- 1Department of Chemistry, University of Buea, Buea, Cameroon
- 2Ersilia Open Source Initiative, Cambridge, United Kingdom
- 3The Wistar Institute, Philadelphia, Pennsylvania, PA, United States
- 4Institute of Pharmacy, Martin-Luther University Halle-Wittenberg, Halle (Saale), Germany
Current antiviral drug discovery efforts face many challenges, including development of new drugs during an outbreak and coping with drug resistance due to rapidly accumulating viral mutations. Emerging artificial intelligence and machine learning (AI/ML) methods can accelerate anti-infective drug discovery and have the potential to reduce overall development costs in Low and Middle-Income Countries (LMIC), which in turn may help to develop new and/or accessible therapies against communicable diseases within these countries. While the marketplace currently offers a plethora of data-driven AI/ML tools, most to date have been developed within the context of non-communicable diseases like cancer, and several barriers have limited the translation of existing tools to the discovery of drugs against infectious diseases. Here, we provide a perspective on the benefits, limitations, and pitfalls of AI/ML tools in the discovery of novel therapeutics with a focus on antivirals. We also discuss available and emerging data sharing models including intellectual property-preserving AI/ML. In addition, we review available data sources and platforms and provide examples for low-cost and accessible screening methods and other virus-based bioassays suitable for implementation of AI/ML-based programs in LMICs. Finally, we introduce an emerging AI/ML-based Center in Cameroon (Central Africa) which is currently developing methods and tools to promote local, independent drug discovery and represents a model that could be replicated among LMIC globally.
Introduction
Even with extensive access to resources, funding, and talent, drug research and development is a complex, expensive, and time-consuming endeavour. Despite the advances made toward drug discovery procedures that combine traditional and modern methods, most drugs fail to achieve regulatory approvals and reach the market, a phenomenon known as attrition (Waring, et al., 2015). Currently, over 90% of drug candidates fail between phase I clinical trials and regulatory approval, resulting in substantial loss of financial investment and resources (Fleming, 2018).
Traditional methods of drug discovery include finding and validating a putative drug target, followed by the development of a target-based bioassay and identifying a lead compound that interacts with the target with significant activity. At this stage, hit compounds generally undergo rounds of hit-to-lead optimization to improve stability, activity, and selectivity over toxicity, among other parameters. Additionally, the compounds being examined are investigated in a batch of assays to test their abilities to produce the same observed response within living animals (in vivo) or isolated living tissues (ex vivo) (Hughes et al., 2011).
One avenue to reduce the cost and duration of drug discovery is the use of in silico protocols in the early stages of the drug research and development pipeline. In silico methods can lower the attrition rate by identifying drug candidates with predicted suitable therapeutic activities and excluding compounds with undesirable traits such as predicted toxicity or poor pharmacokinetics (Beresford et al., 2004; Hughes J. D. et al., 2008; Hughes L. D. et al., 2008; Gawwehn et al., 2016; Zhang et al., 2017). Approaches like molecular docking and quantitative structure-activity relationship (QSAR) modeling are used to identify hits in virtual compound libraries as well as predict and optimize molecular bioactivity (Golbraikh et al., 2016). Predictions that can be obtained and tested experimentally for accuracy include physicochemical properties (such as logP and solubility) and the binding mode of a ligand (small molecule/protein) to a target (protein). To predict ligand-protein interactions, a high-resolution protein structure is necessary, ideally with previous knowledge of other ligands bound to the intended binding site. Fine-grained molecular dynamics simulations/relaxations, for instance, can be used to understand the atomistic details of the ideal ligand-protein complex, which in turn leads to a reduced number of suggested final molecules for the experimentalists (i.e., medicinal chemists and biologists) that potentially have better activities when compared to the starting/reference compound. However, while modern physics-based computational methods such as docking and molecular dynamics simulations are able to simulate specific ligand-target interactions, a current challenge of computational drug discovery is the modeling of compound effects at phenotypic and physiological levels in order to improve translation to in vivo experiments, where issues related to efficacy and drug absorption, distribution, metabolism excretion, and toxicity (ADMET) may emerge (Cherkasov et al., 2014). These predictions are generated by data-driven approaches, which ultimately relies on the notion that similar molecules tend to have similar activities. Limitations of such predictions are traced to small training sets to build the models, (Zhao, 2017), the narrow chemical space covered by these training sets (Stouch et al., 2003), experimental data errors (Fourches et al., 2010), and a lack of prospective experimental validations (Tropsha, 2010). Additionally, the hypothesis that similar compounds will have similar activities could be limited if only based on chemical structure and target activity (Zhang et al., 2017), potentially resulting in inaccurate predictions in the presence of activity cliffs (Stumpfe et al., 2019).
Data-driven drug discovery, and in particular the application of artificial intelligence and machine learning (AI/ML) tools, have been suggested as promising strategies to model compound effects that cannot be simulated with physics-based methods alone (Schneider et al., 2020; Jayatunga et al., 2022), as well as to devise sophisticated, more robust, and biologically relevant similarity metrics between compounds (Fernández-Torras et al., 2022a). From a practical perspective, AI/ML methods can be considered to be QSAR models, where a set of predefined physicochemical or structural descriptors of the molecules (molecular weight, number of hydrogen bond donors, etc.) are used as predictor variables of an activity of interest (e.g. cellular growth inhibition). Typically, these models require substantial pre-existing experimental knowledge (Baskin 2019), which limits their potential to generate genuinely novel chemistries or be applied to understudied disease areas. By contrast, modern AI/ML algorithms, including those that can be trained with only a few training samples (Altae-Tran et al., 2017), are self-trained and/or can learn from multiple datasets simultaneously (Stanley et al., 2021). Modern AI/ML algorithms may provide a viable data-driven solution to operate in low-data regimes. Moreover, AI/ML models for drug discovery can perform tasks beyond bioactivity prediction, including a broad set of techniques to capture complex ‘omics’ profiles, the design of retrosynthesis pathways, hit-to-lead optimization through generative models, among many others (Schneider et al., 2020).
In principle, AI/ML approaches to drug discovery could be applied to any disease area, ranging from non-communicable diseases such as cancer and Alzheimer’s to communicable diseases such as viral and bacterial infections. To this end, access to biological and chemical data is essential (Gupta et al., 2021). Features like structural properties, gene expression levels and/or gene sequencing, subcellular locations and network topological features can be used to identify or predict drug targets (Hu et al., 2019) as well as estimate factors like toxicity, solubility, selectivity, and kinetics (Brown, 2020). At the moment, the majority of AI/ML tools available to the research community have been trained on historical (public) data collected from large chemical and bioactivity databases, as well as ‘omics’ resources and biomedical knowledge bases. Therefore, the availability and performance of AI/ML models are biased, to a great extent, towards disease areas that have traditionally received more attention and for which richer datasets are consequently available. Indeed, infectious disease research is hampered by the lack of validated targets, poor molecular characterization of the pathogens and scarcity of large screening datasets (De Rycker et al., 2018).
The amount of available data for a particular disease area is tightly bound to research investment. The intrinsic cost and risk of investment in drug discovery have caused pharmaceutical companies and research funding agencies to focus on diseases for which incentives are high, i.e. non-communicable diseases that affect the Global North or High-Income Countries (HIC). Currently, only 15% of the drugs in development are targeting infectious diseases (WHO, 2022), effectively neglecting the needs of Low and Lower Middle-Income Countries (LMIC), which carry most of the world’s communicable disease burden. For example, as of 2016, approved antiviral drugs targeted only about 10 of the over 200 viruses known to infect humans (de Clercq and Li 2016), with several challenges hampering the antiviral drug discovery pipeline, including not only lack of funding but also lack of knowledge on viral biology (Adamson et al., 2021). Likewise, there is a need for novel antibacterial and antifungal therapies (Perfect, 2017; De Rycker et al., 2018). Many LMIC governments are unable to prioritize investment in scientific innovation, with most countries dedicating less than 0.5% of their domestic gross product to research and development activities (UNESCO, 2020). Arguably, AI/ML methods can have the greatest impact in settings where the cost and time to conduct effective experiments remain prohibitive. Paradoxically, though, these methods are not being developed in these settings precisely because pre-existing datasets and incentives are almost nonexistent. In addition, the shortage of skills and training in data science, computer science, chemoinformatics and bioinformatics in LMIC further hampers the development of AI/ML methods in low-resourced countries. As a result, the research inequality that characterizes drug discovery (i.e. greater investment in non-communicable diseases that affect the Global North and poor investment in communicable diseases that affect the Global South) extends to AI/ML research.
In this review article, we discuss existing and potential attempts to reverse these trends with a focus on antiviral drug discovery on the African continent. In particular, we discuss available data sources and their limitations while emphasizing existing African natural products databases, an untapped resource of novel chemical structures. In addition, we describe new models for data sharing and highlight a set of AI/ML-based initiatives to facilitate access to computational tools worldwide. Finally, we present an emerging initiative for a leading drug discovery center based in Central Africa that will capitalize on such computational tools to provide cost-effective drugs against infectious and communicable diseases.
Available data for antiviral drug discovery
Availability of good quality, task-specific data is perhaps the most important requirement for successful AI/ML modeling. Applied antiviral drug discovery involves knowledge of viral protein targets and their ligands, as well as phenotypic response measurements in infected cells. Knowledge of human targets may also be relevant, especially for host-directed therapies and host-pathogen interaction disruption. Generally, publicly available databases of small molecules and their bioactivities and human targets (ChEMBL (Mendez et al., 2019), PubChem (Kim et al., 2022) and DrugBank (Wishart et al., 2018), among others) provide starting points for experimental testing and AI/ML model training. In the context of research performed in LMIC, three specific regions of the chemical space are very interesting: natural product (NP) databases (especially from endemic plant and marine species) (Newman and Cragg, 2020; Ebob et al., 2021), known antiviral catalogs, and approved/advanced experimental drug databases to be used in drug repurposing (Duran-Frigola et al., 2017). Notably, Table 1 presents a summary of the most remarkable databases for NP-based drug discovery, as well as antiviral-oriented databases. In Table 2 we present a selection of drug databases, with potential for drug repurposing, along with target resources.
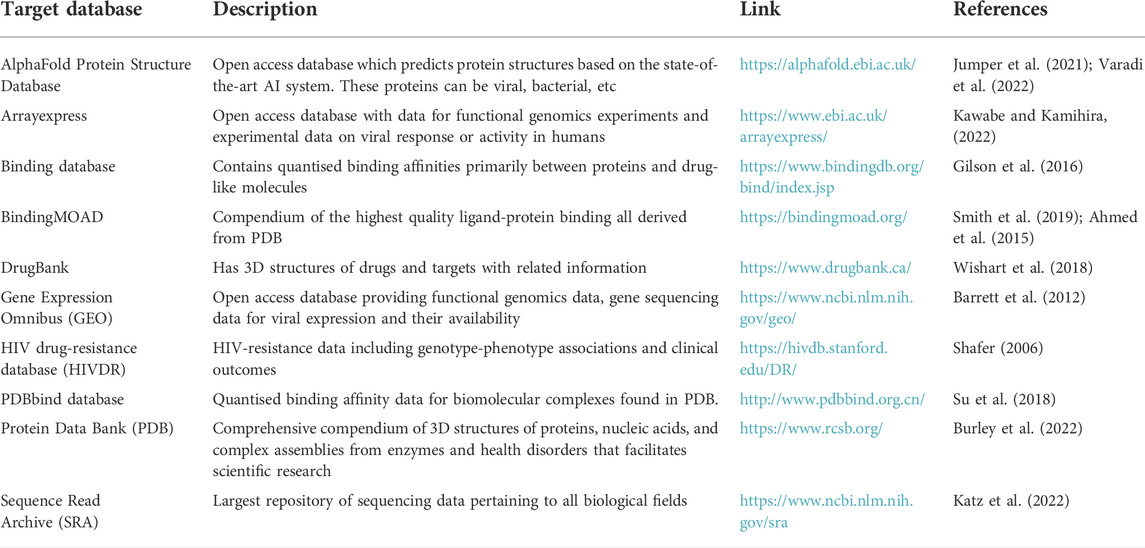
TABLE 2. Selected gene centric databases for integrative knowledge graphs, with a focus on drugs and drug target interactions.
As shown in Table 1, there is a growing number of open databases that provide good starting points for antiviral drug discovery, including a rich repertoire of natural products. For example, many of these NPs have shown antiviral potency against SARS-CoV-2 at concentrations less than 10 µM (Ebob et al., 2021).
However, several challenges need to be addressed to streamline these and other datasets in computational drug discovery pipelines (Krallinger et al., 2015; Tetko et al., 2016). First, data redundancy between the different available databases may cause bias in the extraction of information from the databases and subsequent analysis (Yonchev et al., 2018). Second, poor quality metadata hampers the interpretation of the available information (Williams et al., 2012; Lamy et al., 2020), and lack of computer-readable standard formats make information extraction difficult (Bauer-Mehren et al., 2009). Finally, links to target- and pathogen-centered databases are typically lacking, creating a disconnect between chemistry-centered and biology-centered resources.
New models for data sharing
Despite ongoing efforts by the scientific community to collect experimental data on putative anti-infective molecules, the scarcity of publicly available data in diseases of interest such as antivirals hinders the development of novel AI/ML tools. An avenue to overcome this limitation is to leverage the knowledge accumulated over the years by pharmaceutical companies. While the discovery of anti-infectives may not have been a top priority for many companies, it is clear that they still treasure the majority of data in this domain, sometimes resulting in remarkable initiatives like the GSK Tres Cantos Open Lab or Drugs for Neglected Diseases Initiative (DNDi). Although pharmaceutical companies often publish their results in scientific publications, they only share a small subset of the molecules screened to, understandably, protect the industry’s intellectual property (IP). This trend is particularly acute in primary screenings, where hundreds of thousands of compounds may have been tested. Incomplete disclosure of these experiments hampers the full realization of data-driven drug discovery (Mervin et al., 2015). Although large-scale open-source drug discovery initiatives exist (Antonova-Koch et al., 2018), these are comparatively rare and may still find IP constraints when private stakeholders are involved.
AI/ML offers a unique opportunity to exploit drug screening results without disclosing the identity of proprietary chemical libraries. The so-called privacy-preserving AI/ML approach proposes that IP-sensitive data can be effectively made available in the form of AI/ML models, which retain the essential properties of the training data but do not reveal the identity of the compounds used to train the model. A foundational example of this approach is the MELLODDY Consortium (Burki, 2019), orchestrating data sharing between 10 pharmaceutical companies, thereby compiling the largest collection of compounds and bioactivity endpoints in an IP-protected setting. A key feature of the MELLODDY approach is the decentralization of data, followed by a training scheme of predictive AI/ML models that prevents exposure to proprietary information. AI/ML models developed by the MELLODDY consortium are likely to have a significant impact on the academic scientific community since they capture a formidable amount of data previously owned by pharmaceutical companies (https://www.melloddy.eu/). Similar consortia have been devised in the medical informatics field, with the goal to improve diagnostics AI/ML models by accessing large patient databases while maintaining confidentiality (Warnat-Herresthal et al., 2021). In this line, tools for AI/ML model encryption are flourishing, offering a data-sharing toolbox for data scientists operating at the intersection between private and public stakeholders (Graepel et al., 2013). Researchers based in the LMIC are expected to be amongst the greatest beneficiaries of new data sharing models since they will gain access to data collected from external sources that would otherwise be inaccessible or unaffordable.
Data integration tools for drug discovery
In addition to greater availability of data to cover the gap in antiviral drug discovery, there is the need to design data integration tools that are able to yield amenable inputs for AI/ML modeling. In the context of non-communicable diseases, and especially in the field of anticancer drug discovery, a plethora of data integration protocols have been suggested, with applications in drug repurposing (Luo et al., 2021), virtual phenotypic screening (Sharifi-Noghabi et al., 2021), and target discovery (Rodrigues and Bernardes, 2020), among others. The underlying principle behind all these data integration methods is that data collected from multiple sources can be unified and harmonised in a single resource that can serve as relevant input data for AI/ML modeling. Examples of the necessary sources to build integrative tools include gene centric databases, disease annotation databases and, especially, chemical-protein interaction data (Table 2). Today, a favorite structure for a unified resource is a so-called biomedical knowledge graph. Early examples of comprehensive knowledge graphs include HetioNet (Himmelstein et al., 2017) and the Harmonizome (Rouillard et al., 2016), where data related to genes/proteins, small molecules, cells, diseases, etc. Is centralized in a large network containing thousands of nodes and millions of edges representing ligand-protein interactions, disease-gene associations, gene expression profiles, etc. Modern versions of these biomedical knowledge graphs may contain up to about a hundred million edges (Santos et al., 2022), and are therefore an extraordinarily rich starting point for AI/ML modeling in many disease areas. Moreover, several resources greatly simplify the adaptation of the data contained within these knowledge graphs into vectorial numerical representations that can be plugged to conventional AI/ML algorithms. For example, the Bioteque contains pre-calculated embeddings (i.e. ready-to-use vectorial representations) for thousands of biological entities, capturing the information contained within a gigantic knowledge graph (Fernandez-Torras et al., 2022b). Two years ago, and with a focus on small molecules, the Chemical Checker (Duran-Frigola et al., 2020) was published, providing an unprecedented amount of standardized and intensively processed data, in the form of numerical vectors, for almost one million bioactive compounds found in the public domain.
Unfortunately, though, all the major integrative knowledge graphs are acutely human-centric, meaning they mostly contain information about human genes and cells. Systematic integration of pathogen genomes and biology is currently lacking. As a result, infectious disease biology is difficult to capture with existing resources. Although several attempts have been made by mapping host-pathogen molecular interactions (most notably in the context of the COVID-19 pandemic) (Gordon et al., 2020), the available data is still far from commensurating with non-communicable disease data, especially cancer data for which a formidable number of genomic and phenotypic screening experiments have been performed. From a methodological standpoint, exploitation of a knowledge graph containing viral or bacterial data would not differ greatly from the already-available approaches suggested by resources like the Bioteque, since graph embedding techniques are relatively domain-agnostic and can be applied to a broad range of data types (Cai et al., 2018). The main challenge lies in the incorporation of pathogen data to the knowledge graph. A better characterisation of pathogen disease biology, including gene functions, metabolic pathways and signaling networks, and a more detailed description of the mechanisms of host-pathogen interactions, are key to achieving a biomedical knowledge graph that represents non-communicable and communicable diseases with equal depth and scope.
Ready-to-use AI/ML
Despite the growing number of AI/ML methods for drug discovery, many of them are either behind a paywall or not accessible in a user-friendly manner. With limited funding and access to data science expertise, this poses a real barrier to adoption by LMIC researchers. In recent years, the concept of ‘model hubs’ has become popular thanks to initiatives such as HuggingFace (Wolf et al., 2020), PyTorch Hub (https://pytorch.org/hub/) or TensorFlow Hub (https://www.tensorflow.org/hub). In short, these platforms provide access to a wealth of ready-to-use AI/ML models, which are transforming the fields of natural language processing and image analysis. The major stakeholders in the AI/ML industry (including tech corporations, academic groups and data science centers) are actively contributing their models to these hubs. As a result, users can run state-of-the-art AI/ML models with minimal effort, which has facilitated the inclusion of AI/ML assets into a broad range of disciplines and real-world applications. Unfortunately, though, the scope of these resources is generalist, with poor representation of computational biology and chemistry in their catalogs. In the biomedical domain, a few open-source initiatives, such as Kipoi (Avsec et al., 2019) and ModelHub.ai (Hosny et al., 2019) aim at disseminating pre-trained AI/ML models specific to certain areas such as genomics or medical image analysis, although a reference resource including a significant amount of drug discovery AI/ML models is still lacking.
In addition to providing out-of-the-box predictions for experimental researchers through model hubs, new resources containing ready-made datasets for AI/ML modeling in drug discovery are an excellent starting point for modeling endeavors. Particularly relevant is the recently published Therapeutics Data Commons (TDC) (Huang et al., 2021), a curated compendium of datasets covering the major stages of drug discovery. TDC works with the concept of leaderboards, so researchers can test their AI/ML algorithms and benchmark them. Other benchmarking includes MoleculeNet (Wu et al., 2018), MOSES (Polykovskiy et al., 2020), some of the Kaggle (https://kaggle.com) competitions, and the DREAM challenges (https://dreamchallenges.org). Recently, open-source drug discovery initiatives such as Open Source Malaria (Williamson et al., 2016; Tse et al.,. 2021) and Open-Source Antibiotics (https://github.com/opensourceantibiotics) have organized AI/ML-oriented challenges as part of their experimental cycle, offering a truly collaborative setting for data scientists and experimentalists.
Finally, the AI/ML community has invested significant efforts towards simplifying the model training procedure, facilitating the creation of competent AI/ML models without the need for advanced data science skills. Overall, automated AI/ML (AutoML) methods like AutoGluon (Erikson et al., 2020), AutoSklearn (Freuer et al., 2022), AutoKeras (Jin et al., 2019), FLAML (Wang et al., 2021), and others, are likely to play a key role in the adoption of AI/ML modeling capacity, freeing the user from algorithmic and hyperparameter search and optimization. In low-resourced settings where data science skills are typically scarce, AutoML functionalities can offer out-of-the-box solutions with competitive performance. A few attempts have been made to provide AutoML functionality for drug discovery (Shen et al., 2021), although the bulk of the existing AI/ML research in the field is still the result of highly specialized work. Greater availability of such AutoML tools is necessary to ensure the incorporation of AI/ML promptly in the drug discovery cycle, without the need to externalize the model creation step.
Biological assays for generating AI/ML models and functional validation of AI/ML predictions
The flip side of drug development in LMICs includes the challenge of functionally validating predictions generated in virtual settings. While AI/ML-based methods can both reduce and prioritize the number of leads that need to be validated, assays that can incorporate functional testing with high-throughput remain necessary. NP and drug repurposing collections, as exemplified in Tables 1 and 2, as well as ‘pathogen boxes’ distributed by initiatives like Medicines for Malaria Venture (MMV; https://mmv.org) may provide the necessary chemical matter to perform these experiments in LMICs, coupled with the development at a relatively limited throughput of chemical series in local synthetic chemistry laboratories.
To also help address these challenges as exemplified in antiviral therapeutics, our group has developed new and leveraged existing assays which can be transferred to laboratories in LMIC for independent research. For example, publicly available cell lines such as the J-Lat T cells (Jordan et al., 2003) which contain an inducible but non-infectious HIV clone encoding a GFP reporter, can be probed to monitor effects of chemical leads on HIV latency reversal or suppression of HIV provirus transcription (Tietjen et al., 2018; Divsalar et al., 2020). If local propagation of live virus is available, infection-based assays that include use of publicly available, lab-adapted subtype B (Adachi et al., 1986) and subtype C (Ndung’u et al., 2000) HIV strains become possible in replication-competent cell lines or locally-acquired peripheral blood mononuclear cells (Leteane et al., 2012; Tietjen et al., 2015). If expression of a protein target of interest in trans affects cell viability, another attractive option includes the yeast growth restoration assay (Balgi and Roberge, 2009), where a multicopy DNA plasmid encoding the protein target of interest is placed under the control of an inducible GAL1 promoter. When expressed in yeast in the presence of galactose, expression of this protein target then inhibits yeast growth over time, as measured by culture turbidity, which in turn can be restored by co-incubation with chemical leads that inhibit the target. This approach, for example, allowed us to validate new inhibitors of the influenza A M2 viroporin that were initially found by virtual screening approaches (Duncan et al., 2020). If disruption of protein-protein interactions is desired, another emerging but attractive option is use of AlphaScreen or homogenous time resolved fluorescence (HTRF)-based methods where tagged proteins of interest are bound to respective donor and acceptor beads. When a binding event occurs in vitro, luminescence or fluorescence is produced, which in turn can be inhibited by binding inhibitors (Yasgar et al., 2016). Such approaches were used by us, for example, to identify natural products that block interactions of the SARS-CoV-2 spike glycoprotein with its host ACE2 entry receptor (Tietjen et al., 2021; Ivernizzi et al., 2022). Chemical leads can also be readily assessed for effects on cell viability or toxicity using colorimetric-based reagents like (3-(4,5-dimethylthiazol-2-yl)-2,5-diphenyltetrazolium bromide (MTT) (Leteane et al., 2012). If viral infection results in extensive cytopathic effects and reduced cell viability in vitro, these reagents can also be used to monitor viral infection and restoration of cell viability by viral inhibitors (Tietjen et al., 2021). Assays like these are also amenable to being scaled up to 96-well format for improved screening throughput across NP or other chemical libraries as well as hits prioritized by AI/ML methods. While these assays do require a level of cell culture and molecular biology infrastructure, luminescence or fluorescence plate readers, and ideally access to flow cytometry, costs for these types of equipment are reducing quickly. Universities with synthetic or medicinal chemistry expertise will also be at an advantage to develop their chemical leads even with relatively straightforward synthesis strategies.
However, challenges in many LMIC include ensuring that proper scientific expertise for AI/ML methods or biological assays is perpetuated in local universities and that required infrastructure is optimally maintained. One potential option toward addressing these challenges includes introducing a series of recurring, intensive, and hands-on workforce development laboratory training and instruction sessions, akin to the Wistar Institute’s Biomedical Technology Training Program (https://wistar.org/education-training/biomedical-technician-training-program), designed to train promising students from underserved or related communities to become research technicians that can readily meet the employment needs of local academic institutions and health science industries. Similar programs can be performed in LMIC once adapted to train students in computational techniques. Alternatively, equipment technicians from HIC can be involved with these programs to not only train students on instrument use and maintenance but also repair and certify local equipment. This change of paradigm in scientific collaborations between HIC and LMIC, where committed knowledge sharing, and capacity building are embedded throughout the project design is essential to sustainably and permanently increase meaningful research capacity in LMIC. This commitment to develop capacity in LMIC is distinct from “helicopter research,” where scientists from HIC liaise with collaborators in LMIC to merely coordinate data collection or extract local resources.
Building local capacity in AI/ML for antiviral drug discovery
Consistent with objectives discussed above, the University of Buea in Cameroon is initiating a Center for Drug Discovery (UB-CeDD) focused on multiple drug discovery pipelines including the discovery of novel plant-based antivirals (Figure 1), among others. The establishment of an integrative center for drug discovery in Central Africa is key to developing the health research and development in the region, akin to what has been successfully demonstrated by the H3D Centre in Southern Africa (Winks et al., 2022). The overall goal of the UB-CeDD is to discover novel antiviral compounds based on NP core structures. Initial antiviral targets of interest include proteins from human immunodeficiency virus (HIV) and severe acute respiratory syndrome coronavirus 2 (SARS-CoV-2), although other targets are intended to be pursued. The UB-CeDD will combine and implement a virtual screening procedure that couples AI/ML models and physics-based methods like molecular docking and molecular dynamics simulations. Primary hits will be identified by machine learning, and these will then be docked, with the docked poses scored using several protein-ligand scoring algorithms. The goal is to develop a cloud-based virtual screening platform that permits compounds to be screened computationally from the African Natural Products Database and others (ANPDB, Ntie-Kang et al., 2017; Simoben et al., 2020). To develop efficient AI/ML models, we will generate a well-curated dataset of compounds that have been tested in antiviral assays within the same laboratory conditions. Since such data are currently scarce, we are screening several hundred natural and synthesized compounds from collaborative partner laboratories through the Nature-inspired Discovery of Novel Antivirals (NiDNA) network. The compounds are being screened, for example, for their inhibitory capacities against vital SARS-CoV-2 drug targets like the main protease and the binding of the viral spike to the angiotensin-converting enzyme 2 (ACE2) and for their potential to reverse latency in HIV-infected cells. Importantly, these assays are transferable to the LMIC laboratories involved in the collaboration. The more compounds are tested in the assays, the more robust will the generated AI/ML models be. Within an LMIC like Cameroon, the generated models will go a long way to train graduate students and postdoctoral researchers on how to implement AI/ML in an academic setting. This will speed up the process toward finding antiviral lead compounds contained in plant biodata and synthesized leads based on pharmacophores contained in NPs and eventually guide the synthesis of novel analogues with high potency and devoid of potential toxicity effects. Some web tools which could potentially be used for developing ML models have been summarized in Table 3.
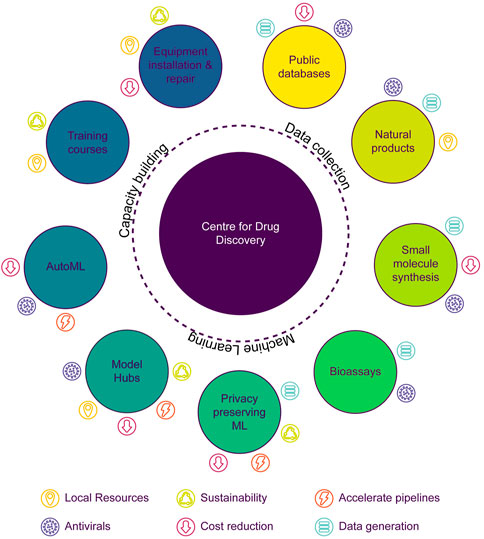
FIGURE 1. Schematic representation of the three main areas being developed at the Center for Drug Discovery at the University of Buea (UB-CeDD), Cameroon, to achieve a sustainable antiviral drug discovery pipeline in Central Africa.
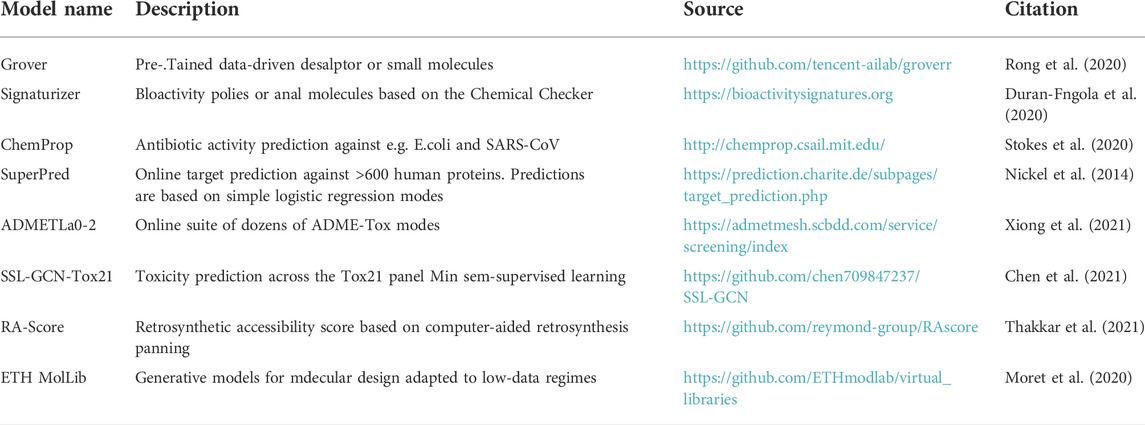
TABLE 3. A short and illustrative list of readily available online AI/ML, covering several stages of the drug discovery process. Please note that the list is not comprehensive. Check resources like the Ersilia Model Hub (https://ersilia.io/model-hub) for a larger compendium.
Conclusion
In this review article, we have discussed the current opportunities to apply AI/ML technologies in underserved research settings. We have focused on the discovery of antiviral drugs, an underserved therapeutic area with great importance in LMIC. To build ML models and use AI to predict biological activities of drug candidates, there is need for data. Such data would include chemical structures with known biological activities (often included in molecule databases). Such data could be included in a broad array of ML models, to make predictions. This is the case with data available in open access platforms/models. Databases of known drug targets for NPs have also been included in this survey. There are also ready-to-use models and web-based tools that only require the user to populate the model with their own data (generated from in-house chemical libraries) or through partnerships with pharmaceutical companies. In this review, we have been focused on compound libraries and ML tools that could be useful to generate predictive tools for antiviral lead compound discovery within economically limited settings like academic institutions in LMICs. We argue that AI/ML can offer a cost-effective solution, although better access to viral assays data and better data integration protocols will be needed for effective adoption of AI/ML tools. We also describe some antiviral assays we plan to conduct and are already conducting in partner laboratories to include in the generation of ML predictions. We propose that a fluent research cycle involving data collection, computational prediction and experimental testing can be implemented in-country, and we propose the emerging CeDD in Buea as an exemplary case for Western and Central Africa.
Author contributions
Conception: MD-F, FN-K, SE and IT; Generation of preliminary data: IT, CTN-N, CVS, FN-K, LM, GT, SE, and MD-F; Writing of the first draft CVS, CTN-N, GT, IT, MD-F, and FN-K; Editing and approval of the final version CVS, CTN-N, GT, IT, LJM, SE, MD-F, and FN-K.
Funding
Financial support is acknowledged from the Bill & Melinda Gates Foundation through the Calestous Juma Science Leadership Fellowship awarded to FN-K (award number: INV-036848). LJM and IT supported by Robert I. Jacobs Fund of The Philadelphia Foundation; LJM is supported by the Herbert Kean, M.D., Family Professorship.
Acknowledgments
The authors acknowledge Kelly Chibale and Wolfgang Sippl for the fruitful scientific discussions.
Conflict of interest
The authors declare that the research was conducted in the absence of any commercial or financial relationships that could be construed as a potential conflict of interest.
Publisher’s note
All claims expressed in this article are solely those of the authors and do not necessarily represent those of their affiliated organizations, or those of the publisher, the editors and the reviewers. Any product that may be evaluated in this article, or claim that may be made by its manufacturer, is not guaranteed or endorsed by the publisher.
References
Adachi, A., Gendelman, H. E., Koenig, S., Folks, T., Willey, R., Rabson, A., et al. (1986). Production of acquired immunodeficiency syndrome-associated retrovirus in human and nonhuman cells transfected with an infectious molecular clone. J. Virol. 59 (2), 284–291. doi:10.1128/JVI.59.2.284-291.1986
Adamson, C. S., Chibale, K., Goss, R. J., Jaspars, M., Newman, D. J., and Dorrington, R. A. (2021). Antiviral drug discovery: Preparing for the next pandemic. Chem. Soc. Rev. 50 (6), 3647–3655. doi:10.1039/d0cs01118e
Ahmed, A., Smith, R. D., Clark, J. J., Dunbar, J. B., and Carlson, H. A. (2015). Recent improvements to binding MOAD: A resource for protein–ligand binding affinities and structures. Nucleic Acids Res. 43 (1), D465–D469. doi:10.1093/nar/gku1088
Altae-Tran, H., Ramsundar, B., Pappu, A. S., and Pande, V. (2017). Low data drug discovery with one-shot learning. ACS Cent. Sci. 3 (4), 283–293. doi:10.1021/acscentsci.6b00367
Antonova-Koch, Y., Meister, S., Abraham, M., Luth, M. R., Ottilie, S., Lukens, A. K., et al. (2018). Open-source discovery of chemical leads for next-generation chemoprotective antimalarials. Science 362 (6419), eaat9446. doi:10.1126/science.aat9446
Avsec, Ž., Kreuzhuber, R., Israeli, J., Xu, N., Cheng, J., Shrikumar, A., et al. (2019). The Kipoi repository accelerates community exchange and reuse of predictive models for genomics. Nat. Biotechnol. 37 (6), 592–600. doi:10.1038/s41587-019-0140-0
Balgi, A. D., and Roberge, M. (2009). Screening for chemical inhibitors of heterologous proteins expressed in yeast using a simple growth-restoration assay. Methods Mol. Biol. 486, 125–137. doi:10.1007/978-1-60327-545-3_9
Banerjee, P., Erehman, J., Gohlke, B. O., Wilhelm, T., Preissner, R., and Dunkel, M. (2015). Super natural II—A database of natural products. Nucleic Acids Res. 43 (1), D935–D939. doi:10.1093/nar/gku886
Barrett, T., Wilhite, S. E., Ledoux, P., Evangelista, C., Kim, I. F., Tomashevsky, M., et al. (2012). NCBI geo: Archive for functional genomics data sets—update. Nucleic Acids Res. 41 (1), D991–D995. doi:10.1093/nar/gks1193
Baskin, I. I. (2019). Is one-shot learning a viable option in drug discovery? Expert Opin. Drug Discov. 14 (7), 601–603. doi:10.1080/17460441.2019.1593368
Bauer‐Mehren, A., Furlong, L. I., and Sanz, F. (2009). Pathway databases and tools for their exploitation: Benefits, current limitations and challenges. Mol. Syst. Biol. 5 (1), 290. doi:10.1038/msb.2009.47
Beresford, A. P., Segall, M., and Tarbit, M. H. (2004). In silico prediction of ADME properties: Are we making progress? Curr. Opin. Drug Discov. Devel. 7 (1), 36–42.
Brown N. (Editor) (2020). Artificial intelligence in drug discovery (London, United Kingdom: Royal Society of Chemistry), 75.
Burki, T. (2019). Pharma blockchains AI for drug development. Lancet 393 (10189), 2382. doi:10.1016/S0140-6736(19)31401-1
Burley, S. K., Bhikadiya, C., Bi, C., Bittrich, S., Chen, L., Crichlow, G. V., et al. (2022). RCSB Protein Data Bank: Celebrating 50 years of the PDB with new tools for understanding and visualizing biological macromolecules in 3D. Protein Sci. 31, 187–208. doi:10.1002/pro.4213
Cai, H., Zheng, V. W., and Chang, K. C. C. (2018). A comprehensive survey of graph embedding: Problems, techniques, and applications. IEEE Trans. Knowl. Data Eng. 30 (9), 1616–1637. doi:10.1109/TKDE.2018.2807452
Chen, C. Y. C. (2011). TCM database@ taiwan: The world's largest traditional Chinese medicine database for drug screening in silico. PloS One 6 (1), e15939. doi:10.1371/journal.pone.0015939
Chen, J., Si, Y. W., Un, C. W., and Siu, S. W. (2021). Chemical toxicity prediction based on semi-supervised learning and graph convolutional neural network. J. Cheminformatics 13 (1), 93. doi:10.1186/s13321-021-00570-8
Cherkasov, A., Muratov, E. N., Fourches, D., Varnek, A., Baskin, I. I., Cronin, M., et al. (2014). QSAR modeling: Where have you been? Where are you going to? J. Med. Chem. 57 (12), 4977–5010. doi:10.1021/jm4004285
Costa, R. P. O., Lucena, L. F., Silva, L. M. A., Zocolo, G. J., Herrera-Acevedo, C., Scotti, L., et al. (2021). The SistematX web portal of natural products: An update. J. Chem. Inf. Model. 61 (6), 2516–2522. doi:10.1021/acs.jcim.1c00083
De Clercq, E., and Li, G. (2016). Approved antiviral drugs over the past 50 years. Clin. Microbiol. Rev. 29 (3), 695–747. doi:10.1128/CMR.00102-15
De Rycker, M., Baragaña, B., Duce, S. L., and Gilbert, I. H. (2018). Challenges and recent progress in drug discovery for tropical diseases. Nature 559 (7715), 498–506. doi:10.1038/s41586-018-0327-4
Diallo, B., Glenister, M., Musyoka, T. M., Lobb, K., and Tastan Bishop, Ö. (2021). Sancdb: An update on South African natural compounds and their readily available analogs. J. Cheminform. 13 (1), 37. doi:10.1186/s13321-021-00514-2
Divsalar, D. N., Simoben, C. V., Schonhofer, C., Richard, R., Sippl, W., Ntie-Kang, F., et al. (2020). Novel histone deacetylase inhibitors and HIV-1 latency-reversing agents identified by large-scale virtual screening. Front. Pharmacol. 11, 905. doi:10.3389/fphar.2020.00905
Duncan, M. C., Ogunéné, P. A., Kihara, I., Nebangwa, D. N., Naidu, M. E., Williams, D. E., et al. (2020). Virtual screening identifies chebulagic acid as an inhibitor of the M2(S31N) viral ion channel and influenza A virus. Molecules 25 (12), 2903. doi:10.3390/molecules25122903
Duran-Frigola, M., Mateo, L., and Aloy, P. (2017). Drug repositioning beyond the low-hanging fruits. Curr. Opin. Syst. Biol. 3, 95–102. doi:10.1016/j.coisb.2017.04.010
Duran-Frigola, M., Pauls, E., Guitart-Pla, O., Bertoni, M., Alcalde, V., Amat, D., et al. (2020). Extending the small-molecule similarity principle to all levels of biology with the Chemical Checker. Nat. Biotechnol. 38 (9), 1087–1096. doi:10.1038/s41587-020-0502-7
Ebob, O. T., Babiaka, S. B., and Ntie-Kang, F. (2021). Natural products as potential lead compounds for drug discovery against SARS-CoV-2. Nat. Prod. Bioprospect. 11 (6), 611–628. doi:10.1007/s13659-021-00317-w
Erikson, N., Mueller, J., Shirkov, A., Zhang, H., Larroy, P., Li, M., et al. (2020). AutoGluon-Tabular: Robust and accurate AutoML for structured data. arXiv, preprint arXiv:2003.06505. doi:10.48550/arXiv.2003.06505
Fernández-Torras, A., Comajuncosa-Creus, A., Duran-Frigola, M., and Aloy, P. (2022a). Connecting chemistry and biology through molecular descriptors. Curr. Opin. Chem. Biol. 66, 102090. doi:10.1016/j.cbpa.2021.09.001
Férnandez-Torras, A., Duran-Frigola, M., Bertoni, M., Locatelli, M., and Aloy, P. (2022b). Integrating and formatting biomedical data as pre-calculated knowledge graph embeddings in the Bioteque. Nat. Commun. 13 (1), 5304. doi:10.1038/s41467-022-33026-0
Fleming, N. (2018). How artificial intelligence is changing drug discovery. Nature 557 (7707), S55–S57. doi:10.1038/d41586-018-05267-x
Fourches, D., Muratov, E., and Tropsha, A. (2010). Trust, but verify: On the importance of chemical structure curation in cheminformatics and QSAR modeling research. J. Chem. Inf. Model. 50 (7), 1189–1204. doi:10.1021/ci100176x
Freuer, M., Eggensperger, K., Falkner, S., Lindauer, M., and Hutter, F. (2022). Auto-sklearn 2.0: Hands-free AutoML via meta-learning. ArXiv, preprint arXiv:2007.04074. doi:10.48550/arXiv.2007.04074
Gawehn, E., Hiss, J. A., and Schneider, G. (2016). Deep learning in drug discovery. Mol. Inf. 35 (1), 3–14. doi:10.1002/minf.201501008
Gilson, M. K., Liu, T., Baitaluk, M., Nicola, G., Hwang, L., and Chong, J. (2016). BindingDB in 2015: A public database for medicinal chemistry, computational chemistry and systems pharmacology. Nucleic Acids Res. 44 (D1), D1045–D1053. doi:10.1093/nar/gkv1072
Golbraikh, A., Wang, X. S., Zhu, H., and Tropsha, A. (2016). Predictive QSAR modeling: Methods and applications in drug discovery and chemical risk assessment. Handb. Comput. Chem. 2016, 1–48.
Gordon, D. E., Jang, G. M., Bouhaddou, M., Xu, J., Obernier, K., White, K. M., et al. (2020). A SARS-CoV-2 protein interaction map reveals targets for drug repurposing. Nature 583 (7816), 459–468. doi:10.1038/s41586-020-2286-9
Graepel, T., Lauter, K., and Naehrig, M. (2013). “ML confidential: Machine learning on encrypted data,” in Information security and cryptology – ICISC 2012. Editors T. Kwon, M. K. Lee, and D. Kwon (Berlin, Heidelberg: Springer), 7839. Lecture Notes in Computer Science. doi:10.1007/978-3-642-37682-5_1
Gupta, R., Srivastava, D., Sahu, M., Tiwari, S., Ambasta, R. K., and Kumar, P. (2021). Artificial intelligence to deep learning: Machine intelligence approach for drug discovery. Mol. Divers. 25 (3), 1315–1360. doi:10.1007/s11030-021-10217-3
Himmelstein, D. S., Lizee, A., Hessler, C., Brueggeman, L., Chen, S. L., Hadley, D., et al. (2017). Systematic integration of biomedical knowledge prioritizes drugs for repurposing. eLife 6, e26726. doi:10.7554/eLife.26726
Hosny, A., Schwier, M., Berger, C., Örnek, E. P., Turan, M., Tran, P. V., et al. (2019). Modelhub. ai: Dissemination platform for deep learning models. arXiv preprint arXiv:1911.13218. doi:10.48550/arXiv.1911.13218
Hu, Y., Zhao, T., Zhang, N., Zhang, Y., and Cheng, L. (2019). A review of recent advances and research on drug target identification methods. Curr. Drug Metab. 20, 209–216. doi:10.2174/1389200219666180925091851
Huang, K., Fu, T., Gao, W., Zhao, Y., Roohani, Y., Leskovec, J., et al. (2021). Therapeutics data commons: Machine learning datasets and tasks for drug discovery and development. arXiv preprint arXiv:2102.09548. doi:10.48550/arXiv.2102.09548
Huang, L., Xie, D., Yu, Y., Liu, H., Shi, Y., Shi, T., et al. (2018). Tcmid 2.0: A comprehensive resource for TCM. Nucleic Acids Res. 46 (1), D1117–D1120. doi:10.1093/nar/gkx1028
Hughes, J. D., Blagg, J., Price, D. A., Bailey, S., DeCrescenzo, G. A., Devraj, R. V., et al. (2008a). Physiochemical drug properties associated with in vivo toxicological outcomes. Bioorg. Med. Chem. Lett. 18 (17), 4872–4875. doi:10.1016/j.bmcl.2008.07.071
Hughes, J. P., Rees, S., Kalindjian, S. B., and Philpott, K. L. (2011). Principles of early drug discovery. Br. J. Pharmacol. 162 (6), 1239–1249. doi:10.1111/j.1476-5381.2010.01127.x
Hughes, L. D., Palmer, D. S., Nigsch, F., and Mitchell, J. B. (2008b). Why are some properties more difficult to predict than others? A study of qspr models of solubility, melting point, and log P. J. Chem. Inf. Model. 48 (1), 220–232. doi:10.1021/ci700307p
Ianevski, A., Simonsen, R. M., Myhre, V., Tenson, T., Oksenych, V., Bjørås, M., et al. (2022). DrugVirus. Info 2.0: An integrative data portal for broad-spectrum antivirals (BSA) and BSA-containing drug combinations (BCCs). Nucleic Acids Res. 50 (1), W272–W275. doi:10.1093/nar/gkac348
Ibezim, A., Debnath, B., Ntie-Kang, F., Mbah, C. J., and Nwodo, N. J. (2017). Binding of anti-trypanosoma natural products from african flora against selected drug targets: A docking study. Med. Chem. Res. 26 (3), 562–579. doi:10.1007/s00044-016-1764-y
Ivernizzi, L., Moyo, P., Cassel, J., Isaacs, F. J., Salvino, J. M., Montaner, L. J., et al. (2022). Use of hyphenated analytical techniques to identify the bioactive constituents of Gunnera perpensa L., a South African medicinal plant, which potently inhibit SARS-CoV-2 spike glycoprotein-host ACE2 binding. Anal. Bioanal. Chem. 414 (13), 3971–3985. doi:10.1007/s00216-022-04041-3
Jayatunga, M. K., Xie, W., Ruder, L., Schulze, U., and Meier, C. (2022). AI in small-molecule drug discovery: A coming wave. Nat. Rev. Drug Discov. 21, 175–176. doi:10.1038/d41573-022-00025-1
Jin, H., Song, Q., and Hu, X. (2019). Auto-keras: An efficient neural architecture search system." Proceedings of the 25th ACM SIGKDD International Conference on Knowledge Discovery & Data Mining, Anchorage AK USA, August 4 - 8, 2019. ACM
Jordan, A., Bisgrove, D., and Verdin, E. (2003). HIV reproducibly establishes a latent infection after acute infection of T cells in vitro. EMBO J. 22 (8), 1868–1877. doi:10.1093/emboj/cdg188
Jumper, J., Evans, R., Pritzel, A., Green, T., Figurnov, M., Ronneberger, O., et al. (2021). Highly accurate protein structure prediction with AlphaFold. Nature 596 (7873), 583–589. doi:10.1038/s41586-021-03819-2
Katz, K., Shutov, O., Lapoint, R., Kimelman, M., Brister, J. R., and O’Sullivan, C. (2022). The sequence read archive: A decade more of explosive growth. Nucleic Acids Res. 50, D387–D390. doi:10.1093/nar/gkab1053
Kawabe, Y., and Kamihira, M. (2022). Novel cell lines derived from Chinese hamster kidney tissue. PloS One 17, e0266061. doi:10.1371/journal.pone.0266061
Kim, S., Cheng, T., He, S., Thiessen, P. A., Li, Q., Gindulyte, A., et al. (2022). PubChem protein, gene, pathway, and taxonomy data collections: Bridging biology and chemistry through target-centric views of PubChem data. J. Mol. Biol. 434 (11), 167514. doi:10.1016/j.jmb.2022.167514
Krallinger, M., Leitner, F., Rabal, O., Vazquez, M., Oyarzabal, J., and Valencia, A. (2015). Chemdner: The drugs and chemical names extraction challenge. J. Cheminformatics 7 (1), S1. doi:10.1186/1758-2946-7-S1-S1
Lamy, J. B., Berthelot, H., Favre, M., and Tsopra, R. (2020). “Limits and variability in drug databases: Lessons learnt from drug comparisons,” in Digital personalized health and medicine (Amsterdam: IOS Press), 1329–1330. doi:10.3233/SHTI200426
Leteane, M. M., Ngwenya, B. N., Muzila, M., Namushe, A., Mwinga, J., Musonda, R., et al. (2012). Old plants newly discovered: Cassia sieberiana D.C. And Cassia abbreviata oliv. Oliv. Root extracts inhibit in vitro HIV-1c replication in peripheral blood mononuclear cells (PBMCs) by different modes of action. J. Ethnopharmacol. 141 (1), 48–56. doi:10.1016/j.jep.2012.01.044
Luo, H., Li, M., Yang, M., Wu, F. X., Li, Y., and Wang, J. (2021). Biomedical data and computational models for drug repositioning: A comprehensive review. Brief. Bioinform. 22 (2), 1604–1619. doi:10.1093/bib/bbz176
Mangal, M., Sagar, P., Singh, H., Raghava, G. P., and Agarwal, S. M. (2013). Npact: Naturally occurring plant-based anti-cancer compound-activity-target database. Nucleic Acids Res. 41 (1), D1124–D1129. doi:10.1093/nar/gks1047
Martin, H., Melo-Filho, C., Korn, D., Eastman, R., Rai, G., Simeonov, A., et al. (2022). Small molecule antiviral compound collection (SMACC): A database to support the discovery of broad-spectrum antiviral drug molecules. bioRxiv. [Preprint]. doi:10.1101/2022.07.09.499397
Mendez, D., Gaulton, A., Bento, A. P., Chambers, J., de Veij, M., Félix, E., et al. (2019). ChEMBL: Towards direct deposition of bioassay data. Nucleic Acids Res. 47, D930–D940. doi:10.1093/nar/gky1075
Mervin, L. H., Afzal, A. M., Drakakis, G., Lewis, R., Engkvist, O., and Bender, A. (2015). Target prediction utilising negative bioactivity data covering large chemical space. J. Cheminform. 7, 51. doi:10.1186/s13321-015-0098-y
Moret, M., Friedrich, L., Grisoni, F., Merk, D., and Schneider, G. (2020). Generative molecular design in low data regimes. Nat. Mach. Intell. 2 (3), 171–180. doi:10.1038/s42256-020-0160-y
Moumbock, A. F., Gao, M., Qaseem, A., Li, J., Kirchner, P. A., Ndingkokhar, B., et al. (2021). StreptomeDB 3.0: An updated compendium of streptomycetes natural products. Nucleic Acids Res. 49, D600–D604. doi:10.1093/nar/gkaa868
Ndung’u, T., Renjifo, B., Novitsky, V. A., McLane, M. F., Gaolekwe, S., and Essex, M. (2000). Molecular cloning and biological characterization of full-length HIV-1 subtype C from Botswana. Virology 278 (2), 390–399. doi:10.1006/viro.2000.0583
Newman, D. J., and Cragg, G. M. (2020). Natural products as sources of new drugs over the nearly four decades from 01/1981 to 09/2019. J. Nat. Prod. 83 (3), 770–803. doi:10.1021/acs.jnatprod.9b01285
Nickel, J., Gohlke, B. O., Erehman, J., Banerjee, P., Rong, W. W., Goede, A., et al. (2014). SuperPred: Update on drug classification and target prediction. Nucleic Acids Res. 42, W26–W31. doi:10.1093/nar/gku477
Ntie-Kang, F., Amoa Onguéné, P., Fotso, G. W., Andrae-Marobela, K., Bezabih, M., Ndom, J. C., et al. (2014b). Virtualizing the p-ANAPL library: A step towards drug discovery from african medicinal plants. PLoS One 9 (3), e90655. doi:10.1371/journal.pone.0090655
Ntie-Kang, F., Nwodo, J. N., Ibezim, A., Simoben, C. V., Karaman, B., Ngwa, V. F., et al. (2014a). Molecular modeling of potential anticancer agents from African medicinal plants. J. Chem. Inf. Model. 54 (9), 2433–2450. doi:10.1021/ci5003697
Ntie-Kang, F., Telukunta, K. K., Döring, K., Simoben, C. V., Moumbock, A., Aurélien, A. F., et al. (2017). Nanpdb: A resource for natural products from northern african sources. J. Nat. Prod. 80 (7), 2067–2076. doi:10.1021/acs.jnatprod.7b00283
Ntie-Kang, F., Zofou, D., Babiaka, S. B., Meudom, R., Scharfe, M., Lifongo, L. L., et al. (2013). AfroDb: A select highly potent and diverse natural product library from african medicinal plants. PloS One 8 (10), e78085. doi:10.1371/journal.pone.0078085
Onguéné, P. A., Ntie-Kang, F., Mbah, J. A., Lifongo, L. L., Ndom, J. C., Sippl, W., et al. (2014). The potential of anti-malarial compounds derived from african medicinal plants, part III: An in silico evaluation of drug metabolism and pharmacokinetics profiling. Org. Med. Chem. Lett. 4 (1), 6. doi:10.1186/s13588-014-0006-x
Perfect, J. R. (2017). The antifungal pipeline: A reality check. Nat. Rev. Drug Discov. 16 (9), 603–616. doi:10.1038/nrd.2017.46
Pilon, A. C., Valli, M., Dametto, A. C., Pinto, M. E. F., Freire, R. T., Castro-Gamboa, I., et al. (2017). NuBBEDB: An updated database to uncover chemical and biological information from Brazilian biodiversity. Sci. Rep. 7, 7215. doi:10.1038/s41598-017-07451-x
Polykovskiy, D., Zhebrak, A., Sanchez-Lengeling, B., Golovanov, S., Tatanov, O., Belyaev, S., et al. (2020). Molecular sets (MOSES): A benchmarking platform for molecular generation models. Front. Pharmacol. 11, 565644. doi:10.3389/fphar.2020.565644
Qureshi, A., Thakur, N., Tandon, H., and Kumar, M. (2014). AVPdb: A database of experimentally validated antiviral peptides targeting medically important viruses. Nucleic Acids Res. 42 (D1), D1147–D1153. doi:10.1093/nar/gkt1191
Rodrigues, T., and Bernardes, G. J. (2020). Machine learning for target discovery in drug development. Curr. Opin. Chem. Biol. 56, 16–22. doi:10.1016/j.cbpa.2019.10.003
Rong, Y., Bian, Y., Xu, T., Xie, W., Wei, Y., Huang, W., et al. (2020). Self-supervised graph transformer on large-scale molecular data. Adv. Neural Inf. Process. Syst. 33, 12559–12571. doi:10.48550/arXiv.2007.02835
Rouillard, A. D., Gundersen, G. W., Fernandez, N. F., Wang, Z., Monteiro, C. D., McDermott, M. G., et al. (2016). The harmonizome: A collection of processed datasets gathered to serve and mine knowledge about genes and proteins. Database 2016, baw100. doi:10.1093/database/baw100
Rutz, A., Sorokina, M., Galgonek, J., Mietchen, D., Willighagen, E., Gaudry, A., et al. (2022). The LOTUS initiative for open knowledge management in natural products research. eLife 11, e70780. doi:10.7554/eLife.70780
Rutz, A., Sorokina, M., Galgonek, J., Mietchen, D., Willighagen, E., Graham, J., et al. (2021). Open natural products research: Curation and dissemination of biological occurrences of chemical structures through wikidata. bioRxiv, preprint. doi:10.1101/2021.02.28.433265
Santos, A., Colaço, A. R., Nielsen, A. B., Niu, L., Strauss, M., Geyer, P. E., et al. (2022). A knowledge graph to interpret clinical proteomics data. Nat. Biotechnol. 40 (5), 692–702. doi:10.1038/s41587-021-01145-6
Schneider, P., Walters, W. P., Plowright, A. T., Sieroka, N., Listgarten, J., Goodnow, R. A., et al. (2020). Rethinking drug design in the artificial intelligence era. Nat. Rev. Drug Discov. 19 (5), 353–364. doi:10.1038/s41573-019-0050-3
Scotti, M. T., Herrera-Acevedo, C., Oliveira, T. B., Costa, R. P. O., Santos, S. Y. K. O., Rodrigues, R. P., et al. (2018). SistematX, an online web-based cheminformatics tool for data management of secondary metabolites. Molecules 23 (1), 103. doi:10.3390/molecules23010103
Shafer, R. W. (2006). Rationale and uses of a public HIV drug‐resistance database. J. Infect. Dis. 194 (1), S51–S58. doi:10.1086/505356
Sharifi-Noghabi, H., Jahangiri-Tazehkand, S., Smirnov, P., Hon, C., Mammoliti, A., Nair, S. K., et al. (2021). Drug sensitivity prediction from cell line-based pharmacogenomics data: Guidelines for developing machine learning models. Brief. Bioinform. 22 (6), bbab294. doi:10.1093/bib/bbab294
Shen, W. X., Zeng, X., Zhu, F., Qin, C., Tan, Y., Jiang, Y. Y., et al. (2021). Out-of-the-box deep learning prediction of pharmaceutical properties by broadly learned knowledge-based molecular representations. Nat. Mach. Intell. 3 (4), 334–343. doi:10.1038/s42256-021-00301-6
Simoben, C. V., Qaseem, A., Moumbock, A. F., Telukunta, K. K., Günther, S., Sippl, W., et al. (2020). Pharmacoinformatic investigation of medicinal plants from East Africa. Mol. Inf. 39, 2000163. doi:10.1002/minf.202000163
Singla, D., Sharma, A., Kaur, J., Panwar, B., and Raghava, G. P. (2010). BIAdb: A curated database of benzylisoquinoline alkaloids. BMC Pharmacol. 10, 4. doi:10.1186/1471-2210-10-4
Smith, R. D., Clark, J. J., Ahmed, A., Orban, Z. J., Dunbar, J. B., and Carlson, H. A. (2019). Updates to binding MOAD (mother of all databases): Polypharmacology tools and their utility in drug repurposing. J. Mol. Biol. 431 (13), 2423–2433. doi:10.1016/j.jmb.2019.05.024
Sorokina, M., Merseburger, P., Rajan, K., Yirik, M. A., and Steinbeck, C. (2021). COCONUT online: Collection of open natural products database. J. Cheminform. 13, 2. doi:10.1186/s13321-020-00478-9
Stanley, M., Bronskill, J. F., Maziarz, K., Misztela, H., Lanini, J., Segler, M., et al. (2021). “August. Fs-Mol: A few-shot learning dataset of molecules.” In Thirty-fifth Conference on Neural Information Processing Systems Datasets and Benchmarks Track (Round 2), May 13, 2021.
Sterling, T., and Irwin, J. J. (2015). ZINC 15–ligand discovery for everyone. J. Chem. Inf. Model. 55 (11), 2324–2337. doi:10.1021/acs.jcim.5b00559
Stokes, J. M., Yang, K., Swanson, K., Jin, W., Cubillos-Ruiz, A., Donghia, N. M., et al. (2020). A deep learning approach to antibiotic discovery. Cell 180 (4), 688–702. e13. doi:10.1016/j.cell.2020.01.021
Stouch, T. R., Kenyon, J. R., Johnson, S. R., Chen, X. Q., Doweyko, A., and Li, Y. (2003). In silico ADME/tox: Why models fail. J. Comput. Aided. Mol. Des. 17 (2-4), 83–92. doi:10.1023/a:1025358319677
Stumpfe, D., Hu, H., and Bajorath, J. (2019). Evolving concept of activity cliffs. ACS Omega 4 (11), 14360–14368. doi:10.1021/acsomega.9b02221
Su, M., Yang, Q., Du, Y., Feng, G., Liu, Z., Li, Y., et al. (2018). Comparative assessment of scoring functions: The CASF-2016 update. J. Chem. Inf. Model. 59 (2), 895–913. doi:10.1021/acs.jcim.8b00545
Tetko, I. V., Engkvist, O., Koch, U., Reymond, J. L., and Chen, H. (2016). Bigchem: Challenges and opportunities for big data analysis in chemistry. Mol. Inf. 35 (11-12), 615–621. doi:10.1002/minf.201600073
Thakkar, A., Chadimová, V., Bjerrum, E. J., Engkvist, O., and Reymond, J. L. (2021). Retrosynthetic accessibility score (RAscore)\x{2013}rapid machine learned synthesizability classification from AI driven retrosynthetic planning. Chem. Sci. 12 (9), 3339–3349. doi:10.1039/d0sc05401a
Tietjen, I., Cassel, J., Register, E. T., Zhou, X. Y., Messick, T. E., Keeney, F., et al. (2021). The natural stilbenoid (-)-hopeaphenol inhibits cellular entry of SARS-CoV-2 USA-WA1/2020, B.1.1.7, and B.1.351 variants. Antimicrob. Agents Chemother. 65 (12), e0077221. doi:10.1128/AAC.00772-21
Tietjen, I., Ngwenya, B. N., Fotso, G., Williams, D. E., Simonambango, S., Ngadjui, B. T., et al. (2018). The Croton megalobotrys Müll Arg. Traditional medicine in HIV/AIDS management: Documentation of patient use, in vitro activation of latent HIV-1 provirus, and isolation of active phorbol esters. J. Ethnopharmacol. 211, 267–277. doi:10.1016/j.jep.2017.09.038
Tietjen, I., Ntie-Kang, F., Mwimanzi, P., Onguéné, P. A., Scull, M. A., Idowu, T. O., et al. (2015). Screening of the Pan-African natural product library identifies ixoratannin A-2 and boldine as novel HIV-1 inhibitors. PLoS One 10 (4), e0121099. doi:10.1371/journal.pone.0121099
Tropsha, A. (2010). Best practices for QSAR model development, validation, and exploitation. Mol. Inf. 29 (6-7), 476–488. doi:10.1002/minf.201000061
Tse, E. G., Aithani, L., Anderson, M., Cardoso-Silva, J., Cincilla, G., Conduit, G. J., et al. (2021). An open drug discovery competition: Experimental validation of predictive models in a series of novel antimalarials. J. Med. Chem. 64 (22), 16450–16463. doi:10.1021/acs.jmedchem.1c00313
UNESCO (2022). Fact sheet 59: Global investments in R&D. Available at: http://uis.unesco.org/sites/default/files/documents/fs59-global-investments-rd-2020-en.pdf [Accessed June, 2022].
Varadi, M., Anyango, S., Deshpande, M., Nair, S., Natassia, C., Yordanova, G., et al. (2022). AlphaFold protein structure database: Massively expanding the structural coverage of protein-sequence space with high-accuracy models. Nucleic Acids Res. 50 (1), D439–D444. doi:10.1093/nar/gkab1061
Wang, C., Wu, Q., Weimer, M., and Zhu, E. (2021). Flaml: A fast and lightweight AutoML library. Part Proc. Mach. Learn. Syst. 3, 434–447. doi:10.48550/arXiv.1911.04706
Waring, M. J., Arrowsmith, J., Leach, A. R., Leeson, P. D., Mandrell, S., Owen, R. M., et al. (2015). An analysis of the attrition of drug candidates from four major pharmaceutical companies. Nat. Rev. Drug Discov. 14 (7), 475–486. doi:10.1038/nrd4609
Warnat-Herresthal, S., Schultze, H., Shastry, K. L., Manamohan, S., Mukherjee, S., Garg, V., et al. (2021). Swarm Learning for decentralized and confidential clinical machine learning. Nature 594, 265–270. doi:10.1038/s41586-021-03583-3
WHO (2022). Health products in the pipeline from discovery to market launch for all diseases. Available at: https://www.who.int/observatories/global-observatory-on-health-research-and-development/monitoring/health-products-in-the-pipeline-from-discovery-to-market-launch-for-all-diseases [Accessed June, 2022].
Williams, A. J., Ekins, S., and Tkachenko, V. (2012). Towards a gold standard: Regarding quality in public domain chemistry databases and approaches to improving the situation. Drug Discov. Today 17 (13), 685–701. doi:10.1016/j.drudis.2012.02.013
Williamson, A. E., Ylioja, P. M., Robertson, M. N., Antonova-Koch, Y., Avery, V., Baell, J. B., et al. (2016). Open source drug discovery: Highly potent antimalarial compounds derived from the Tres Cantos arylpyrroles. ACS Cent. Sci. 2 (10), 687–701. doi:10.1021/acscentsci.6b00086
Winks, S., Woodland, J. G., Pillai, G., and Chibale, K. (2022). Fostering drug discovery and development in Africa. Nat. Med. 28, 1523–1526. doi:10.1038/s41591-022-01885-1
Wishart, D. S., Feunang, Y. D., Guo, A. C., Lo, E. J., Marcu, A., Grant, J. R., et al. (2018). DrugBank 5.0: A major update to the DrugBank database for 2018. Nucleic Acids Res. 46 (D1), D1074–D1082. doi:10.1093/nar/gkx1037
Wolf, T., Debut, L., Sanh, V., Chaumond, J., Delangue, C., Moi, A., et al. (2020). Transformers: State-of-the-Art natural language processing. In Proceedings of the 2020 Conference on Empirical Methods in Natural Language Processing: System Demonstrations, January 01, 2020. 38–45, Online. Association for Computational Linguistics.
Wu, Z., Ramsundar, B., Feinberg, E. N., Gomes, J., Geniesse, C., Pappu, A. S., et al. (2018). MoleculeNet: A benchmark for molecular machine learning. Chem. Sci. 9 (2), 513–530. doi:10.1039/c7sc02664a
Xiong, G., Wu, Z., Yi, J., Fu, L., Yang, Z., Hsieh, C., et al. (2021). ADMETlab 2.0: an integrated online platform for accurate and comprehensive predictions of ADMET properties. Nucleic Acids Res. 49 (W1), W5–W14. doi:10.1093/nar/gkab255
Yasgar, A., Jadhav, A., Simeonov, A., and Coussens, N. P. (2016). AlphaScreen-based assays: Ultra-high-throughput screening for small molecule inhibitors of challenging enzymes and protein-protein interactions. Methods Mol. Biol. 1439, 77–98. doi:10.1007/978-1-4939-3673-1_5
Yonchev, D., Dimova, D., Stumpfe, D., Vogt, M., and Bajorath, J. (2018). Redundancy in two major compound databases. Drug Discov. Today 23 (6), 1183–1186. doi:10.1016/j.drudis.2018.03.005
Zeng, X., Zhang, P., He, W., Qin, C., Chen, S., Tao, L., et al. (2018). Npass: Natural product activity and species source database for natural product research, discovery and tool development. Nucleic Acids Res. 46 (1), D1217–D1222. doi:10.1093/nar/gkx1026
Zeng, X., Zhang, P., Wang, Y., Qin, C., Chen, S., He, W., et al. (2019). Cmaup: A database of collective molecular activities of useful plants. Nucleic Acids Res. 47 (1), D1118–D1127. doi:10.1093/nar/gky965
Zhang, L., Tan, J., Han, D., and Zhu, H. (2017). From machine learning to deep learning: Progress in machine intelligence for rational drug discovery. Drug Discov. Today 22 (11), 1680–1685. doi:10.1016/j.drudis.2017.08.010
Keywords: antivirals, artificial intelligence, machine learning, drug discovery, low- and lower-middle-income countries
Citation: Namba-Nzanguim CT, Turon G, Simoben CV, Tietjen I, Montaner LJ, Efange SMN, Duran-Frigola M and Ntie-Kang F (2022) Artificial intelligence for antiviral drug discovery in low resourced settings: A perspective. Front. Drug. Discov. 2:1013285. doi: 10.3389/fddsv.2022.1013285
Received: 06 August 2022; Accepted: 05 October 2022;
Published: 02 November 2022.
Edited by:
José L Medina-Franco, National Autonomous University of Mexico, MexicoReviewed by:
Marcus Scotti, Federal University of Paraíba, BrazilAna Luisa Chávez Hernández, Department of Pharmacy, Faculty of Chemistry, National Autonomous University of Mexico, Mexico
Copyright © 2022 Namba-Nzanguim, Turon, Simoben, Tietjen, Montaner, Efange, Duran-Frigola and Ntie-Kang. This is an open-access article distributed under the terms of the Creative Commons Attribution License (CC BY). The use, distribution or reproduction in other forums is permitted, provided the original author(s) and the copyright owner(s) are credited and that the original publication in this journal is cited, in accordance with accepted academic practice. No use, distribution or reproduction is permitted which does not comply with these terms.
*Correspondence: Miquel Duran-Frigola, miquel@ersilia.io; Fidele Ntie-Kang, fidele.ntie-kang@ubuea.cm
†These could be considered equal contributors.