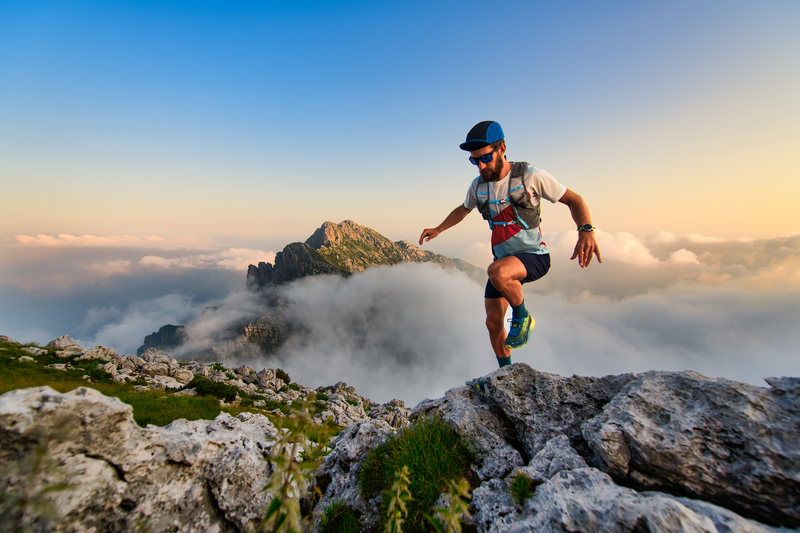
95% of researchers rate our articles as excellent or good
Learn more about the work of our research integrity team to safeguard the quality of each article we publish.
Find out more
REVIEW article
Front. Drug Discov. , 08 April 2024
Sec. Technologies and Strategies to Enable Drug Discovery
Volume 4 - 2024 | https://doi.org/10.3389/fddsv.2024.1355044
This article is part of the Research Topic Drug Discovery and Development Explained: Introductory Notes for the General Public View all 11 articles
Animals like mice and rats have long been used in medical research to help understand disease and test potential new treatments before human trials. However, while animal studies have contributed to important advances, too much reliance on animal models can also mislead drug development. This article explains for a general audience how animal research is used to develop new medicines, its benefits and limitations, and how more accurate and humane techniques—alternatives to animal testing—could improve this process.
Developing new medications is long and challenging. Before a drug can be sold, it must proceed through preclinical studies in cells and animals and usually three phases of human clinical trials: healthy volunteers, a small group of patients to assess patient safety, who may differ greatly from healthy volunteers, and then a large patient trial to prove the beneficial effect. This helps ensure the drug is reasonably safe and effective for its intended use. Animal research in the preclinical phase and in some safety studies continuing in parallel to the clinical studies provides useful but imperfect information about how drugs will behave in people. However, overreliance on animal models results—as I will explain—in many clinical trial failures and unsafe drugs reaching patients. Nevertheless, animals remain necessary until better techniques are available and broadly accepted. This article summarizes for a general audience how animals are used in drug development, their limitations in predicting human responses, and how more accurate human-cell-based and computer models could improve this process.
Historically, from the 1920s to the 1970s, animal experiments were the predominant technology in life sciences. Figure 1 shows how the use of laboratory animals peaked in the 1970s, largely for drug development. Other methods have now begun to complement and even replace animal testing, despite its continued high regard in scientific and regulatory circles. Ethical concerns were the primary drivers for questioning the use of animal experiments; the debate over the justification of animal suffering for scientific advancement varies, but public opinion is increasingly critical. In response, the scientific community has implemented measures to make animal experiments more rigorous, requiring formal justifications, permissions, and adherence to rising standards of animal welfare. Concurrently, there has been significant support for developing alternatives.
Figure 1. This figure, kindly provided by Dr. Andrew Rowan, shows that that all animal use peaked in the 1970s in most industrialized countries. Taken from Wellbeing International (WBI) newsletter 31 December 2021 with permission (https://wellbeingintl.org).
Recent challenges to animal experiments extend beyond ethics. They are resource-intensive, costly, time-consuming, and have limited predictivity for humans—issues highlighted by the European REACH program’s struggle to test thousands of industrial chemicals and by the pharmaceutical industry’s crisis of low success to the market. The latter refers to the extremely high failure rate in clinical trials for drug candidates due to issues like lack of efficacy or safety problems in human testing. These problems have sparked a broader discussion on the “reproducibility crisis” in science.
The drive to find alternatives to traditional animal testing—notably in toxicology, which uses about 10% of all experimental animals (according to European statistics)—has led to significant work in this area. Reasons why most work into alternatives takes place in toxicology include government funding, legislative acts like the European cosmetics test ban and REACH chemical legislation, and the relative stability of internationally standardized guideline tests.
Despite all biomedical progress, we are far from understanding the complex networked systems of the human organism and, even farther, their perturbation in disease. Intervening in these disease mechanisms as a remedy involves much trial and error. Increasingly, identifying a certain mechanism of disease or a possible target for a drug can change the odds of finding something that ultimately works. Such so-called pharmacological “targets” can be, for example, a misbehaving cell type or a receptor protein on cells in an organ that positively influences the course of disease or ameliorates a certain symptom. These observations (on the cell types or receptors) may often occur in animal “models” of disease, and this species difference compounds the difficulty with translating observations from the laboratory bench to the clinic. It is still an enormous undertaking to develop a therapy from this and bring a successful drug to the market.
On average, drug development takes 12 years and costs $2.4 billion. This 12-year timeframe, also called time-to-market, has been quite stable over time. The main reason is that a patent’s lifetime is only 20 years; when it expires, competitors can offer the same drug and so prices plummet. Longer development times therefore eat into the time in which the company must at least recoup the money it has invested. If we simplify that a company has 8 years to recoup $2.4 billion, then every additional day is worth approximately $1 million. However, we must also factor in the many abandoned drug projects which never lead to marketed product. Forbes estimated that, already in 2012, about $4–11 billion was spent by the industry for a single market release. This highlights the importance of each step in the decision tree with respect to time and forgone revenue.
However, some drugs do make tens of billions of dollars per year. This creates a “goldrush” situation (Figure 2). There is in fact some similarity between drug development and a goldrush: it takes many for a few to find something—the abundance of pills on the AI-generated image in Figure 2 is actually misleading. As in a real goldrush, few get rich, and those who sell the sieves and shovels are the ones getting rich. However, “gold washing” often describes the process well, where many stones must be washed to find a rare “golden” pill (Figure 3).
Figure 2. Visualizing the analogy of a gold rush to describe the drug discovery process using DALL-E 3.
Figure 3. Visualization that the “golden pill” is actually a rare find among many rocks, done with DALL-E 3.
Indeed, as summarized in Figure 4, the drug development process requires, as a rule of thumb, about 10,000 chemicals to enter preclinical experiments to ultimately produce one marketed drug. In recent decades, many companies start with even more molecules (sometimes several million in what is called a “chemical library”) to identify some promising structures through robotized testing—so-called high-throughput screening. As no animals are used in this step, it does not change the argument of this article. However, this has also not dramatically changed development times and success rates. Some companies start the search with biological materials such as plants. They often contain several tens of thousands of molecules in these “biological libraries” with the later problem of finding out which in this mix has the desired effect or just being stuck with a “phytopharmaceutical”—essentially, a plant extract. Although many customers like such products, it often requires difficult controls, such as the following: When to harvest? How to process to maximize effects? Are all sources equivalent? How stable is the product? Are there other components in the mix which have negative effects? Such matters will not be discussed here further as the challenge is to prove safety and efficacy, and thus, the role of animal studies and their alternatives is not much different.
To stay with the goldrush metaphor, companies typically hope to make the really big find, not just “a few nuggets from the river.” The dream is gold mines, not gold washing (Figure 5): companies hope for the big wins, the “blockbuster” drug or technology which brings in big money. A “blockbuster” is typically a drug which sells more than $1 billion per year. This can mean finding new important targets (how to change the course of disease), new drug entities such as genetic drugs or nano-particles in more recent years, higher throughput in drug development by faster methods (for example the current discussion around AI-generated drugs—the novel tools that employ artificial intelligence to accelerate drug discovery), and anything promising to lower “attrition,” the so-called failure rate in clinical trials leading to less side effects, earlier detection, or higher efficacy of the resulting medicines. The attrition rate is really the magic number for drug companies. A 2012 study by Arrowsmith et al. showed that 95% of drug candidates failed in the clinical development stage. This means somewhere between $0.9 billion for preclinical development and $2.6 billion investment for full clinical development (using the DiMasi data again), and 19 of 20 drug development projects being abandoned. This is even lower than the rule of thumb that only 1 in 10 substances entering the clinical phase will make it to the market (Figure 4). Some 20%–40% fail because of side effects, or toxicities. Even when a drug makes it to the market, about 8% are later withdrawn, usually because of unacceptably severe or even life-threatening side effects. It has been calculated that 1 in 100 patients in hospital for any reason dies from adverse drug reactions, often from interactions between drugs that patients receive at the same time. The safety of drugs thus continues to be a concern after marketing commences. Typically, a so-called phase-IV trial monitors drugs entering the market to review their safety and efficacy under real-life conditions, and possible drug side effects are also recorded by physicians to build a knowledge base to find rare problems.
Figure 5. Visualization of the difference between gold washing and gold mining as a metaphor for drug development breakthroughs, done with DALL-E 3.
As in any gold rush, there are many animals involved (Figure 6). However, the first question for this article is how much do animals really help? They are costly, take a long time, and have limited reproducibility and predictivity for humans.
Figure 6. Many animals are used in drug development, staying in the metaphor of a gold rush, done with DALL-E 3.
Animals like mice, rats, dogs, and monkeys share much biology with humans, enabling several types of preclinical studies (Box 1). About a century ago, small rodents in particular became a primary research tool in biomedicine, with a supply industry emerging. Until the 1970s, they were the almost exclusive tool for finding new drugs (Figure 2), often in the absence of any idea how they might work. Then, most animals were used in drug development; today, according to European figures, drug development is only responsible for about 20% of all animal use (plus about 5% for drug safety testing and 5% for vaccine batch control); this is an overall drastic reduction of all animal use to about 40% of 1970s numbers. And while drugs required most of animal use in 1970s, it is now about 30%. It should be noted that a culture of systematically testing candidate drugs only emerged after scandals in the 1930s. In the USA, the 1937 sulfanilamide scandal (Figure 7) killed more than 100 people (mostly children), leading to legislation that empowered the Food and Drug Administration (FDA). This disaster was pivotal in the history of drug regulation in the United States. Sulfanilamide was used to treat streptococcal infections and was effectively formulated as a tablet and powder. However, in an attempt to create a liquid formulation, the Massengill Company, a pharmaceutical manufacturer, dissolved sulfanilamide in diethylene glycol (DEG), an untested solvent. DEG is poisonous to humans, but this was not well-known at the time. The company did not conduct any safety tests on the new formulation, which was marketed as “Elixir Sulfanilamide.” The product was distributed widely and resulted in over 100 deaths, many of which were of children, due to kidney failure caused by the DEG.
Box 1. Types of biomedical studies in drug developmentIn vivo: Studies in live animalsIn vitro: Cells, tissues, or embryos studied outside a living organismMicrophysiological systems: Bioengineered in vitro systems, which recreate aspects of organ architecture and functionality, often with perfusion as vasculature equivalent forming (multi-)organ-on-chip systemsEx vivo: Analysis of organs, tissues, or biofluids from treated animalsIn silico: Computational models, increasingly based on artificial intelligence (AI)Toxicology (safety): Testing for toxic and adverse effectsEfficacy: Assessing potential treatment benefitsPharmacokinetics: Absorption, distribution, metabolism, and excretion of the drug
This tragedy highlighted the lack of regulation in the drug industry, particularly regarding the safety and testing of new drug formulations. At the time, the FDA had little power to regulate pharmaceuticals. The Federal Food, Drug, and Cosmetic Act of 1938 was passed in direct response to this incident and significantly increased the FDA’s authority. The Act mandated that new drugs must be proven safe before being marketed, laying the groundwork for modern drug approval processes. This incident is often cited as a turning point in pharmaceutical regulation, demonstrating the critical need for rigorous drug testing and approval processes to ensure public safety.
The safety testing toolbox expanded continuously with problems as a patch for the future. A prominent example was the thalidomide (ConterganR) scandal in the late 1950s and early 1960s, one of the most notorious medical disasters in history. Thalidomide, marketed under the brand name Contergan among others, was introduced as a sedative and later used widely to alleviate morning sickness in pregnant women.
However, thalidomide was not adequately tested for its effects during pregnancy. It was soon discovered that the drug caused severe birth defects in thousands of children (Figures 8, 9) that primarily affected limb development but also caused damage to the ears, eyes, heart, and nervous system. The drug was available in many countries, including Germany, the United Kingdom, and Australia, but was not approved in the United States. The tragedy led to a massive global overhaul of drug testing and regulatory processes. The extent of the birth defects caused by thalidomide brought to light the need for rigorous drug testing, especially for teratogenic effects (the potential to cause fetal abnormalities). In response, many countries strengthened their drug regulation laws and the processes for drug approval, making them more stringent and emphasizing the need for comprehensive clinical trials, including assessing effects on pregnancy. Since then, testing on animals has provided initial safety and efficacy data not ethically possible from humans. However, due to biological differences, small study sizes, and lack of diversity, animal research has important limitations. The thalidomide scandal remains a critical example in medical and regulatory circles of the importance of thorough drug testing and the potential consequences of inadequate drug regulation.
Figure 8. Title page of German weekly journal Der Spiegel from 5 December 1962 titled “Sick by remedies”, archive of the author.
Figure 9. Excerpt from 1966 letter by the FDA to Procter & Gamble on introduction of the two-generation study for reproductive toxicity, following the thalidomide scandal (courtesy of Dr. George Daston, P&G).
George Daston from Proctor and Gamble shared with me a letter from the FDA to their company in 1966 (Figure 10), when the “patch” for the reproductive effects of substances was created. It shows quite nicely how the increasing number of toxicity concerns led to an enlarged toolbox of safety tests. Notably, the letter ends “It must be realized that even these improved guidelines reflect merely the ‘state of the art’ at the present time, and undoubtedly further modifications will be needed in the future as additional knowledge in this area is developed.” In fact, the very demanding animal study done on rats and rabbits did not even reliably detect the teratogenic effects (causing birth defects) of thalidomide. Several factors contribute to this discrepancy:
• Species-specific differences in drug metabolism and sensitivity: different species metabolize drugs differently. Thalidomide’s teratogenic effects are highly species-specific. It is known to cause birth defects in certain strains of mice, primates, and specific breeds of rabbit, but not in others, including the standard laboratory strains of rats and rabbits. This highlights a crucial limitation of animal studies in predicting human outcomes due to interspecies variability.
• Mechanism of action: thalidomide’s teratogenic mechanism is complex and until recently not fully understood. It involves multiple pathways and is influenced by genetic and environmental factors that may not be present or that may differ significantly in animal models compared to humans.
• Dosage and exposure timing: the manifestation of thalidomide’s effects is highly dependent upon the timing of exposure during pregnancy and the dosage. These factors can vary greatly between humans and animals, affecting the outcome and reliability of animal studies.
• Lack of early detection methods: when thalidomide was introduced, the methodologies for detecting teratogenic effects, especially subtle ones or those manifesting later in development, were not as advanced as today. This limited the ability to detect such effects in animal studies.
Figure 10. Height distribution by gender, This dataset was created by an unknown author for the blog “Why Sex Differences Don’t Always Measure Up” available at https://sugarandslugs.wordpress.com/2011/02/13/sex-differences/(last accessed 12 December 2023).
The thalidomide tragedy fundamentally changed the way drugs are tested for safety, underscoring the need for more predictive and human-relevant models in teratology. It led to stricter regulatory requirements for drug testing, including the need for more comprehensive animal testing and the development of alternative methods to better predict human outcomes. However, the case of thalidomide remains a classic example of the limitations of animal models in accurately predicting human drug responses, especially in the context of developmental and reproductive toxicity. It is also a showcase of how, over almost 60 years, the “quick fix” of 1966 has not been replaced. The shortcomings mentioned when introducing the test have been forgotten, and it is now a standard that is difficult to replace. “We just got used to it”, in the words of Petr Skrabanek and James McCormick in their wonderful book Follies and Fallacies in Medicine (Tarragon Press, Glasgow, 1989): “Learning from experience may be nothing more than learning to make the same mistakes with increasing confidence.”
On the other hand, aspirin, a widely used medication, presents a notable case where animal studies (would have) made findings that are not entirely relevant or predictive of its effects in humans. In my 2009 article “Per aspirin ad astra,” I critically examined the implications of traditional animal testing methods, underscoring the paradox of aspirin’s toxicological profile—its widespread acceptance was fortunate due to the lack of stringent regulatory toxicology in 1899. In animal models, aspirin has demonstrated a range of toxic effects that are not typically observed in humans or that are observed under different conditions. These discrepancies highlight the limitations of extrapolating data from animal studies to human physiology and medicine. Aspirin when ingested is classified as harmful, with an LD50, or lethal dose of 50%, to the rats used in testing, ranging from 150 to 200 mg/kg for the rodents, which is exactly the maximum daily dose used in humans. This is not a 100–1,000-fold safety factor usually suggested by toxicologists to indicate acute toxicity. Aspirin irritates the eyes, respiratory system, and skin. Although it is not directly carcinogenic, it acts as a co-carcinogen, meaning that it can promote cancer in the presence of other carcinogenic agents. Its mutagenic potential remains unclear, suggesting uncertainty about its ability to cause genetic mutations. Studies in various animal models, including cats, dogs, rats, mice, rabbits, and monkeys, have shown that it causes embryonic malformations—but not in humans, where one study analyzed 90,000 pregnancies. Due to this extensive profile of harmful effects, it is likely that such a substance would face significant challenges in the drug approval process today, making it unlikely that it would be brought to the market. In a 2009 article, I looked critically at traditional animal testing methods using the example of aspirin. I highlighted the paradox that aspirin is widely accepted and used despite results from animal tests that might have blocked its initial approval under today’s strict rules.
Animal studies show that aspirin can have a range of toxic effects not typically seen in humans, or only at very high doses rarely used in patients. For example, tests suggest that aspirin is quite toxic based on lethal dose experiments in rats using the same maximum daily levels given to people. Animal studies also indicate that it may irritate eyes and airways and possibly act as a co-carcinogen—promoting cancer development alongside other chemicals. Its effects on potential gene mutations also remain unclear. Meanwhile, additional animal research implies that aspirin might cause birth defects, which over 90,000 human pregnancies that have been tracked disproved.
Due to this concerning toxicology profile from animal tests, aspirin likely would have faced major obstacles getting initially approval if today’s stringent safety regulations existed back in 1899. The conflicting results between laboratory animals and human patients highlight limitations in using animal studies alone to predict safety in people. Findings in animals do not always match up with outcomes when drugs are actually given to diverse groups of people. Therefore, while useful, data from animal models have major shortcomings that impact the progress of drugs from early laboratories to patient bedsides.
Remarkably, until the 1970s, there were no efficacy data, meaning that convincing evidence that drugs are promising for curing a disease were formally required, just that they are not likely to cause harm.
Drugs today are developed to act through a defined target—a structure or component of the body to be altered by the treatment. Such targeted therapeutics are designed to specifically effect molecules associated with disease, unlike traditional chemotherapies, for example, that can also damage healthy cells. This increased specificity aims to improve treatment effectiveness and reduce side effects. However, developing a targeted therapeutic is a long, expensive, and risky process, taking on average about 12 years. Extensive testing in animals plays a crucial role in this process—typically 10-20,000 animals per drug development today. The Nuffield Council on Bioethics has estimated that 5%–15% are used to identify targets for drug action and possible medicines, 60%–80% for lead identification and optimization—choosing the optimal candidate substance—and 10%–20% for selecting candidate medicines going into clinical trials. Notably, according to European statistics, the pharmaceutical industry uses about 20% of all laboratory animals for drug development, down from about 30% in 2005, despite increasing research spending indicating that the industry is transitioning to other methods. The continuing need for animals is because cell cultures and computer models cannot replicate the full complex biology of a living organism. The traditional view is that animal testing provides invaluable data about real-world efficacy and safety that often cannot be obtained by other means. Preclinical testing with a combination of animals and, increasingly, other tools enables researchers to select the most promising candidates to move forward into clinical trials. This minimizes risks to human participants and increases the chance of success in later-stage clinical testing. Although targeted therapeutics provide exciting possibilities for treating disease, developing them often requires extensive animal research. Preclinical testing in appropriate animal models is still an essential part of bringing safe, effective targeted therapies to the clinic. The high degree of similarity between many animal species and humans leads many researchers to believe that this enables key data to be collected for guiding therapeutic development and improving human health. This rather optimistic view of the role of animal studies in drug development is slowly being eroded, given the perceived inefficacy of the process of drug development with its many failures in the clinical (attrition) phase and increasing cases where the limitations of animal testing have been apparent. Importantly, the use of animals is also prompted by the expectations of regulators of receiving such data for decision-making and the fear of the industry that not meeting these expectations will result in delay or even refusal of registration.
Once safety and efficacy are demonstrated in animals, the most promising targeted therapeutic candidates advance to testing in humans. Clinical trials are performed sequentially in healthy volunteers and patients with disease to definitively determine overall benefit and risk. Animal research provides the foundation of knowledge necessary to justify testing new drugs in people.
Although the historic cases of thalidomide and aspirin shed some light on how the safety testing of drugs was introduced and was flawed from start, this section will address limitations more systematically and with more recent examples. Although somewhat useful, animal models frequently fail to predict human clinical trial outcomes. Reliance on inadequate animal data results in the following:
• Many false negative errors: potentially good drugs are abandoned due to lack of efficacy or side effects in animals that would not occur in human trials (which never happened because of the animal findings).
• False positives: drugs that “work” in animals may still fail in human trials.
• Adverse events and safety issues in human volunteers and patients that were missed by prior animal testing.
• Several factors limit the accuracy of animal models, including biological differences; inbred strains vs. genetic diversity in humans; often young, healthy animals, unlike aged, sick humans; molecular differences altering drug effects; artificial experimental conditions; housing, diet, and environments that differ from human lifestyles.
• Disease that is induced artificially may differ from naturally occurring illness.
• Study design: small, short studies vs. lifelong human exposures; high doses triggering irrelevant effects; each test uses limited animal groups unlike large, diverse human trials.
• Animal research retirement is not yet feasible but should be reduced. Imperfect animal models need to be supplemented with more reliable human-based techniques such as: miniature bioengineered “organs-on-chips”; advanced computer models of human disease; big data mining of patient health records and genetic databases; small, carefully designed human clinical studies.
Used intelligently in combination, old and new methods can transform drug development to reliably predict safety and benefits for patients. Scientists have an obligation to use the most predictive tools available to efficiently develop effective medicines.
Animal testing has been an entrenched part of drug development for decades. However, there are numerous concerning examples where animal tests have misled clinical development due to inherent physiological differences between species, leading to dangerous outcomes in human trials.
The immunosuppressant drugs cyclosporine and tacrolimus, widely used today to enable organ transplantation, were almost abandoned because animal toxicities failed to predict efficacy and safety in desperate patients. Corticosteroids, in contrast, appeared beneficial in animal models of septic shock but worsened mortality rates when administered to critically ill patients.
An Alzheimer’s vaccine caused severe brain swelling in early human trials despite appearing safe in animal tests. A 2006 “cytokine storm” induced by an immunomodulatory agent by Tegenero left healthy volunteers with catastrophic organ failure, despite prior animal studies being unremarkable. In 2016, one volunteer died and four suffered severe neurological damage in a French trial, although the drug showed promise and acceptable safety margins across four animal species. Severe liver injury and multiple deaths forced the termination of a hepatitis B drug trial despite earlier encouraging animal data. Differential species sensitivity to drugs like acetaminophen further highlights the pitfalls of reliance on animal models. Gene therapy vectors that have been safe in animal tests have caused liver failure and brain swelling in children. HIV vaccines, stroke treatments, inflammatory disease agents, and Alzheimer’s therapies have all elicited enthusiasm in animal models yet utterly failed in human trials.
These sobering examples have played out over decades, leaving patients dead or devastated in their wake. Notably, while these included extreme examples of unanticipated side effects, many milder problems might never be detected as patients already have many health problems and the additional negative effects of drugs are not easy to identify. On the other hand, many potentially lifesaving medicines may have been lost at the same time because they performed poorly in flawed animal models. Recurrent failures speak to inherent limitations of evolving human treatments in divergent species. These cautionary tales underscore growing calls to move away from unreliable animal testing toward human-relevant alternatives for future drug development.
The last chapter gave some anecdotal examples of limitations of animal tests. Over the last decades an approach, which is called a systematic review, has evolved, which defines clearly upfront the question of interest and how to find the respective evidence and analyze it. This has been applied to some extent also to the value of animal testing.
The Systematic Review Centre for Laboratory-animal Experimentation (SYRCLE) works to improve the quality and reliability of animal studies used in drug discovery. One of the main tools developed by SYRCLE is the “risk of bias” (RoB) tool, which aims to assess the methodological quality of animal studies and has been adapted for aspects of bias that play a role in animal experiments. The tool is designed to enhance transparency and applicability, and it includes signaling questions to facilitate judgment. The widespread adoption and implementation of this tool are expected to facilitate and improve the critical appraisal of evidence from animal studies. This may subsequently enhance the efficiency of translating animal research into clinical practice and increase awareness of the necessity of improving the methodological quality of animal studies. SYRCLE identified that a significant portion of animal research is conducted at a low standard, leading to unreliable data. This includes low rates of random allocation, allocation concealment, and blinded outcome assessment, all of which contribute to an overestimation of the benefits of experimental interventions. Furthermore, animal research often suffers from selective analysis and biased outcome reporting, where only the most positive outcomes are reported. This leads to an inflated proportion of studies with positive results and an overestimation of beneficial treatment effects. Systematic reviews have also highlighted redundancy and waste in animal research, with continued experimentation even after beneficial effects were already well documented, leading to unnecessary use of animals and resources. There is evidence that shortcomings in almost every aspect of the scientific design, conduct, and reporting of animal studies contribute to their inability to translate into benefits for humans. Such findings indicate the need for improved methodological quality in animal research to ensure its clinical relevance and enhance its efficiency and reliability translating into clinical practice.
SYRCLE also advocates for the registration of all animal experiments at inception and the publication of protocols of animal studies in various journals. These practices are expected to improve the standard of research in animal sciences. However, it is important to note that animal studies have inherent limitations and can sometimes be misleading in drug discovery. For instance, a drug that shows promise in animal models may not necessarily be effective in humans due to species-specific influences and differences in biology. Importantly, SYRCLE recommends that the risk of bias assessment should be conducted by at least two independent reviewers to ensure objectivity and that any disagreements be resolved through consensus-oriented discussions or by consulting a third person. This approach underscores the need for critical and unbiased assessment in animal studies, which can significantly impact the translation of research findings from animal models to clinical applications. In summary, the work of SYRCLE, particularly through its RoB tool, has been instrumental in identifying and mitigating bias in animal studies, thereby enhancing the reliability and translatability of these studies into human clinical research—especially in the context of drug discovery. Therefore, while tools like SYRCLE’s RoB tool can help improve the quality of animal studies, they cannot completely eliminate these fundamental challenges.
A review by researchers at Astra Zeneca found that over half of the protocols for forthcoming animal experiments needed amendment for proper experimental design, appropriate sample sizes, and measures to control bias. Additionally, revealing reports from pharmaceutical companies have found that much data from academia are irreproducible, indicating problems of poor experimental design and scientific conduct, as well as incomplete reporting.
The Collaborative Approach to Meta-Analysis and Review of Animal Data from Experimental Studies (CAMARADES) is a research group that aims to improve the quality of preclinical research, particularly in the context of animal studies used in drug discovery. CAMARADES works to address these issues by promoting rigorous, high-quality, and transparent animal research. This includes advocating the use of systematic reviews and meta-analyses, improving experimental design and reporting, and developing new methodologies to assess the quality of preclinical research. CAMARADES is a database that tracks the reliability and limitations of animal research used in drug development and disease research. It was created in response to the recognition that animal studies frequently do not translate to humans, wasting resources and potentially misleading medical research. For example, one analysis found that only 37% of highly cited animal research was translated at the level of human randomized trials. Another study found that only 8% of basic science discoveries enter routine clinical use within 20 years. The reasons why animal studies can be misleading include differences in biology and physiology between species, poor experimental design and reporting, publication bias, and overinterpretation of results. CAMARADES reviews animal studies systematically and critically to assess their limitations and risk of bias. The goal is to improve the design, analysis, and reporting of preclinical animal studies so that their results are more relevant to human health and avoid wasted resources. CAMARADES has reviewed numerous animal studies of drugs and conditions like stroke, amyotrophic lateral sclerosis, and sepsis, demonstrating how animal models failed to predict human outcomes. Overall, CAMARADES aims to act as a watchdog for animal research, promoting more rigorous methodology and cautious interpretation to prevent animal studies from misleading medical research.
While flawed, animal research remains necessary for developing new medicines. Some of the reasons it persists include:
- Living systems: animals are complex living organisms that cannot yet be mimicked in the laboratory. Seeing responses across multiple organs over time requires whole-animal studies.
- Rules and expectations: regulators overseeing drug safety expect animal data before human trials can proceed. Companies must comply to keep development programs on track.
- Early safety checks: animal tests allow safety assessments at high doses so that lower, likely safe human doses can be set. Without animals as a first check, putting chemicals into people would be too risky.
- Mechanism exploration: animal tests shed light on disease mechanisms and biological pathways to help guide human research, despite not always directly predicting outcomes.
Hence, while animals are far from ideal for predicting human drug responses, they fill important gaps until technology like organ chips and computer models can provide comparable living system data (as discussed below). Animal research therefore remains ingrained in medical advancement at present.
Such strategies include the following:
1) Assessing for flaws: tools that help analyze the quality of animal methods to improve their applicability to humans.
2) Tracking outcomes: the registration of animal trials enables the subsequent tracing of results versus initial expectations.
3) Documentation: cataloging animal study successes versus failures can inform realistic healthcare promises.
Additionally, boosting reproducibility—consistency in results—returns more knowledge per animal used. This involves careful experimental design and transparent outcome reporting, whether positive, negative, or inconclusive. In summary, wasting animal lives on poorly designed, biased research is unethical, but ensuring the thoughtful conducted of robust animal studies via quality checks while tracking outcomes will advance human medicine with care while alternatives are developed.
A helpful estimate for the accuracy of a test is its reproducibility as no test can be more accurate than it is reproducible. Reproducibility in animal testing is a significant concern in the scientific community, with many studies highlighting the challenges and proposing strategies to improve the situation. One of the key issues affecting reproducibility is biological variation, which can cause organisms’ responses to experimental treatments to vary with both genetic differences and environmental conditions. Another contributing factor is the extreme standardization of trial design, which can lead to different results with slight deviations in test conditions. Even with well-planned and well-reported protocols, reproducibility is not automatically guaranteed. This is known as the “reproducibility crisis”, which has led to a growing awareness that the rigorous standardization of experimental conditions may contribute to the poor reproducibility of animal studies.
Estimating the overall accuracy of animal testing in predicting efficacy and safety in human trials is challenging, based on available data. Animal studies seem to have relatively low accuracy for predicting efficacy—estimates range from about 37% to 60% correlation with human outcomes, suggesting substantial limitations. The models remain quite imperfect for their core intended purpose. There are significant inter-species differences in biology and disease progression for even highly conserved pathways. However, animal studies seem moderately accurate regarding safety, with estimates of about 70% accuracy for identifying toxic side effects that also manifest in humans. So, while still imperfect, animal testing appears, on average, better attuned to flagging potential safety issues that translate across mammals. Overall, however, predicting efficacy via animal models seems scarcely better than a coin flip based on meta-analysis. However, for safety, animal testing achieves perhaps higher accuracy under optimal conditions. Combined into an overall likelihood of success, this aligns with very high late-stage drug failure rates; animal studies do not sufficiently recapitulate human biology to reliably identify those rare winning drug candidates out of the thousands investigated. Improved models and biomarkers remain a key necessity.
These accuracy limitations highlight why robust statistics and good judgment are so crucial when interpreting pre-clinical animal research for candidate prioritization and advancement decisions—a nuanced understanding of what questions different models can actually address is essential to avoid wasting of resources by chasing false signals.
Testing means that individual chemicals are subjected to a measure to classify them as belonging, in the simplest case, to either of two classes—for example, effective on a target or not, or toxic/non-toxic. The problem is that there are no perfect tests, and some misclassifications occur: “false-positives” (ascribing a property which something does not have) and “false-negatives” (missing an individual chemical that is, in fact, a property). The basis for a test is that we can measure something which distinguishes the two groups. For example, if we want to distinguish male and female individuals, we might exploit the difference in height. This might not be the best possible characteristic, as Figure 10 shows. However, if we take a cut-off of 1.70 m, we actually identify about 90% of women and include only 35% of all men—27% of the below-1.70 m group are men, or an accuracy of 77.7%. This is about the accuracy we can hope to achieve with an animal experiment when looking for a property. While this works astonishingly well, what happens when there are less women in the group to be analyzed? If we assume that the number of women is only one tenth of the actual proportion, we now still find 90% of these and the same number of men as before. The problem is that we still find the same number of small men, who are now 79% of all identified, so the false-positives (men mistaken as women based on height) now predominate. The accuracy drops to 68% if we continue and reduce the number of women in the group again to another 10th, so the real women found are less than 4% of all small people identified, corresponding to an accuracy of 66%. Why is the accuracy still that good when only one woman per 25 men is among those identified? Because the method is very good at identifying non-women: the large number of tall men with now very few tall women is making the test fairly reliable for identifying them. This is a very fundamental problem, which most people do not understand: we test our methods with more or less equal numbers of what we want to identify, and our methods do very well. Then, we move to real life, and there are very few suitable substances we want to identify among those we evaluate. This is called the prevalence problem (see next section).
This example can also serve to show how we can change the performance of the test by setting our cut-off. We can make the test more sensitive (find more of what we are looking for) or more specific (minimize the false calls). The cut-off at 1.70 m found 90% of women, but of all called women, only 72.9% were in fact women. Changing the cut-off to 1.60 m finds only 46% of all women. Changing to 1.80 m finds 89.7% of all women but only 58.2% of those identified were correct. The accuracy of the test drops from 77.7% to 64.1% and 62.4%, respectively. This illustrates how our choices allow us either to be confident in the result (specific) or not to miss out on positive things (sensitive). Translated to drug screening, this means either quickly reducing the number of possible substances or being careful not to lose the good ones.
When developing new drugs, researchers face a tricky problem: many of the effects they seek, both positive and negative, are quite rare. For example, out of thousands of drug candidates tested, only a small percentage end up being sufficiently safe and effective to bring to market as approved treatments. Regarding safety, dangerous side effects may also only occur in a tiny fraction of patients, still prohibiting their use. This means that, even when using very good laboratory tests and clinical trials, it can be hard to reliably detect these rare events—a drug could fail late in development over toxicity seen in 1 in 10,000 people, for instance. So scientists must test large numbers of drug candidates and use very large patient groups, which takes extra time and money. Careful testing design and statistics are key to properly estimate the likely benefits and risks of dealing with such low probabilities. Just as diagnostic tests in medicine work best for common diseases, the drug development process works far better for more prevalent drug effects. Clever ways to accurately find “needles in the haystack” during development is a permanent challenge; the intrinsic challenge of identifying rare yet significant events hampers the discovery of a truly effective drug or the detection of uncommon toxic effects of drug candidates. The vast majority of compounds investigated do not make it to market, either due to lack of efficacy or to adverse effects that may only be evident in a small fraction of the population or under specific conditions. This “needle in a haystack” problem is compounded by the fact that preclinical models, such as animal studies, do not always accurately predict human responses. Consequently, a drug that appears promising in preclinical trials may fail in clinical phases due to unforeseen toxicities or lack of therapeutic effect. On the other hand, a potentially useful drug might be erroneously discarded if its benefits are not readily apparent in the early stages of testing or if its side effects are overrepresented in preclinical models. Therefore, the efficiency of drug development is often hindered by the difficulty of extrapolating data from a limited set of preclinical results to the diverse human population, where genetic, environmental, and lifestyle factors can greatly influence drug responses. The prevalence problem underscores the need for more predictive models and testing methods that can better capture the complexity of human biology and disease. As in diagnostics, the predictive value of clinical trials decreases dramatically the less prevalent an outcome is. Companies must account for this limitation with very large and lengthy studies, at substantial cost. Clever trial designs to accurately detect these “needles in the haystack” remain an ongoing necessity in drug development.
Rare phenomena of high impact are sometimes called “black swan events”. Nicolas Taleb in his book The Black Swan (2007) used this metaphor to especially describe events on the stock market. He defines black swan events by the “…triplet: rarity, extreme impact and retrospective (though not prospective) predictability.” This is exactly what drug discovery is: real hits are rare, they are a goldmine, and arguably, we can explain why they work so well only in retrospect. The identification of a new marketable drug requires much searching and luck. The same can be said inversely of the rare toxic effects of drugs coming to the market. Side effects which only occur in one in 1000 or 10,000 patients cannot be predicted: they are black swans. Taleb notes, “What is surprising is not the magnitude of our forecast errors, but our absence of awareness of it.” This is when the black swan hurts. “True, our knowledge does grow, but it is threatened by greater increases in confidence, which makes our increase in knowledge at the same time an increase in confusion, ignorance, and conceit.” This notion can easily be translated to adverse drug effects, where late discoveries of highly problematic side effects are rare but game-changing events.
A prime example is the case of the painkiller Vioxx (rofecoxib). Vioxx was initially hailed as a breakthrough for its effectiveness in relieving pain with fewer gastrointestinal side effects than other painkillers. This was a significant development, given that gastrointestinal complications are a common and serious side effect of the long-term use of such drugs. It was approved and marketed for 5 years before being withdrawn due to increased risk of heart attack and stroke. During clinical trials, it was observed that 2.4% of the 1,287 participants taking Vioxx suffered serious cardiac events, such as heart attacks, chest pain, or sudden death. This rate was notably higher than the less than 1% of patients who received a placebo. This significant increase in risk, although relatively small in percentage terms, led to the drug’s withdrawal from the market due to safety concerns. The problem was that these cardiovascular risks occurred in only a small proportion of patients—about 1 in 200 over a year of treatment based on later analyses. So even with thorough testing, this rare side effect was initially missed. The company had to spend over $100 million on one study alone to properly detect these risks, requiring a huge sample of over 24,000 arthritis patients. Since heart disease progresses at a background rate regardless, only by analyzing such large numbers could Vioxx’s small but real added risk be identified. The Vioxx case illustrates why finding rare adverse events or benefits is so difficult during development; even the most rigorous testing can miss effects that occur at rates of less than 1 in 1000. In preclinical trials and early clinical studies, Vioxx did not show significant adverse effects and was therefore approved by the FDA. However, after it was widely marketed and prescribed, it became apparent that there was an increased risk of heart attack and stroke associated with its use, which was not evident in the smaller, controlled clinical trials. The economic consequences of the Vioxx withdrawal were profound and multifaceted. Vioxx, which had been on the market since 1999, was generating over $2.5 billion annually for Merck, accounting for approximately 10% of its worldwide sales. When the drug was withdrawn in September 2004, Merck’s sales plummeted, and the company’s stock value took a significant hit. Moreover, the withdrawal triggered numerous high-profile product-liability lawsuits, leading to years of litigation that cost Merck billions of dollars. The Vioxx case remains a cautionary tale in the pharmaceutical industry, illustrating the staggering financial risks when safety concerns emerge post-market. The industry continued to feel the repercussions of the Vioxx withdrawal up to a decade later as it highlighted the vulnerabilities in drug safety surveillance and the potential for significant economic loss when widely prescribed medications are retracted. The Vioxx case demonstrates the prevalence problem where rare but critical adverse events may not be detected until after a drug is approved and taken by a large and diverse patient population. It also shows the limitations of preclinical models in predicting human real-life outcomes, given that the cardiovascular risks associated with Vioxx use were not captured in earlier studies. This highlights the need for more comprehensive and sensitive methods for detecting rare events in drug safety and efficacy evaluations. We will later discuss the opportunities of human-relevant bioengineered models (microphysiological systems), mechanistic understanding, and big-data-driven analyses and modeling.
Another prime example is the cholesterol-lowering drug Lipobay (cerivastatin), which was withdrawn in 2001 after reports of serious muscle toxicity (rhabdomyolysis). This side effect occurred in approximately 1 out of every 1000 patients per year who took the approved dose. While quite rare, the results could be fatal. Even though cerivastatin had undergone extensive laboratory testing and clinical trials with thousands of patients prior to approval, this low probability meant that the risk was initially missed. The analysis of over a million patient years of post-approval prescription data was required to finally detect and quantify the risk. The Lipobay case, like that of Vioxx, demonstrates how developing or approved drugs can fail to identify rare but dangerous risks that only show up when tested in extremely large populations. As in medical diagnostics, even rigorous testing can easily miss outcomes that occur at rates less than around 1 in 1000. Companies must account for this limitation by conducting very large and lengthy studies to properly estimate safety and efficacy; however, even these might not be large enough to conclusively rule out some risks. Careful trial analysis for faint signals in the data is crucial.
As seen above, drug discovery means ultimately finding one marketable drug out of more than 10,000 chemicals. The problem is that our tools are far from perfect. This holds for both animal tests and their alternatives. This is like solving a riddle with glasses not tailored to our eyesight. Let us assume that an animal tests deliver 90% correct results—a relatively high bar, with no more than 80% accuracy much more likely; however, for illustration, assume a 90% accurate animal test to try to discover one approvable drug out of 10,000 chemical candidates. Testing 10,000 chemicals, the 90% accurate test would correctly identify the one truly effective compound that will ultimately make it to market. However, with 10% false positives, it would also flag around 999 other chemicals as “hits” that will actually fail later. So, while not missing the promising needle in the haystack, initial results are unable to distinguish it from almost a thousand false positives. A large fraction of those 1000 extras would drop out in further rounds due to other limitations of course—but companies might still fruitlessly pursue 100 through later stages as if they were promising, based on the inaccurate early read. This thought exercise illustrates why, despite relatively good animal tests, failure rates in human trials remain high—rare actual positives get lost amongst the noise of greater numbers of false signals when working in domains of very low prevalence. Clever multi-parameter testing is important, but statistics dictate inevitable disappointment much of the time.
Here is an illustration of what would happen if the 1000 chemicals flagged as positives from the first 90% accurate animal test were run through a second, independent 90% accurate animal test: putting those 1000 chemicals through a second 90% accurate animal test independent of the first (an unlikely assumption, but useful here) might help refine the list, but major issues remain due to the low prevalence. The true promising drug would be confirmed, while around 900 of the original 999 false positives would now test negative and could be set aside. However, around 100 (10%) of those false leads would be incorrectly flagged positive again. Therefore, out of 110 total positives between the two tests, only one is the real winner, over 100 remain misleading false leads, and optimization between tests still cannot avoid this. Even added testing helps far less than intuition would suggest when fundamental probabilities are so low. Statistics dictate that reliability decreases exponentially the less prevalent the needles sought in research haystacks become. At huge scale, noise drowns signal without escape. While these are simple examples for illustration, these dynamics genuinely occur in real drug development pipelines, contributing to late failure and showing how proper expectations are vital when hunting for rare events like a 1-in-10,000 for a future drug (Figure 11).
Figure 11. Illustration of the consequences of limited accuracy of finding 1 in 10,000 drug candidates The calculations show how less accurate tests require more testing rounds to bring the candidates down to about 10, which can be managed in clinical trials but also increase the risk of losing the winning one. If we assume that there are 10 equally good candidates, there is a much better chance that at least one will proceed to clinical trials.
If we assume a series of independent 90% accurate animal tests to narrow down the initial 10,000 compounds, we can analyze the number of tests to get to 10 remaining candidates, and the cumulative risk of losing the one truly promising compound:
*Round 1 test: ∼1000 compounds flagged as positives (= candidates, ∼1 true, ∼999 false)
*Round 2 test: ∼100 compounds flagged as positive (on average ∼1 true, ∼99 false)
*Round 3: ∼10 flagged (∼1 true, ∼9 false)
It thus takes three sequential 90% accurate tests to narrow the 10,000 down to 10 compounds (one likely true positive, nine remaining false positives). However, there is in each round a 10% chance of the truly promising compound testing negative in one of the rounds and being incorrectly discarded. Over three tests, this means that there is actually a 27% chance (1–0.93) that the best candidate is lost along the way. This demonstrates how prevalence limitations mean that even an unlikely-to-achieve series of nearly perfect laboratory tests carries large risks of losing the rare “needle” when searching complex multidimensional haystacks like potential drug spaces. Confirming true signals remains improbable until late, so balancing information gain versus discarding promising niche opportunities remains an ongoing challenge throughout the drug discovery pipeline. What happens when we use less than ideal tests? Using 80% accurate tests, we need four rounds to get us to ∼16 compounds, and the likelihood of still including the golden one is 41%. Using a series of 70% accurate tests, six test rounds will get us to ∼7 compounds with only a 12% chance of still having the one we are looking for included.
These calculations assume that there is only one marketable substance in the 10,000 we start with. That is probably not the case. Assuming that were ten suitable compounds among the 10,000 at start, a series of 90% accurate tests gets us, in three rounds, to ∼17 compounds including seven possible winners, and 80% accurate tests in four rounds to ∼20 compounds with four possible winners, as well as 70% accurate tests leading in six rounds to ∼9 candidates including one promising one. The latter scenario seems to best agree with the experience that one in ten compounds later prove to work in clinical trials, with some of the numbers around 70% for the reproducibility of animal studies.
If we come back to the sensitivity vs. specificity discussion from above, we can illustrate the consequences here. We saw that 70% accurate tests (equal sensitivity and specificity) in six rounds brought us to nine compounds (one good). If we now use 80% sensitive/60% specific tests, we need seven rounds to get to 19 (two good ones), while the opposite 60% sensitive/80% specific gets us to 17 in four rounds (one good one). This again illustrates the compromise between sensitivity and specificity: higher specificity sorts the compounds faster at the risk of losing the winner; higher sensitivity means more effort (seven rounds) but no real gain in the probability of including the winner.
Do we know the accuracy (sensitivity and specificity of animal tests)? Often not, because that requires an assessment of the assay against some reference, such as chemicals, which are known to do what the drug discovery is seeking. We call this “target validation”. The above calculations thus better serve the purpose of explaining why so much testing does not necessarily lead to substances which succeed in the clinic.
Safety testing—that is, toxicology —traditionally occurs just before human trials and in part concurrently. This means that the ∼10 compounds entering the clinical phase of drug development need to be considered. Applying the estimated 70% safety accuracy of animal studies to a scenario with a 20% prevalence of a toxicity across 10 candidate compounds means that two compounds would be truly toxic to humans and eight would be truly safe. Using a hypothetical 70% sensitive/specific animal test on the two toxic compounds, it would correctly flag one or two as toxic; of the eight safe compounds, it would correctly identify six to seven. It would also incorrectly flag one to two of the safe compounds as toxic and misidentify one of the truly toxic compounds. Therefore, even with a relatively high prevalence toxicity of 20% and a good animal test with 70% accuracy, predictions can easily miss 25% of the unsafe human compounds while allowing unsafe candidates through at a 10%–20% rate. This demonstrates how testing limitations can quickly add up, even under idealized conditions—rare but dangerous outcomes get missed completely, and false safety signals erode confidence in labeling. Layered risk mitigation is key, but balancing information value against decision risk given the constraints around rare event prediction remains highly challenging throughout pharmaceutical pipelines.
In real life, not all steps will be run on all compounds. Such a brute-force approach is simply not realistic and affordable. Early rounds will likely be done with simple in vitro and in chemico tests with limited scope but better reproducibility. With additional information such as the intellectual properties for chemicals, ease of synthesis, estimated environmental stability, and chemicophysical properties, lead compound selection will proceed faster. Often, new chemical structure variants will be brought in on the lead-optimization phase. This does not necessarily improve the odds of ultimate success as this is somewhat a gamble based on experience and circumstantial information. It is quite possible that these considerations have a similar accuracy of about 70% and thus leave us within the calculations; in fact, 20 years ago, Romualdo Benigni and colleagues had scientists guess the outcome of cancer tests on chemicals and achieved 60%–65% accuracy. So, they were about as good as mathematical models or the reproducibility of cancer testing itself. The above scenario also assumes that the different tests per round are independent; this is very unlikely as they are all built around the same pharmacological target, which reduces the probability of success. So this represents a theoretical exercise of testing all and everything in a sequence of test rounds, which serves mainly to illustrate how the tools stand up against the task.
There are a few approaches that can help improve the odds when searching for extremely rare positive events, like 1-in-10,000 successful drug candidates:
1) Test more compounds: this helps detect more of the few true signals hiding amidst the noise. This is accomplished with robotized testing—so-called high-throughput testing with libraries of often millions of chemicals. Artificial intelligence can examine even more theoretical structures, but the contribution of this new approach is still to be shown. However, returns of actual testing more compounds diminish quickly and costs scale up, limiting feasibility. This is only possible with broader use of non-animal methods.
2) Using more replicates per test such as larger animal groups: while this increases the accuracy of tests when variability is the problem, it again increases costs, effort, and animal use.
3) Multi-parameter testing: assessing multiple aspects of each compound provides backup if the primary indicator is misleading. However, interpreting interactions quickly becomes complex, and such “multiple testing” can weaken statistical power.
4) Seek supplementary data: extra information like structure analyses or genetic associations can flag higher probability starting points tied to known biology. This aids in prioritizing what to screen first, especially when the quantity of substances is limited.
5) Refine models over time: statistical models predicting success can incrementally improve as more test data accumulates across pipelines.
6) Limit false positives: overly sensitive screenings should be avoided, even if they capture most true hits; generating excessive false leads that consume resources is counterproductive when positives are the priority.
7) Expect imperfection: appreciating prevalence constraints means properly setting expectations around reliability and uncertainty given the state of knowledge.
8) Use methods with higher reproducibility, fidelity, and accuracy. Most cell culture systems and, certainly, computational models are more reproducible than animal experiments. With respect to modeling human responses, at least microphysiological systems (MPS) promise fewer species differences. In general, models which are based on the same mechanisms as in humans promise better fidelity. With respect to accuracy, determining which model is more accurate must be shown case-by-case; however, AI models have already outperformed animal tests for a number of toxicological hazards.
In the end, no solution can avoid the direct implications of probability theory that extremely rare events intrinsically strain the predictive capacity of any analytical approach. However, combining strategic testing with an understanding of these inherent limitations helps maximize the likelihood of teasing out promising needles from the early biomedical haystacks faced during drug discovery.
Pharmaceutical companies conduct a lot of animal testing during drug development to establish safety and efficacy. Animal studies play a critical role in preclinical testing and are used more extensively in pharmaceutical R&D than in most other industries, such as industrial chemicals, consumer products, or food. However, pharmaceutical companies have also pioneered many alternative methods and been early adopters of new technologies to reduce animal use. Some key differences to other industries are as follows:
• Market pressures are different, with enormous upfront investments rewarded by higher prices and profit margins. Pharmaceutical companies face strong incentives to bring drugs to market quickly, so they are motivated to use the most predictive methods, whether animal or alternative. Speed and human relevance are more valued than following traditional protocols.
• R&D spending is massive, estimated at more than $2.6 billion per successful drug development. Pharma devotes tens of billions annually to R&D, giving it resources to implement new technologies. The scale of animal use also makes reduction efforts very cost-relevant.
• There is extensive regulation, but also flexibility. Drug development is heavily regulated to ensure safety, but regulatory agencies allow some discretion in test methods. Pharma takes advantage of opportunities to waive animal tests when alternatives exist.
• The range of tests required is broader. Pharma must assess a wide range of endpoints, from pharmacodynamics to carcinogenicity, which requires a diverse arsenal of animal and non-animal methods.
• Product development cycles are long because development is much more sophisticated. Drug development takes about 12 years on average, so new alternative methods may take time to impact animal use. However, each marketed drug is tested for years, so replacements can eventually have great impacts.
• Focus on mechanism. Understanding drug molecular mechanisms, especially with omics technologies (i.e., simultaneously measuring as many active genes, or proteins and metabolite changes, as possible) informs human biology relevance and helps justify waivers of animal tests.
In summary, pharmaceutical companies are highly motivated to implement improvements in safety testing that can accelerate drug development, improve clinical predictivity, and reduce costs. This has made them forerunners in adopting alternative methods for efficacy testing, despite continuing extensive animal testing requirements for safety.
The philosopher Peter Singer once said, “I don’t think there’s much point in bemoaning the state of the world unless there’s some way you can think of to improve it. Otherwise, don’t bother writing a book; go and find a tropical island and lie in the sun.” So, how can we improve? The main alternatives to animal testing are in vitro and in silico approaches (Figure 12). In vitro methods, while cheaper and faster, face issues like genetic instability and non-physiological culture conditions. However, advances in technology and practices, such as Good Cell Culture Practice (GCCP), are helping to overcome these limitations. In silico methods are now central to life sciences as they have evolved significantly, especially based on AI and also in regulatory contexts. Tools like Good Read-Across Practices and automated read-across, which leverage large toxicological databases, are increasingly used in drug discovery and other applications. Integrated testing strategies (ITS) are emerging which combine in vitro, in silico, and sometimes in vivo methods, recognizing that no single method can fulfill all information needs. This strategy, still in its early stages, is gaining traction in safety sciences with a more mechanistic design approach.
Figure 12. Increasing use of alternatives to animal testing in drug development The figure uses the same simplified work flow as Figure 4, where the new alternative approaches (green) discussed in this article are predominantly used. MPS = microphysiological systems, AI = artificial intelligence. It also makes the point that −50% of new drugs are biologicals, which usually cannot be adequately tested in animals anyway.
The shift towards non-animal methods aligns with a stronger focus on mechanistic research in biochemistry and molecular biology, offering a deeper understanding of physiology and disease. It is challenging to identify disease mechanisms in whole organisms or test specific mechanisms using complex animal models. Systems biology approaches are increasingly modeling this complexity.
Increasingly, mechanistic studies—that is, work elucidating the cellular and molecular aspects of disease and drug action—lead to surrogate measures (“biomarkers”) of drug effects which can then be used in clinical trials to monitor efficacy more subtly and earlier than by clinical outcomes. This is also known as “translational medicine”, which translates from preclinical to clinical work.
In summary, the reliance on animals to study human physiology and diseases is being questioned due to the emergence of alternative methods. These alternatives, although partial and simplistic, offer cheaper, faster, and potentially more robust means of data generation. Combining these methods in ITS or systems biology approaches is helping to overcome the limitations of each method, leading to a decreased reliance on animal testing in the scientific process.
A major challenge in developing new medications is that animal studies often fail to accurately predict whether a drug will be safe and effective in human patients. Animals differ from people in their biology and physiology, so drugs may behave differently in humans than in test animals. Conventional cell cultures also lack key features of real human organs.
To address this problem, scientists have developed innovative “organs on chips” that use microfluidic culture systems to mimic aspects of living human organs and tissues. Tiny channels allow cells to be cultured with flowing fluids that recreate blood flow and breathing motions. Multiple cell types can interact, like blood vessel cells linked to immune cells. Some systems even connect chips of different organs, like gut, liver, and brain.
These “microphysiological systems” (MPS) aim to model human biology more accurately than animal studies or regular cell cultures. Their ultimate goal is to better predict patient responses to drugs before human trials and thus reduce failures. Early studies suggest organ chips could help:
• Model complex human diseases involving multiple organs.
• Identify possible targets for drug action on cellular and molecular levels.
• Identify lead compounds out of a set of candidates.
• Optimize lead compounds by comparative testing of modifications.
• Identify biomarkers of clinical success to be measured later in clinical studies.
• Support an IND (initial drug development) review to move into clinical studies (“first in humans”).
• Detect dangerous side effects missed in animal tests.
• Predict drug absorption, distribution, metabolism, and excretion.
• Test patient-derived cells for personalized medicine.
Challenges remain in validating organ chips and gaining regulatory acceptance. Nevertheless, combined with computer models and small, careful human studies, they could transform drug development to efficiently deliver effective, safe medicines matched to individual patients. While still experimental, the organ chip approach shows promise in providing more reliable human data on drug effects than animal models.
A major focus in the development of new drugs is detecting potential safety issues early, before patients are harmed in clinical trials. However, current safety testing methods often fail to predict all the adverse effects that emerge later. This leads to expensive late-stage drug failures and withdrawals of approved drugs.
To address this problem, the concept of “adverse outcome pathways” (AOPs) is gaining interest. AOPs map the chain of events from initial chemical–cell interactions to subsequent organ responses that ultimately lead to adverse health effects. They organize existing mechanistic knowledge into a sequence of:
• Molecular initiating events—how a chemical first interacts with a biomolecule.
• Key events—cellular, tissue, and organ responses.
• Adverse outcome—the adverse health effect.
AOPs aim to represent established pathways that lead to toxicity. Their development was driven by chemical safety regulations but they are relevant for drug toxicity as well. AOPs could improve drug toxicity prediction by the following:
• Elucidating species differences in toxicity pathways.
• Justifying when animal toxicity findings may not apply to humans.
• Allowing more mechanism-based safety testing methods.
AOPs are strengthened by broader “pathway of toxicity” (PoT) approaches that experimentally map early molecular perturbations using advanced omics technologies. PoTs provide detailed, dynamic networks while AOPs summarize established knowledge. Used together, AOPs and PoTs can enhance mechanistic understanding and modeling of drug safety.
Further efforts are still needed to expand and validate AOPs and PoTs. Nevertheless, mapping adverse outcome pathways promises to ultimately provide a compendium of toxicity mechanism knowledge. This could transform chemical and drug safety assessment to rely less on animal studies and more on human-relevant pathway-based approaches. Understanding toxicity pathways will enable the earlier and more reliable detection of key human hazards, vastly improving the drug development process.
Pharmacology and toxicology have experienced a data revolution, transitioning from a historically small-scale discipline to one generating vast and heterogeneous evidence from high-throughput assays, omics technologies, electronic health records, and more. This exponential growth, coupled with increasing computational power, has created major opportunities for integrating artificial intelligence (AI) techniques to enhance chemical selection and hazard assessment. Early rule-based expert systems have given way to modern machine learning and, especially, deep learning models that find patterns in large datasets to predict toxicity. Notably, these methods are agnostic with respect to what effects are predicted, and similar approaches are available to predict pharmacological effects. Key developments include the following:
• Quantitative structure–activity relationships (QSARs) relating chemical descriptors to bioactivity.
• Public toxicity data repositories like Tox21, enabling AI model development.
• Deep neural networks that integrate chemical and bioassay data to predict diverse hazards.
• Natural language processing, exemplified by the current boom in large language models and mining legacy animal studies and literature.
• Explaining model behavior through explainable AI (xAI) techniques.
AI promises to transform areas like predictive toxicology, drug design, mechanistic understanding, risk assessment, and evidence integration. It can handle multifaceted data and capture uncertainties for robust probabilistic risk modeling. AI-derived knowledge graphs could link to adverse outcome pathways. However, biases, reproducibility, and interpretability remain challenges. AI models require extensive curated training data. Multidisciplinary collaboration is essential for human-centered, trustworthy systems tailored to enhance chemical safety decisions. AI is not a panacea but rather an enabling tool that must be thoughtfully designed and utilized alongside ongoing efforts to improve primary evidence generation and appraisal. It increasingly qualifies as a copilot but is not yet ready to take the pilot’s seat. Overall, the symbiotic integration of AI and modern data-rich toxicology has immense potential to transition the field into a more predictive, mechanistic, and evidence-based scientific discipline to effectively promote human and environmental health.
Toxicity testing has traditionally relied heavily on animal models. However, differences between species mean that animal data do not always accurately predict human responses. There is a growing focus on new approach methodologies (NAMs) to replace or reduce animal use for ethical and scientific reasons, but individual alternative tests are often limited, requiring combination into integrated strategies.
Integrated approaches to testing and assessment (IATA) strategically combine results from multiple NAMs. Sources can include computer models, cell cultures, organ chips, and lower animal species. IATAs also incorporate existing data via weight-of-evidence assessment.
IATAs follow three key steps: 1) compile existing data on a chemical, 2) evaluate data to determine if they are sufficient for decision-making or if new data are needed, and 3) generate new data through targeted testing to fill gaps. IATAs have been developed for skin and eye hazard testing, incorporating animal-free methods like reconstructed human tissue models. Work is ongoing for complex endpoints like cancer and developmental toxicity.
Challenges for IATAs include 1) determining the best test combinations, 2) assessing predictivity, 3) validating integrated approaches, and 4) obtaining regulatory acceptance. IATAs do not necessarily avoid animal tests completely, but they do strategically combine new methods to significantly reduce and refine animal use. An intelligent combination of advanced models with computational approaches offers a path to enhanced predictivity of human outcomes. IATAs represent a pragmatic approach to transition from an animal-centered paradigm to more human-relevant 21st century toxicology. Animal models provide useful but limited data on drug effects in humans. Their flaws lead to many trial failures and to unsafe drugs reaching patients. However, animals remain necessary where better techniques are lacking. Ongoing advances in human cell studies, tissue chips, computer models, and innovative small human trials can make drug development more accurate, ethical, and effective for the diverse spectrum of patients needing safe and beneficial new treatments. The concept of integrated testing strategies originated mainly from the testing challenges for industrial chemicals. However, the concept applies well to the drug discovery process. This includes the combined use of tests with complementary characteristics, with results analyzed together and not sequentially.
The journey of drug development is marked by a paradoxical reliance on preclinical animal research, despite its inherent flaws and limitations. This review has considered the complexities of this relationship, highlighting both the indispensable role and significant challenges posed by animal studies in the realm of therapeutic development.
Flaws and limitations of animal studies, while being a cornerstone of drug development, include physiological differences and misleading outcomes. Even systematic approaches, while improving methodological robustness, cannot fully overcome the inherent limitations of animal models, such as species-specific biological differences. The low translation to clinical use of approximately of 8% within two decades underscores the pitfalls of animal studies. The reproducibility of animal testing, a cornerstone for the reliability of any test, is under significant scrutiny. Biological variation and extreme standardization in trial designs contribute to this crisis, leading to poor reproducibility and questionable accuracy in predicting human efficacy and safety—the reproducibility crisis.
Drug discovery confronts a unique challenge: the rarity of both positive and negative effects sought in drug candidates. The “needle in a haystack” problem is exacerbated by the fact that preclinical models, such as animal studies, do not always accurately predict human responses, making the identification of effective drugs or the detection of toxic effects exceptionally difficult. Drug discovery has been likened to searching for black swan events—rare, impactful, and mostly unpredictable occurrences. The identification of new marketable drugs and the late discovery of significant side effects in widely used drugs are quintessential examples of such events in the pharmaceutical industry.
Several strategies to enhance the probability of identifying successful drug candidates have been discussed here. In summary, while animal research remains a crucial element in therapeutic development, its limitations and flaws necessitate continuous improvement in study design, methodology, and complementary human-relevant systems. The complexity of translating animal data to human applications underlines the need for more predictive models and testing methods that can better capture human biology and disease nuances, including but not limited to microphysiological systems (MPS) and artificial intelligence (AI). As the field evolves, a balanced approach that acknowledges both the value and the limitations of animal studies will be essential in advancing drug development. The new approach methods are already performing, sometimes astonishingly well. Franz Kafka once said, “There is a goal but no way; what we call the way is our hesitation!” This sentiment resonates in the context of animal experiments in drug safety testing, and perhaps we should stop hesitating and just embrace the new opportunities.
TH: writing–original draft and writing–review and editing.
The author declares that no financial support was received for the research, authorship, and/or publication of this article.
The author declares that the research was conducted in the absence of any commercial or financial relationships that could be construed as a potential conflict of interest.
The author declared that they were an editorial board member of Frontiers, at the time of submission. This had no impact on the peer review process and the final decision.
All claims expressed in this article are solely those of the authors and do not necessarily represent those of their affiliated organizations, or those of the publisher, the editors, and the reviewers. Any product that may be evaluated in this article, or claim that may be made by its manufacturer, is not guaranteed or endorsed by the publisher.
Artificial intelligence (AI), computer programs (machine learning tools), which perform tasks, which typically require human intelligence; Attrition refers to the high rate of failure that drug candidates experience during clinical development. Biased outcome reporting, it is easier to publish an effect than no effect: this is a classic example of bias in the scientific literature; Blockbuster, a blockbuster drug is a pharmaceutical product that generates annual sales of $1 billion or more for the company that sells it; Drug target, it is essentially a molecule within the body that a drug interacts with to produce its therapeutic effect; European cosmetics test ban, EU legislation from 2003 bans animal testing, enforced by marketing bans, for finished cosmetic products, for products including ingredients tested on animals where alternatives were accepted (after 2004), for acute and topical (eye and skin) test (after 2009), and all other hazards (after 2013); Generic drugs, a generic drug is a medication that is created to be the same as an already marketed brand-name drug in dosage form, safety, strength, route of administration, quality, performance characteristics, and intended use; Hazard, adverse effect of a substance; High-throughput screening (HTS), a method used in scientific discovery, particularly in drug discovery, biology, materials science, and chemistry. It involves the use of automated equipment to rapidly test thousands to millions of samples for biological activity or chemical reactions; Immunosuppressant drugs, drugs used to hinder transplant rejections or autoimmune diseases; Lead optimization, the process of iteratively synthesizing and testing chemical variants of initial hit compounds from screens to improve potency, selectivity, and drug-like properties, and develop optimized lead molecules as strong final candidates for clinical development; LD50, or lethal dose for 50%, a way to compare the toxic potential of substances through the dose at which 50% of rats die; Nanoparticles, defined as particles of matter with dimensions ranging from 1 to 100 nm (nm) in diameter; they can exhibit significantly different physical and chemical properties compared to their bulk material counterparts due to their high surface area to volume ratio; Omics technologies, simultaneous measurement of as many active genes (transcriptomics), proteins (proteomics), or metabolites (metabolomics) changes as possible; REACH program, acronym for Registration, Evaluation, Authorisation, and Restriction of Chemicals, a comprehensive regulation of the European Union designed to ensure a high level of protection for human health and the environment from the risks posed by chemicals. It was enacted on 1 June 2007; Reproducibility crisis, also known as the replication crisis, refers to the growing concern that many scientific studies’ results are difficult or impossible to reproduce; Selective analysis, aka subgroup analysis, focuses on part of the data, neglecting the overall results, to obtain significant results. This is a common source for irreproducible results; Teratogenic effects, causing birth defects.
Akhtar, A. (2015). The flaws and human harms of animal experimentation. Camb. Q. Healthc. Ethics 24 (4), 407–419. doi:10.1017/S0963180115000079
Allen, A. (2006). Of mice or men: the problems with animal testing. Slate. Available at: https://slate.com/technology/2006/06/does-animal-testing-work.html (Accessed December 11, 2023).
Bailey, J., and Balls, M. (2019). Recent efforts to elucidate the scientific validity of animal-based drug tests by the pharmaceutical industry, pro-testing lobby groups, and animal welfare organisations. BMC Med. Ethics 20, 16. doi:10.1186/s12910-019-0352-3
Baker, M. (2016). 1,500 scientists lift the lid on reproducibility. Nature 533, 452–454. doi:10.1038/533452a
Check Hayden, E., and Hayden, T. (2014). Strike threat over jailed primatologist. Nature 448, 634. doi:10.1038/448634a
Daneshian, M., Busquet, F., Hartung, T., and Leist, M. (2015). Animal use for science in Europe. ALTEX 32, 261–274. doi:10.14573/altex.1509081
DiMasi, J. A., Grabowski, H. G., and Hansen, R. W. (2016). Innovation in the pharmaceutical industry: new estimates of R&D costs. J. Health Econ. 47, 20–33. doi:10.1016/j.jhealeco.2016.01.012
Everitt, J. I. (2015). The future of preclinical animal models in pharmaceutical discovery and development: a need to bring in cerebro to the in vivo discussions. Toxicol. Pathol. 43, 70–77. doi:10.1177/0192623314555162
Fratta, I. D., Sigg, E. B., and Maiorana, K. (1965). Teratogenic effects of thalidomide in rabbits, rats, hamsters, and mice. Toxicol. Appl. Pharmacol. 7, 268–286. doi:10.1016/0041-008X(65)90095-5
Frommlet, F. (2020). Improving reproducibility in animal research. Sci. Rep. 10, 19239. doi:10.1038/s41598-020-76398-3
Frommlet, F., and Heinze, G. (2021). Experimental replications in animal trials. Lab. Anim. 55, 65–75. doi:10.1177/0023677220907617
Harris, R. (2017). Rigor mortis: how sloppy science creates worthless cures, crushes hope, and wastes billions. New York: Basic Books.
Hartung, T. (2007). Food for thought … on cell culture. ALTEX 24, 143–152. doi:10.14573/altex.2007.3.143
Hartung, T. (2009). Per aspirin ad astra. ATLA - Altern. Lab. Anim. 37 (Suppl. 2), 45–47. doi:10.1177/026119290903702S10
Hartung, T. (2013). Look Back in anger – what clinical studies tell us about preclinical work. ALTEX 30, 275–291. doi:10.14573/altex.2013.3.275
Hartung, T. (2016). Making big sense from big data in toxicology by read-across. ALTEX 33, 83–93. doi:10.14573/altex.1603091
Hartung, T. (2023). ToxAIcology - the evolving role of artificial intelligence in advancing toxicology and modernizing regulatory science. ALTEX 40, 559–570. doi:10.14573/altex.2309191
Hooijmans, C. R., de Vries, R. B. M., Ritskes-Hoitinga, M., Rovers, M. M., Leeflang, M. M., IntHout, J., et al. (2018). Facilitating healthcare decisions by assessing the certainty in the evidence from preclinical animal studies. PLoS ONE 13, e0187271. doi:10.1371/journal.pone.0187271
Hooijmans, C. R., Rovers, M. M., de Vries, R. B., Leenaars, M., Ritskes-Hoitinga, M., and Langendam, M. W. (2014). SYRCLE's risk of bias tool for animal studies. BMC Med. Res. Methodol. 14, 43. doi:10.1186/1471-2288-14-43
Leist, M., and Hartung, T. (2013). Inflammatory findings on species extrapolations: humans are definitely no 70-kg mice. Arch. Toxicol. 87, 563–567. doi:10.1007/s00204-013-1038-0
Meigs, L., Smirnova, L., Rovida, C., Leist, M., and Hartung, T. (2018). Animal testing and its alternatives – the most important omics is economics. ALTEX 35, 275–305. doi:10.14573/altex.1807041
Nuffield Council on Bioethics (2005). The ethics of research involving animals. Available at: https://www.nuffieldbioethics.org/wp-content/uploads/The-ethics-of-research-involving-animals-full-report.pdf (last accessed December 12, 2023).
Pound, P. (2023). Rat trap – breaking free from the illusion of progress in animal research, 304. Matador.
Pound, P., and Bracken, M. B. (2014). Is animal research sufficiently evidence based to be a cornerstone of biomedical research? Brit. Med. J. 348, g3387. doi:10.1136/bmj.g3387
Pound, P., and Ritskes-Hoitinga, M. (2018). Is it possible to overcome issues of external validity in preclinical animal research? Why most animal models are bound to fail. J. Transl. Med. 16, 304. doi:10.1186/s12967-018-1678-1
Richter, S. H., Garner, J. P., and Würbel, H. (2009). Environmental standardization: cure or cause of poor reproducibility in animal experiments? Nat. Methods. 6, 257–261. doi:10.1038/nmeth.1312
Ritskes-Hoitinga, M., and Pound, P. (2022). The role of systematic reviews in identifying the limitations of preclinical animal research, 2000 – 2022. JLL Bulletin: commentaries on the history of treatment evaluation. Available at: https://www.jameslindlibrary.org/articles/the-role-of-systematic-reviews-in-identifying-the-limitations-of-preclinical-animal-research-2000-2022/ (Accessed December 12, 2023).
Skrabanek, P., and McCormick, J. (1989). Follies and fallacies in medicine. Glasgow: Tarragon Press. Available at: https://chagall.med.cornell.edu/Skrabanek/Follies-and-Fallacies-in-Medicine.pdf.
Spanagel, R. (2022). Ten points to improve reproducibility and translation of animal research. Front. Behav. Neurosci. 16, 869511. doi:10.3389/fnbeh.2022.869511
Van Norman, G. A. (2019). Limitations of animal studies for predicting toxicity in clinical trials: is it time to rethink our current approach? JACC Basic Transl. Sci. 4, 845–854. doi:10.1016/j.jacbts.2019.10.008
Van Norman, G. A. (2020). Limitations of animal studies for predicting toxicity in clinical trials: Part 2: potential alternatives to the use of animals in preclinical trials. JACC Basic Transl. Sci. 5, 387–397. doi:10.1016/j.jacbts.2020.03.010
Vargesson, N. (2015). Thalidomide-induced teratogenesis: history and mechanisms. Birth Defects Res. C Embryo Today 105, 140–156. doi:10.1002/bdrc.21096
Voelkl, B., Altman, N. S., Forsman, A., Forstmeier, W., Gurevitch, J., Jaric, I., et al. (2020). Reproducibility of animal research in light of biological variation. Nat. Rev. Neurosci. 21, 384–393. doi:10.1038/s41583-020-0313-3
Keywords: animal models, drug development, preclinical research, clinical trials, predictive methods, alternatives to animal testing
Citation: Hartung T (2024) The (misleading) role of animal models in drug development. Front. Drug Discov. 4:1355044. doi: 10.3389/fddsv.2024.1355044
Received: 13 December 2023; Accepted: 27 February 2024;
Published: 08 April 2024.
Edited by:
Bruno Villoutreix, Hôpital Robert Debré, FranceReviewed by:
Olivier Taboureau, Université Paris Cité, FranceCopyright © 2024 Hartung. This is an open-access article distributed under the terms of the Creative Commons Attribution License (CC BY). The use, distribution or reproduction in other forums is permitted, provided the original author(s) and the copyright owner(s) are credited and that the original publication in this journal is cited, in accordance with accepted academic practice. No use, distribution or reproduction is permitted which does not comply with these terms.
*Correspondence: Thomas Hartung, dGhhcnR1bmdAamhzcGguZWR1
Disclaimer: All claims expressed in this article are solely those of the authors and do not necessarily represent those of their affiliated organizations, or those of the publisher, the editors and the reviewers. Any product that may be evaluated in this article or claim that may be made by its manufacturer is not guaranteed or endorsed by the publisher.
Research integrity at Frontiers
Learn more about the work of our research integrity team to safeguard the quality of each article we publish.