- 1DIBRIS – Università degli Studi di Genova, Genova, Italy
- 2E.O. Ospedali Galliera, Genova, Italy
The process of aging affects an individual’s potential in several dimensions, encompassing the physical, cognitive, psychological, economic, and social domains. The assessment of frailty in elderly patients is key to estimate their overall well-being and to predict mortality risk. In the clinical practice, frailty is usually estimated through medical tests and questionnaires performed sporadically. Continuous automatic assessment may help physicians in evaluating frailty by complementing their assessments with quantitative and non sporadic measurements. In this paper, we present the state-of-the-art in frailty evaluation, we summarize recent research achievements that could lead to an improved assessment, and we illustrate a case study we are conducting in our institution. Finally, based on our experience and results, we comment on the open challenges of automatic assessment of frailty.
1. Introduction
A human being is a complex organism, whose well-being may be described following several dimensions, encompassing the physical, cognitive, psychological, economic, and social domains. The process of aging typically reduces the individual’s potential in one or more of these domains, leading to a condition of vulnerability and clinical instability.
To highlight this condition, in the past decade, medical literature has introduced the definition of frail elderly, a fragile individual with an elevated risk of complications, that may result in loss of functional autonomy and in a high risk for adverse health outcomes, including hospitalization, institutionalization, and mortality (Fried et al., 2004).
As pointed out by Pilotto et al. (2008), a careful evaluation of the frailty is important to assess the overall well-being of the patient, to estimate the likelihood of functional loss, and to predict mortality risk. This evaluation is particularly important during hospital stay, since it is well known that hospitalization, especially if extended, may lead to new disabilities and may drastically deteriorate the risk of associated mortality [see Volpato et al. (2007) and Volpato et al. (2016) for an exhaustive analysis of this aspect].
Over the years, geriatricians developed different protocols to evaluate frailty — the main approaches will be reviewed in Section 2. These protocols are characterized by the same goal, but lacking a universally accepted standard, they follow different strategies (De Vries et al., 2011). Some of them are one-dimensional, as they focus on one specific domain (either cognitive or physical), other are multi-dimensional and take into account different domains, as discussed in Roppolo et al. (2015). In any case, all of them are sporadic, subjective, and can not be performed by a non-expert physician. Last, but not least, they suffer from bias due to the possible effect of patient’s anxiety toward medical tests and questionnaires.
Recently, with the advent of the assistive technologies, various approaches to the automatic analysis of a patient’s health status and behavior have been proposed. In Section 3, we will summarize the results of research carried out in the field, with a special reference to methods estimating automatically gait, behavior, motility, or activities of daily living, see for instance (Gianaria et al., 2016). In this paper, we discuss the potential of these approaches in the assessment of frailty. A core aspect of automatic methods is their objectivity, and the fact they allow for a better, or complementary analysis of human’s behavior. Moreover, automatic systems also have a potential for a continuous analysis of the patient, or at least for an assessment covering longer periods of time, which would improve the statistical significance of the outcomes (Cao et al., 2009; Zouba et al., 2010). As a further potential application, such automatic analysis could be carried out in homey environments, where the patient feels more comfortable, inducing a lower behavioral bias. These aspects will be discussed with the aim of identifying a longer term perspective of current research.
Since our analysis grounds on a study case, we are carrying out within the MoDiPro project, a prototype of a smart environment designed as a protected discharge facility. MoDiPro is located in E.O. Ospedali Galliera (Genova, Italy) with the goal of assessing the feasibility and acceptability of automatic and continuous assessment of frailty and overall well-being. In Section 4, we will describe the concept underlying the project, summarize meaningful results we have achieved so far on the continuous and non invasive evaluation of motility features, and future development, and highlight its potential on the automatic continuous assessment of frailty. Finally, in Section 5, we analyze the potential and the challenges the research community will have to face, both from the medical and the technological view point.
2. How Frailty is Evaluated by Geriatricians
One of the most commonly accepted operational definition of frailty is the classification proposed by Fried et al. (2001), where the authors define frailty as a clinical syndrome in which three or more of the following criteria are present: unintentional weight loss, exhaustion, decreased grip strength, slow gait speed, and low physical activity.
An accurate estimation of frailty of an elderly person is an important objective to assess the overall patient well-being and to predict the risk of mortality (Pilotto et al., 2008; Angleman et al., 2015). Moreover, a correct quantification of frailty is particularly useful in elderly patients after a prolonged hospital stay, as it can lead to the development of new disabilities and dramatically worsen the risk of mortality, as pointed out by Volpato et al. (2016) [see also Volpato et al. (2007)].
While it is common knowledge that an accurate estimation and follow-up of frailty is essential for assisting geriatric patients, a universally shared definition of how to evaluate it has not yet been reached. Over 20 very heterogeneous indices have been introduced in the literature (De Vries et al., 2011). As discussed in Azzopardi et al. (2016) and in Rockwood et al. (2015), most state-of-the-art tools to evaluate fragility are not homogeneous: many are simply dichotomous, other are more exhaustive and consider different aspects of the overall health status of a patient. We mention the Functional Domains tool (Strawbridge et al., 1998), the Frail Elderly Functional Assessment Questionnaire (Gloth et al., 1999), and the Groningen Frailty Indicator (Schuurmans et al., 2004). More recently, new multi-dimensional strategies were proposed, such as the multi-dimensional prognostic index (MPI) scores (Pilotto et al., 2008). All these variants have some limitations, such as the lack of objective unbiased measures based on performance tests. This lack of systematization makes frailty indices difficult to use in the clinical practice.
Current scores are based on episodic evaluations of several dimensions (clinical, cognitive, functional, nutritional, and social) and on multi-morbidity defined according to the International Classification of functioning, disability, and health.1
Motility deficits are assessed by medical staff through sporadic quantitative tests, such as the evaluation of the hand grip, the Time Up and Go test, and the 4 m walk test. The other dimensions are normally assessed through qualitative evaluations and patient’s self-reported questionnaires. The latter are being used more frequently in epidemiological studies, health service research, and in clinical trials to evaluate therapeutic interventions because they can capture the self-perception of the disease (Gobbens et al., 2010; Mulasso et al., 2016).
It is clear how defining a score based on quantitative and frequent observations over an extended period of time would improve the assessment of frailty and, possibly, provide a more reproducible and stable measure over time.
3. Advantages and Potential of Continuous Automatic Assessment
Recently, with the advent of assistive technologies and more effective data analysis tools, various approaches have been proposed that could be effectively used to provide an automatic assessment to frailty. Most of these approaches would also allow for a less sporadic and more objective evaluation. In this section, we summarize relevant research highlighting its potential benefit to the frailty index estimation.
A first line of research explored the possibility of providing physicians with automatic analysis tools to adopt their daily practice. The work of Greene et al. (2013) compares the performances in manually classifying frail or non-frail by means of the TUG (Time Up and Go) test and grip strength, with an automatic classification based on a regressor analyzing inertial sensor data acquired during TUG. The results show the benefits of adopting an automatic analysis. Gianaria et al. (2016) propose a method based on passive depth sensors (Kinect) and computational vision techniques to analyze the patient’s gait and infer information on motility. An automatic analysis of frailty, based on routine visits combined with the digital health record (EHR), was recently proposed by Clegg et al. (2016).
The benefit of an automatic system is that it would provide an alternative or additional information to the geriatrician and it could be used by the non-specialist/general practitioner for a less sporadic assessment on frailty.
A second line of research is the so-called smart environments that would allow to monitoring patients at home. Cao et al. (2009) present a context-aware system based on video analysis and a reasoning mechanism; Zouba et al. (2010) describe the prototype of a smart home equipped with cameras and environment sensors, Bathrinarayanan et al. (2013) evaluate an event recognition system based on video analysis. In all cases, the main effort is in recognizing actions and instrumental activities. They primarily focus on the functional domain, trying to detect anomalies with the goal of raising alarms.
The benefit of smart environments is that they may produce an up-to-date continuous analysis of the patient’s status, in a less stressful context. Moreover, the very large amount of data they gather may be the source for a more complex multi-dimensional analysis of the patient’s habits and behavior.
To summarize, automatic systems may participate in the health status assessment at different levels. Indeed, we may consider
• A continuous assessment of the patient at home, whose outcome is more robust as it relies on a longer term analysis, but it is harder to accept by the patient and the family and it is more complex to implement.
• An automatic, but not continuous analysis at the doctor’s office which may add an objective or complementary view, but it might be influenced by anxiety toward medical tests.
• A short-term continuous assessment during hospital stay that could be seen as a trade-off between pros and cons from the above alternatives, but has the limit of analyzing the patient in a not so comfortable setting, where stay in bed is encouraged and needed.
• A short-term continuous assessment in an ad hoc home-like hospital facility to be used for a short time (with a maximum of 3 or 4 days), where the patient is free to move and carry out common daily activities, and in the meanwhile the system analyses the patient considering multiple dimensions.
The latter is the possibility that appears to have a higher benefit in the overall evaluation of the patients, to the price of an initial investment in designing an ad hoc environment. In the next section, we will summarize the main features of the prototype system we developed as a consequence of the above analysis. We believe it may be a meaningful course of motivations to encourage scientists investing their research in that direction and medical staff to try and replicate the model in their institutions.
4. A Case Study: MoDiPro
In this section, we describe and discuss the MoDiPro (Modello Dimissione Protetta, Protected Discharge Model) facility hosted by E.O. Ospedali Galliera (Genova, Italy), which we present as a case study of a short-term continuous assessment system. A first important motivation of the project is the observation that prolonged hospital stays may worsen elderly patient’s health status and quality of life, not to mention they also cause a waste of economic and human resources (Lenzi et al., 2014). To this purpose, we have designed and developed a working prototype of a protected hospital-discharge facility, a comfortable two-bedroom apartment, whose long-term goal is to host patient’s prior discharge in a home-like environment. Here, patients may spend a few days being monitored automatically by sensors, while physicians and nurses can check on the patient remotely. The reduced presence of nurses and doctors will be, to some extent, balanced by the presence of sensors which monitor the patient’s activities and health measures. This concept would have a positive economic impact on the hospital infrastructure.
A second important aim of the project is to monitor the patient’s health status and independence (i.e., readiness to go back home), taking advantage of the time spent by the patient in the facility to carry out a further unobtrusive analysis of motility, activity of daily living and cognitive assessments. This analysis would be very useful. In particular, motility information could be added to the classical MPI, which is the reference index we adopt in our clinical practice. The concept implemented in MoDiPro is sketched in Figure 1. We carry out intelligent analysis of data streams provided by sensors installed in the facility, obtaining quantitative measurements that are added to the standard medical protocol. A first set of results on the analysis of RGB-D data have been published in Noceti et al. (2017). Today, we are testing the facility with volunteer users. The goal of these tests is to assess the robustness of our automatic motility assessment, and to evaluate the correlation with tests carried out by medical experts in their daily practice, in particular MPI (Multi-dimensional Prognostic Index), SPPB (Short Physical Performance Battery), TUG (Time Up and Go). The analysis considers the following measurements computed automatically over the whole observation period:
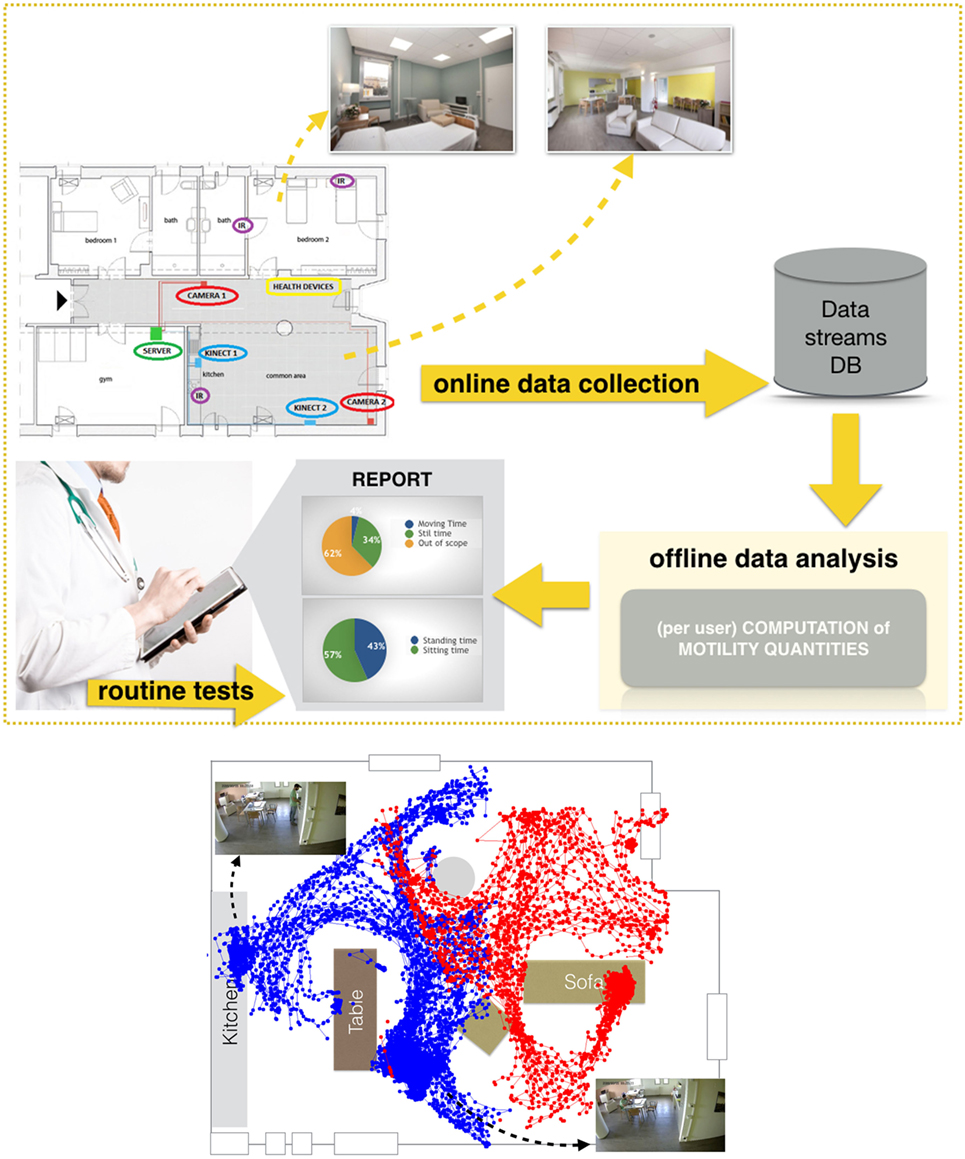
Figure 1. Top: MoDiPro facility and the generated data flow. Bottom: an example of a patient localization in the main hall, with data recorded by two RGB-D sensors—color coded in red and blue, respectively. (The volunteer depicted in the figure has agreed to participate in the study and signed a written informed consent for the publication of the image).
• Motility index (MI) describing the motility of a patient during the observation period; it is based on the overall time spent by walking/moving, the number of sit-to-stand transitions, the length of walks.
• Estimated maximum and average velocities of the walking instances in the observation period.
We have conducted two different experiments: a first one with an initial set of 5 volunteers we used for validating the system and fine tuning the parameters, and a second one with 15 test volunteers. All volunteers are active and healthy aging over 65 years old, with MPI ≤ 0.250. The same protocol has been followed for all subjects: each volunteer was first interviewed by geriatricians, who estimated MPI, SPPB, and TUG. After that, the volunteers spent at most 2 h in the facility, having the opportunity of moving, reading, watching television, having a slight snack or a drink. At the end of the first experiment, we computed a correlation analysis (Spearman test) and obtained the following results:
• MI and SPPB: Spearman coefficient = 0.95 p-val = 0.067
• MI and MPI: Spearman coefficient = 0.77, p-val = 0.14
• MI and hand grip strength: Spearman coefficient = 0.96 p-val = 0.008.
We notice a very high correlation between our motility index and the aggregate measurement SPPB and with one specific dimension (hand grip strength). We also observe a moderate correlation with MPI; this is not surprising, as MPI incorporates a wider class of dimensions which are not directly related with motility.
In the second set of experiments, we set the system parameters and the whole data stream was analyzed automatically. We obtained a much lower correlation between the automatic measurement and the standard ones (we report, for instance, a Spearman coefficient between MI and SPPB around 0.59). At a second inspection, we noticed that there is a significant degradation of the correlation results if we consider observation ranges below 30 min. If we restrict our analysis to patients spending at least 30 min in the facility, we obtain a Spearman coefficient between MI and SPPB equals to 0.90 with p-val = 0.012. It is more difficult to assess the reliability of our estimated velocities, as they are computed over a very heterogeneous and highly subjective set of activities, while the ones carried out by physicians are clearly associated with a specific request (e.g., walk along a line from a starting point to an end point). We report a correlation coefficient between the velocity associated with the TUG test and our estimated average velocity of about 0.71, p-val = 0.07 on both experiments.
The obtained results confirm the automatic measurements are meaningful, nicely correlated with medical tests, and can thus be used to assess motility between the sporadic medical evaluations.
5. Open Challenges of Continuous Automatic Assessment
From the analysis of current medical practice and recent research activities, including the outcomes of our MoDiPro project, we identify a set of open issues and suggestions for researchers interested in designing methods for a continuous automatic assessment of elderly patients and for physicians interested in adopting technological advanced solution.
• What are the minimal requirements? As it clearly emerged from our experimental analysis, for an effective continuous assessment of patients’ motility, the observation needs to be carried out for some time to be able to capture meaningful information and associate reliable estimates. To reduce the observation time, users should be encouraged to carry out activities. For instance, leaving a snack by the kitchen or fresh water in the fridge could encourage them to perform instrumental activities and walk across the room. Additionally, it would be very useful to create stimuli to obtain more reliable quantitative measurements (e.g., ring the door bell to guarantee the patient is going to walk toward a given, pre-defined direction: this would be useful for a reliable estimate of velocity).
• How to guarantee a good spatial-temporal coverage of the continuous assessment? A technical challenge one needs to face involves the complexity of analyzing day and night indoor environments of variable size and complexity. Redundant measurements, heterogeneous sensors, and multiple dimensions are an important aspect of our approach and should be enhanced and fully exploited; different sensors have different precision in space and time (e.g., cameras provide limited information during the night and no information in privacy protected areas such as toilettes), and some areas are more informative than others and thus would require an extra care, and possibly the coverage of multiple sources. For this reason, MoDiPro hosts a redundant set of devices which have been included in the apartment during its design: non-recording cameras, depth sensors, passive infra-red sensors, ambient and wearable localization sensors.
• How to merge and complement automatic continuous assessment and sporadic domain-expert analysis? Currently, the assessment provided by the continuous automatic analysis is provided as a separate report. As an insight for future research, it would be worth investigating that the benefit in integrating the output of manual tests within the automatic analysis procedure. This could be applied to both manual tests on frailty and cognitive status as well as other clinical observations.
• How to incorporate the patient’s specificities? Common practice in continuous data analysis is to set up a training phase during which the system is calibrated and tuned to meet the specificity of a given environment or situation. This would mean adjusting parameters and fine tuning the system to better reflect the user’s habits. This approach is appropriate in the case of a long-term analysis; it is instead less effective in short-term continuous evaluations, as in the case of MoDiPro. To mitigate this issue, one could exploit the doctor’s opinion after check in, to derive meaningful information on the patient’s abilities to be used as a guideline for the following automatic assessment.
• Can this model participate in a more general healthcare data collection? Healthcare data come from very heterogeneous sources. They may be structured, unstructured, and semi-structured data types, including laboratory results, medications prescriptions, and clinical observations, such as physician’s diagnosis, recommendations, discharge summaries, or operative reports. All these quantitative and qualitative data describe the patient from different points of view, but they are often difficult to integrate. The availability of state-of-the-art data analysis tools will provide, in the years to come, an up-to-date profile of the overall health status of the patient, to be shared among specialists, general practitioners, and families.
On this respect, the outcome an automatic assessment of frailty could be a meaningful complementary part of this general picture, providing additional information and automatic measurements, for a better tailored treatment and follow-up.
Ethics Statement
The MoDiPro project has been approved by the Comitato Etico Regionale – Regione Liguria (Regional Ethical Committee – Liguria Region, Italy). All participants to the study accepted and signed a written informed consent.
Author Contributions
AB, AV, FO, GR, and AP contributed to the conception and design of the study. CM and AC performed the statistical analysis. CM performed the design and implementation of the numerical tests. AC took care of the clinical protocol and tests. FO wrote the first draft of the manuscript. CM, AB and FO wrote sections of the manuscript. All authors contributed to manuscript revision, read and approved the submitted version.
Conflict of Interest Statement
The authors declare that the research was conducted in the absence of any commercial or financial relationships that could be construed as a potential conflict of interest.
Acknowledgments
Authors are indebted to Nicoletta Noceti for useful discussions.
Footnote
References
Angleman, S.B., Santoni, G., Pilotto, A., Fratiglioni, L., Welmer, A.-K., Investigators, M.P., et al. (2015). Multidimensional prognostic index in association with future mortality and number of hospital days in a population-based sample of older adults: results of the EU funded MPI_AGE project. Plos One 10:e0133789. doi: 10.1371/journal.pone.0133789
Azzopardi, R.V., Vermeiren, S., Gorus, E., Habbig, A.-K., Petrovic, M., Van Den Noortgate, N., et al. (2016). Linking frailty instruments to the international classification of functioning, disability, and health: a systematic review. Journal of the American Medical Directors Association 17: 1066–e1. doi:10.1016/j.jamda.2016.07.023
Bathrinarayanan, V., Fosty, B., Konig, A., Romdhane, R., Thonnat, M., Bremond, F., et al. (2013). Evaluation of a monitoring system for event recognition of older people. In 2013 10th IEEE International Conference on Advanced Video and Signal Based Surveillance (AVSS), Krakow, Poland, 165–170.
Cao, Y., Tao, L., and Xu, G. (2009). An event-driven context model in elderly health monitoring. In 2009. UIC-ATC’09. Symposia and Workshops on Ubiquitous, Autonomic and Trusted Computing (Brisbane, Australia: IEEE), 120–124.
Clegg, A., Bates, C., Young, J., Ryan, R., Nichols, L., Teale, E.A., et al. (2016). Development and validation of an electronic frailty index using routine primary care electronic health record data. Age and Ageing 45: 353–60. doi:10.1093/ageing/afw039
De Vries, N., Staal, J., Van Ravensberg, C., Hobbelen, J., Rikkert, M.O., and Nijhuis-Van der Sanden, M. (2011). Outcome instruments to measure frailty: a systematic review. Ageing Research Reviews 10: 104–14. doi:10.1016/j.arr.2010.09.001
Fried, L.P., Ferrucci, L., Darer, J., Williamson, J.D., and Anderson, G. (2004). Untangling the concepts of disability, frailty, and comorbidity: implications for improved targeting and care. The Journals of Gerontology Series A: Biological Sciences and Medical Sciences 59: M255–63. doi:10.1093/gerona/59.3.M255
Fried, L.P., Tangen, C.M., Walston, J., Newman, A.B., Hirsch, C., Gottdiener, J., et al. (2001). Frailty in older adults: evidence for a phenotype. The Journals of Gerontology Series A: Biological Sciences and Medical Sciences 56: M146–57. doi:10.1093/gerona/56.3.M146
Gianaria, E., Grangetto, M., Roppolo, M., Mulasso, A., and Rabaglietti, E. (2016). Kinect-based gait analysis for automatic frailty syndrome assessment. In 2016 IEEE International Conference on Image Processing (ICIP) (Phoenix, USA: IEEE), 1314–1318.
Gloth, F., Scheme, A.A., Shah, S., Ashton, R., and McKinney, R. (1999). The frail elderly functional assessment questionnaire: its responsiveness and validity in alternative settings. Archives of Physical Medicine and Rehabilitation 80: 1572–6. doi:10.1016/S0003-9993(99)90332-5
Gobbens, R., van Assen, M., Luijkx, K., Wijnen-Sponselee, M., and Schols, J. (2010). The Tilburg frailty indicator: psychometric properties. Journal American Medical Directors Association 11: 344–55. doi:10.1016/j.jamda.2009.11.003
Greene, B.R., Doheny, E.P., O’Halloran, A., and Kenny, R.A. (2013). Frailty status can be accurately assessed using inertial sensors and the tug test. Age and Ageing 43: 406–11. doi:10.1093/ageing/aft176
Lenzi, J., Mongardi, M., Rucci, P., Di Ruscio, E., Vizioli, M., Randazzo, C., et al. (2014). Sociodemographic, clinical and organisational factors associated with delayed hospital discharges: a cross-sectional study. BMC Health Services Research 14:1. doi:10.1186/1472-6963-14-128
Mulasso, A., Roppolo, M., Gobbens, R.J., and Rabaglietti, E. (2016). The Italian version of the Tilburg frailty indicator: analysis of psychometric properties. Research on Aging 38: 842–63. doi:10.1177/0164027515606192
Noceti, N., Chessa, M., Martini, C., Solari, F., and Odone, F. (2017). Design of Assistive Tools for the Market. Computer Vision and Pattern Recognition Series (Elsevier).
Pilotto, A., Ferrucci, L., Franceschi, M., D’Ambrosio, L.P., Scarcelli, C., Cascavilla, L., et al. (2008). Development and validation of a multidimensional prognostic index for one-year mortality from comprehensive geriatric assessment in hospitalized older patients. Rejuvenation Research 11: 151–61. doi:10.1089/rej.2007.0569
Rockwood, K., Theou, O., and Mitnitski, A. (2015). What are frailty instruments for? Age and Ageing 44: 545–7. doi:10.1093/ageing/afv043
Roppolo, M., Mulasso, A., Gobbens, R., Mosso, C., and Rabaglietti, E. (2015). A comparison between uni- and multidimensional frailty measures: prevalence, functional status, and relationships with disability. Clinical Interventions in Aging 10: 1669–78. doi:10.2147/CIA.S92328
Schuurmans, H., Steverink, N., Lindenberg, S., Frieswijk, N., and Slaets, J. (2004). Old or frail: what tells us more? The Journals of Gerontology Series A: Biological Sciences and Medical Sciences 59: M962–5. doi:10.1093/gerona/59.9.M962
Strawbridge, W., Shema, S., Balfour, J., Higby, H., and GA, K. (1998). Antecedents of frailty over three decades in an older cohort. The Journal Gerontology Series B: Psychology Sciences and Social Sciences 53: S9–16.
Volpato, S., Daragjati, J., Simonato, M., Fontana, A., Ferrucci, L., and Pilotto, A. (2016). Change in the multidimensional prognostic index score during hospitalization in older patients. Rejuvenation Research 19: 244–51. doi:10.1089/rej.2015.1715
Volpato, S., Onder, G., Cavalieri, M., Guerra, G., Sioulis, F., Maraldi, C., et al. (2007). Characteristics of nondisabled older patients developing new disability associated with medical illnesses and hospitalization. Journal of General Internal Medicine 22: 668–74. doi:10.1007/s11606-007-0152-1
Keywords: frailty index, assistive technologies, smart environments, continuous automatic assessment, motility index, computational vision
Citation: Martini C, Barla A, Odone F, Verri A, Cella A, Rollandi GA and Pilotto A (2018) Data-Driven Continuous Assessment of Frailty in Older People. Front. Digit. Humanit. 5:6. doi: 10.3389/fdigh.2018.00006
Received: 15 December 2017; Accepted: 08 March 2018;
Published: 17 April 2018
Edited by:
Enrico Capobianco, University of Miami, United StatesReviewed by:
Gokarna Sharma, Kent State University, United StatesJuan Julián Merelo, University of Granada, Spain
Copyright: © 2018 Martini, Barla, Odone, Verri, Cella, Rollandi and Pilotto. This is an open-access article distributed under the terms of the Creative Commons Attribution License (CC BY). The use, distribution or reproduction in other forums is permitted, provided the original author(s) and the copyright owner are credited and that the original publication in this journal is cited, in accordance with accepted academic practice. No use, distribution or reproduction is permitted which does not comply with these terms.
*Correspondence: Francesca Odone, ZnJhbmNlc2NhLm9kb25lJiN4MDAwNDA7dW5pZ2UuaXQ=