- Departamento de Farmacia, Facultad de Química, Universidad Nacional Autónoma de México, Mexico City, Mexico
Human epigenetic enzyme disruptor of telomeric silencing 1-like (DOT1L) is a key drug target for treating acute myeloid leukemia. Several nucleoside and non-nucleoside DOT1L inhibitors have been developed to inhibit its histone methyltransferase activity. Non-mechanism-based nucleoside DOT1L inhibitors have shown good inhibitory activity and high on-target residence times. Previous computational studies have explored the dynamic behavior of this group of molecules on DOT1L to design compounds with enhanced binding affinities. Nevertheless, it is well known that drug-target kinetics also plays a crucial role in the discovery of new drugs. Therefore, we performed τ-Random Acceleration Molecular Dynamics (τRAMD) simulations to estimate the residence times of nucleoside DOT1L inhibitors. The high correlation between the calculated and experimental residence times suggested that the method can reliably estimate the residence time of nucleoside DOT1L inhibitors when modifications are made to those substituents that occupy the buried hydrophobic pocket of the active site, exhibit hydrophobic interactions with F245 or that form H-bonds with D161 and G163. Overall, this study will be a step toward understanding the binding kinetics of nucleoside DOT1L inhibitors for the treatment of acute myeloid leukemia.
1 Introduction
Disruptor of telomeric silencing 1-like (DOT1L) is a non-SET domain nucleosomal histone methyltransferase (HMTase) involved in the specific methylation of histone H3 at lysine 79 (H3K79) (Feng et al., 2002). The human DOT1L is a 1,537 amino acids epigenetic enzyme, comprising an HMTase catalytic N-terminal domain (a. a. 1–332, Figure 1A) and a long-disordered C-terminal segment essential for binding to nucleosomes and DNA (a. a. 333–1,537) (Min et al., 2003). Several studies have demonstrated that the DOT1L C-terminal domain also interacts with mixed lineage leukemia (MLL) fusion partners, such as ENL, AF4, AF9, and AF10 (Mueller et al., 2007; Kuntimaddi et al., 2015; Okuda et al., 2017; Song et al., 2019). Overexpression of different MLL target genes due to the recruitment of DOT1L by these fusion partners has been associated to the development of acute myeloid leukemia (AML) (Hosseini and Minucci, 2018). For this reason, DOT1L has emerged as a key drug target for the treatment of AML.
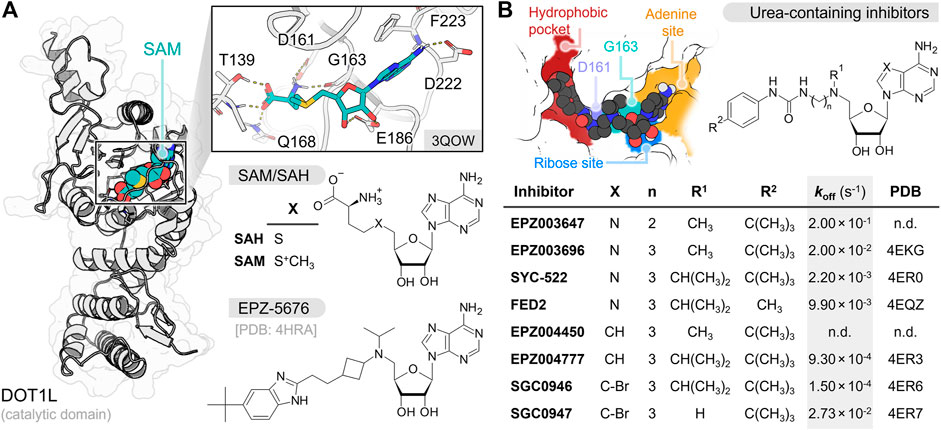
FIGURE 1. (A) Depiction of SAM interaction in DOT1L N-terminal catalytic domain. SAM, SAH and EPZ-5676 chemical structures are shown at the bottom (B) Urea-containing inhibitors chemical structures with dissociation rate constant (koff) values and PDB identification codes (n.d. not determined) (Basavapathruni et al., 2012; Yu et al., 2012; Daigle et al., 2013). The figure at the top left cornner displays the key interaction sites of this group of inhibitors at the DOT1L catalytic pocket.
To date, several nucleoside DOT1L inhibitors have been synthetized and tested against MLL-rearranged leukemia models (Anglin and Song, 2013). These inhibitors share an adenosine moiety that facilitates their recognition by the S-adenosyl-L-methionine (SAM) cofactor pocket in the DOT1L catalytic domain. Nucleoside inhibitors inactivate DOT1L through mechanism- or non-mechanism-based inhibition processes. SAH, the demethylated product of SAM, is a potent non-mechanism-based nucleoside DOT1L inhibitor (Ki = 160–320 nM, KD = 71 nM) (Anglin et al., 2012; Basavapathruni et al., 2012). Unfortunately, this molecule also inhibits DNA methyltransferases and other HMTases (Zhang and Zheng, 2016; Zhang et al., 2021; Talukdar et al., 2022). To increase selectivity towards DOT1L, several modifications have been made to SAH structure (Figure 1B; Supplementary Table S1) (Anglin et al., 2012; Yu et al., 2013; Spurr et al., 2016). In previous works, the amino acid fragment of SAH has been replaced by carbamate, urea, and benzimidazole groups to keep the hydrogen bond (H-bond) interaction with D161, and a bulky substituent has been added at their distal end to interact with the buried hydrophobic pocket (Yi and Ge, 2022). Moreover, the sulfide group has been replaced by a tertiary amine to mimic the methyl-sulfonium substituent of SAM and display an H-bond interaction with G163 main-chain carbonyl. From the latter, EPZ003647 (KD = 167 nM), EPZ003696 (KD = 1.70 nM), SYC-522/FED1 (KD = 1.99 nM), FED2 (KD = 26.9 nM), and EPZ-5676/pinometostat (Ki = 0.08 nM) exhibited the best inhibitory results (Anglin et al., 2012; Basavapathruni et al., 2012; Yu et al., 2012; Daigle et al., 2013). Other research groups have further replaced the adenosine moiety by a 7-deazaadenosine, leading to compounds EPZ004450 (Ki = 4.0 nM), EPZ004777 (KD = 0.253 nM), SGC0946 (KD = 0.056 nM), and SGC0947 (KD = 3.35 nM) (Basavapathruni et al., 2012; Yu et al., 2012). Overall, urea/benzimidazole-containing inhibitors displayed high selectivity for DOT1L over other HMTases and potent antileukemic activity (Daigle et al., 2011; Perner et al., 2020).
In addition to their high affinity and selectivity for DOT1L, surface plasmon resonance (SPR) studies have shown that urea-containing inhibitors also exhibit long on-target residence times (τ = 1/koff) on this epigenetic protein (Basavapathruni et al., 2012; Yu et al., 2012). Current computer-aided drug design protocols seeking novel DOT1L inhibitors have focus on the “interaction strength” (binding affinity) between the ligand and DOT1L (Raj et al., 2015; Luo et al., 2016). Nevertheless, drug-target kinetics plays a key role in the drug discovery paradigm (Tonge, 2018). Therefore, we carried out τ-Random Acceleration Molecular Dynamics (τRAMD) (Kokh et al., 2018) simulations to estimate the τ of urea-containing inhibitors and compare our predictions with reported binding kinetics experimental data. The conventional simulations performed to generate the starting replicas of the τRAMD dissociation trajectories were also analyzed to study the interaction profile of the inhibitors at the DOT1L catalytic site. This study will be useful to estimate the binding kinetics of novel DOT1L inhibitors for treating AML.
2 Models and methods
2.1 Systems setup
DOT1L prepared models complexed with SAH, EPZ003696, SYC-522, FED2, EPZ004777, SGC0946, and SGC0947 were obtained from the recent Quantitative Structure−Kinetics Relationship (QSKR) study carried out by Li and coworkers (Liu et al., 2022). EPZ003647 was docked with LeDock (Wang et al., 2016) in the 4EKI prepared model using a 30 × 30 × 30 Å3 grid box size centered in G163. The N-isopropyl substituent of EPZ004777 was replaced to N-methyl in the prepared system to generate the DOT1L-EPZ004450 complex. For EPZ-5676, we retrieved the crystal structure coordinates of 4HRA entry from the Protein Data Bank (PDB) (Berman et al., 2000). Missing loops and side chain residues were completed using Modeller 10.3 (Webb and Sali, 2016) with the model/refine loops module of Chimera UCSF v1.15 (Pettersen et al., 2004). Water molecules and external ligands were removed, alternate positions of the side-chain residues were fixed, and hydrogen atoms were added using the Dock Prep tool.
Additionally, non-nucleoside DOT1L inhibitors CHEMBL4534250 and CHEMBL4590355 were docked into the active site of PDB IDs 5DSX and 5DT2 (Chen et al., 2016), respectively, using the AutoDock 4.2 (Morris et al., 2009) module implemented in AMDock (Valdés-Tresanco et al., 2020). Both PDB structures were previously prepared with Chimera UCSF v1.15 as described above, while the ligands were constructed and protonated using Avogadro (Hanwell et al., 2012) and submitted to an energy minimization with the PM6 method employing Gaussian 16 (Frisch et al., 2016). A total of 100 runs and 10 × 106 evaluations using the Lamarckian genetic algorithm were performed with a 30 × 30 × 30 Å3 grid box size centered in the DOT1L active site.
We employed 4EKI, 5DSX, and 5DT2 for the molecular docking of EPZ003647, CHEMBL4534250, and CHEMBL4590355, respectively, since the inhibitors co-crystallized in those DOT1L structures share a high structural similarity with these compounds (see Supplementary Figure S1). Moreover, three different programs, AutoDock 4.2, LeDock, and AutoDock Vina 1.2 (Eberhardt et al., 2021), were employed to dock these nucleoside inhibitors into the DOT1L active site. The docking algorithms for each inhibitor type were finally selected based on the binding mode similarity to the co-crystal compounds. A previous comprehensive docking benchmark demonstrated that no single docking program offers dominant advantages over another, and a combination of these tools should be tested to select the most appropriate approach for a particular project (Wang et al., 2016).
2.2 Molecular dynamics simulations
Each DOT1L-inhibitor complex was submitted to three independent 100 ns unrestrained molecular dynamics (MD) simulations (total time of the 12 systems = 3.9 μs) using the AMBER 14SB force field (Maier et al., 2015) implemented for GROMACS 2020 (Abraham et al., 2015). The ligand topologies were prepared using ACPYPE (Sousa da Silva and Vranken, 2012), assigning the atoms partial charges with the AM1-BCC method. The prepared DOT1L-inhibitor complexes were solvated in a periodic cubic box employing the TIP3P model with a minimum distance of 1.0 nm between the protein and the edges of the box. Sodium and chlorine ions were randomly added to neutralize the system charge and reach a 0.15 M concentration. Each system was energy minimized and equilibrated for 1.0 ns under canonical (NVT) and isothermal-isobaric (NPT) ensembles. The temperature was fixed to 300 K with the V-rescale (Bussi et al., 2007) coupling thermostat, while the pressure was set at 1.0 bar using the Parrinello–Rahman barostat (Parrinello and Rahman, 1982) algorithm. All bonds involving hydrogens were constrained using the Linear Constraint Solver (LINCS) algorithm. Full electrostatic interactions and Lennard-Jones potential cut-off radius were set to 1.2 nm, and the Particle Mesh Ewald (PME) approach was used to approximate the infinite periodic Coulomb sum. The final MD trajectories were analyzed using GROMACS built-in tools. Hydrophobic interactions were computed with the standalone version of Protein-Ligand Interaction Profiler (PLIP) (Adasme et al., 2021). PyMOL v2.5.4 (Schrödinger, 2022) and Gnuplot v5.2 (Williams et al., 2017) were used to generate the figures and plots, respectively.
2.3 τ-random acceleration molecular dynamics
The atomic coordinates and velocities from the last snapshots of the three independent 100 ns MD simulations performed for each DOT1L-inhibitor complex were employed as starting replicas to carry out the τRAMD simulations (Kokh et al., 2018). Each starting replica was submitted to 20 τRAMD dissociation trajectories (total = 720 τRAMD trajectories) by applying a force with magnitude of 879 and 586 kJ mol−1 nm−1 to the ligand center of mass (LIGcom) of nucleoside and non-nucleoside inhibitors, respectively. Random directions of the force were retained (LIGcom moved at least 0.0025 nm) or fixed (LIGcom moved less than 0.0025 nm) after 50 steps (100 fs). Dissociation simulations were stopped when reaching a maximum distance of 5.0 nm of the LIGcom. Finally, we computed the relative τ from the 20 starting replicas of the three extracted snapshots for each complex using a time distribution analysis. The logarithmic value of both calculated and experimental residence times were computed for each system and normalized using the following min-max normalization algorithm:
3 Results
The root-mean-square deviation (RMSD) and fluctuation (RMSF) analysis of the three independent 100 ns MD replicas performed for each system are reported in the Supplementary Material, as well as the statistical analysis of the τRAMD dissociation trajectories employed to compute the τcalc (ps) (see Supplementary Table S2; Supplementary Figures S2–S5). The RMSF analysis highlighted the low mobility of binding site residues (a. a. 140–245) due to their intermolecular interaction with the bounded compounds, while the RMSD of the ligands confirmed the high stability of the DOT1L inhibitors at the active site during the simulation time. Table 1 shows the experimental [τexp (s)] and τRAMD calculated [τcalc (ps)] on-target residence times for the nucleoside DOT1L inhibitors. As in other MD simulation studies to determine on-target residence times (Mollica et al., 2015; Schuetz et al., 2019), the τ values were normalized to compare the estimated residence times with the experimental data. The inhibitors-dissociation pathway and normalized log(τ) values of τcalc and τexp plotted against each other are shown in Figure 2. The high coefficient of determination (R2 = 0.87) demonstrated that τcalc computed with the τRAMD method could reliably predict the experimental residence times.
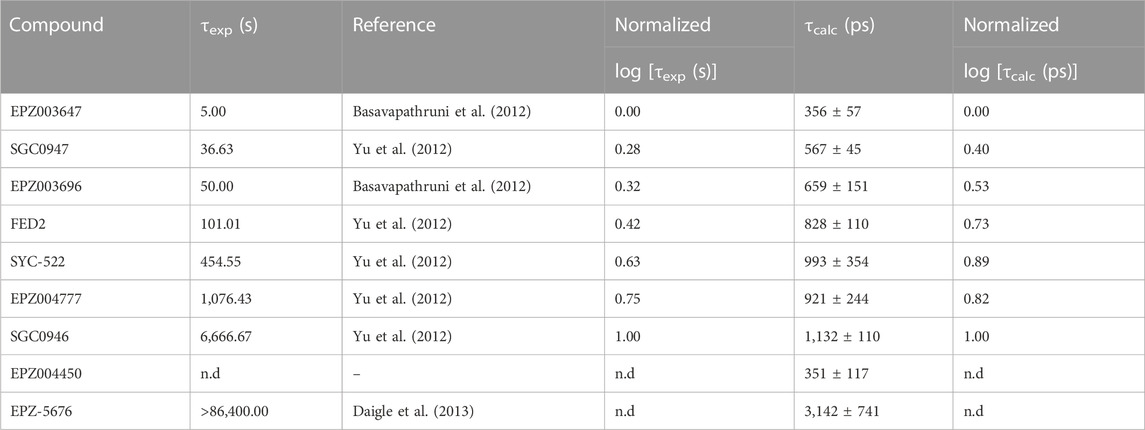
TABLE 1. Experimental (τexp) and τRAMD calculated (τcalc) on-target residence times of nucleoside DOT1L inhibitors.
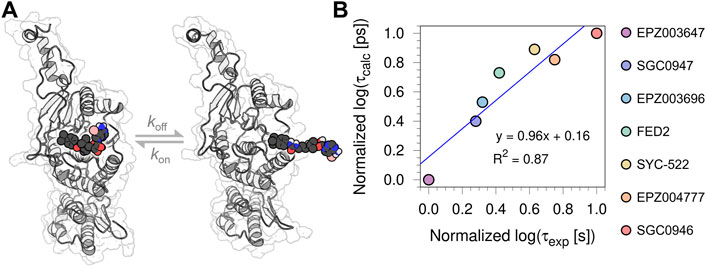
FIGURE 2. (A) Depiction of the inhibitors-dissociation pathway in DOT1L (B) Normalized log [τcalc (ps)] plotted against normalized log [τexp (s)] of the seven urea-containing inhibitors.
In order to understand the role of the protein-ligand interaction profiles in the on-target residence times, we calculated the interaction fractions (IF) of the H-bond (HB) and hydrophobic (HI) contacts formed between DOT1L and the nucleoside inhibitors from the last 50 ns of the three independent replicas for each simulated complex. Noteworthy, no significant salt bridge or π-stacking interactions were identified during the simulations of the nine nucleoside DOT1L inhibitors. Heat maps in Figure 3 show the IF values of each intermolecular interaction formed during the analyzed simulation time. The structure depicts the DOT1L active site residues and inhibitor substituents involved in the protein-ligand interaction profile. Dark colors in the heatmaps highlight the regions with the highest frequency protein-ligand interactions, while lighter colors indicate the less frequent interaction pairs.
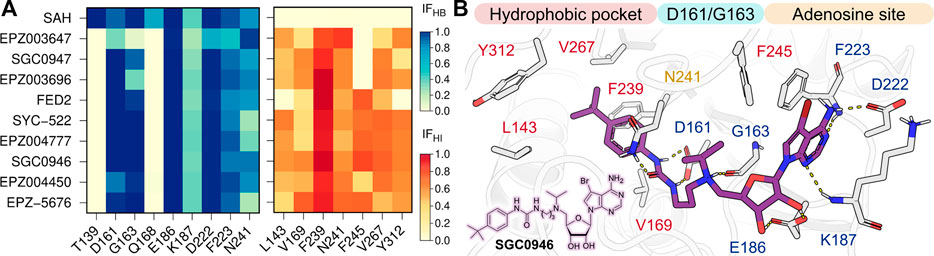
FIGURE 3. (A) H-bond (HB, blue) and hydrophobic (HI, red) interaction fractions (IF) of the ten nucleoside inhibitors with DOT1L active site residues. IF were computed for the last 50 ns of the three independent MD simulations (B) Depiction of SGC0946 interaction with DOT1L. H-bonds are showed as dotted yellow lines.
Contrary to SAH interaction profile, nucleoside DOT1L inhibitors did not display HB interactions with T139 and Q168. EPZ003647, SGC0947, and EPZ003696 exhibited a lower number of HBs with key residues D161 and G163 when compared to other compounds. Furthermore, these three compounds did not exhibit hydrophobic interactions with F245 due to their small amine substituent. Interestingly, these mismatches in the H-bond and hydrophobic interactions explain the low residence time predicted for EPZ004450 and a high residence time estimated for EPZ-5676.
We also computed the residence times of non-nucleoside DOT1L inhibitors CHEMBL4534250 (τcalc = 493 ± 70 ps) and CHEMBL4590355 (τcalc = 416 ± 47 ps) using a lower force magnitude during the τRAMD simulations. However, the predicted residence times do not correlate with the experimental information since a higher τ value was expected for CHEMBL4590355 (τexp > 14,400 s) than for CHEMBL4534250 (τexp = 2,580 s) (Chen et al., 2016).
4 Discussion
The binding affinity of a compound towards a target is a widely used parameter employed in drug design to determine the “strength” of the target-ligand complex. Nevertheless, the time it takes for the complex to dissociate (binding kinetics) also plays a key role during the drug discovery workflow. Herein, we performed τRAMD simulations (Kokh et al., 2018) to estimate the on-target residence time (τcalc) of nucleoside DOT1L urea-containing inhibitors. Data normalization was performed to compare the computed τRAMD residence time with previously reported experimental information. A similar data treatment has been carried out in previous studies (Mollica et al., 2015; Schuetz et al., 2019). The high correlation (R2 = 0.87) demonstrated that the method reliably predicts nucleoside DOT1L inhibitors’ residence time compared to reported experimental data (τexp). It is worth mentioning that an increase in ionizable groups overestimates the residence time of compounds, which is why the estimated residence time (τcalc = 1,322 ± 355 ps) and experimental data (τexp = 10 s) (Basavapathruni et al., 2012) of SAH were not included during the analysis.
The high similarity between the τcalc values for SYC-522, EPZ004777, and SGC0946 suggests that the approach is poorly sensitive to changes made to the adenosine moiety. However, significant differences were observed in those ligands with modifications in the amino group and in the bulky substituent that occupies the hydrophobic pocket. For the first, the interaction profile analysis showed that the absence of the isopropyl substituent on the amine leads to the loss of a crucial hydrophobic interaction with F245 residue. Our results also suggest that this intermolecular interaction acts as an anchor that allows the ligand to keep H-bond interactions with D161 and G163 and increase its residence time at the catalytic site. As can be seen in the interaction fraction heatmaps (see Figure 3A), the absence of the isopropyl group also appears to substantially reduce the number of H-bonds between the protonated amine and the G163 backbone. In particular, the reduction of the aliphatic linker between the amine and the urea (EPZ003647) leads to the loss of H-bond interactions with both G163 and D161 since the substituent could not reorient and form both interactions at the same time. Although this behavior could also be attributed to a possible artifact of the molecular docking, we compared our DOT1L-EPZ003647 complex with that reported by Li and coworkers, in which the H-bond interaction with G163 was not initially displayed. Interestingly, the three independent MD simulations and τRAMD dissociation trajectories with this complex also showed a low fraction of HBs with D161 (IF = 0.33) and G163 (IF = 0.03) and a low on-target residence time of this compound (τcalc = 140 ± 20 ps) (see Supplementary Figure S6). Therefore, this result confirms that low residence time of EPZ003647 could be due to the loss of these key H-bond interactions. On the other hand, 4-tert-butylphenyl replacement by 4-methylphenyl in FED2 resulted in the loss of interactions with L143 and Y312 residues comprising the hydrophobic pocket. As with the amine substitution, the loss of these interactions with the hydrophobic cavity could explain the low residence time for FED2.
It is well known that the equilibrium dissociation constant (KD = koff/kon) and the residence time (τ = 1/koff) are not always inversely correlated due to the variance of the association rate constant (kon) (Copeland, 2016; Tonge, 2018). Several studies have demonstrated that compounds with similar binding affinities to a common drug target can exhibit vastly different residence times (Copeland, 2021). Nevertheless, Copeland (Copeland, 2016) found a high correlation between the KD and koff of the nucleoside DOT1L inhibitors reported by (Basavapathruni et al., 2012). The latter was possible because the kon values between the different inhibitors are practically invariant. Herein, we plotted the normalized log [τexp (s)] and log [τcalc (ps)] values against the normalized negative logarithm of the experimental KD [pKD = –log KD (M)] (see Supplementary Figure S7). Our analysis showed that the τexp has a strong correlation with KD (R2 = 0.80), while a lower correlation was obtained with the τcalc values (R2 = 0.62). The decrease in the coefficient of determination between KD and τ could be related to the association rate constant of FED2 (kon = 3.68 × 105 M−1 s−1), since this value is greater than those reported for the other nucleoside inhibitors (kon = 1.20 × 107 to 8.37 × 106 M−1 s−1) (Basavapathruni et al., 2012; Yu et al., 2012). Notwithstanding the foregoing, the high correlation between these data suggests that the lack of intermolecular interactions observed for inhibitors with short τ values could also be able to explain their low binding affinities to DOT1L. Therefore, the preservation of H-bond interactions with D161 and G163, as well as the contacts with the hydrophobic pocket and F245, are key factors to design nucleoside DOT1L inhibitors with desirable binding affinities and on-target residence times.
Although EPZ004450 predicted residence time was not included during the correlation analysis due to the absence of experimental data, the interaction profile and residence time of this compound resembles those estimated for ligands that do not have an isopropylamine group (i.e., EPZ003647, SGC0947, and EPZ003696). On the other hand, the interaction and residence time (τcalc = 3,142 ± 741 ps) predictions of EPZ-55676 are very similar to those observed with SYC-522, EPZ004777, and SGC0946, which agrees with its high residence time on DOT1L determined by SPR (τexp > 86,400.00 s) (Daigle et al., 2013). Therefore, it is very likely that the method can reliably estimate the residence time of this type of DOT1L inhibitors. However, this estimation might not be carried out with non-nucleoside inhibitors since the τcalc values obtained for CHEMBL4534250 and CHEMBL4590355 do not correlate with experimental observations. In this case, both compounds exhibit a very similar interaction profile, changing only the frequency of a few hydrophobic interactions (see Supplementary Figure S8). Unlike the nucleoside inhibitors, the difference in the interaction profile does not allow us to explain the residence time estimations. Therefore, the method might not be useful for predicting the on-target residence times of non-nucleoside inhibitors. Nevertheless, more experimental determinations with non-nucleoside inhibitors will be necessary to support this conclusion.
Data availability statement
The original contributions presented in the study are included in the article/Supplementary Material, further inquiries can be directed to the corresponding author.
Author contributions
All authors prepared the initial protein-ligand complex systems, performed the conventional and τRAMD simulations, and analyzed the data. RA-O wrote the first version of the manuscript. CF-L, LC-P, and MS-C reviewed and edited the manuscript.
Funding
This study was sponsored by Programa de Apoyo a la Investigación y el Posgrado (PAIP 5000-9197).
Acknowledgments
RA-O thanks the Dirección General de Cómputo y Tecnologías de Información y Comunicación (DGTIC) for the support received in the use of the HP Cluster Platform 3000SL supercomputer “Miztli” (LANCAD-UNAM-DGTIC-398).
Conflict of interest
The authors declare that the research was conducted in the absence of any commercial or financial relationships that could be construed as a potential conflict of interest.
Publisher’s note
All claims expressed in this article are solely those of the authors and do not necessarily represent those of their affiliated organizations, or those of the publisher, the editors and the reviewers. Any product that may be evaluated in this article, or claim that may be made by its manufacturer, is not guaranteed or endorsed by the publisher.
Supplementary material
The Supplementary Material for this article can be found online at: https://www.frontiersin.org/articles/10.3389/fddsv.2022.1083198/full#supplementary-material
References
Abraham, M. J., Murtola, T., Schulz, R., Páall, S., Smith, J. C., Hess, B., et al. (2015). Gromacs: High performance molecular simulations through multi-level parallelism from laptops to supercomputers. SoftwareX 1 (2), 19–25. doi:10.1016/j.softx.2015.06.001
Adasme, M. F., Linnemann, K. L., Bolz, S. N., Kaiser, F., Salentin, S., Haupt, V. J., et al. (2021). Plip 2021: Expanding the scope of the protein–ligand interaction profiler to DNA and RNA. Nucleic Acids Res. 49, W530–W534. doi:10.1093/nar/gkab294
Anglin, J. L., Deng, L., Yao, Y., Cai, G., Liu, Z., Jiang, H., et al. (2012). Synthesis and structure–activity relationship investigation of adenosine-containing inhibitors of histone methyltransferase DOT1L. J. Med. Chem. 55, 8066–8074. doi:10.1021/jm300917h
Anglin, J. L., and Song, Y. (2013). A medicinal Chemistry perspective for targeting histone H3 lysine-79 methyltransferase DOT1L. J. Med. Chem. 56, 8972–8983. doi:10.1021/jm4007752
Basavapathruni, A., Jin, L., Daigle, S. R., Majer, C. R. A., Therkelsen, C. A., Wigle, T. J., et al. (2012). Conformational adaptation drives potent, selective and durable inhibition of the human protein methyltransferase DOT1L. Chem. Biol. Drug Des. 80, 971–980. doi:10.1111/cbdd.12050
Berman, H. M., Wstbrook, J., Feng, Z., Gilliland, G., Bhat, T. N., Weissing, H., et al. (2000). The protein Data Bank. Nucleic Acids Res. 28, 235–242. doi:10.1093/nar/28.1.235
Bussi, G., Donadio, D., and Parrinello, M. (2007). Canonical sampling through velocity rescaling. J. Chem. Phys. 126, 014101–014107. doi:10.1063/1.2408420
Chen, C., Zhu, H., Stauffer, F., Caravatti, G., Vollmer, S., Machauer, R., et al. (2016). Discovery of novel Dot1L inhibitors through a structure-based fragmentation approach. ACS Med. Chem. Lett. 7, 735–740. doi:10.1021/acsmedchemlett.6b00167
Copeland, R. A. (2021). Evolution of the drug-target residence time model. Expert Opin. Drug Discov. 16, 1441–1451. doi:10.1080/17460441.2021.1948997
Copeland, R. A. (2016). The drug–target residence time model: A 10-year retrospective. Nat. Rev. Drug Discov. 15, 87–95. doi:10.1038/nrd.2015.18
Daigle, S. R., Olhava, E. J., Therkelsen, C. A., Basavapathruni, A., Jin, L., Boriack-Sjodin, P. A., et al. (2013). Potent inhibition of DOT1L as treatment of MLL-fusion leukemia. Blood 122, 1017–1025. doi:10.1182/blood-2013-04-497644
Daigle, S. R., Olhava, E. J., Therkelsen, C. A., Majer, C. R., Sneeringer, C. J., Song, J., et al. (2011). Selective killing of mixed lineage leukemia cells by a potent small-molecule DOT1L inhibitor. Cancer Cell 20, 53–65. doi:10.1016/j.ccr.2011.06.009
Eberhardt, J., Santos-Martins, D., Tillack, A. F., and Forli, S. (2021). AutoDock Vina 1.2.0: New docking methods, expanded force field, and Python bindings. J. Chem. Inf. Model. 61, 3891–3898. doi:10.1021/acs.jcim.1c00203
Feng, Q., Wang, H., Ng, H. H., Erdjument-Bromage, H., Tempst, P., Struhl, K., et al. (2002). Methylation of H3-lysine 79 is mediated by a new family of HMTases without a SET domain. Curr. Biol. 12, 1052–1058. doi:10.1016/S0960-9822(02)00901-6
Frisch, M. J., Trucks, G. W., Schlegel, H. B., Scuseria, G. E., Robb, M. A., CheesemanScalmani, J. R. G., et al. (2016). Gaussian 16, revision C.01. Wallingford CT: Gaussian, Inc.
Hanwell, M. D., Curtis, D. E., Lonie, D. C., Vandermeersch, T., Zurek, E., and Hutchison, G. R. (2012). Avogadro: An advanced semantic chemical editor, visualization, and analysis platform. J. Cheminform. 4, 17. doi:10.1186/1758-2946-4-17
Hosseini, A., and Minucci, S. (2018). “Alterations of histone modifications in cancer,” in Epigenetics in human disease (Elsevier), 141–217. doi:10.1016/B978-0-12-812215-0.00006-6
Kokh, D. B., Amaral, M., Bomke, J., Grädler, U., Musil, D., Buchstaller, H.-P., et al. (2018). Estimation of drug-target residence times by τ-random acceleration molecular dynamics simulations. J. Chem. Theory Comput. 14, 3859–3869. doi:10.1021/acs.jctc.8b00230
Kuntimaddi, A., Achille, N. J., Thorpe, J., Lokken, A. A., Singh, R., Hemenway, C. S., et al. (2015). Degree of recruitment of DOT1L to MLL-AF9 defines level of H3K79 di- and tri-methylation on target genes and transformation potential. Cell Rep. 11, 808–820. doi:10.1016/j.celrep.2015.04.004
Liu, H., Su, M., Lin, H.-X., Wang, R., and Li, Y. (2022). Public data set of protein–ligand dissociation kinetic constants for quantitative structure–kinetics relationship studies. ACS Omega 7, 18985–18996. doi:10.1021/acsomega.2c02156
Luo, M., Wang, H., Zou, Y., Zhang, S., Xiao, J., Jiang, G., et al. (2016). Identification of phenoxyacetamide derivatives as novel DOT1L inhibitors via docking screening and molecular dynamics simulation. J. Mol. Graph. Model. 68, 128–139. doi:10.1016/j.jmgm.2016.06.011
Maier, J. A., Martinez, C., Kasavajhala, K., Wickstrom, L., Hauser, K. E., and Simmerling, C. (2015). ff14SB: Improving the accuracy of protein side chain and backbone parameters from ff99SB. J. Chem. Theory Comput. 11, 3696–3713. doi:10.1021/acs.jctc.5b00255
Min, J., Feng, Q., Li, Z., Zhang, Y., and Xu, R.-M. (2003). Structure of the catalytic domain of human DOT1L, a non-SET domain nucleosomal histone methyltransferase. Cell 112, 711–723. doi:10.1016/S0092-8674(03)00114-4
Mollica, L., Decherchi, S., Zia, S. R., Gaspari, R., Cavalli, A., and Rocchia, W. (2015). Kinetics of protein-ligand unbinding via smoothed potential molecular dynamics simulations. Sci. Rep. 5, 11539. doi:10.1038/srep11539
Morris, G. M., Huey, R., Lindstrom, W., Sanner, M. F., Belew, R. K., Goodsell, D. S., et al. (2009). AutoDock4 and AutoDockTools4: Automated docking with selective receptor flexibility. J. Comput. Chem. 30, 2785–2791. doi:10.1002/jcc.21256
Mueller, D., Bach, C., Zeisig, D., Garcia-Cuellar, M.-P., Monroe, S., Sreekumar, A., et al. (2007). A role for the MLL fusion partner ENL in transcriptional elongation and chromatin modification. Blood 110, 4445–4454. doi:10.1182/blood-2007-05-090514
Okuda, H., Stanojevic, B., Kanai, A., Kawamura, T., Takahashi, S., Matsui, H., et al. (2017). Cooperative gene activation by AF4 and DOT1L drives MLL-rearranged leukemia. J. Clin. Invest. 127, 1918–1931. doi:10.1172/JCI91406
Parrinello, M., and Rahman, A. (1982). Strain fluctuations and elastic constants. J. Chem. Phys. 76, 2662–2666. doi:10.1063/1.443248
Perner, F., Gadrey, J. Y., Xiong, Y., Hatton, C., Eschle, B. K., Weiss, A., et al. (2020). Novel inhibitors of the histone methyltransferase DOT1L show potent antileukemic activity in patient-derived xenografts. Blood 136, 1983–1988. doi:10.1182/blood.2020006113
Pettersen, E. F., Goddard, T. D., Huang, C. C., Couch, G. S., Greenblatt, D. M., Meng, E. C., et al. (2004). UCSF Chimera--a visualization system for exploratory research and analysis. J. Comput. Chem. 25, 1605–1612. doi:10.1002/jcc.20084
Raj, U., Kumar, H., Gupta, S., and Varadwaj, P. K. (2015). Novel DOT1L ReceptorNatural inhibitors involved in mixed lineage leukemia: A virtual screening, molecular docking and dynamics simulation study. Asian Pac. J. Cancer Prev. 16, 3817–3825. doi:10.7314/APJCP.2015.16.9.3817
Schuetz, D. A., Bernetti, M., Bertazzo, M., Musil, D., Eggenweiler, H.-M., Recanatini, M., et al. (2019). Predicting residence time and drug unbinding pathway through scaled molecular dynamics. J. Chem. Inf. Model. 59, 535–549. doi:10.1021/acs.jcim.8b00614
Song, X., Yang, L., Wang, M., Gu, Y., Ye, B., Fan, Z., et al. (2019). A higher-order configuration of the heterodimeric DOT1L–AF10 coiled-coil domains potentiates their leukemogenenic activity. Proc. Natl. Acad. Sci. 116, 19917–19923. doi:10.1073/pnas.1904672116
Sousa da Silva, A. W., and Vranken, W. F. (2012). Acpype - AnteChamber PYthon parser interfacE. BMC Res. Notes 5, 367. doi:10.1186/1756-0500-5-367
Spurr, S. S., Bayle, E. D., Yu, W., Li, F., Tempel, W., Vedadi, M., et al. (2016). New small molecule inhibitors of histone methyl transferase DOT1L with a nitrile as a non-traditional replacement for heavy halogen atoms. Bioorg. Med. Chem. Lett. 26, 4518–4522. doi:10.1016/j.bmcl.2016.07.041
Talukdar, A., Mukherjee, A., and Bhattacharya, D. (2022). Fascinating transformation of SAM-competitive protein methyltransferase inhibitors from nucleoside analogues to non-nucleoside analogues. J. Med. Chem. 65, 1662–1684. doi:10.1021/acs.jmedchem.1c01208
Tonge, P. J. (2018). Drug–target kinetics in drug discovery. ACS Chem. Neurosci. 9, 29–39. doi:10.1021/acschemneuro.7b00185
Valdés-Tresanco, M. S., Valdés-Tresanco, M. E., Valiente, P. A., and Moreno, E. (2020). AMDock: A versatile graphical tool for assisting molecular docking with autodock Vina and Autodock4. Biol. Direct 15, 12. doi:10.1186/s13062-020-00267-2
Wang, Z., Sun, H., Yao, X., Li, D., Xu, L., Li, Y., et al. (2016). Comprehensive evaluation of ten docking programs on a diverse set of protein-ligand complexes: The prediction accuracy of sampling power and scoring power. Phys. Chem. Chem. Phys. 18, 12964–12975. doi:10.1039/c6cp01555g
Webb, B., and Sali, A. (2016). Comparative protein structure modeling using MODELLER. Curr. Protoc. Bioinforma. 54, 5–37. doi:10.1002/cpbi.3
Williams, T., Kelley, C., Bersch, C., Bröker, H.-B., Campbell, J., Cunningham, R., et al. (2017). Gnuplot 5.0: An Interactive Plotting Program, 7.
Yi, Y., and Ge, S. (2022). Targeting the histone H3 lysine 79 methyltransferase DOT1L in MLL-rearranged leukemias. J. Hematol. Oncol. 15, 35. doi:10.1186/s13045-022-01251-1
Yu, W., Chory, E. J., Wernimont, A. K., Tempel, W., Scopton, A., Federation, A., et al. (2012). Catalytic site remodelling of the DOT1L methyltransferase by selective inhibitors. Nat. Commun. 3, 1288. doi:10.1038/ncomms2304
Yu, W., Smil, D., Li, F., Tempel, W., Fedorov, O., Nguyen, K. T., et al. (2013). Bromo-deaza-SAH: A potent and selective DOT1L inhibitor. Bioorg. Med. Chem. 21, 1787–1794. doi:10.1016/j.bmc.2013.01.049
Zhang, C., Sultan, S. A., T, R., and Chen, X. (2021). Biotechnological applications of S-adenosyl-methionine-dependent methyltransferases for natural products biosynthesis and diversification. Bioresour. Bioprocess. 8, 72. doi:10.1186/s40643-021-00425-y
Keywords: DOT1L, nucleoside inhibitors, molecular dynamics, τRAMD, residence time
Citation: Flores-León CD, Colorado-Pablo LF, Santos-Contreras MÁ and Aguayo-Ortiz R (2023) Determination of nucleoside DOT1L inhibitors’ residence times by τRAMD simulations. Front. Drug. Discov. 2:1083198. doi: 10.3389/fddsv.2022.1083198
Received: 28 October 2022; Accepted: 21 December 2022;
Published: 09 January 2023.
Edited by:
Yudibeth Sixto-López, University of Granada, SpainReviewed by:
Arménio Jorge Moura Barbosa, New University of Lisbon, PortugalArindam Talukdar, Indian Institute of Chemical Biology (CSIR), India
Copyright © 2023 Flores-León, Colorado-Pablo, Santos-Contreras and Aguayo-Ortiz. This is an open-access article distributed under the terms of the Creative Commons Attribution License (CC BY). The use, distribution or reproduction in other forums is permitted, provided the original author(s) and the copyright owner(s) are credited and that the original publication in this journal is cited, in accordance with accepted academic practice. No use, distribution or reproduction is permitted which does not comply with these terms.
*Correspondence: Rodrigo Aguayo-Ortiz, rodaguayo@comunidad.unam.mx