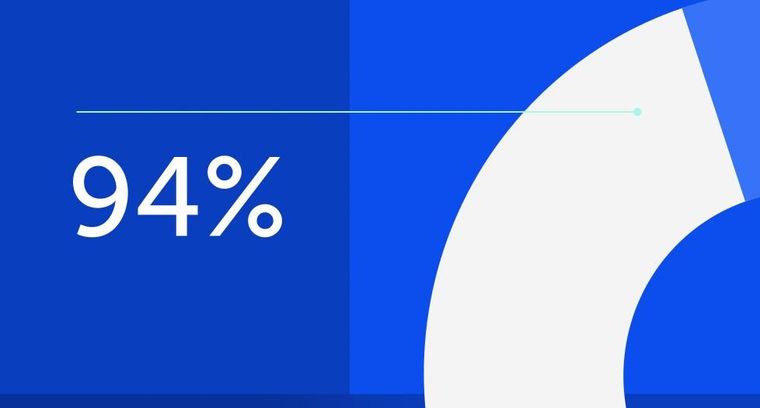
94% of researchers rate our articles as excellent or good
Learn more about the work of our research integrity team to safeguard the quality of each article we publish.
Find out more
METHODS article
Front. Drug Saf. Regul.
Sec. Advanced Methods in Pharmacovigilance and Pharmacoepidemiology
Volume 5 - 2025 | doi: 10.3389/fdsfr.2025.1579922
The final, formatted version of the article will be published soon.
Select one of your emails
You have multiple emails registered with Frontiers:
Notify me on publication
Please enter your email address:
If you already have an account, please login
You don't have a Frontiers account ? You can register here
Regulatory agencies aim to ensure the safety and efficacy of medical products but often face legal and privacy concerns that hinder collaboration at the data level. In this paper, we propose federated learning as an innovative method to enhance data-centric collaboration among regulatory agencies by enabling collaborative training of machine learning models without the need for direct data sharing, thereby preserving privacy and overcoming legal hurdles. We illustrate how Swissmedic, the Swiss Agency for Therapeutic Products, together with its partner agencies, proposes to use federated learning to improve TRICIA, an AI tool for assessing incoming reports of serious incidents related to medical devices. This approach enables the development of robust, generalizable risk assessment models that can potentially improve current processes. A proof of concept was deployed and thoroughly tested during the 14th Global Summit on Regulatory Science using synthetic data with participants from Swissmedic, the U.S. Food and Drug Administration (FDA), and the Danish Medicines Agency (DKMA), with promising initial results. This innovation has the potential to serve as a roadmap for other regulators to adopt similar approaches to optimize their own regulatory processes, contributing to a more integrated and efficient regulatory environment worldwide.
Keywords: Federated learning, Regulatory sciences, medical devices, Risk Assessment, Swissmedic
Received: 19 Feb 2025; Accepted: 11 Apr 2025.
Copyright: © 2025 Horst, Loustalot, Yoganathan, Li, Tong, Xu, Schneider, Di Renzo, Perez-Löffler and Renaudin. This is an open-access article distributed under the terms of the Creative Commons Attribution License (CC BY). The use, distribution or reproduction in other forums is permitted, provided the original author(s) or licensor are credited and that the original publication in this journal is cited, in accordance with accepted academic practice. No use, distribution or reproduction is permitted which does not comply with these terms.
* Correspondence: Alexander Horst, Swissmedic, Bern, Switzerland
Disclaimer: All claims expressed in this article are solely those of the authors and do not necessarily represent those of their affiliated organizations, or those of the publisher, the editors and the reviewers. Any product that may be evaluated in this article or claim that may be made by its manufacturer is not guaranteed or endorsed by the publisher.
Supplementary Material
Research integrity at Frontiers
Learn more about the work of our research integrity team to safeguard the quality of each article we publish.