., and Li, L. (2012). Assuring the quality of volunteered geographic information. Spat. Stat. 1, 110–120. doi: 10.1016/j.spasta.2012.03.002
Hammond, M. J., Chen, A. S., Djordjević, S., Butler, D., and Mark, O. (2015). Urban flood impact assessment: a state-of-the-art review. Urban Water J. 12, 14–29. doi: 10.1080/1573062X.2013.857421
Hendricks, M. D., Meyer, M. A., Gharaibeh, N. G., Van Zandt, S., Masterson, J., Cooper, J., et al. (2018). The development of a participatory assessment technique for infrastructure: neighborhood-level monitoring towards sustainable infrastructure systems. Sustain. Cities Soc. 38, 265–274. doi: 10.1016/j.scs.2017.12.039
Holderness, T., and Turpin, E. (2015). “From social media to geosocial intelligence: crowdsourcing civic co-management for flood response in Jakarta, Inddonesia,” in Social Media for Government Services, eds S. Nepal, C. Paris, and D. Georgakopoulos (Cham: Springer), 115–133. doi: 10.1007/978-3-319-27237-5_6
IPCC (2014). “Climate Change 2014: impacts, Adaptation, and Vulnerability. Part A: global and Sectoral Aspects,” in Proceedings of the Contribution of Working Group II to the Fifth Assessment Report of the Intergovernmental Panel on Climate Change, eds C. B. Field, V. R. Barros, D. J. Dokken, K. J. Mach, M. D. Mastrandrea, T. E. Bilir, et al. (Cambridge: Cambridge University Press), 1132.
Jollymore, A., Haines, M. J., Satterfield, T., and Johnson, M. S. (2017). Citizen science for water quality monitoring: data implications of citizen perspectives. J. Environ. Manag. 200, 456–467. doi: 10.1016/j.jenvman.2017.05.083
Kiatpanont, R., Tanlamai, U., and Chongstitvatana, P. (2016). Extraction of actionable information from crowdsourced disaster data. J. Emerg. Manag. 14, 377–390. doi: 10.5055/jem.2016.0302
Kim, K., Pant, P., and Yamashita, E. (2015). “Disasters, drones, and crowd-sourced damage assessment,” in Proceedings of the 14th International Conference on Computers in Urban Planning and Urban Management CUPUM 2015 (Cambridge, MA: MIT Press).
Kosmala, M., Wiggins, A., Swanson, A., and Simmons, B. (2016). Assessing data quality in citizen science. Front. Ecol. Environ. 14, 551–560. doi: 10.1002/fee.1436
Koswatte, S., McDougall, K., and Liu, X. (2015). SDI and crowdsourced spatial information management automation for disaster management. Survey Rev. 47, 307–315. doi: 10.1179/1752270615Y.0000000008
Koswatte, S., Mcdougall, K., and Liu, X. (2016). “Semantic location extraction from crowdsourced data,” in Proceedings of the International Archives of the Photogrammetry, Remote Sensing and Spatial Information Sciences - ISPRS Archives, Prague, 543–547. doi: 10.5194/isprsarchives-XLI-B2-543-2016
Koswatte, S., McDougall, K., and Liu, X. (2018). VGI and crowdsourced data credibility analysis using spam email detection techniques. Int. J. Dig. Earth 11, 520–532. doi: 10.1080/17538947.2017.1341558
Kutija, V., Bertsch, R., Glenis, V., Alderson, D., Parkin, G., Walsh, C. L., et al. (2014). “Model valiation using crowd-sourced data from a large pluvial flood,” in Proceedings of the 11th International Conference on Hydroinformatics (HIC 2014), (New York, NY: Curran Associates, Inc.).
Le Coz, J., Patalano, A., Collins, D., Guillén, N. F., García, C. M., Smart, G. M., et al. (2016). Crowdsourced data for flood hydrology: feedback from recent citizen science projects in Argentina, France and New Zealand. J. Hydrol. 541, 766–777. doi: 10.1016/j.jhydrol.2016.07.036
Lewis, M. W., and Silkstone, N. (2017). Improvements in nowcasting capability: analysis of three structurally distinct severe thunderstorms across northern England on 1 July 2015. Weather 72, 91–98. doi: 10.1002/wea.2837
Leyh, W. (2018). A conceptual building-block and practical openstreetmap-interface for sharing references to hydrologic features. Adv. Intell. Syst. Comput. 600, 137–148. doi: 10.1007/978-3-319-60450-3_14
Lin, W.-Y., Wu, T.-H., Tsai, M.-H., Hsu, W.-C., Chou, Y.-T., and Kang, S.-C. (2018). Filtering disaster responses using crowdsourcing. Autom. Constr. 91, 182–192. doi: 10.1016/j.autcon.2018.03.016
Liu, Y., Piyawongwisal, P., Handa, S., Yu, L., Xu, Y., and Samuel, A. (2011). “Going beyond citizen data collection with mapster: a mobile++cloud real-time citizen science experiment,” in Proceedings - 7th IEEE International Conference on e-Science Workshops, eScienceW (Stockholm: IEEE), 1–6. doi: 10.1109/eScienceW.2011.23
Loftis, J. D., Wang, H., Forrest, D., Rhee, S., and Nguyen, C. (2017). “Emerging flood model validation frameworks for street-level inundation modeling with storm sense,” in Proceedings - 2017 2nd International Workshop on Science of Smart City Operations and Platforms Engineering, in partnership with Global City Teams Challenge. SCOPE, Pittsburg, PA, 13–18. doi: 10.1145/3063386.3063764
Ludwig, T., Siebigteroth, T., and Pipek, V. (2015). Crowdmonitor: monitoring physical and digital activities of citizens during emergencies. Lecture Notes Comput. Sci. 8852, 421–428. doi: 10.1007/978-3-319-15168-7_51
Lukyanenko, R., Parsons, J., and Wiersma, Y. F. (2016). Emerging problems of data quality in citizen science: editorial. Conserv. Biol. 30, 447–449. doi: 10.1111/cobi.12706
Maddox, I. (2014). The Risks of Hazard. Three Common Types of Flood Explained. Available at: https://www.intermap.com/risks-of-hazard-blog/three-common-types-of-flood-explained
Middelhoff, M., Widera, A., Van Den Berg, R. P., Hellingrath, B., Anferbauer, D., Havlik, D., et al. (2017). “Crowdsourcing and crowdtasking in crisis management lessons learned from a field experiment simulating a flooding in the city of the hague,” in Proceedings of the 2016 3rd International Conference on Information and Communication Technologies for Disaster Management, ICT-DM 2016 (Vienna: IEEE). doi: 10.1109/ICT-DM.2016.7857212
Minn, Q. T., Chi, T. N., and Toulouse, M. (2017). “Toward a crowdsourcing-based urban flood mitigation platform,” in Proceedings of the ACM International Conference Proceeding Series, Nha Trang City, 301–308. doi: 10.1145/3155133.3155153
Molinari, D., Mazuran, M., Arias, C., Minucci, G., Atun, F., and Ardagna, D. (2014). Implementing tools to meet the Floods Directive requirements: a “procedure” to collect, store and manage damage data in the aftermath of flood events. WIT Trans. Ecol. Environ. 184, 215–226. doi: 10.2495/FRIAR140181
Naik, N. (2016). “Flooded streets-A crowdsourced sensing system for disaster response: a case study,” in Proceedings of the ISSE 2016 - 2016 International Symposium on Systems Engineering (Edinburgh: IEEE). doi: 10.1109/SysEng.2016.7753186
Ogie, R. I., Clarke, R. J., Forehead, H., and Perez, P. (2018). Crowdsourced social media data for disaster management: lessons from the PetaJakarta.org project. Comput. Environ. Urban Syst. 73, 108–117. doi: 10.1016/j.compenvurbsys.2018.09.002
Ogie, R. I., and Forehead, H. (2018). “Investigating the accuracy of georeferenced social media data for flood mapping: the PetaJakarta.org case study,” in Proceedings of the 2017 4th International Conference on Information and Communication Technologies for Disaster Management. ICT-DM (Piscataway, NJ: IEEE), 1–6. doi: 10.1109/ICT-DM.2017.8275672
Okolloh, O. (2009). “Ushahidi, or ‘testimony’: Web 2.0 tools for crowdsourcing crisis information,” in Change at hand: Web 2.0 for development Participatory learning and action, ed. H. Ashley (London: International Institute for Environment and Development), 65–70.
Pandey, N., and Natarajan, S. (2016). “How social media can contribute during disaster events? Case study of Chennai floods 2015,” in Proceedings of the International Conference on Advances in Computing, Communications and Informatics, ICACCI 2016 (Jaipur: IEEE), 1352–1356. doi: 10.1109/ICACCI.2016.7732236
Pánek, J., Marek, L., Pászto, V., and Valůch, J. (2017). The Crisis Map of the Czech Republic: the nationwide deployment of an Ushahidi application for disasters. Disasters 41, 649–671. doi: 10.1111/disa.12221
Sahay, A., Kumar, A. A., Pongpaichet, S., and Jain, R. (2017). “Multimedia rescue systems for floods,” in Proceedings of the 9th International Conference on Management of Digital EcoSystems, Bangkok, 210–215. doi: 10.1145/3167020.3167052
Schnebele, E., Cervone, G., and Waters, N. (2014). Road assessment after flood events using non-authoritative data. Nat. Hazards Earth Syst. Sci. 14, 1007–1015. doi: 10.5194/nhess-14-1007-2014
See, L., Mooney, P., Foody, G., Bastin, L., Comber, A., Estima, J., et al. (2016). Crowdsourcing, citizen science or Volunteered Geographic Information? The current state of crowdsourced geographic information. ISPRS Int. J. Geo Inf. 5:55. doi: 10.3390/ijgi5050055
Smith, B., and Rodriguez, S. (2017). Spatial analysis of high-resolution radar rainfall and citizen-reported flash flood data in ultra-urban New York City. Water 9:736. doi: 10.3390/w9100736
Victorino, J. N. C., and Estuar, M. R. J. E. (2014). “Profiling flood risk through crowdsourced flood level reports,” in Proceedings of the 2014 International Conference on IT Convergence and Security, ICITCS 2014 (Beijing: IEEE). doi: 10.1109/ICITCS.2014.7021800
Victorino, J. N. C., Estuar, M. R. J. E., and Lagmay, A. M. F. A. (2016). “Validating the voice of the crowd during disasters,” in Lecture Notes in Computer Science (including subseries Lecture Notes in Artificial Intelligence and Lecture Notes in Bioinformatics) 9708 LNCS, eds K. Xu, D. Reitter, D. Lee, and N. Osgood, (Cham: Springer), 301–310. doi: 10.1007/978-3-319-39931-7_29
Wan, Z., Hong, Y., Khan, S., Gourley, J., Flamig, Z., Kirschbaum, D., et al. (2014). A cloud-based global flood disaster community cyber-infrastructure: development and demonstration. Environ. Modell. Softw. 58, 86–94. doi: 10.1016/j.envsoft.2014.04.007
Wang, R.-Q., Mao, H., Wang, Y., Rae, C., and Shaw, W. (2018). Hyper-resolution monitoring of urban flooding with social media and crowdsourcing data. Comput. Geosci. 111, 139–147. doi: 10.1016/j.cageo.2017.11.008
Witherow, M. A., Elbakary, M. I., Iftekharuddin, K. M., and Cetin, M. (2018a). “Analysis of crowdsourced images for flooding detection,” in Lecture Notes in Computational Vision and Biomechanics, Vol. 27, eds J. Avares and R. Natal Jorge, (Cham: Springer), 140–149. doi: 10.1007/978-3-319-68195-5_15
Witherow, M. A., Sazara, C., Winter-Arboleda, I. M., Elbakary, M. I., Cetin, M., and Iftekharuddin, K. M. (2018b). Floodwater detection on roadways from crowdsourced images. Comput. Methods Biomech. Biomed. Eng. doi: 10.1080/21681163.2018.1488223
Wu, Y., and Wang, Y. (2014). “A portable flood detection system using heterogeneous sensor networks,” in Proceeding of the 2014 IEEE 33rd International Performance Computing and Communications Conference (IPCCC), (Austin, TX: IEEE), 1–2. doi: 10.1109/PCCC.2014.7017026
Yabut, E. R., Balceda, C. D., Juan, R. E. Q. S., Tumamak, J. R., Velasquez, R. E., Jamis, M. N., et al. (2018). “e-wasBaha: A mobile application framework for flood monitoring in Metro Manila using crowdsourcing,” in Proceedings of the 9th International Conference on Humanoid, Nanotechnology, Information Technology, Communication and Control, Environment and Management HNICEM 2017 (Manila: IEEE), 1–6. doi: 10.1109/HNICEM.2017.8269533
Yadav, M., and Rahman, Z. (2016). The social role of social media: the case of Chennai rains-2015. Soc. Netw. Anal. Min. 6:101. doi: 10.1007/s13278-016-0410-5
Yang, K., Michael, K., Abbas, R., and Holderness, T. (2018). “Urban flood modelling using geo-social intelligence,” in Proceedings of the International Symposium on Technology and Society, Proceedings (Sydney, NSW: IEEE), 1–9. doi: 10.1109/ISTAS.2017.8319086
Yu, D., Yin, J., and Liu, M. (2016). Validating city-scale surface water flood modelling using crowd-sourced data. Environ. Res. Lett. 11:124011. doi: 10.1088/1748-9326/11/12/124011
Keywords: pluvial flooding, urban flooding, citizen science, crowdsourcing, mobile apps, sensors
Citation: See L (2019) A Review of Citizen Science and Crowdsourcing in Applications of Pluvial Flooding. Front. Earth Sci. 7:44. doi: 10.3389/feart.2019.00044
Received: 01 November 2018; Accepted: 22 February 2019;
Published: 07 March 2019.
Edited by:
Jonathan D. Paul, Imperial College London, United KingdomReviewed by:
Joern Lauterjung, Helmholtz Centre Potsdam, GermanyCopyright © 2019 See. This is an open-access article distributed under the terms of the Creative Commons Attribution License (CC BY). The use, distribution or reproduction in other forums is permitted, provided the original author(s) and the copyright owner(s) are credited and that the original publication in this journal is cited, in accordance with accepted academic practice. No use, distribution or reproduction is permitted which does not comply with these terms.
*Correspondence: Linda See, c2VlQGlpYXNhLmFjLmF0
Disclaimer: All claims expressed in this article are solely those of the authors and do not necessarily represent those of their affiliated organizations, or those of the publisher, the editors and the reviewers. Any product that may be evaluated in this article or claim that may be made by its manufacturer is not guaranteed or endorsed by the publisher.
Research integrity at Frontiers
Learn more about the work of our research integrity team to safeguard the quality of each article we publish.