- 1Inserm U1141, Hospital Robert Debré, University Paris Cité, Paris, France
- 2Akttyva Therapeutics, Inc., Boston, MA, United States
- 3Onco-Dermatology and Therapies, Inserm UMRS976, Hospital Saint Louis, France Institut de Recherche Saint Louis, University Paris Cité, Paris, France
- 4Department of Environmental Health Sciences, Johns Hopkins Bloomberg School of Public Health, Baltimore, MD, United States
Editorial on the Research Topic
Drug discovery and development explained: introductory notes for the general public
Finding new medications is a complex and costly process. Yet, as we work towards creating better, safer, and faster treatments, it is essential to make this process more understandable and accessible to everyone. This Research Topic is dedicated to introducing the main concepts and methods in a way that is accessible to all. With contributions from experts across the field, this Research Topic aims to demystify the drug development pipeline and address some of the its most pressing challenges (Figure 1).
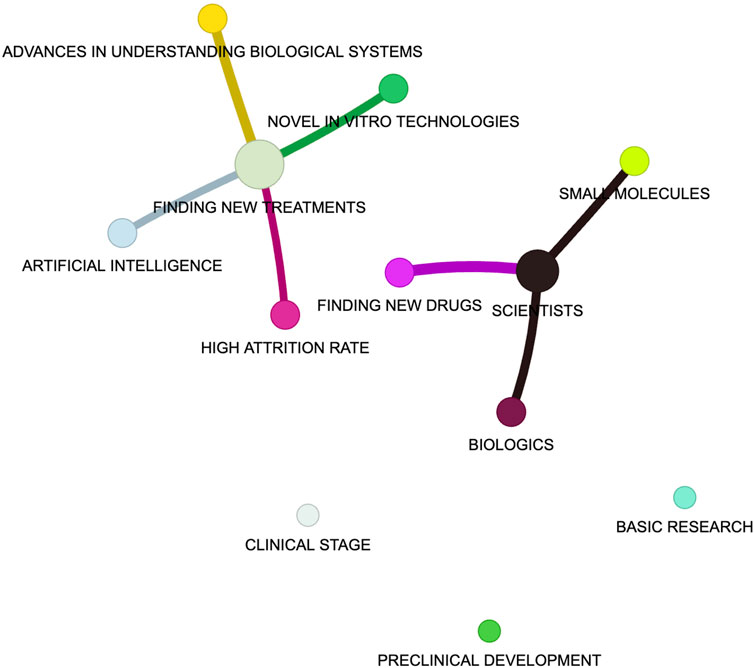
Figure 1. The graph illustrates a semantic network constructed automatically (i.e., without human-in-the-loop) using the LightRAG framework (https://arxiv.org/abs/2410.05779) and the abstracts of this Research Topic. LightRAG builds upon existing retrieval-augmented generation (RAG) methodologies by integrating graph-based retrieval in a more efficient, context-aware, and dynamic manner. It uses advanced language processing and a large language model (LLM, here we used Mistral-7B Instruct) to identify key concepts (nodes) and their relationships (edges) within a selected document. This process involves breaking down the text, embedding it into a high-dimensional space, and extracting meaningful entities and their interactions, resulting in a visual map that highlights the main themes and connections across the analyzed text. Such AI methods (and many others), used on scientific results and data, may assist the drug discovery process in a near future.
The article by Singh et al. provides a comprehensive overview of the entire drug discovery process, explaining the key steps from basic research to the final stages of clinical trials and post-market surveillance. By laying out the five main stages of drug discovery: pre-discovery, discovery, preclinical development, clinical trials, and approval, this article serves as a primer for readers unfamiliar with the field. The review also explains what are the main therapeutic agents (e.g., small molecules, peptides, biologics like antibodies…), the pros and cons of drug repurposing and highlights the high cost, long timelines and high attrition rates associated with drug discovery and development. The integration of artificial intelligence (AI) with traditional or novel experimental technologies, offers promising avenues to eventually streamline the process. Yet, many obstacles remain, including the lack of high quality data and the difficulty in understanding the disease state and human biology.
Chavez-Hernandez et al. explore the critical role of chemical and biological data in drug discovery. Their review underscores the importance of balancing the quantity and quality of data, especially as AI and machine learning methods become integral to the drug design process. The authors advocate for a better reporting of both active and inactive compounds to foster a more comprehensive understanding of bioactivity, emphasizing the need for balanced datasets that should drive more accurate predictions and hopefully lead to better treatments.
Gadiya et al. explain the concept of FAIR (Findable, Accessible, Interoperable, Reusable) data management. In an era where data is often siloed within institutions and companies, the lack of accessible datasets hinders progress. The authors first suggest that embracing FAIR principles across the drug discovery pipeline can enhance collaboration and thus improve the process. Then they further mention that the FAIR approach should help drug developers to learn from past efforts, thus reducing redundancy and accelerating the development of new therapies.
Two articles discuss specifically the most commonly used types of therapeutics: small molecules. Southey and Brunavs explore small molecule drug discovery, outlining the various steps and the challenges in the field. They note that despite well-established protocols and novel knowledge, the process still remains very complex. The authors then present emerging technologies aimed at overcoming current limitations, hopefully making the path to new drug approvals more efficient. In a related discussion, Giraud provides a mini-review explaining how high-throughput screening and biophysical methods are used in the early stages of drug discovery. The article highlights the two main strategies used in the field: target-based and phenotypic-based discovery.
Munsier et al. present a compelling and timely review on the transformative potential of AI in the discovery of biologics, with a particular focus on antibodies, a major therapeutic area driving innovation in drug development. Traditionally, antibody discovery relied heavily on animal models and lengthy experimental processes. The authors highlight the advancements in in silico approaches, which are now capable of accelerating antibody design while reducing reliance on animal testing. AI-driven approaches are presented to showcase the shift towards more efficient and de-risked antibody discovery processes, marking the beginning of an exciting new chapter in developing biologic treatments.
Public engagement and participation are crucial components of advancing drug development. Wang et al. present the results of a survey on public awareness and willingness to participate in drug clinical trials (DCTs) in China. Their findings reveal significant gaps in knowledge and highlight the demographic factors influencing participation rates. The authors call for improved public outreach and communication strategies to foster greater understanding and involvement in DCTs. This could have a significant impact on the success of treatments.
The focus of several articles is about the growing concern of using animal models in drug discovery. Marshall and Conlee discuss the limitations of animal testing, noting the high failure rates of drug candidates that appear safe in animals but prove ineffective or toxic in humans. The article suggests to move towards human biology-based testing methods, which not only promise to be more predictive of human responses but also align with ethical imperatives to reduce animal use. Krebs and Herrmann provide an overview of the international movement towards reducing animal testing in biomedical research. They review new non-animal research approaches that mimic human physiology. Despite of the emergence of these new approaches, the authors acknowledge the persistence of an animal methods bias. They call for a cultural shift in the scientific community, supported by changes in regulatory policies and funding incentives. Hartung offers a balanced perspective on the role of animal models in medical research. This author acknowledges their historical contributions but also highlight their limitations. The article argues for better, more humane alternatives that use novel methods, envisioning a future where drug development is both more effective and ethical.
Together, these articles offer a comprehensive yet accessible overview of the drug discovery process. This Research Topic aims to help the public and patient communities better understand the world of drug discovery, empowering them to engage in the dialogue surrounding drug development. As the field continues to evolve, informed public involvement will be key to shaping a more transparent, efficient, and patient-centered approach to discovering new medicines.
Author contributions
BV: Conceptualization, Funding acquisition, Investigation, Methodology, Software, Visualization, Writing–original draft. J-LP: Writing–review and editing. KT: Writing–review and editing.
Funding
The author(s) declare that no financial support was received for the research, authorship, and/or publication of this article.
Acknowledgments
The Research Topic Editors express their gratefulness to all the contributors for submitting their work to this Research Topic, to the Review Editors, and external Reviewers who participated in the review process, and to the Editorial and Production teams of Frontiers for their valuable assistance through the various stages of the publication process.
Conflict of interest
Authors BV and KT were employed by Akttyva Therapeutics, Inc.
The remaining authors declare that the research was conducted in the absence of any commercial or financial relationships that could be construed as a potential conflict of interest.
Publisher’s note
All claims expressed in this article are solely those of the authors and do not necessarily represent those of their affiliated organizations, or those of the publisher, the editors and the reviewers. Any product that may be evaluated in this article, or claim that may be made by its manufacturer, is not guaranteed or endorsed by the publisher.
Keywords: small molecules, peptides, antibody, therapeutic agents, animal model, drug discovery, artificial intelligence
Citation: Villoutreix BO, Poyet J-L and Tsaioun K (2024) Editorial: Drug discovery and development explained: introductory notes for the general public. Front. Drug Discov. 4:1528467. doi: 10.3389/fddsv.2024.1528467
Received: 14 November 2024; Accepted: 18 November 2024;
Published: 29 November 2024.
Edited and reviewed by:
Liang Xu, University of Kansas, United StatesCopyright © 2024 Villoutreix, Poyet and Tsaioun. This is an open-access article distributed under the terms of the Creative Commons Attribution License (CC BY). The use, distribution or reproduction in other forums is permitted, provided the original author(s) and the copyright owner(s) are credited and that the original publication in this journal is cited, in accordance with accepted academic practice. No use, distribution or reproduction is permitted which does not comply with these terms.
*Correspondence: Bruno O. Villoutreix, YnJ1bm8udmlsbG91dHJlaXhAaW5zZXJtLmZy