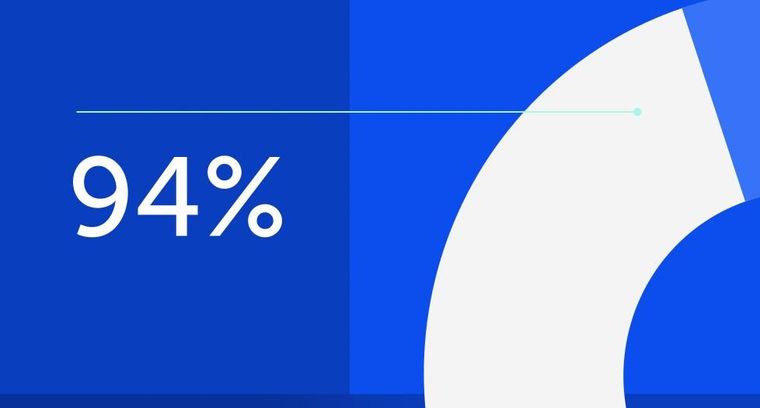
94% of researchers rate our articles as excellent or good
Learn more about the work of our research integrity team to safeguard the quality of each article we publish.
Find out more
BRIEF RESEARCH REPORT article
Front. Digit. Health
Sec. Health Technology Implementation
Volume 7 - 2025 | doi: 10.3389/fdgth.2025.1569554
The final, formatted version of the article will be published soon.
Select one of your emails
You have multiple emails registered with Frontiers:
Notify me on publication
Please enter your email address:
If you already have an account, please login
You don't have a Frontiers account ? You can register here
Background: Concise synopses of clinical evidence support treatment decision-making but are time-consuming to curate. Large language models (LLMs) offer potential but they may provide inaccurate information. We objectively assessed the abilities of four commercially available LLMs to generate synopses for six treatment regimens in multiple myeloma and amyloid light chain (AL) amyloidosis. Methods: We compared the performance of four LLMs: Claude 3.5, ChatGPT 4.0; Gemini 1.0 and Llama-3.1. Each LLM was prompted to write synopses for six regimens. Two hematologists independently assessed accuracy, completeness, relevance, clarity, coherence, and hallucinations using Likert scales. Mean scores with 95% confidence intervals (CI) were calculated across all domains and inter-rater reliability was evaluated using Cohen’s quadratic weighted kappa. Results: Claude demonstrated the highest performance in all domains, outperforming the other LLMs in accuracy: mean Likert score 3.92 (95% CI 3.54-4.29); ChatGPT 3.25 (2.76-3.74); Gemini 3.17 (2.54-3.80); Llama 1.92 (1.41-2.43);completeness: mean Likert score 4.00 (3.66-4.34); GPT 2.58 (2.02-3.15); Gemini 2.58 (2.02-3.15); Llama 1.67 (1.39-1.95); and extentofhallucinations: mean Likert score 4.00 (4.00-4.00); ChatGPT 2.75 (2.06-3.44); Gemini 3.25 (2.65-3.85); Llama 1.92 (1.26-2.57). Llama performed considerably poorer across all the studied domains. ChatGPT and Gemini had intermediate performance. Notably, none of the LLMs registered perfect accuracy, completeness, or relevance. Conclusion: Claude performed at a consistently higher level than other LLMs, all tested LLMs required careful editing from a domain expert to become usable. More time will be needed to determine the suitability of LLMsto independently generate clinical synopses.
Keywords: Conceptualization, supervision, Writingoriginal draft, Writingreview & editing. Aleenah Mohsin: Methodology, Writingreview & editing. Jeremy L. Warner: Writingoriginal draft, Writingreview & editing. Mary Kwok: Writing original draft, Writingreview & editing. Peter Yang: Writingoriginal draft, Writingreview & editing. Rahul Banerjee: Methodology
Received: 07 Feb 2025; Accepted: 14 Apr 2025.
Copyright: © 2025 Rubinstein, Mohsin, Banerjee, Ma, Mishra, Kwok, Yang, Warner and Cowan. This is an open-access article distributed under the terms of the Creative Commons Attribution License (CC BY). The use, distribution or reproduction in other forums is permitted, provided the original author(s) or licensor are credited and that the original publication in this journal is cited, in accordance with accepted academic practice. No use, distribution or reproduction is permitted which does not comply with these terms.
* Correspondence: Andrew J. Cowan, University of Washington, Seattle, 98195-4550, Washington, United States
Disclaimer: All claims expressed in this article are solely those of the authors and do not necessarily represent those of their affiliated organizations, or those of the publisher, the editors and the reviewers. Any product that may be evaluated in this article or claim that may be made by its manufacturer is not guaranteed or endorsed by the publisher.
Supplementary Material
Research integrity at Frontiers
Learn more about the work of our research integrity team to safeguard the quality of each article we publish.