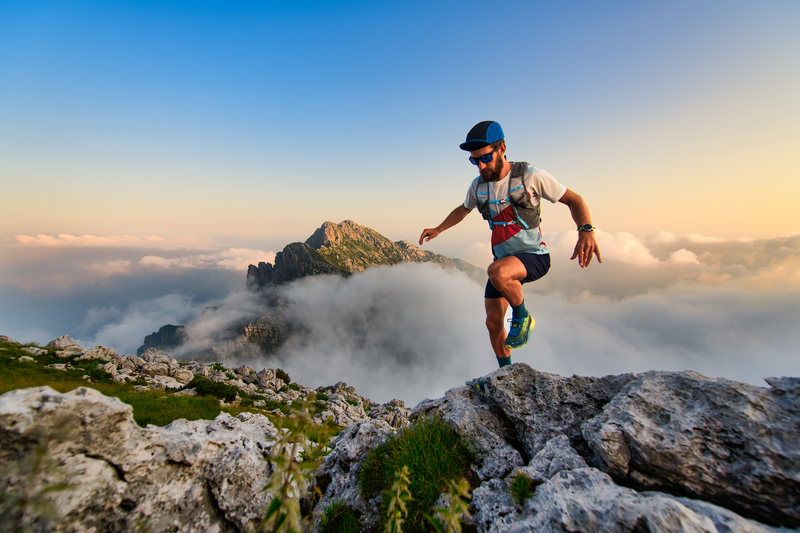
95% of researchers rate our articles as excellent or good
Learn more about the work of our research integrity team to safeguard the quality of each article we publish.
Find out more
ORIGINAL RESEARCH article
Front. Digit. Health
Sec. Health Informatics
Volume 7 - 2025 | doi: 10.3389/fdgth.2025.1558589
This article is part of the Research Topic Artificial Intelligence in Cardiovascular Research View all 4 articles
The final, formatted version of the article will be published soon.
You have multiple emails registered with Frontiers:
Please enter your email address:
If you already have an account, please login
You don't have a Frontiers account ? You can register here
Background: 12-lead electrocardiograms (ECGs) are a cornerstone for diagnosing and monitoring cardiovascular diseases (CVDs). They play a key role in detecting abnormalities such as arrhythmias and myocardial infarction, enabling early intervention and risk stratification.However, traditional analysis relies heavily on manual interpretation, which is time-consuming and expertise-dependent. Moreover, existing machine learning models often lack personalization, as they fail to integrate subject-specific anatomical and demographic information. Advances in deep generative models offer an opportunity to overcome these challenges by synthesizing personalized ECGs and extracting clinically relevant features for improved risk assessment.We propose a conditional Variational Autoencoder (cVAE) framework to generate realistic, subject-specific 12-lead ECGs by incorporating demographic metadata, anatomical heart features, and ECG electrodes' positions as conditioning factors. This allows for physiologically consistent and personalized ECG synthesis. Furthermore, we introduce a revised Cox proportional-hazards regression model that utilizes the latent embeddings learned by the cVAE to predict future CVD risk. This approach not only enhances the interpretability of ECG-derived risk factors but also demonstrates the potential of deep generative models in personalized cardiac assessment.: Our model is trained and validated on the UK Biobank dataset and in silico simulation data. By incorporating heart position and electrodes' positions, the generated ECGs demonstrate strong consistency with in silico simulations, providing insights into the relationship between cardiac anatomy and ECG morphology. Furthermore, our CVD risk prediction model achieves a Cindex of 0.65, indicating that ECG signals, together with demographic and anatomical information, contain valuable prognostic information for stratifying subjects based on future cardiovascular risk.This work marks a significant advancement in ECG analysis by providing a conditional VAE framework that not only improves ECG generation but also enriches our 1 Sang et al.understanding of the relationship between ECG patterns and subject-specific information.Importantly, our approach enables clinically significant information to be extracted from 12-lead ECGs, providing valuable insights for predicting future CVD risks.
Keywords: Cardiac MRI, cardiovascular disease, Cardiovascular risk prediction, ECG electrodes, ECG Generation, Variational autoencoder
Received: 10 Jan 2025; Accepted: 25 Mar 2025.
Copyright: © 2025 Sang, Banerjee, Beetz and Grau. This is an open-access article distributed under the terms of the Creative Commons Attribution License (CC BY). The use, distribution or reproduction in other forums is permitted, provided the original author(s) or licensor are credited and that the original publication in this journal is cited, in accordance with accepted academic practice. No use, distribution or reproduction is permitted which does not comply with these terms.
* Correspondence:
Abhirup Banerjee, Institute of Biomedical Engineering, Department of Engineering Science, Mathematical, Physical and Life Sciences Division, University of Oxford, Oxford, United Kingdom
Disclaimer: All claims expressed in this article are solely those of the authors and do not necessarily represent those of their affiliated organizations, or those of the publisher, the editors and the reviewers. Any product that may be evaluated in this article or claim that may be made by its manufacturer is not guaranteed or endorsed by the publisher.
Research integrity at Frontiers
Learn more about the work of our research integrity team to safeguard the quality of each article we publish.