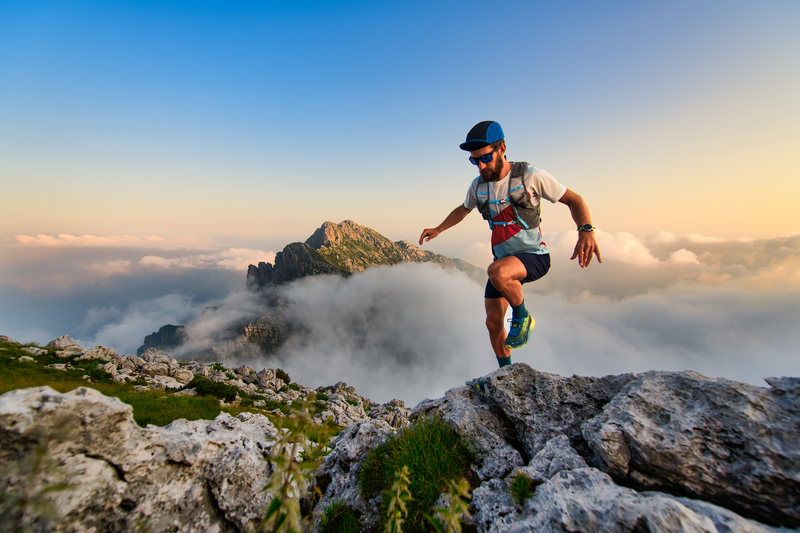
94% of researchers rate our articles as excellent or good
Learn more about the work of our research integrity team to safeguard the quality of each article we publish.
Find out more
ORIGINAL RESEARCH article
Front. Earth Sci. , 12 February 2018
Sec. Cryospheric Sciences
Volume 6 - 2018 | https://doi.org/10.3389/feart.2018.00008
This article is part of the Research Topic Climate Impacts on Glaciers and Biosphere in Fuego-Patagonia View all 11 articles
We compare two independent estimates of the rate of elevation change and geodetic mass balance of the Northern Patagonian Icefield (NPI) between 2000 (3,856 km2) and 2012 (3,740 km2) from space-borne data. The first is obtained by differencing the Shuttle Radar Topography Mission (SRTM) digital elevation model (DEM) from February 2000 and a Satellite pour l'Observation de la Terre 5 (SPOT5) DEM from March 2012. The second is deduced by fitting pixel-based linear elevation trends over 118 DEMs calculated from Advanced Spaceborne Thermal Emission and Reflection Radiometer (ASTER) stereo images acquired between 2000 and 2012. Both methods lead to similar and strongly negative icefield-wide mass balance rates of −1.02 ± 0.21 and −1.06 ± 0.14 m w.e. yr−1 respectively, which is in agreement with earlier studies. Contrasting glacier responses are observed, with individual glacier mass balance rates ranging from −0.15 to −2.30 m w.e. yr−1 (standard deviation = 0.49 m w.e. yr−1; N = 38). For individual glaciers, the two methods agree within error bars, except for small glaciers poorly sampled in the SPOT5 DEM due to clouds. Importantly, our study confirms the lack of penetration of the C-band SRTM radar signal into the NPI snow and firn except for a region above 2,900 m a.s.l. covering <1% of the total area. Ignoring penetration would bias the mass balance by only 0.005 m w.e. yr−1. A strong advantage of the ASTER method is that it relies only on freely available data and can thus be extended to other glacierized areas.
Patagonian glaciers and icefields, including the Northern Patagonian Icefield (NPI), are essential indicators of climate change in the southern hemisphere and also stand as one of the major contributors to recent sea level rise (Gardner et al., 2013; Mernild and Wilson, 2016). Direct glaciological observations in this remote region are still scarce, mainly due to the region's inaccessibility and its harsh climate (Buttstädt et al., 2009; Mernild et al., 2015; Schaefer et al., 2015). In recent decades, remote sensing techniques have enabled the spatial coverage of glaciological observations to be extended. They are particularly useful in remote and inaccessible areas like the NPI, making it possible to observe and measure glacier variations and to estimate mass balance rate at regional scales (Rignot et al., 2003; Rivera et al., 2007; Lopez et al., 2010; Davies and Glasser, 2012; Willis et al., 2012a,b; Dixon and Ambinakudige, 2015; Jaber et al., 2016). Among those studies, mass balance rate estimates are often based on the geodetic method, which allows calculation of glacier volume changes by differentiation of two or more multi-temporal digital elevation models (DEMs) (e.g., Paul et al., 2015; Marzeion et al., 2017). In particular for the NPI, Willis et al. (2012a) measured mass balance using the Shuttle Radar Topography Mission (SRTM) DEM and a collection of freely available Advanced Spaceborne Thermal Emission and Reflection Radiometer (ASTER) DEMs from 2001 to 2011. Jaber et al. (2016) also provided a mass balance estimate for 2000–2014 by comparing two interferometric DEMs, SRTM, and TanDEM-X.
The goal of our study is to produce two new and independent geodetic estimates of the NPI mass balance rate for the time period 2000–2012 using, on one hand, SRTM and SPOT5 and, on the other hand, ASTER DEMs. Although the NPI mass balance rate during this time period has already been estimated in two previous studies (Willis et al., 2012a; Jaber et al., 2016) our contribution is justified by:
(i) The use of ASTER DEMs calculated using the Ames Stereo Pipeline (ASP) (Shean et al., 2016). As shown in the supplement of our study, these DEMs have less artifacts than the 14DMO DEM available from LP-DAAC and used in many earlier studies including the one of Willis et al. (2012a) over the NPI.
(ii) The need to verify the lack of penetration of the February 2000 SRTM radar signal. Until now the effect of radar wave penetration into snow and ice has been assumed to be negligible in Patagonia due to the likely wet condition at the glacier surface in summer, favoring surface scattering of the signal (Willis et al., 2012a; Jaber et al., 2013, 2016). However, this assumption has not been verified yet.
(iii) The need to account for changes in ice-covered areas when calculating the glacier mass balance. None of the earlier studies considered these area changes, known to be large in Patagonia (Davies and Glasser, 2012).
The NPI is the second largest temperate ice mass in the southern hemisphere, after the Southern Patagonian Icefield. Located in Chile, it extends from 46°30′S to 47°30′S, stretching for almost 125 km north–south (Figure 1). It covers an area of ~3,740 km2 (measured in this study for year 2012) extending from sea level to altitudes higher than 4000 m a.s.l. at the summit of Mount San Valentin. It is composed of 38 glaciers larger than 0.5 km2. San Quintin is the largest glacier, covering 790 km2. San Rafael (714 km2) is the only tidewater calving glacier of the icefield and the lowest-latitude tidewater glacier as well as one of the fastest-flowing glaciers in the world (Rignot et al., 1996). The largest proportion of glaciers present fresh-water calving fronts (66% of the total area), whilst a smaller proportion (16%) correspond to land-terminating fronts (Rivera et al., 2007).
Figure 1. Location of the Northern Patagonian Icefield in Chile. (a) Elevation map of the NPI from the SRTM (void-filled version) on top of a cloud-free Landsat image acquired on 11 March 2001. Glacier outline corresponds to ice extents of year 2000. (b) 38 glaciers of the NPI are represented using the outline of year 2012 and ice divides from Mouginot and Rignot (2015) with the shaded relief from the SRTM void-filled DEM as background. Colors correspond to different glacier types: dark green is for the single tidewater calving glacier (San Rafael), light green for freshwater calving (i.e., lake-terminating) glaciers and purple for land-terminating glaciers as defined by Rivera et al. (2007).
The climate of the Northern Patagonian region is dominated by the southern hemisphere westerlies that bring moisture from the Pacific, and the equatorial Pacific sea surface temperature that regulates the El Niño Southern Oscillation (ENSO) and the Pacific Decadal Oscillation (Aravena and Luckman, 2009). This later two large scale modes of natural climate variability, together with the southern annular mode (SAM), are key modulators of temperature and precipitation in the southern Andes at inter-annual and inter-decadal timescales (Gillett et al., 2006; Garreaud et al., 2009). The north–south orientation and high altitude of the Andes chain at this latitude acts as a geographical barrier for the moisture, generating a föhn effect that creates a strong west-to-east gradient of precipitation, with considerably dryer conditions and higher temperatures on the eastern side of the icefield (Fujiyoshi et al., 1987). The average annual precipitation between 1975 and 2011 is 8.03 ± 0.37 m (Schaefer et al., 2013) with little seasonal variability. The 0°C isotherm altitude is at about 2,000 m a.s.l. during the summer, dropping to 900 m a.s.l. during winter (Barcaza et al., 2009).
The SRTM acquired elevation data between 11 and 22 February 2000 using an interferometric aperture radar sensor (SAR) (Farr et al., 2007). We used the SRTM DEM processed by NASA from the raw C-band radar signal at 1 arcsecond resolution (about 30 m). Due to radar layover or loss of coherence over vegetation and steep terrain, the SRTM DEM may contain data voids that are sometimes filled by interpolation or using an external DEM. For the purposes of DEM comparison, we use the non-void-filled version in which data voids cover 13% of the total NPI glaciated area. By specific elevation bands the percentage of data voids is 3% below 1,000 m a.s.l (total area 1,163 km2), 22% between 1,000 and 2,000 m a.s.l. (2,323 km2) and 13% above 2,000 m a.s.l. (370 km2). A second, void-filled SRTM DEM (version 4, 3 arcsec resolution) produced by the CGIAR-CSI (http://srtm.csi.cgiar.org) is also used to obtain a complete hypsometry of the icefield, necessary for the mass balance calculation.
The SPIRIT project produced DEMs over glaciated zones from stereo-pairs acquired by the SPOT5 high-resolution stereoscopic (HRS) sensor (Korona et al., 2009). A 40 m DEM, acquired on 18 March 2012, provides a complete coverage of the NPI (Supplementary Figure S1). The DEM is delivered with a reliability mask and one ortho-image generated from the rear HRS image. Reliability mask values range from 0 to 255, with 0 indicating the least reliable elevations that are thus masked out in the SPOT5 DEM. Data voids cover 16% of the total NPI glaciated area. By specific elevation bands the percentage of data voids is 7% below 1,000 m a.s.l, 22% between 1,000 and 2,000 m a.s.l. and 13% above 2,000 m a.s.l.
Raw stereo images obtained by the ASTER sensor on board the TERRA satellite were ordered free of charge from the ECHO/REVERB website (REVERB)1 from April 2000 to April 2012. After excluding all images with cloud cover fraction larger than 80%, a total of 118 ASTER Level 1A stereo images intersecting the NPI were selected. For the sake of concision, the list of ASTER images used in this study were not provided but is available upon request to the corresponding author.
Four late-summer nearly cloud-free LANDSAT L7 ETM+ orthoimages at 30 m spatial resolution acquired from the USGS Earth Explorer were used to improve glacier and water outlines for years 2000 and 2012 (8 March 2000 and 21 January, 22 February, 26 April 2012).
Initial outlines for glacier limits and water bodies over the NPI area were obtained from online datasets. The Global Land Ice Measurements from Space (GLIMS) database and the Randolph Glacier Inventory version 5.0 (RGI 5.0) offer access to glacier outlines from previous studies and inventories over the Southern Andes region (Rivera et al., 2007; Davies and Glasser, 2012; Pfeffer et al., 2014; Mouginot and Rignot, 2015). Following visual assessment, RGI5.0 was selected as the best existing outline, corresponding to the ice extent of year 2001 (RGI6.0 was not available at the time of our study). RGI5.0 was not used “as is” but was improved as explained in section Improvement of the Glacier Outlines. Water body outlines were acquired from the USGS Earth Explorer SRTM water body data (earthexplorer.usgs.gov), and the ice divides derived from velocity fields (Mouginot and Rignot, 2015) were used to split the icefield into individual glaciers.
The RGI 5.0 glacier outline was corrected manually using late-summer and cloud-free LANDSAT, ASTER, and SPOT5-HRS images as references to adjust the position of the ice for the years 2000 and 2012. RGI 5.0 ice divides were replaced by the ice divides from Mouginot and Rignot (2015). A visual comparison between our final outlines and best existing previous ones can be observed in Supplementary Figure S2. Significant errors and gaps were found in the SRTM water body data, so manual corrections were also performed. Furthermore, a cloud mask was drawn manually to exclude the cloudy pixels over the SPOT5-HRS DEM. No cloud mask was created for ASTER DEMs because the lack of correlation over the clouds leads directly to data voids in the DEMs.
As explained above, two different methods were applied to derive the map of the rate of elevation changes over the NPI: SRTM and SPOT5 DEM differencing (SPOT-SRTM hereafter) and linear trend analysis of multiple ASTER DEMs (ASTER_trend hereafter).
ASP is a NASA open source pipeline that allows for the mass production of DEMs out of high-resolution stereo images (Shean et al., 2016). We generated 118 ASTER-ASP DEMs with a grid spacing of 30 m from the raw ASTER L1A stereo images. ASP processing parameters are available upon request to the authors. Each ASTER DEM has a different footprint and, given the 60 km swath of ASTER, does not cover the entire NPI. The result is a spatially-varying number of ASTER DEMs available over the NPI (Supplementary Figure S1).
The DEMs used in this study are produced independently using different methods and without ground control points such that a horizontal shift is expected to exist between them. 2D coregistration (i.e., horizontal adjustment) should be performed on stable terrain and in regions where DEMs are most reliable (Paul et al., 2015). Stable terrain corresponds to all pixels with valid elevation values after excluding ice, snow, water bodies, and clouds.
The SRTM DEM was used as reference, and all other DEMs (SPOT5 and ASTER) were adjusted to it. For SPOT-SRTM, the horizontal shift is determined by an iterative minimization of the standard deviation of the elevation difference (dh) on stable terrain (Berthier et al., 2007). For ASTER_trend, an approach based on terrain slope and aspect was used to calculate the horizontal shift (Kääb, 2005; Nuth and Kääb, 2011). Both coregistration methods lead to similar results. The Nuth and Kääb method is preferred to coregister ASTER DEMs as it is numerically faster and thus more suitable for managing large amounts of data (Paul et al., 2015).
Three different types of vertical biases are quantified on stable terrain and corrected in SPOT5 and ASTER DEMs. We first correct for a simple vertical shift, then biases along and across the satellite tracks and finally we account for elevation difference due to different DEM resolution.
For SPOT5 and ASTER DEMs, the median of the altitude difference with the SRTM DEM over stable terrain is first substracted. To avoid anomalous values that are usually found over steep terrain, only pixels where the slope is <30° are considered to estimate this median vertical shift (Berthier et al., 2016). We recognize that this limit is somewhat arbitrary but further justified by the fact that over 90% of the NPI surface has a slope lower than 30°.
For SPOT5 and ASTER DEMs, all derived from stereo imagery, the satellite acquisition geometry and its particular trajectory may induce biases in the DEMs along and across the satellite track direction (Berthier et al., 2007; Scherler et al., 2008; Nuth and Kääb, 2011). We rotate the original coordinate system of the SPOT5 and ASTER DEMs tracks to match their specific trajectory (Nuth and Kääb, 2011). The elevation difference (dh) is then computed over stable terrain along and across the satellite track, and corrected using a fifth-order polynomial fit.
We applied a curvature-dependent correction to the SPOT5 and ASTER DEMs. This correction is calculated on the stable terrain by fitting a fifth-order polynomial to the elevation difference with the SRTM DEM plotted as a function of terrain maximum curvature (Gardelle et al., 2012).
After all adjustments, pixels from the SPOT5 and ASTER DEMs which have an absolute elevation difference with SRTM greater than 150 m are considered as outliers and excluded from all subsequent analysis.
The maps of elevation change rates (dh/dt) are obtained differently for the two methods. For SPOT-SRTM, a simple DEM difference is computed and divided by their time separation (12.1 yr) to calculate dh/dtSPOT−SRTM. In the case of ASTER_trend, for each pixel, a first linear trend is fit through all the available elevation values (from all ASTER DEMs and the SRTM DEM) during 2000–2012. The final dh/dtASTER_trend is calculated from a second fit considering only the ASTER DEMs within the 95% confidence interval of the first regression line and excluding the SRTM DEM to avoid possible bias due to signal penetration. For the majority of the NPI area, the first valid elevation measurements used in this second linear fit come from a DEM acquired in April 2000, and the last measurements from two DEMs acquired in March 2012 and April 2012 (Supplementary Figure S3). We did not observe any obvious temporal concentration of ASTER DEMs. Thus, the time-stamp of the dh/dt maps from the two methods is similar, i.e., 2000–2012.
In the conversion from dh/dt to mass balance, only reliable pixels from glaciated terrain are considered. For ASTER_trend, we further exclude all pixels for which the uncertainty of the second linear fit is larger than 3 m yr−1 (at the 95% confidence level).
NPI individual glaciers have shown different volume change rates during recent years, with differential responses within glaciers and a strong contrast between the eastern and western margins (Rivera et al., 2007; Willis et al., 2012a). To take into account these local effects, separate calculations were made for each glacier, and then the overall volume change rate and mass balance rate of the NPI were calculated as the area-weighted sum of every glacier. Each individual glacier is divided into 50 m altitude bands using the void-filled version of the SRTM DEM. For each altitude band, we compute the mean dh/dt after excluding all values lying outside of ±3*NMAD (normalized median absolute deviation).This is an efficient way to exclude outliers (Berthier et al., 2004; Gardelle et al., 2013). The band average dh/dt is then weighted by its area to calculate the glacier-wide average dh/dt. In the rare cases where no measurement is available for an altitude band, the rate of elevation change is assumed to be 0.
Following Fischer et al. (2015), we considered the varying areas of each individual glacier between 2000 and 2012 to convert volume change rate to mass balance rate. We use a value of 850 ± 60 kg m−3 as the volume-to-mass conversion factor, which is reasonable regarding the duration of our study (>5 years) (Huss, 2013).
The ASTER_trend method allows rough estimation of the SRTM penetration depth (Wang and Kääb, 2015; Berthier et al., 2016). By extrapolating dh/dt to the date of acquisition of the SRTM DEM, a reconstructed SRTM DEM (called hereafter SRTMrec) can be obtained. The differencing of SRTMrec-SRTM maps the penetration depth of the C-band radar signal.
Formal uncertainties for mass balance estimates were calculated taking into account six main sources of error: errors on dh/dt, errors in glacier area, error on the density conversion factor, errors due to data voids, errors due to seasonal cycle sampling irregularities and departure from a linear trend.
The uncertainty on the elevation change of a pixel is mainly given by the uncertainty inherent in the original DEMs, including the random and systematic errors produced during their coregistration. To assess this error we split the stable terrain dh/dt maps into 3 × 3 tiles as in Berthier et al. (2016). For each tile the median of dh/dt on stable ground is computed to visualize the spatial pattern of biases in dh/dtASTER and dh/dtSPOT−SRTMmaps (Figure 2). We then compute the error in dh/dt as the mean of the absolute difference of the median dh/dt for these 9 tiles (Figure 2). We obtained an error of 0.07 m yr−1 for SPOT-SRTM and 0.06 m yr−1 for ASTER_trend.
Figure 2. Rate of elevation change over stable terrain in the NPI region during 2000–2012, calculated by the SPOT-SRTM (left) and ASTER_trend (right) methods. The study region is divided into 3 × 3 tiles. The values correspond to the median dh/dt on stable ground in each tile. For comparison, the color scale is the same as for the maps of dh/dt over glaciers in Figure 4.
Glacier outlines were improved manually and contain an error that is inherent to the individual that performed the work. The error in glacier area was assessed by drawing buffers around the glacier outline (Granshaw and Fountain, 2006). A one-pixel buffer is a reasonable error level for glacier outlines produced manually, except for debris-covered glaciers (Paul et al., 2015). As the NPI debris coverage is limited, a one-pixel buffer was applied to the NPI outline, leading to an error of about 5% of the measured area. This value is consistent with Paul et al. (2015) and Pfeffer et al. (2014).
For density the error of ±60 kg m−3 is obtained from Huss (2013).
A conservative multiplicative factor of 5 is used for the uncertainties in the unsurveyed areas (Berthier et al., 2014).
Systematic errors in the dh/dt from ASTER_trend could come from an irregular sampling of the seasonal elevation change cycle as the ASTER DEMs were acquired at different times of the year. For example, a large systematic error (i.e., too negative mass balance) could occur if ASTER DEMs were acquired mostly in winter at the beginning of the study period and mostly in summer toward the end of the study period. To quantify the magnitude of this potential error, we sample a hypothetical seasonal cycle at the time of acquisition of the ASTER DEMs. The amplitude of the seasonal cycle is poorly known for Patagonia and must be spatially variable, so we prefer to use a normalized value of 1 m, with a minimum at the end of April and a maximum at the end of October. As shown in Figure 3, the ASTER temporal sampling is sufficiently random that the regression slope is only −0.0013 m yr−1. Even if the seasonal elevation change cycle was as large as 10 m (a high value but not unrealistic for the maritime NPI), the resulting systematic error would be 0.013 m yr−1, well below other sources of uncertainties. This source of error is thus neglected.
Figure 3. Temporal sampling of the 118 ASTER DEMs used in the ASTER_trend method. The amplitude of the seasonal cycle is normalized to 1 m. Dots correspond to theoretical elevations at the time of acquisition of the ASTER DEM; the red line shows the linear fit to the elevation time series, with a slope of −0.0013 m yr−1.
The assumption of linearity is one of the weaknesses of our ASTER-based method, as in principle glacier elevation change do not follow a linear behavior. To detect potential changes of the ASTER trends (and mass balances) over time, we divided 2000–2012 into two sub-periods: 2000 to March 2007 (2007.3) and 2007 to May 2012 (2012.5). We did not split the 12-year study period in two sub-periods of equal duration because there was no DEM for year 2006. Conversely, several useful ASTER DEMs were acquired in 2007 (late summer, no clouds, and good spatial coverage of the NPI), thus we decided to cut the period at 2007 and include summer 2007 DEMs in both trends. The NPI-wide mass balance from 2000 to 2007.3 was −1.06 ± 0.20 m w.e. yr−1, and −0.99 ± 0.20 m w.e. yr−1 from 2007 to 2012.5. Given error bars, mass balance rates for both sub-periods are not different and agree with the estimate for the whole period 2000–2012 (−1.06 ± 0.15 m w.e. yr−1). This result justifies the assumption of linearity and demonstrates that, within the error bars of our method, we are not able to detect a temporal change in the NPI mass balance.
We then consider all the significant sources of error to calculate our formal uncertainties on mass balance. First, the uncertainty in glacier rate of volume change , assuming that the uncertainty on area is independent of the uncertainty on rate of elevation change, was calculated as:
where is the mean rate of elevation change, , and g is the proportion of data gaps from the total area.
Assuming that the uncertainty in rate of volume change is independent of the uncertainty of the density conversion factor, the uncertainty on geodetic mass balance rate (σṀ), was calculated as:
where is the density conversion factor and is the uncertainty on the density conversion factor (Huss, 2013).
The NPI had a total glacierized area of 3,856 ± 211 km2 in 2000, and 3,740 ± 200 km2 in 2012. The total area loss for the period 2000–2012 is 116 km2 (3%), at a rate of −9.7 km2 yr−1.
Areal changes are heterogeneous and do not follow any obvious spatial pattern. Largest areal changes are observed on glaciers U4 (19%) located in the south-east, and glaciers Circo (12%) and Grosse (10%) located in the north of the NPI. The largest frontal retreats were observed in the tongues of two glaciers in the south-west, Steffens (3.3 km frontal retreat) and HPN1 (2.5 km retreat over its main tongue and even 3.1 km retreat over one of its tributaries), and San Quintin in the north-west (2.5 km frontal retreat). Glaciers Gualas, Grosse and Reicher in the north and glacier Acodado in the south also showed significant frontal retreats of the order of 1 and 2 km between 2000 and 2012.
Both methods (SPOT-SRTM and ASTER_trend) resulted in a similar spatial distribution of dh/dt over the NPI (Figure 4), with maximum thinning rates localized at low elevations over the glacier tongues. Icefield-wide mass balances rates during 2000–2012 are also in excellent agreement: −1.02 ± 0.21 m w.e. yr−1 for SPOT-SRTM and −1.06 ± 0.15 m w.e. yr−1 for ASTER_trend (Table 1).
Figure 4. Rate of elevation change (m yr−1) over the NPI for the time period 2000–2012. (A) Rate of elevation change from SPOT-SRTM; white blank corresponds to data gaps. (B) Rate of elevation change from ASTER_trend; white blank corresponds to data gaps. (C) Distribution of the rate of elevation changes as function of altitude; red dots represent SPOT-SRTM and orange dots represent ASTER_trend. The histograms show the hypsometry of the NPI by 100 m elevation bands (gray bars), and the corresponding measured area from SPOT-SRTM (blue bars) and ASTER_trend (purple bars). (Note that hypsometry is shown here every 100 m bands. This is to help visualization although the entire processing was made using 50 m bands).
Table 1. NPI-wide volume change rate, thinning rate, and mass balance rate estimates for the 2000–2012 time period for the two methods.
Strongest thinning rates are observed over the outlet glaciers of the western margins of the icefield, with dh/dt rates as negative as −10 m yr−1 for the terminating tongues of Frankel, Benito, HPN1 and Acodado glaciers. The largest differences between the two dh/dt maps are observed over San Rafael glacier, where thinning rates are more negative for SPOT-SRTM, and over the north-east margins. Looking closely at the dh/dt vs. altitude curves for the two methods, a strong thickening is observed above 2,900 m a.s.l. for SPOT-SRTM, whereas a slight thinning is measured by ASTER_trend (Figure 4C). The anomalously positive values in SPOT-SRTM dh/dt curves at high altitude will be discussed in section Penetration of SRTM Signal Over NPI.
Spatial coverage of valid dh/dt rates is different for the two methods, with 68% of the total NPI area covered in SPOT-SRTM, and a better coverage of 82% in ASTER_trend. Almost complete coverage was achieved below 1,000 m a.s.l. in both cases. Higher concentrations of data gaps exist in the accumulation zone (>1,000 m a.s.l.) with 35% gaps for SPOT-SRTM vs. 22% for ASTER_trend. Both the SPOT5 reliability mask and the ASTER error grid produced during the linear fit suggest larger errors in this region. The lack of image texture over the flat and homogeneous snow surface makes correlation of the stereo images either impossible or erroneous. Higher altitudes are better represented in ASTER_trend, with only 10% of data gaps above 2,000 m a.s.l. vs. 49% for SPOT-SRTM; the latter is mainly due to voids in the SRTM DEM.
The coverage of individual glaciers with valid dh/dt measurements is much better for ASTER_trend, where all glaciers show two-thirds (66%) of their total area with valid data. In the case of SPOT-SRTM, coverage is not as good. Fourteen out of thirty-eight glaciers, corresponding to 77% of the total NPI area, show between one- and two-thirds of their area with valid measurements, while 11 glaciers (6% of the NPI area) show less than one-third (Figure 5A).
Figure 5. Mass balance rate of NPI individual glaciers from 2000 to 2012. (A) Mass balance rate obtained from method SPOT-SRTM. Hatched glaciers have <33% data coverage whereas pointed glaciers have between 33 and 66% coverage. (B) Mass balance rate obtained from method ASTER_trend. All glaciers present more than 66% of their area with valid dh/dt values.
Individual glacier mass balance rates (Ṁ) for the period 2000–2012 are shown in Figure 5 and Supplementary Table S1 for both methods. All glaciers show negative ṀASTER_trend. Contrarily, ṀSPOT−SRTM for glaciers Grosse, Exploradores, Bayo, and Leones in the north-east part of the NPI and glacier Pissis in the south are slightly positive. However, these five glaciers were poorly sampled due to gaps in the SPOT5 DEM. Both methods agree on highly negative mass balances rates for south-west glaciers. The most negative Ṁ coincide in glacier HPN1 with values of −2.38 ± 0.32 and −2.30 ± 0.24 m w.e. yr−1 for ASTER_trend and SPOT-SRTM respectively. The Ṁ for the three largest glaciers of the icefield, San Quintin, San Rafael and Steffens, accounting together for 51% of the total area, are strongly negative and in good agreement between methods.
For all glaciers larger than 100 km2, Ṁ from both methods agree within error bars, and their absolute difference is smaller than 0.3 m w.e. yr−1 (Figure 6). Conversely, smaller glaciers tend to show larger disagreement between methods, with Ṁ differences up to 1.6 m w.e. yr−1. The largest differences are observed for glaciers poorly sampled in SPOT-SRTM (green and orange dots).
Figure 6. Comparison of individual glacier mass balances from the two methods. (A) Mass balance rates obtained from method SPOT-SRTM as function of mass balance rates obtained from method SPOT-SRTM. The dashed line corresponds to the 1:1 line. (B) Absolute difference of Ṁ (m w.e. yr−1) between the two methods as a function of glacier area.
Our area estimate for 2000 is in good agreement with previous studies, especially with Jaber et al. (2016), suggesting that our error bars are conservative (Table 2). Davies and Glasser (2012) obtained a slightly larger value for the NPI area in 2001 because they considered some of the rock nunataks as part of the icefield area. Similarly, Rivera et al. (2007) included adjacent small icy areas as part of the icefield. To our knowledge, we provide the first estimate of NPI area for 2012.
Glacier areal changes are not homogeneous through the NPI. In general the largest frontal retreats were observed for glacier tongues situated in the south-west of the icefield (glaciers Steffens and HPN1), also in agreement with previous studies (Rivera et al., 2007; Lopez et al., 2010; Davies and Glasser, 2012; Willis et al., 2012a). No convincing relationship was observed between glacier-specific area loss and mass balance. This conclusion is in agreement with earlier findings for Alaskan glaciers (Arendt et al., 2002).
We accounted for these area changes in our mass balance rate estimates. At the scale of the entire icefield, the effect is minor as the NPI mass balance rate is changed by 0.01 m w.e. yr−1 if only the 2000 inventory is available. Yet, the mass balance rate can vary by up to 10% for the fastest retreating glaciers.
Some positive dh values were found above 2,900 m a.s.l. with the SPOT-SRTM method. These positive values are anomalous as, to our knowledge, no study documented thickening in the upper part of the NPI since 2000. Figure 7 shows the distribution with altitude of our estimate of the SRTM penetration depth (SRTMrec-SRTM), a side product of the ASTER_trend method. Positive values are observed above 2,900 m a.s.l. This is consistent with the apparent thickening observed above 2,900 m a.s.l. in the SPOT-SRTM dh/dt and thus suggest possible penetration of the SRTM C-band signal on the NPI snow located above 2,900 m a.s.l.
Figure 7. Penetration of the SRTM C-band radar signal as a function of altitude. Curves represent the total elevation difference from SPOT-SRTM (red curve) and SRTMrec-SRTM (green curve). Positive values in SRTMrec-SRTM represent penetration. The histogram shows the area/altitude distribution.
The SRTMrec-SRTM and the SPOT-SRTM curves in Figure 7 do not match perfectly over this high-altitude zone. Likewise, the SRTMrec-SRTM curve shows positive values between 1,000 and 2,900 m a.s.l. However, these differences remain within the uncertainties of the SRTM penetration depth, assumed to be roughly ±3.1 m in Berthier et al. (2016), and cannot be further interpreted. We further confirmed that this ±3.1 m is conservative by extrapolating dh/dtASTER_trend to reconstruct two DEMs at the dates of acquisition of the SPOT5 2012 DEM and of an additional SPOT5 DEM acquired 18 May 2005 (not used in the study for measuring elevation changes but with similar specifications as the SPOT5 DEM 2012). Differencing between SPOT5rec_2012-SPOT52012 and SPOT5rec_2005-SPOT52005 yielded mean dh on the NPI of 1.03 m and 0.14 m, respectively, both values well below the 3.1 m assumed error.
If we consider the region above 2,900 m a.s.l., the mean penetration depth is 10 m, a value similar to the 10 m C-band penetration depth found on the dry and cold firn of Greenland and Alaska (Rignot et al., 2001). Averaged over the whole icefield, the mean penetration depth is negligible (0.02 m), in agreement with a recent study in New Zealand (also in the southern hemisphere), where no penetration was found (Wang and Kääb, 2015).
Up to now, SRTM penetration depth has been considered negligible in Patagonia due to almost constant wet conditions at the glacier surface in February 2000 (Jaber et al., 2013). Here we show that penetration is actually occurring at the highest elevations, where temperatures are lower and firn is probably dry even in summer. Nevertheless, only 0.75% of the NPI area (29 km2) is found above 2,900 m a.s.l., a fraction small enough not to affect the overall mass balance rate of the icefield (the corresponding bias is <0.01 m w.e. yr−1). Therefore, SRTM penetration can be assumed negligible over the NPI. Still, future geodetic mass balance studies in Patagonia using SRTM C-band DEM, should carefully consider the penetration depth, especially when a significant fraction of the glacier lies at high elevations.
The lack of SRTM penetration, except at the highest elevations, is in line with a backscattering analysis using the SRTM swath image data (SRTM-IMGR) in Jaber et al. (2016). They found low backscattering values over the plateau, indicating the presence of wet snow and consequent insignificant penetration depth. Less negative backscattering values are also observed over the highest zones, suggesting dryer snow where the C-band signal can penetrate.
Both methods led to similar estimates of the NPI mass balance rate for the 2000–2012 period (Table 3).
Table 3. Global volume change rates estimates for the NPI from this and previous studies during similar time periods.
The SPOT-SRTM method has already been used in the southern Andes (Falaschi et al., 2016) but this method is all the more relevant now that we have verified the negligible penetration of the SRTM C-band signal at this latitude.
Even though individual ASTER DEMs have a higher noise level than the SPOT5 DEM when subtracted from the SRTM DEM (mean standard deviation of the residual for ASTER DEMs: 14 vs. 8 m for SPOT5 DEMs), their larger number led to final errors on stable ground very similar to those obtained with the SPOT-STRM method.
Icefield-wide mass balance error differs slightly between methods (±0.21 m w.e. yr−1 for SPOT-SRTM and ±0.15 m w.e yr−1 for ASTER_trend). This difference is mainly related to the amount of data gaps, higher in SPOT-SRTM. The distribution of data gaps coincides over the NPI plateau (~1,200–1,700 m a.s.l.) for both methods. Yet, over some glaciers in the north-east margins of the NPI, specifically Grosse, Bayo, and Exploradores glaciers, less than one-third of their area is covered with the SPOT-SRTM method due to clouds in the SPOT5 images. Coverage is also restricted on small steep glaciers like glacier Pissis in the south, where a high percentage of data gaps exists over the SRTM DEM. These latter glaciers are those for which the mass balance rate differs most between the two methods. The redundancy of the acquisitions used in the ASTER_trend method overcomes this issue.
The 2000–2012 Ṁ are in agreement within error bars between the two methods for all glaciers with coverage larger than 66%. Specific cases where Ṁ do not agree within error bars correspond to glaciers poorly sampled in the SPOT-SRTM method due to clouds or SRTM data gaps. The three largest glaciers of the icefield (51% of the total area) present Ṁ differences smaller than 0.2 m w.e. yr−1.
Availability of the data is also important to consider when comparing the two methods. A strong advantage of the ASTER_trend method is that it is based on freely and nearly globally available ASTER L1A stereo images. SPOT5 stereo images were only acquired over specific areas and are not yet freely available. Thus, the ASTER_trend has the potential to be extended to wider areas.
Many studies already estimated the geodetic mass balance rates of the NPI for diverse time periods (Rignot et al., 2003; Aniya, 2007; Rivera et al., 2007; Glasser et al., 2011; Willis et al., 2012a; Jaber et al., 2016). However, only two of these studies examined the same time period as us and can therefore be directly compared with our results (Table 3). NPI-wide surface mass balances have also been estimated from atmospheric models (Schaefer et al., 2013; Mernild and Wilson, 2016), but these numbers cannot be compared with ours as they do not account for the mass loss by calving which is an important component of the mass loss for the NPI.
Generally, good agreement is found between our two estimates and previous geodetic estimates (Table 3). Although they used ASTER stereo images like us, Willis et al. (2012a) found a smaller mass loss. The difference may result either from the use of different ASTER DEMs, different coregistration methods, the amount of DEMs considered to extract dh/dt (55 in Willis et al., 2012a, 118 in our study) or the slight difference between time periods. We generated our own ASTER DEM using ASP, and they exhibit less artifacts than the DEMs from the 14DMO product used in Willis et al. (2012a) (Supplementary Figure S4).
Our mass loss estimates agree with Jaber et al. (2016) who compared the SRTM DEM with a TanDEM-X DEM mosaic. These two DEMs, derived from radar interferometry, are not affected by clouds and provide good coverage of the accumulation zone. Nevertheless, their coverage of the icefield was not complete, as northernmost NPI glaciers were not covered by TanDEM-X DEMs.
Error estimates in Jaber et al. (2016) and Willis et al. (2012a) are three to four times smaller than ours. One reason for our larger errors could be the factor of five that we conservatively allocated to errors over data gaps. However, mass balance differences of typically 0.2 m w.e. yr−1 between our two methods for well-sampled glaciers (Figure 6, Supplementary Table S1) suggest that our error bars are reasonable and not too conservative.
We compared two geodetic methods to obtain the region-wide and glacier-wide mass balance rates of the NPI. The first is based on the standard differencing of two DEMs (SRTM and SPOT5) whereas the second method extracts the rate of surface elevation change by fitting a linear trend to over 118 DEMs derived from ASTER stereo images.
For the entire NPI (about 3,800 km2), excellent agreement is found between the two methods. Following previous studies, our analysis confirms the strong mass loss, with an icefield-wide mass balance rate of −1.06 ± 0.15 m w.e. yr−1 (ASTER_trend). The thinning rate reaches up to 10 m yr−1 for some low-elevation glacier tongues. Importantly, we also verify the lack of significant penetration of the SRTM C-band radar signal in the wet and temperate firn of the icefield except for the highest altitudes where dry snow was present in February 2000 (>2,900 m a.s.l.; <1% of the icefield).
For individual glaciers, larger differences exist between the two methods, especially when the data coverage is more limited. By definition the SPOT5-SRTM estimate does not cover the area where clouds are present in the SPOT5 stereo images. The ASTER_trend method is less prone to this effect because it relies on numerous (>100) ASTER DEMs. Both methods exhibit data gaps in the upper accumulation areas due to the lack of texture in the optical stereo images. This issue could be solved in the future by using images with higher spatial resolution and better radiometric depth.
Here we have provided insight into the effectiveness, advantages and disadvantages of two methods for estimating the geodetic mass balance rates of Patagonian glaciers and icefields. Both methods lead to coherent and satisfying results, though the free availability of the extensive archive of ASTER stereo images is a strong advantage.
ID: performed all the DEM analysis with inputs from EB and FB. ID: Led the writing of the paper and all other co-authors contributed to it.
The authors declare that the research was conducted in the absence of any commercial or financial relationships that could be construed as a potential conflict of interest.
ID acknowledges a Ph.D. fellowship from the France Space Agency (CNES) and the Région Occitanie. Etienne Berthier acknowledges support from the CNES and the Programme National de Télédétection Spatiale (PNTS, http://www.insu.cnrs.fr/pnts), grant PNTS-2016-01. ASTER images are courtesy of NASA/METI/AIST/Japan Space systems and U.S./Japan ASTER Science Team. SPOT5 images and DEMs were provided by the SPIRIT project (Korona et al., 2009), funded by CNES. This work could not have been performed without the GLIMS project that allowed populating a vast archive of freely available ASTER stereo images over glaciers. We also acknowledge the Ames Stereo Pipeline developer teams for their help and Vincent Favier for his comments on an earlier version of this manuscript. We are grateful to Ken Moxham for reviewing the English. We thank the editor, Matthias Braun, and two reviewers for their very useful comments on our manuscript.
The Supplementary Material for this article can be found online at: https://www.frontiersin.org/articles/10.3389/feart.2018.00008/full#supplementary-material
Supplementary Figure S1. Spatial footprint of the SPOT5-HRS DEM for year 2012 (red line). The color code corresponds to the number of ASTER elevation measurements available for every pixel from year 2000 to 2012 (after excluding elevation values outside the following accepted elevation range: within 150 m from the median of all DEMs on ice, and within 100 m off ice).
Supplementary Figure S2. Comparison of three NPI glacier inventories. (a) The Blue line corresponds to the inventory created and used in this study for year 2000 (see section Glacier Outlines, Water Bodies, and Ice Divides), ice divides are obtained from Mouginot and Rignot (2015). The red and green lines correspond to the RGI 5.0 and Rivera et al. (2007) inventories for year 2001 (recently incorporated in RGI 6.0), respectively. Named glaciers are those showing the largest area differences between inventories. (b) Zoom over the Cachet glacier area where inventories largely disagree on ice divides. Landsat Image for the 8 of mars 2000 as background. (c) Table shows the differences in glacier area between the inventories from Rivera et al. (2007) and the RGI 5.0 compared to the one used in this study, for the 7 glaciers that showed the largest differences. Note that the three largest glaciers of the NPI, San Quintin, San Rafael and Steffens, covering together 51% of the NPI area are included in this table.
Supplementary Figure S3. Spatial distribution of the minimum (a) and maximum (b) dates of ASTER DEMs used in our study. White gaps correspond to water bodies and locations where the uncertainty of dh/dtASTER_trend is larger than 3 m yr−1 (at the 95% confidence level).
Supplementary Figure S4. Comparison of SRTM, ASTER-14DMO and ASTER-ASP DEMs along a transverse profile of San Quintin glacier. (a) Location of the profile including glacier and stable terrain; transitions are presented as blue lines. (b) Elevation profiles extracted from the SRTM (February 2000) ASTER-14DMO and ASTER-ASP (18 March 2012) DEMs. Both ASTER DEMs are derived from the same Level 1A stereo pair. The ASTER-14DMO DEM (used in Willis et al., 2012a) was downloaded from LPDAAC. This profile illustrates the occurrence of numerous artifacts over the glacier surface in the ASTER-14DMO DEM. Conversely, the ASTER-ASP DEM is smoother and follows nicely the undulations of the SRTM DEM with an offset due to glacier thinning between 2000 and 2012. Hence, ASTER-ASP DEMs are more precise and should be preferred to ASTER-DMO DEMs to study glacier elevation changes.
Supplementary Table S1. Mass balance of NPI individual glaciers from 2000 to 2012 and percentage area measured for SPOT-SRTM and ASTER_trend methods.
1. ^REVERB. Available online at: https://reverb.echo.nasa.gov/#utf8=%E2%9C%93&spatial_map=satellite&spatial_type=rectangle
Aniya, M. (2007). Glacier variations of Hielo patagónico Norte, Chile, for 1944/45-2004/05. Bull. Glaciol. Res. 24, 59–70.
Aravena, J.-C., and Luckman, B. H. (2009). Spatio-temporal rainfall patterns in Southern South America. Int. J. Climatol. 29, 2106–2120. doi: 10.1002/joc.1761
Arendt, A. A., Echelmeyer, K. A., Harrison, W. D., Lingle, C. S., and Valentine, V. B. (2002). Rapid wastage of Alaska glaciers and Their contribution to rising sea level. Science 297, 382–386. doi: 10.1126/science.1072497
Barcaza, G., Aniya, M., Matsumoto, T., and Aoki, T. (2009). Satellite-derived equilibrium lines in Northern Patagonia icefield, Chile, and their implications to glacier variations. Arct. Antarct. Alp. Res. 41, 174–182. doi: 10.1657/1938-4246-41.2.174
Berthier, E., Arnaud, Y., Baratoux, D., Vincent, C., and Rémy, F. (2004). Recent rapid thinning of the “Mer de Glace” glacier derived from satellite optical images. Geophys. Res. Lett. 31:L17401. doi: 10.1029/2004GL020706
Berthier, E., Arnaud, Y., Kumar, R., Ahmad, S., Wagnon, P., and Chevallier, P. (2007). Remote sensing estimates of glacier mass balances in the Himachal Pradesh (Western Himalaya, India). Remote Sens. Environ. 108, 327–338. doi: 10.1016/j.rse.2006.11.017
Berthier, E., Cabot, V., Vincent, C., and Six, D. (2016). Decadal region-wide and glacier-wide mass balances derived from multi-temporal ASTER satellite digital elevation models. Validation over the Mont-Blanc area. Cryospheric Sci. 4:63. doi: 10.3389/feart.2016.00063
Berthier, E., Vincent, C., Magnússon, E., Gunnlaugsson, Á. Þ*., Pitte, P., Le Meur, E., et al. (2014). Glacier topography and elevation changes derived from pléiades sub-meter stereo images. Cryosphere 8, 2275–2291. doi: 10.5194/tc-8-2275-2014
Buttstädt, M., Möller, M., Iturraspe, R., and Schneider, C. (2009). Mass balance evolution of martial Este Glacier, Tierra del Fuego (Argentina) for the period 1960–2099. Adv. Geosci. 22, 117–124. doi: 10.5194/adgeo-22-117-2009
Davies, B. J., and Glasser, N. F. (2012). Accelerating shrinkage of Patagonian glaciers from the little ice Age (~AD 1870) to 2011. J. Glaciol. 58, 1063–1084. doi: 10.3189/2012JoG12J026
Dixon, L., and Ambinakudige, S. (2015). Remote sensing study of glacial change in the Northern Patagonian icefield. Adv. Remote Sens. 4, 270–279. doi: 10.4236/ars.2015.44022
Falaschi, D., Bolch, T., Rastner, P., Lenzano, M. G., Lenzano, L., Vecchio, A. L., et al. (2016). Mass changes of alpine glaciers at the eastern margin of the Northern and Southern Patagonian icefields between 2000 and 2012. J. Glaciol. 63, 258–272. doi: 10.1017/jog.2016.136
Farr, T. G., Rosen, P. A., Caro, E., Crippen, R., Duren, R., Hensley, S., et al. (2007). The shuttle radar topography mission. Rev. Geophys. 45:RG2004. doi: 10.1029/2005RG000183
Fischer, M., Huss, M., and Hoelzle, M. (2015). Surface elevation and mass changes of all Swiss glaciers 1980–2010. Cryosphere 9, 525–540. doi: 10.5194/tc-9-525-2015
Fujiyoshi, Y., Kondo, H., Inoue, J., and Yamada, T. (1987). Characteristics of precipitation and vertical structure of air temperature in the northern Patagonia. Bull. Glacier Res. 4, 15–23.
Gardelle, J., Berthier, E., and Arnaud, Y. (2012). Impact of resolution and radar penetration on glacier elevation changes computed from DEM differencing. J. Glaciol. 58, 419–422. doi: 10.3189/2012JoG11J175
Gardelle, J., Berthier, E., Arnaud, Y., and Kääb, A. (2013). Region-wide glacier mass balances over the Pamir-Karakoram-Himalaya during 1999–2011. Cryosphere 7, 1263–1286. doi: 10.5194/tc-7-1263-2013
Gardner, A. S., Moholdt, G., Cogley, J. G., Wouters, B., Arendt, A. A., Wahr, J., et al. (2013). A reconciled estimate of glacier contributions to sea level rise: 2003 to 2009. Science 340, 852–857. doi: 10.1126/science.1234532
Garreaud, R. D., Vuille, M., Compagnucci, R., and Marengo, J. (2009). Present-day South American climate. Palaeogeogr. Palaeoclimatol. Palaeoecol. 281, 180–195. doi: 10.1016/j.palaeo.2007.10.032
Gillett, N. P., Kell, T. D., and Jones, P. D. (2006). Regional climate impacts of the Southern annular mode. Geophys. Res. Lett. 33:L23704. doi: 10.1029/2006GL027721
Glasser, N. F., Harrison, S., Jansson, K. N., Anderson, K., and Cowley, A. (2011). Global sea-level contribution from the Patagonian icefields since the little ice age maximum. Nat. Geosci. 4, 303–307. doi: 10.1038/ngeo1122
Granshaw, F. D., and Fountain, A. G. (2006). Glacier change (1958–1998) in the north cascades national park complex, Washington, USA. J. Glaciol. 52, 251–256. doi: 10.3189/172756506781828782
Huss, M. (2013). Density assumptions for converting geodetic glacier volume change to mass change. Cryosphere 7, 877–887. doi: 10.5194/tc-7-877-2013
Jaber, W. A., Floricioiu, D., and Rott, H. (2016). “Geodetic mass balance of the Patagonian icefields derived from SRTM and TanDEM-X data,” in 2016 IEEE International Geoscience and Remote Sensing Symposium (IGARSS) (Bijing), 342–345.
Jaber, W. A., Floricioiu, D., Rott, H., and Eineder, M. (2013). “Surface elevation changes of glaciers derived from SRTM and TanDEM-X DEM differences,” in 2013 IEEE International Geoscience and Remote Sensing Symposium - IGARSS (Melbourne), 1893–1896.
Kääb, A. (2005). Combination of SRTM3 and repeat ASTER data for deriving alpine glacier flow velocities in the Bhutan Himalaya. Remote Sens. Environ. 94, 463–474. doi: 10.1016/j.rse.2004.11.003
Korona, J., Berthier, E., Bernard, M., Rémy, F., and Thouvenot, E. (2009). SPIRIT. SPOT 5 stereoscopic survey of polar ice: reference images and topographies during the fourth international polar year (2007–2009). ISPRS J. Photogramm. Remote Sens. 64, 204–212. doi: 10.1016/j.isprsjprs.2008.10.005
Lopez, P., Chevallier, P., Favier, V., Pouyaud, B., Ordenes, F., and Oerlemans, J. (2010). A regional view of fluctuations in glacier length in southern South America. Glob. Planet. Change 71, 85–108. doi: 10.1016/j.gloplacha.2009.12.009
Marzeion, B., Champollion, N., Haeberli, W., Langley, K., Leclercq, P., and Paul, F. (2017). Observation-based estimates of global glacier mass change and its contribution to sea-level change. Surv. Geophys. 38, 105–130. doi: 10.1007/s10712-016-9394-y
Mernild, S. H., Beckerman, A. P., Yde, J. C., Hanna, E., Malmros, J. K., Wilson, R., et al. (2015). Mass loss and imbalance of glaciers along the Andes Cordillera to the sub-Antarctic islands. Glob. Planet. Change 133, 109–119. doi: 10.1016/j.gloplacha.2015.08.009
Mernild, S., and Wilson, R. (2016). The Andes Cordillera. Part III: glacier surface mass balance and contribution to sea level rise (1979–2014). Int. J. Climatol. 37, 3154–3174. doi: 10.1002/joc.4907
Mouginot, J., and Rignot, E. (2015). Ice motion of the Patagonian icefields of South America: 1984–2014. Geophys. Res. Lett. 42, 1441–1449. doi: 10.1002/2014GL062661
Nuth, C., and Kääb, A. (2011). Co-registration and bias corrections of satellite elevation data sets for quantifying glacier thickness change. Cryosphere 5, 271–290. doi: 10.5194/tc-5-271-2011
Paul, F., Bolch, T., Kääb, A., Nagler, T., Nuth, C., Scharrer, K., et al. (2015). The glaciers climate change initiative: methods for creating glacier area, elevation change and velocity products. Remote Sens. Environ. 162, 408–426. doi: 10.1016/j.rse.2013.07.043
Pfeffer, W. T., Arendt, A. A., Bliss, A., Bolch, T., Cogley, J. G., Gardner, A. S., et al. (2014). The Randolph glacier inventory: a globally complete inventory of glaciers. J. Glaciol. 60, 537–552. doi: 10.3189/2014JoG13J176
Rignot, E., Echelmeyer, K., and Krabill, W. (2001). Penetration depth of interferometric synthetic-aperture radar signal in snow and ice. Geophys. Res. Lett. 28, 3501–3504. doi: 10.1029/2000GL012484
Rignot, E., Forster, R., and Isacks, B. (1996). Mapping of glacial motion and surface topography of Hielo Patagónico Norte, Chile, using satellite SAR L-band interferometry data. Ann. Glaciol. 23, 209–216. doi: 10.1017/S026030550001346X
Rignot, E., Rivera, A., and Casassa, G. (2003). Contribution of the Patagonia icefields of South America to sea level rise. Science 302, 434–437. doi: 10.1126/science.1087393
Rivera, A., Benham, T., Casassa, G., Bamber, J., and Dowdeswell, J. A. (2007). Ice elevation and areal changes of glaciers from the Northern Patagonia icefield, Chile. Glob. Planet. Change 59, 126–137. doi: 10.1016/j.gloplacha.2006.11.037
Schaefer, M., MacHguth, H., Falvey, M., and Casassa, G. (2013). Modeling past and future surface mass balance of the Northern Patagonia icefield. J. Geophys. Res. Earth Surf. 118, 571–588. doi: 10.1002/jgrf.20038
Schaefer, M., MacHguth, H., Falvey, M., Casassa, G., and Rignot, E. (2015). Quantifying mass balance processes on the Southern Patagonia icefield. Cryosphere 9, 25–35. doi: 10.5194/tc-9-25-2015
Scherler, D., Leprince, S., and Strecker, M. R. (2008). Glacier-surface velocities in alpine terrain from optical satellite imagery—accuracy improvement and quality assessment. Remote Sens. Environ. 112, 3806–3819. doi: 10.1016/j.rse.2008.05.018
Shean, D. E., Alexandrov, O., Moratto, Z. M., Smith, B. E., Joughin, I. R., Porter, C., et al. (2016). An automated, open-source pipeline for mass production of digital elevation models (DEMs) from very-high-resolution commercial stereo satellite imagery. ISPRS J. Photogramm. Remote Sens. 116, 101–117. doi: 10.1016/j.isprsjprs.2016.03.012
Wang, D., and Kääb, A. (2015). Modeling glacier elevation change from DEM time series. Remote Sens. 7, 10117–10142. doi: 10.3390/rs70810117
Willis, M. J., Melkonian, A. K., Pritchard, M. E., and Ramage, J. M. (2012a). Ice loss rates at the Northern Patagonian icefield derived using a decade of satellite remote sensing. Remote Sens. Environ. 117, 184–198. doi: 10.1016/j.rse.2011.09.017
Keywords: glacier mass balance, geodetic method, North Patagonian Icefield, radar penetration, Patagonia
Citation: Dussaillant I, Berthier E and Brun F (2018) Geodetic Mass Balance of the Northern Patagonian Icefield from 2000 to 2012 Using Two Independent Methods. Front. Earth Sci. 6:8. doi: 10.3389/feart.2018.00008
Received: 19 September 2017; Accepted: 22 January 2018;
Published: 12 February 2018.
Edited by:
Matthias Holger Braun, University of Erlangen-Nuremberg, GermanyReviewed by:
Marco Möller, University of Bremen, GermanyCopyright © 2018 Dussaillant, Berthier and Brun. This is an open-access article distributed under the terms of the Creative Commons Attribution License (CC BY). The use, distribution or reproduction in other forums is permitted, provided the original author(s) and the copyright owner are credited and that the original publication in this journal is cited, in accordance with accepted academic practice. No use, distribution or reproduction is permitted which does not comply with these terms.
*Correspondence: Inés Dussaillant, aW5lcy5kdXNzYWlsbGFudEBsZWdvcy5vYnMtbWlwLmZy
Disclaimer: All claims expressed in this article are solely those of the authors and do not necessarily represent those of their affiliated organizations, or those of the publisher, the editors and the reviewers. Any product that may be evaluated in this article or claim that may be made by its manufacturer is not guaranteed or endorsed by the publisher.
Research integrity at Frontiers
Learn more about the work of our research integrity team to safeguard the quality of each article we publish.