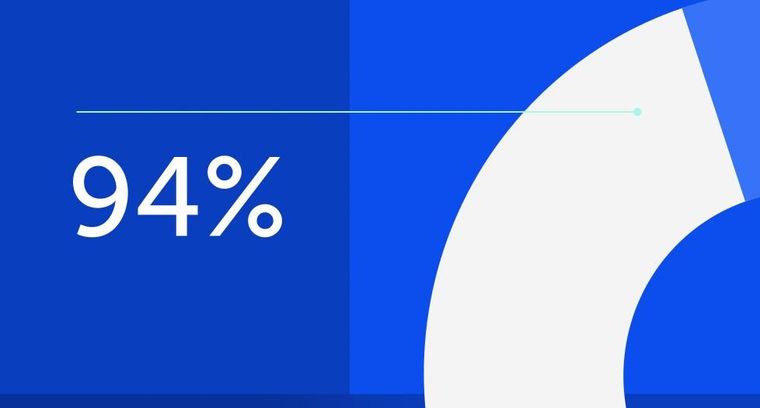
94% of researchers rate our articles as excellent or good
Learn more about the work of our research integrity team to safeguard the quality of each article we publish.
Find out more
ORIGINAL RESEARCH article
Front. Digit. Health
Sec. Health Informatics
Volume 7 - 2025 | doi: 10.3389/fdgth.2025.1576290
This article is part of the Research TopicPrivacy Enhancing Technology: a Top 10 Emerging Technology to Revolutionize HealthcareView all articles
The final, formatted version of the article will be published soon.
Select one of your emails
You have multiple emails registered with Frontiers:
Notify me on publication
Please enter your email address:
If you already have an account, please login
You don't have a Frontiers account ? You can register here
The generation of synthetic tabular data has emerged as a key privacy-enhancing technology to address challenges in data sharing, particularly in healthcare, where sensitive attributes can compromise patient privacy. Despite significant progress, balancing fidelity, utility, and privacy in complex medical datasets remains a substantial challenge. This paper introduces a comprehensive and holistic evaluation framework for synthetic tabular data, consolidating metrics and privacy risk measures across three key categories (fidelity, utility and privacy) and incorporating a fidelity-utility tradeoff metric. The framework was applied to three open-source medical datasets to evaluate synthetic tabular data generated by five generative models, both with and without differential privacy. Results showed that simpler models generally achieved better fidelity and utility, while more complex models provided lower privacy risks. The addition of differential privacy enhanced privacy preservation but often reduced fidelity and utility, highlighting the complexity of balancing fidelity, utility and privacy in synthetic data generation for medical datasets. Despite its contributions, this study acknowledges limitations, such as the lack of evaluation metrics neither privacy risk measures for required model training time and resource usage, reliance on default model parameters, and the assessment of models that incorporates differential privacy with only a single privacy budget. Future work should explore parameter optimization, alternative privacy mechanisms, broader applications of the framework to diverse datasets and domains, and collaborations with clinicians for clinical utility evaluation. This study provides a foundation for improving synthetic tabular data evaluation and advancing privacy-preserving data sharing in healthcare.
Keywords: Synthetic data generation, Generative models, synthetic data fidelity, synthetic data utility, privacy risk measureattacks, Synthetic data evaluation, Differential privacy, synthetic data privacy
Received: 13 Feb 2025; Accepted: 09 Apr 2025.
Copyright: © 2025 Hernandez, Osorio-Marulanda, Catalina, Loinaz, Epelde and Aginako. This is an open-access article distributed under the terms of the Creative Commons Attribution License (CC BY). The use, distribution or reproduction in other forums is permitted, provided the original author(s) or licensor are credited and that the original publication in this journal is cited, in accordance with accepted academic practice. No use, distribution or reproduction is permitted which does not comply with these terms.
* Correspondence: Mikel Hernandez, Digital Health and Biomedical Technologies, Vicomtech, San Sebastian, Spain
Disclaimer: All claims expressed in this article are solely those of the authors and do not necessarily represent those of their affiliated organizations, or those of the publisher, the editors and the reviewers. Any product that may be evaluated in this article or claim that may be made by its manufacturer is not guaranteed or endorsed by the publisher.
Supplementary Material
Research integrity at Frontiers
Learn more about the work of our research integrity team to safeguard the quality of each article we publish.