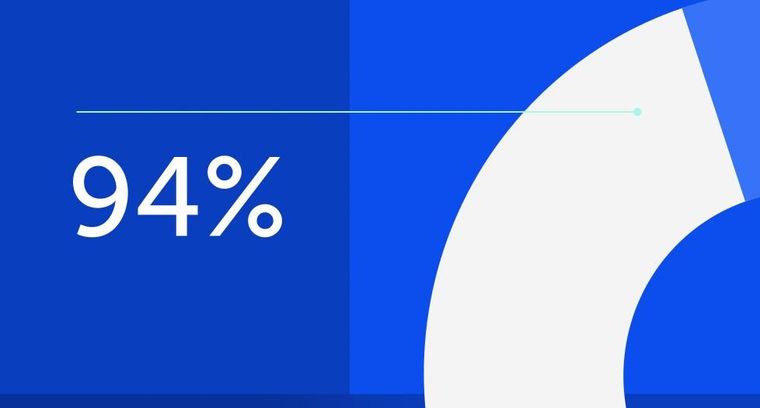
94% of researchers rate our articles as excellent or good
Learn more about the work of our research integrity team to safeguard the quality of each article we publish.
Find out more
ORIGINAL RESEARCH article
Front. Digit. Health
Sec. Health Technology Implementation
Volume 7 - 2025 | doi: 10.3389/fdgth.2025.1566138
This article is part of the Research TopicAdvances in Artificial Intelligence Transforming the Medical and Healthcare SectorsView all 11 articles
The final, formatted version of the article will be published soon.
Select one of your emails
You have multiple emails registered with Frontiers:
Notify me on publication
Please enter your email address:
If you already have an account, please login
You don't have a Frontiers account ? You can register here
Minimally invasive cholecystectomy is one of the most frequently performed surgical procedures. However, iatrogenic injury related to hepatocystic triangle anatomy can occur even among experienced clinicians; therefore, an objective method can help in preventing this damage during cholecystectomy. The purpose of this study was to develop an image recognition model using the deep learning method to identify the hepatocystic triangle through indocyanine green (ICG) based near-infrared cholangiography (NIRC) during minimally invasive cholecystectomy Methods: Prediction of the object landmark (hepatocystic triangle) was evaluated using the YOLOv5 S model, an algorithm for real-time object detection in computer vision. We extracted 3,796 images from 200 cholecystectomy videos and divided them into 2979 training and 817 validation datasets. To annotate the process, we overlayed original and ICG images and estimated image prediction to identify the target, i.e., the hepatocystic triangle.When predicting landmarks, the YOLO model outlines the bounding boxes on each landmark against the image files.Results: Using the non-maximum suppression (NMS) algorithm, model performance changes according to the intersection over union (IoU) threshold; this high level of IoU threshold (0.7-0.9) results in duplicate predictions. The optimal IoU of NMS was 0.6 in multiple experiments, and the average precision score was 0.859.We successfully developed an image prediction model using intraoperative ICG NIRC to prevent bile duct damage during cholecystectomy. This experiment is a study based on the location of the actual hepatocystic triangle, and its findings are expected to be helpful in clinical practice because it can predict the bile duct before tissue dissection.
Keywords: artificial intelligence, laparoscopic cholecystectomy, Robot cholecystectomy, Indocyanine green cholangiography, Image recognition system
Received: 24 Jan 2025; Accepted: 17 Mar 2025.
Copyright: © 2025 Hou, Yoo, Park, Lee, Cho, Park, Shin and Kwon. This is an open-access article distributed under the terms of the Creative Commons Attribution License (CC BY). The use, distribution or reproduction in other forums is permitted, provided the original author(s) or licensor are credited and that the original publication in this journal is cited, in accordance with accepted academic practice. No use, distribution or reproduction is permitted which does not comply with these terms.
* Correspondence: Tae Yoo, Department of surgery, Hallym University Dongtan Sacred Heart Hospital, Hwaseong, Republic of Korea
Disclaimer: All claims expressed in this article are solely those of the authors and do not necessarily represent those of their affiliated organizations, or those of the publisher, the editors and the reviewers. Any product that may be evaluated in this article or claim that may be made by its manufacturer is not guaranteed or endorsed by the publisher.
Supplementary Material
Research integrity at Frontiers
Learn more about the work of our research integrity team to safeguard the quality of each article we publish.