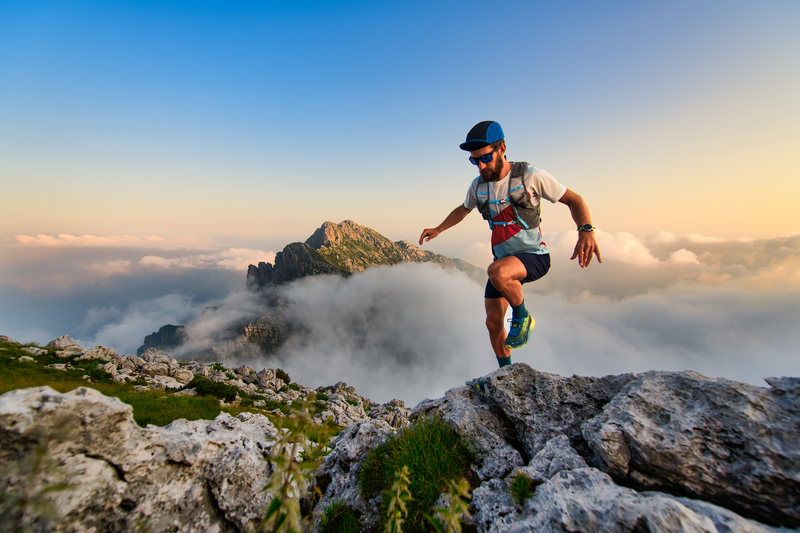
95% of researchers rate our articles as excellent or good
Learn more about the work of our research integrity team to safeguard the quality of each article we publish.
Find out more
SYSTEMATIC REVIEW article
Front. Digit. Health , 27 March 2025
Sec. Health Technology Implementation
Volume 7 - 2025 | https://doi.org/10.3389/fdgth.2025.1557467
This article is part of the Research Topic Advances in Artificial Intelligence Transforming the Medical and Healthcare Sectors View all 10 articles
Background: Type 2 Diabetes Mellitus (T2DM) remains a critical global health challenge, necessitating robust predictive models to enable early detection and personalized interventions. This study presents a comprehensive bibliometric and systematic review of 33 years (1991-2024) of research on machine learning (ML) and artificial intelligence (AI) applications in T2DM prediction. It highlights the growing complexity of the field and identifies key trends, methodologies, and research gaps.
Methods: A systematic methodology guided the literature selection process, starting with keyword identification using Term Frequency-Inverse Document Frequency (TF-IDF) and expert input. Based on these refined keywords, literature was systematically selected using PRISMA guidelines, resulting in a dataset of 2,351 articles from Web of Science and Scopus databases. Bibliometric analysis was performed on the entire selected dataset using tools such as VOSviewer and Bibliometrix, enabling thematic clustering, co-citation analysis, and network visualization. To assess the most impactful literature, a dual-criteria methodology combining relevance and impact scores was applied. Articles were qualitatively assessed on their alignment with T2DM prediction using a four-point relevance scale and quantitatively evaluated based on citation metrics normalized within subject, journal, and publication year. Articles scoring above a predefined threshold were selected for detailed review. The selected literature spans four time periods: 1991–2000, 2001–2010, 2011–2020, and 2021–2024.
Results: The bibliometric findings reveal exponential growth in publications since 2010, with the USA and UK leading contributions, followed by emerging players like Singapore and India. Key thematic clusters include foundational ML techniques, epidemiological forecasting, predictive modelling, and clinical applications. Ensemble methods (e.g., Random Forest, Gradient Boosting) and deep learning models (e.g., Convolutional Neural Networks) dominate recent advancements. Literature analysis reveals that, early studies primarily used demographic and clinical variables, while recent efforts integrate genetic, lifestyle, and environmental predictors. Additionally, literature analysis highlights advances in integrating real-world datasets, emerging trends like federated learning, and explainability tools such as SHAP (SHapley Additive exPlanations) and LIME (Local Interpretable Model-agnostic Explanations).
Conclusion: Future work should address gaps in generalizability, interdisciplinary T2DM prediction research, and psychosocial integration, while also focusing on clinically actionable solutions and real-world applicability to combat the growing diabetes epidemic effectively.
Diabetes Mellitus is a chronic disease that has potentially fatal consequences if left undetected. It has the potential to lead to serious illness, such as kidney failure, sight loss and limb amputations and even fatal consequences if not detected and treated effectively (1). The disease has affected millions of people worldwide, and its prevalence is expected to surge in the future given that ageing and obesity are major risk factors and both are rising.
Diabetes can be broadly classified into two main types, namely Type 1 Mellitus (T1DM) and Type 2 Diabetes Mellitus (T2DM). T1DM is caused by the autoimmune destruction of insulin-producing pancreatic cells, leading to insulin deficiency and chronic hyperglycemia, usually affecting children or teenagers. T2DM is a chronic metabolic disorder with a number of causal factors, including genetic predisposition and lifestyle factors, such as poor diet, a lack of physical activity, high blood pressure, and obesity. While both T1DM and T2DM are serious conditions requiring ongoing management, T2DM is more common and is largely preventable with early detection and lifestyle interventions (2).
According to the latest estimates from the International Diabetes Federation (3), 537 million people worldwide had diabetes in 2021, and this number is projected to rise to 643 million by 2030 and 783 million by 2045. The same report predicts that 541 million adults worldwide are at increased risk of developing T2DM. The increasing prevalence of diabetes is a major public health concern and emphasizes the need for effective and smart prediction, prevention, and management strategies.
Machine learning (ML) models and artificial intelligence (AI) have great potential in developing personalized prediction systems for diabetes. Scientists have leveraged ML and data mining techniques in several research areas related to diabetes, including identifying diagnostic and predictive factors in diabetes development, predicting diabetes, analyzing diabetic complications, developing drugs and therapies for diabetes, and studying the impact of genetic and environmental factors on the onset and progression of diabetes (4). By analyzing vast amounts of diabetes-related data, ML models can transform raw information into invaluable knowledge, unlocking new avenues for more effective prognosis, diagnosis, and treatment of diabetes (5, 6).
In recent years, several survey articles have explored the use of ML models and AI in diabetes research. Some of these reviews have examined the application of ML tools across various diabetes-related domains (4). Others have taken a more targeted approach, focusing on specific areas such as diabetes detection (7, 8), diabetes management (9, 10), or diabetes prediction (11, 12). These reviews offer valuable insights into the application of ML and AI in diabetes management and prognosis.
However, with the recent surge in publications related to ML and AI in diabetes research, conducting a bibliometric analysis of the literature can provide several valuable insights, including publication trends, research hotspots, geographical distribution, collaboration networks, journal analysis, methodological trends, funding and support, thematic clusters, and gaps and opportunities. Therefore, several bibliometric studies have also been conducted in the diabetes field, focusing on various aspects and research domains. For instance, (13) conducted a bibliometric analysis of diabetes prediction (in general) using ML algorithms. This study focused on a 12-year period (2009–2020), provided a snapshot of recent trends, and emphasized publication trends while identifying the leading countries and journals. Another bibliometric study (14) examined the growth of literature in the field of diabetes (in general) by utilizing data from the MEDLINE database for the period 1995–2004. The study aimed to identify the core journals in this field during that time. The authors in (15) conducted a bibliometric analysis to identify, visualize, and characterize meta-analyses on diabetic foot ulcer research, focusing on treatment approaches, risk factor analysis, and economic evaluations. This study covered publications from 1999 to 2022, with data retrieved from the Web of Science (WoS) core collection database. The authors in (16) performed a bibliometric analysis of research papers published in the field of ML and deep learning (DL) techniques applied to diabetes research (in general) from 2000 to 2022 (22 years). The articles were categorized into detection, prediction, and management. This involved the statistical analysis of published literature to identify global research trends and networks, highlighting key countries, institutions, journals, articles, citations, and research topics.
Despite the valuable insights provided by these studies, several limitations highlight the need for further investigation.
• Firstly, many existing studies (13, 14, 16) tend to focus on diabetes as a whole, without distinguishing between T1DM and T2DM. These are distinct research areas with unique pathophysiologies, management strategies, and challenges, and a broad approach often overlooks the nuanced priorities specific to each type.
• Secondly, the variability in the time periods covered by these studies, with some focusing on relatively short durations, limits the ability to derive comprehensive longitudinal insights into the evolution of research trends.
• Thirdly, while robust bibliometric techniques are applied, these studies often lack detailed analysis of thematic clusters and the methodological advancements that have occurred over time, which are critical for understanding shifts in research paradigms.
• Lastly, there is a notable gap in the literature regarding targeted analyses of machine learning prediction models for T2DM, as much of the focus has been on complications or broader applications of machine learning in diabetes research. These gaps highlight the need for a more focused and nuanced approach in future bibliometric analyses.
To address these gaps, this research conducted a comprehensive bibliometric and literature analysis of the T2DM prediction research using ML and AI over a 33-year period (1991–2024). To the best of our knowledge, this is the first bibliometric and literature analysis focused on the prediction of T2DM using ML and AI techniques. We offer a historical perspective and trace the evolution of research methodologies, utilizing more advanced bibliometric tools such as Bibliometrics. Specifically, the study seeks to map the intellectual structure through co-citation network analysis, identify and analyze distinct thematic clusters, assess the contributions and centrality of different ML methodologies, and highlight the foundational and applied research in the field. Our analysis delves deeper into methodological evolution, datasets utilized, most influential key features to train the ML models, and future research directions, with a particular emphasis on interdisciplinary approaches and emerging technologies.
The objectives of this study are as follows:
1. Publication trends, citation analysis, and global collaboration patterns: To analyze publication and citation trends over time, and explore international collaboration patterns and key countries’ roles.
2. Thematic clusters: To identify thematic clusters in T2DM research using ML. Summarize each cluster’s focus and centrality, assess the impact of ML models on prediction accuracy.
3. Foundational methods, datasets, and key predictors: To evaluate the foundational methods, datasets and predominant predictors used in T2DM prediction research, particularly focusing on ML algorithms and their effectiveness.
4. Research gaps, emerging trends, and future directions: To identify research gaps and analyze emerging trends in methodologies for predicting T2DM over decades. Highlight challenges and suggest future research directions.
This study aims to evaluate the current research landscape, assess the evolution and impact of ML models in T2DM research, and identify key trends and gaps in the literature. Specifically, this study will explore the following research questions.
1. How has the research landscape on T2DM prediction evolved in terms of publication frequency, citation metrics, and international collaborations from 1991 to 2024?
2. How do different ML methodologies and applications contribute to the various thematic clusters within the field of T2DM prediction research, and what are the intellectual connections and centralities among these clusters as revealed by co-citation network analysis?
3. How have ML models evolved in the prediction of T2DM, and what trends and methodologies have emerged over the different decades from 1991 to 2024 in terms of data sources, algorithms, and predictors?
4. What are the future areas of research and associated challenges?
Our research study is organized as follows: Section 2 details the comprehensive approach employed to select and analyze the keywords used in this study. The detailed bibliometric analysis by analyzing author affiliations, citation counts, publication trends, and international collaboration patterns has been presented in Section 3. The discussion on the network analysis is presented in Section 4. Section 5 analyses ML applications in predicting T2DM from 1991 to 2024, divided into four eras: 1991–2000, 2001–2010, 2011–2020, and 2021–2024. Section 6 presents future directions and Section 7 concludes our study.
This section begins by outlining the comprehensive approach used to select and analyze the keywords relevant to this study, specifically addressing research question 1. Following this, research methodology employed to select the research articles based on the identified keywords is discussed.
The keyword selection process began with an initial gathering of keywords, guided by input from domain experts. This input was then combined with Term Frequency-Inverse Document Frequency (TF-IDF) (17) to identify keywords that are both relevant and comprehensive for the bibliometric research. Word clouds were used to visually represent the selected keywords. Finally, a curated set of keywords was finalised for dataset extraction.
Table 1a shows the initial set of keywords that were generated through the solicitation of domain expertise, encompassing both broad and specific terms relevant to T2DM and predictive modelling. The keywords were divided into primary and secondary categories based on their relevance and importance to the study. Each primary keyword was systematically combined with every secondary keyword using logical “AND” and “OR” operators to ensure comprehensive coverage and relevancy in search queries. The terms included fundamental descriptors like Diabetes mellitus and Type 2 diabetes, as well as methodological keywords such as Machine learning, Logistic Regression (LR), and Deep learning, to name a few. Furthermore, to capture various permutations of crucial terms such as “prediction” and “predicting,” the “*” operator for truncation alongside root keywords has been employed.
Table 1b presents the refined set of articles specifically focusing on T2DM prediction using ML algorithms. This refinement process involved a careful review of the titles and abstracts of the initially identified articles. The criteria for selection were strictly based on the relevance to T2DM prediction and the application of machine learning techniques. Consequently, we identified 1,808 articles as our initial dataset of T2DM literature. By narrowing down the dataset through this rigorous screening process, we ensured that the articles included in this study were directly pertinent to our research objectives.
After establishing a foundational set of keywords, further refinement was performed using the TF-IDF algorithm on the preliminary keyword screening dataset. For more details, please refer to Appendix.
During this phase, we curated a set of keywords for the ultimate extraction of articles from multiple In review databases. The chosen keywords, as presented in Table 1c, were selected with the overarching objectives of the literature review in mind, as outlined in the Section “Aims and Objectives of Study.” A threshold of 0.70 was set to guide the selection process, ensuring that only the most relevant and impactful keywords were included. For instance, foundational keywords such as Diabetes mellitus’ and Type2 diabetes’ were chosen to delineate the research domain, ensuring that the corpus reflected the specific disease focus. Acknowledging the diverse landscape of predictive analytics, we adopted an interdisciplinary strategy by integrating “Data mining” and “Logistic regression,” showcasing the fusion of statistical and computational domains. Introducing “learning algorithm” and “neural network” allowed us to encompass a wide range of algorithmic methodologies, spanning from traditional statistical techniques to innovative AI methods.
The predictive emphasis of the analysis was enhanced with terms like “Risk factor,” “Risk score,” “Risk assessment,” and “Predictive Model,” directing attention to literature focusing on prognostic evaluation. Interestingly, clinical terms like “Risk factor” and “Risk score” carry considerable importance, surpassing the anticipated prominence of algorithm-related terms such as “Machine learning” and “Neural network.” This indicates that while advanced algorithms are vital, the core of diabetes prediction research lies in their integration with traditional clinical assessments. “Risk assessment” bridges these algorithmic and clinical aspects, underscoring the importance of evaluation in utilizing predictive analytics effectively. Notably, we combined “Predictive model” and “Prediction model,” both of which had significant TF-IDF scores, into a single keyword “Predict* model” to streamline our search and ensure comprehensive coverage of predictive modeling research. Additionally, although terms like “Diabetes dataset” and “Diabetes patient” met the threshold criteria, they were considered too generic and therefore excluded from the final keyword set. Instead, we opted for more specific terms to ensure the precision of the literature retrieved. However, “Risk prediction” given its relevance and specificity, was included as a primary keyword to capture studies focused on risk prediction and assessment in diabetes.
The process of literature selection and data collection was conducted systematically, following the PRISMA guidelines, and is detailed in the PRISMA flow diagram (Figure 1). Each step taken to refine the dataset is described below:
1. Identification: Articles were sourced from two comprehensive databases, Web of Science (WoS) and Scopus, to maximize coverage and minimize the exclusion of relevant studies. This broad scope reduced the risk of missing key literature due to database limitations. A finalized set of primary and secondary keywords, informed by expert input and refined through the TF-IDF method, guided the search. The refined results for the keyword sets are presented in Table 1c. The search targeted articles published between 1991 and 2024, relevant to T2DM prediction using ML techniques. This process initially identified 3,245 research articles. These articles were distributed equally among the five authors for review. Each author independently assessed their assigned articles using predefined relevance criteria to ensure consistency. Any discrepancies between reviewers were resolved through group discussions, ensuring objectivity and minimizing bias. Automation tools, including the Bibliometrix package in RStudio, were employed during the eligibility phase to identify and remove duplicate records. This automation streamlined the dataset, reducing it to 2,351 full-text articles for further analysis. The last search was conducted in August 2024. Bibliometric methods, including thematic clustering and co-citation network analysis, were applied to the refined dataset. Trends were assessed across four time periods: 1991–2000, 2001–2010, 2011–2020, and 2021–2024. Predictive models, datasets, and key variables were systematically evaluated during this process.
2. Screening: During the screening phase, irrelevant records were removed, reducing the dataset by 2,795 articles. Non-English articles, conference papers, and those unrelated to T2DM prediction were excluded. Further refinement removed records focusing on diagnosis, prevention, treatment, or complications of Type1 and gestational diabetes. 450 articles focusing on diagnosis, prevention, treatment or complications, Type1 and gestational diabetes were not included in the review.
3. Eligibility: After the initial screening, 444 duplicate records were identified and removed using the Bibliometrix tool in RStudio (18). This refinement left 2,351 full-text articles, which were assessed for eligibility.
4. Inclusion: Following the assessment, all 2,351 articles were deemed eligible and included in the research for further analysis. No articles were excluded at this stage.
To address research question 2 and to meet the objective of examining key attributes of diabetes prediction literature, this section presents a detailed bibliometric analysis. To visualize and map the literature database, we employed the Bibliometric package (18). Furthermore, the co-citation network, co-occurrence network, and collaboration network were graphically represented using the VOSViewer software (19). VOSViewer is a software tool used for constructing and visualizing bibliometric networks. These networks can include journals, researchers, or individual publications, and can be created based on citation, bibliographic coupling, co-citation, or co-authorship relations.
Analyzing citation counts, publication trends, and international collaborations provides insights into the evolution and impact of research in this field. As summarized in Table 2, the study spans 1991–2024, includes 1,115 sources, and reports an average of 12.09 citations per article. The authors in (20) highlighted the early 1990s as pivotal for advancements in AI and ML in healthcare, with researchers exploring these techniques for processing medical data, particularly for Type 1 and Type 2 diabetes. This study captures the evolution of AI and ML in diabetes management, from these early breakthroughs to recent advancements in 2024.
Our bibliometric analysis offers an in-depth view of how scholarly publication volume and citation metrics have evolved over time. As illustrated in Figure 2, we focused on two principal elements: the trends in citations across publication years (Figure 2a), and the temporal relationships among variables—including the number of articles published (No_of_Articles), the number of citable years (CitableYears), mean total citations per article (MeanTCperArt), and mean total citations per year (MeanTCperYear)—using a correlation matrix heatmap (Figure 2b).
1. Exponential growth in research output and its consequences: Post-2015, annual publication output surged from fewer than 50 articles in 2010 to over 450 by 2022 (Figure 2a). This surge aligns with growing interdisciplinary interest, increased funding, and AI advancements in healthcare. However, a negative correlation () between publication volume and mean citations per article (Figure 2b) suggests that as quantity increases, individual impact diminishes. Similar trends in AI-driven healthcare research indicate a focus on novelty over meaningful innovation, often resulting in redundant studies with incremental improvements (21–23). In T2DM prediction, frequent reuse of datasets like the UCI Pima Indians Diabetes Dataset has led to limited generalizability and reduced clinical relevance (4, 24). This lack of diverse population data restricts generalizability, clinical value, and translational impact, limiting the broader applicability of these predictive models (25). Without greater emphasis on real-world validation, and interdisciplinary collaboration, research risks stagnating in theoretical improvements rather than delivering meaningful advancements in healthcare.
2. Citation trends: Research saturation and diminishing impact: Despite the rapid rise in publications, the red line in Figure 2a (mean citations per article) fluctuates without sustained growth, while the green line (mean citations per year) remains relatively flat. This suggests inefficiencies in knowledge dissemination, where an expanding body of research does not necessarily translate into broader scientific progress. This suggests inefficient knowledge dissemination and “research saturation,” where publication proliferation does not equate to innovation (22). AI-driven T2DM prediction models often emphasize technical novelty over interpretability and validation, limiting clinical applicability (26). High-impact studies tend to integrate diverse data, explainable AI, and real-world implementation (27, 28). Addressing these gaps requires shifting research priorities from sheer publication volume to rigorously validated, clinically relevant work.
3. Correlation matrix insights: The unequal distribution of research impact: The correlation matrix (Figure 2b) reveals an uneven distribution of research influence, with a strong positive correlation () between mean citations per year and mean citations per article. This indicates that a small subset of highly cited studies disproportionately impacts the field, while most publications contribute minimally. To address this imbalance, targeted investments should focus on high-quality, interdisciplinary research that integrates longitudinal datasets, diverse patient populations, explainable AI, and clinical validation to ensure real-world relevance (26). Without such efforts, the current trend risks further widening the gap between research volume and meaningful scientific advancements.
4. Implications for researchers, journals, & policymakers: These trends have significant implications for researchers, journals, and policymakers. Researchers often face mounting pressure to maintain high publication counts potentially reducing the time and resources devoted to deeper, more impactful investigations (29). It is also evidenced by the negative correlation between publication volume and mean citations per article. Journals, witnessing a marked influx of submissions, must strengthen peer-review standards and encourage practices such as data sharing, reproducibility, replication studies, and open-data initiatives (30). Policymakers and funding agencies should promote interdisciplinary collaborations and translational research to enhance the real-world impact of AI in healthcare. Encouraging global research partnerships and knowledge-sharing can facilitate the development of interoperable AI-driven health innovations. Recent bibliometric analyses highlight the growing prominence of AI in health informatics, underscoring the need for strategic investment in high-impact research that addresses emerging healthcare challenges (31). While numerous articles continue to appear each year, only a fraction yield novel insights, such as integrating real-world electronic health records (EHRs) or leveraging advanced deep learning architectures for precision risk stratification. Correspondingly, highly cited works in this space are often those that bridge multiple domains (e.g., endocrinology, computer science, bioinformatics) or that focus on interpretable AI to aid clinicians in practical decision-making. This underscores the broader theme that breakthrough research—encompassing originality, methodological rigour, and real-world utility—tends to have a more profound citation footprint and lasting impact on healthcare practice.
Figure 2. Analysis of research articles published annually w.r.t citations. (a) Citation trend. (b) Correlation matrix.
In summary, while AI-driven healthcare research continues to expand, bibliometric trends highlight the need to prioritize impactful studies over sheer publication volume. Addressing dataset limitations, ensuring clinical applicability, and fostering interdisciplinary collaboration are essential for meaningful progress. Insights from Figure 2 underscore the imperative to recalibrate research priorities. While the increasing volume of publications reflects strong engagement, citation data reveal gaps in quality and innovation. A collective effort among researchers, journals, and funding agencies is necessary to realign incentives toward interdisciplinary, impactful, and reproducible work. By doing so, the rapidly growing body of literature can drive tangible advancements in healthcare, particularly in T2DM prevention and management.
In this study, we employ a network analysis to explore the patterns of association between various countries. Figure 3 shows the visual representation of the network created using VOSviewer, which facilitates the comprehensive examination and interpretation of complex datasets. This visualization allows us to discern clusters and relationships among countries, providing a foundation for deeper analysis. Table 3 complements the visual insights gained from the network analysis by presenting quantitative metrics for the top five nodes within each cluster. These metrics include bridging centrality, closeness, and PageRank, which offer valuable insights into the roles and influence of individual countries within the collaboration network.
Bridging centrality measures a node’s role as a bridge between different parts of the network. A higher bridging centrality indicates that a country acts as a key connector or conduit through which interactions between other countries occur. Closeness centrality reflects the average distance of a node to all other nodes in the network. A country with high closeness centrality can be interpreted as having direct and short paths to other nodes, indicating a potential for swift and efficient interactions. PageRank is a measure of node importance, which considers not only the quantity of connections but also the quality, as connections from more significant nodes carry more weight. In this context, a country with a high PageRank is seen as influential within the network, likely contributing to or benefiting from robust interactions.
It can be observed from Figure 3 and Table 3 that Cluster-1 comprised of countries such as Saudi Arabia, Egypt, Pakistan, and others, demonstrating moderate levels of bridging centrality. These countries appear to act as mediators, facilitating connections between other countries in the network. However, their closeness and PageRank values were relatively lower, suggesting a more peripheral role in the broader collaboration landscape. Cluster-2 encompassed countries like Spain, Italy, Netherlands, and Singapore, among others. Italy emerged as a notable influencer within this cluster, boasting the highest PageRank score. Meanwhile, Singapore and the Netherlands exhibited significant bridging centrality, indicating their pivotal roles in linking various countries within the collaboration network. The cohesive nature of this cluster suggests a tight-knit group of countries with strong collaborative ties, potentially focusing on prediction.
Cluster-3 included leading nations such as the USA, China, India, and others, each wielding considerable influence and connectivity within the collaboration network. The USA and the UK stood out with the highest PageRank values, underscoring their dominant positions in global collaboration networks. These countries play crucial roles in shaping research agendas, driving innovation, and fostering international partnerships across diverse fields. Overall, the analysis of international collaborations highlights the roles of key countries and regions, indicating a robust and interconnected global research network. Countries such as the USA and the UK continue to lead in terms of influence and collaboration, while emerging contributors like India and Singapore show the expanding geographical scope of impactful research.
Bibliometric methods for network analysis have proven to be effective tools for revealing both well-established and novel research topics. In this section, we utilized co-citation network analysis, a bibliometric method, to establish intellectual connections between significant research papers and map the intellectual structure of diabetes prediction research. This method focuses on the relationship or interaction between two publications and provides an overview of publications that have been cited together in other research articles. When two or more articles are cited together more frequently in other research articles, the probability of similarity between them is higher (32). As illustrated in our co-citation network analysis (Figure 4), there are four distinct clusters, each representing a unique aspect of the intersection between ML and diabetes studies: the blue, red, pink, and green clusters. These clusters were analyzed based on their thematic focus, as well as their bridging and closeness centrality measures within the co-citation network, providing insights into their roles and interconnections within the broader research landscape. A detailed summary of each cluster, including their thematic focus and centrality measures within the co-citation network, is provided in Table 4.
• Foundational ML & statistical methodologies (Blue cluster): In the context of our co-citation analysis, the blue cluster represents foundational ML and statistical methodologies that are fundamental to the advancement of diabetes research. This cluster incorporates seminal works that have contributed to the development and refinement of algorithms, particularly addressing prevalent issues in data science such as imbalanced datasets, exemplified by the work of (33) on the Synthetic Minority Over-sampling Technique (SMOTE). Furthermore, it includes references to widely-used tools such as sci-kit-learn, which have democratized the application of ML through their ease of access and versatility (34). It can be observed that there is a moderate closeness centrality observed in the blue cluster. It suggests that the methodologies it encompasses are broadly relevant to a wide array of studies within diabetes research. This relevance is attributed to the universal nature of foundational ML techniques, which are applicable across various subdomains, from basic biological research to clinical applications. Such techniques are often necessary prerequisites for more advanced, specialized research and provide a common language for scientists across disciplines. However, the cluster’s lower bridging centrality reveals a nuanced role. This could suggest that, although these foundational methods are widely used within individual research domains, they are less often at the forefront of interdisciplinary integrations that link disparate strands of diabetes research.
• Epidemiological forecasts & lifestyle interventions (Pink cluster): Encompassing a wide range of studies, the pink cluster explores epidemiological forecasts, lifestyle interventions, and the integration of digital health technologies in managing diabetes. It reflects a broader clinical and public health perspective, focusing on risk assessments [e.g., (35, 36)] and the global burden of diabetes (37), all of which are essential for a comprehensive understanding of diabetes management and prevention. The high closeness centrality associated with the pink cluster’s themes within the bibliometric network underlines the integral role these studies play in the broader scope of diabetes research. The research contained within this cluster lays the groundwork for various other domains within diabetes research, making it foundational. It offers essential insights into public health strategies, the development of clinical practices, and the formulation of healthcare policies.
• Application of ML in predictive modeling (Green cluster): In the green cluster of the co-citation network analysis, the central theme encapsulates the application of ML techniques to enhance the predictive modelling and analysis in diabetes research [e.g., (38, 39)]. Key findings within this cluster reveal that decision trees (DT), random forests (RF), neural networks (NN), and Gradient Boosting Machines (GBM) are particularly effective in predicting the onset of diabetes mellitus using a range of clinical and demographic data [e.g., (40, 41)]. Comparative studies within the cluster suggest that the more sophisticated ML models do not always yield clinically relevant enhancements over traditional regression models (42). This is critical as it underscores the need for carefully considering the choice of a prediction model in practical settings. Moreover, it can be observed that the green cluster demonstrates a high closeness centrality, which indicates their significant linkage within the research network. This closeness centrality is due to the cluster’s contribution to predictive health informatics, an area of heightened importance that harnesses ML techniques to foresee diabetes onset and progression. Such predictions are crucial for planning public health interventions and managing resources in healthcare systems. Moreover, the moderate bridging centrality of this cluster reveals its role in blending methodological advancements from machine learning with practical, actionable insights for clinical and epidemiological purposes.
• Application of ML in clinical diabetes research (Red cluster): The red cluster specifically targets the application of ML techniques for diagnosing, predicting, and managing diabetes. It includes innovative uses of algorithms for clinical data analysis aiming to enhance patient care and outcomes [e.g., (43, 44)]. A notable feature of the red cluster is its high centrality from the methodological (blue), public health-oriented (pink), and epidemiological (green) research clusters. The divergence is partly due to the unique data and specialized patient information required for clinical studies, which contrasts with the population-level data prevalent in public health and epidemiology studies. Moreover, the red cluster embodies the intersection of ML with clinical medicine, a path that is often separate from the public health and foundational research trajectories due to differing methodologies, publication cultures, and terminologies. The regulatory and ethical landscape governing clinical research further contributes to this separation, as these considerations demand stringent adherence to privacy and safety standards, which may not align with the broader ML research cited by the other clusters. Additionally, clinical application research is often driven by the immediacy of patient-centred outcomes and the rapid development and deployment cycle of medical technologies, creating a focused body of literature that prioritizes efficacy and safety. This patient-centric approach is less likely to interlace with the exploratory or predictive nature of the research found in the remaining clusters. Consequently, the red cluster’s progression forms a distinct branch within the research landscape, signalling a need for more concerted interdisciplinary efforts to bridge the gap and foster a more cohesive dialogue between these crucial areas of diabetes research.
Overall summary: Our bibliometric analysis highlights the multi-faceted nature of ML research in diabetes, spanning foundational algorithm development, clinical studies, public health analyses, and predictive modeling. The thematic clusters underscore interconnected efforts to leverage ML for better understanding, predicting, and managing diabetes. Foundational methodologies (blue cluster) offer adaptable tools, public health insights (pink cluster) inform large-scale prevention strategies, predictive modeling advancements (green cluster) enable robust applications, and clinical applications (red cluster) translate advancements into patient-centered outcomes. Future research should prioritize interdisciplinary collaboration to bridge gaps between clusters, integrating methodologies, clinical insights, and public health strategies. Addressing regulatory and ethical challenges will be key to real-world implementation. The continued evolution of these clusters promises advancements in diabetes prediction research, improving prevention, diagnosis, and management globally.
This section addresses research question 3 of how ML models have evolved in the prediction of T2DM and complies with the objectives of evaluating foundational methodologies and statistical techniques, identifying gaps, and analyzing emerging trends from 1991 to 2024. It thoroughly reviews the models and techniques used over different decades, evaluates predominant predictors, assesses the impact of datasets on model accuracy, and highlights challenges and future research directions.
This content analysis focuses specifically on the application of ML in predicting T2DMs. For the reader’s convenience, we analyzed the content for each decade, starting from 1991 to 2024, divided into the following eras: 1991–2000, 2001–2010, 2011–2020, and 2021–2024. For each era, we provide literature and discussion on (i) the ML models used for T2DM prediction, (ii) the datasets utilized to train the ML models, (iii) the predictors used, and (iv) emerging trends or topics in that era. Figure 5 provides a visual summary of these key elements for each era.
We follow the strategy outlined by Marcus et al. (45) for conducting a literature analysis on our curated dataset of 2,351 publications, utilizing a systematic and structured approach of TF-IDF (Section 2). To ensure a comprehensive analysis, we developed a systematic four-point scale for both qualitative assessment (relevancy score) and quantitative assessment (impact score). Two reviewers were selected to implement this methodology. Each reviewer independently assessed articles based on predefined relevance criteria. Any discrepancies in their ratings were resolved through discussion until a consensus was reached. This process helped maintain objectivity and minimize bias in our relevance scoring. The relevance score assesses how closely an article aligns with the specific focus of the study.
This has been determined based on the following criteria, scored on a four-point scale.
1. Score 4 (Highly relevant): Articles focused exclusively on ML algorithms for T2DM prediction, providing detailed analyses, results, and discussions.
2. Score 3 (Moderately relevant): Articles discussing ML for health outcomes, including T2DM, but not exclusively focused on T2DM prediction.
3. Score 2 (Slightly relevant): Articles addressing ML broadly or T2DM without specifically using ML for prediction.
4. Score 1 (Not relevant): Articles mentioning ML or T2DM only tangentially, with minimal relevance to the core topic.
After the qualitative assessment, articles that were deemed relevant underwent a quantitative assessment through impact score. The impact score evaluates the quantitative influence of an article, typically based on citation metrics and the article’s reach within the scientific community. We first obtained the total number of citations for each article from databases such as Google Scholar, Scopus, and Web of Science. Then to ensure a fair comparison, we normalized the citation counts. This involved calculating the average number of citations for similar articles (considering subject, journal, and publication year).1 Each article was assigned an impact score based on its citation count compared to the normalized average, using a quartile-based system to quantitatively assess its relative impact.
1. Score 4 (Highly influential): This score is assigned to articles in the top 25% (Q1 quartile) of citation counts, indicating they have the highest influence.
2. Score 3 (Influential): Articles in the second quartile (25%–50%) of citation counts receive this score. These articles have a high influence but fall below the top 25%.
3. Score 2 (Average influence): Assigned to articles in the third quartile (50%-75%) of citation counts, representing an average level of influence.
4. Score 1 (Below average influence): This score is given to articles in the bottom 25% (Q4 quartile) of citation counts, indicating they have the lowest influence among the set.
The overall score for each article was determined by summing its relevance and impact scores. To prioritize articles for detailed review, we averaged the combined relevance and impact scores from each rater. Articles with an average score exceeding a predetermined threshold of 3.5 were included in the in-depth analysis phase. This dual-criteria approach, integrating qualitative relevance assessment with quantitative impact analysis, ensures that the selected articles are both highly relevant and impactful in the field of T2DM prediction using ML. This methodology provides a comprehensive and systematic approach to identifying and analyzing the most significant research articles in this domain.
This era has been predominantly focused on the utilization of ML algorithms and existing technologies for the management of Type 1 diabetes, particularly in predicting and controlling blood glucose levels. For instance, (46–49).
In contrast, little attention has been paid to the prediction of T2DM, with only one study (50) employing ML algorithms to predict this condition. The study (50) evaluates the efficacy of Artificial Neural Networks (ANNs) in predicting diabetes and compares its performance with LR and the ADAP (Adaptative Perceptron) learning algorithm. Utilizing the Pima Indian diabetes dataset (51), which includes 768 cases with eight significant predictor variables (e.g., number of times pregnant, plasma glucose concentration, etc), the study identifies plasma glucose concentration, Body Mass Index (BMI), and age as the best predictors through a backward-elimination, stepwise approach. The NN model with one hidden node outperformed LR and ADAP, achieving a training classification accuracy of 77.43% and a test classification accuracy of 81.25%, compared to LR’s 77.60% and 79.17%, and ADAP’s 76% test accuracy.
In comparison to the initial era (1991–2000), several studies conducted during 2001–2010, have focused on T2DM using various ML algorithms, datasets, and predictive features. Based on our developed systematic four-point scale for qualitative and quantitative assessment, we selected 18 highly influential articles published during this era.
ML algorithms utilized: During this period, several ML models were explored for T2DM prediction. Prominent models include Support Vector Machines (SVM), ANN, Hybrid Models, Semi-supervised Learning Models, DT (C4.5 Algorithm), General Regression Neural Networks (GRNN), K-means Clustering (KNN), LR, Fuzzy Neural Networks (FNN), Rule-based Methods such as Sequential Covering Approach (SQRex-SVM), and Eclectic Method for Rule Extraction. These algorithms reflect a variety of approaches, from classification and regression techniques to clustering and rule-based methods, highlighting the breadth of ML applications in T2DM prediction research during this period.
Datasets utilised: Several key datasets were utilized during this period, each contributing to the robustness of the research findings. Pima Indians Diabetes Dataset was extensively utilized for its detailed clinical features relevant to diabetes prediction. It was referenced in studies like (52–62) etc., highlighting its importance in ML research for diabetes. UCI Irvin ML Repository (63) was another frequently used dataset, supporting various studies such as (56, 64–66). This repository’s diverse datasets facilitated a broad range of ML applications. National Health and Nutrition Examination Survey (NHANES) provided a comprehensive set of health-related data, which was leveraged in the (67) for the prediction of T2DM study, illustrating its utility in large-scale health data analysis.
Predominant predictors used for training ML models: During this era, the predominant predictors used for training ML models in T2DM prediction research were primarily clinical and demographic variables. Clinical predictors such as BMI, plasma glucose concentration, diastolic blood pressure, triceps skin fold thickness, 2-hour serum insulin levels, diabetes pedigree function, and cholesterol levels were frequently employed due to their strong association with diabetes risk. Demographic predictors, particularly age and sex, were also commonly used, reflecting their significant impact on the likelihood of developing T2DM. These predictors were integral to many studies, including those leveraging datasets from the UCI Machine Learning Repository and the Pima Indians Diabetes Dataset.
In contrast, less emphasis was placed on lifestyle predictors such as physical activity, diet, smoking status, alcohol consumption, and exercise habits. Although these factors are recognized as influential in diabetes development, they were not as prominently featured in the predictive models of this period. Only a few studies, such as (67) integrated these lifestyle factors into their analysis. Additionally, genetic predictors like Single Nucleotide Polymorphisms (SNPs) were used in only one study (68), likely due to the limited availability of comprehensive genetic data during this timeframe. The focus on traditional clinical and demographic predictors reflects the research priorities and data availability of the era, while the relative underuse of lifestyle and genetic factors indicates areas for future exploration and integration in predictive modelling.
Emerging trends: This era saw innovative trends in T2DM prediction, including hybrid models, semi-supervised learning, and rule-based systems to enhance interoperability.
Hybrid models combine multiple algorithms to leverage the strengths of each and improve predictive performance. This approach helps in addressing the limitations of individual algorithms and enhances the robustness of the predictive models. For instance, the authors in (69) used the Simple KNN Algorithm for initial data validation and the C4.5 Algorithm for building the final classifier contributed to the robustness and high performance of the HPM, making it a reliable tool for predicting the incidence of T2DM in newly diagnosed patients. The study (70) applied SVM with rule extraction techniques to improve model interoperability and validate the model using a real-life dataset to ensure high prediction accuracy, sensitivity, and specificity. The paper (56) developed a hybrid neural network system that combines ANNs and FNN for the classification and prediction of T2DM. The primary objective is to increase the classification accuracy of medical data by integrating both fuzzy and crisp data and to evaluate the performance of this proposed hybrid system. The study (71) focuses on enhancing prediction accuracy by integrating fuzzy logic and NN techniques to model the complex relationships in diabetes data. The objectives are to demonstrate the effectiveness of this hybrid approach and to compare its performance with traditional ML methods.
Semi-supervised learning methods incorporate both labelled and unlabeled data into the model training process. This approach is particularly useful when labelled data is scarce or expensive to obtain, as it allows the model to learn from a larger dataset that includes unlabeled data. The author in (57) employs a semi-supervised learning method known as Laplacian SVM (LapSVM), that integrates labelled data with a significant amount of unlabeled data, leveraging the latter to improve the learning process. The model achieved higher accuracy and better generalization by utilizing the additional information from unlabeled data. Another study (57), applied General Regression Neural Networks (GRNN) for diabetes prediction and also incorporated semi-supervised learning algorithms. The use of GRNN, combined with semi-supervised learning, allowed the model to leverage the additional information provided by the unlabeled data, resulting in enhanced performance.
Based on our developed systematic four-point scale, we selected and reviewed 55 highly influential articles published to predict T2DM using various ML models. In comparison to the 2001–2010 time period, the period from 2011 to 2020 has been transformative for T2DM prediction. The adoption of advanced ML algorithms, the expansion of feature sets, and the emphasis on real-world applicability represent the key emerging trends.
ML algorithms utilized: The most commonly utilized ML algorithms across these studies are LR, RF, SVM, Naïve Bayes (NB), kNN, GBM, Classification and Regression Trees (CART), and Gaussian Naïve Bayes. LR has been widely used across multiple studies due to its simplicity, effectiveness, and performance in binary classification problems (72–76). In comparative studies, RF has often outperformed other algorithms such as LR, KNN, and Gaussian Naïve Bayes (42, 73, 75, 76). It has been successfully applied across various datasets, including electronic medical records (EMR) and large-scale cohort studies (73, 76). SVM has been utilized in studies dealing with high-dimensional data spaces (77–80). While SVMs have shown competitive results, ensemble methods such as RF and GBM have often surpassed SVM in predictive accuracy (75, 76, 78, 79, 81, 82). Naïve Bayes is frequently utilized due to its simplicity and efficiency in handling large datasets [e.g., (75)]. KNN has been employed in studies emphasizing interpretability and simplicity (77, 80). However, KNN typically yields lower performance compared to more complex algorithms. GBM has gained popularity due to its high accuracy. Studies have utilized GBM and achieved high AUCs, with boosting techniques significantly improving model accuracy (76, 79). Classification and Regression Trees were often used in conjunction with other algorithms or as part of ensemble methods like RF (73, 76). While providing solid baseline performance, CART’s results were generally enhanced when used within ensemble methods. Gaussian Naïve Bayes has been employed in scenarios requiring probabilistic interpretation of predictions (73).
Datasets utilized: Similar to 2001–2010 era, among the most commonly used datasets is the Pima Indians Diabetes Dataset (75, 83–87). Additionally, the Henan Rural Cohort Study is used [e.g., (76)], providing valuable data from a large population sample in rural China. Furthermore, many studies leverage routinely collected EHR data from multiple health centres, offering rich, real-world insights into patient health metrics and outcomes (77, 80, 88).
Predominant predictors used for training ML models: Numerous studies in this era have focused on identifying demographic information such as age and gender, alongside medical conditions like hypertension and dyslipidemia, and lifestyle factors including physical activity and diet. Anthropometric measures like BMI and waist circumference, along with blood parameters such as glycated haemoglobin (A1c), fasting plasma glucose, triglycerides, and cholesterol levels, are commonly used to assess T2DM risk. Studies [e.g., (73, 89–91)] highlight that older age, high BMI, increased waist circumference, and a family history of diabetes are strong T2DM indicators. Incorporating HbA1c into predictive models has demonstrated high accuracy in identifying at-risk individuals (74, 92, 93). Additionally, lipid profiles, particularly elevated triglycerides and low HDL cholesterol levels, are significant predictors commonly associated with insulin resistance, a precursor to T2DM (90, 94).
Despite substantial advancements in research, there remain notable deficiencies, particularly in the integration of detailed dietary habits and nutritional patterns. Incorporating these variables could yield valuable insights into their impact on diabetes risk. Additionally, investigating genetic predispositions and their interactions with lifestyle and environmental factors could enhance the comprehensiveness of risk assessments. Furthermore, examining psychosocial factors, such as stress and mental health, could provide a more holistic understanding of diabetes development and management. Addressing these underrepresented areas in future studies will refine predictive models and contribute to a more thorough understanding of diabetes risk factors, ultimately improving prevention and management strategies.
Emerging trends: This period witnessed a notable transition in diabetes prediction research. Especially 2011–2016 was marked by a shift towards the utilization of ML tools and the exploration of diverse predictive variables. We observed three different research groups during this time period. The first group includes studies that utilize traditional clinical diabetes risk prediction techniques, which focus on large cohorts, but employ limited feature sets, such as (73, 95). The second group focuses on comparing ML models by utilizing classical diabetes risk factors as features, as demonstrated in (96, 97). The third group of related work considers a broader set of features that can be utilized to predict various diseases, such as (74). Additionally, during this time period, there was a trend of comparing different ML algorithms [e.g., (98, 99)] and identifying risk scores for variables associated with diabetes prediction [e.g., (5, 90)]. However, very few studies belonged to group 3. Furthermore, most studies were not generalizable to other populations, and handling missing values in large datasets was not frequently addressed. It is worth noting that the use of EMR for diabetes prediction dates back to 2012 (73).
From 2017 to 2020, researchers increasingly turned to more sophisticated algorithms and larger datasets to enhance the accuracy of diabetes prediction. For example, in (81), hidden patterns were extracted from data to anticipate outcomes for diabetes classification. In (100) and (101), fuzzy rules were generated using different methods for diabetes prediction. Additionally, researchers [e.g., (76, 101)] are working to identify novel optimal features such as urine and sweet taste that can aid in diabetes prediction, beyond basic features like age, gender, and BMI. We also observed a growing trend in utilizing socio-demographic and clinical/laboratory attributes (39, 76) and addressing issues such as missing data in predictive modelling, as evidenced in studies such as (102) and (103). While many authors have reported accuracy rates exceeding 85%, the majority of these studies have not been validated on populations with different race/ethnicity, and most of them have only used a limited number of features. Therefore, it is uncertain whether these models can be generalized to a larger population and how they will perform when more features are incorporated.
A critical trend observed is the diversity in the datasets utilized for developing these predictive models. The scope expanded to include a broader range of features, including biochemical markers, lifestyle factors, and even genetic data. This comprehensive approach has allowed for more accurate and personalized risk assessments. For instance, some studies incorporated electronic medical records and claims data, which provided a richer context and improved the models’ ability to predict diabetes onset at a population level.
The emphasis on feature engineering and the extraction of detailed features has been another notable trend. Researchers have extensively identified and validated a variety of predictive features. This granular approach has significantly enhanced the predictive power of the models. Moreover, the use of ensemble methods and hybrid models has become prevalent, combining multiple algorithms to leverage their strengths and mitigate individual weaknesses. The decade also saw a growing interest in real-world applications of these predictive models. Several studies (73, 104, 105) aimed to develop tools that could be integrated into clinical practice, enabling healthcare providers to identify high-risk individuals early and tailor preventive strategies accordingly.
In summary, these developments promise to enhance the accuracy and utility of predictive models, ultimately improving outcomes for individuals at risk of T2DM. Moreover, ensemble methods like RF and GBM were commonly the top performers across various studies, highlighting their robustness and high accuracy. LR continues to be a reliable benchmark model due to its simplicity and interpretability. Many studies emphasized the importance of feature selection and engineering, significantly impacting the performance of the models.
Based on our developed systematic four-point scale strategy, we reviewed 65 highly influential papers published during this era. Researchers have increasingly combined advanced technologies such as medical devices, wearable and sensor technologies with ML, deep learning, and AI approaches to forecast T2DM (106–109). Additionally, similar to the 2011-2020 era, there is continued emphasis on identifying novel and effective non-invasive features that can assist in predicting T2DM (109–114).
ML Algorithms utilized: Analysis from 2021–2024 reveals that RF, SVM, XGBoost, and KNN remain popular (115–125). In contrast to 2001–2020, researchers focused on deep learning models, particularly NN (e.g., (126, 127), Convolutional Neural Networks (CNNs) [e.g., (128, 129)], and Long Short-Term Memory (LSTM) networks [e.g., (129, 130)], have become more prevalent due to their superior performance in handling large and complex datasets. Federated learning has emerged as a promising approach for collaborative research, allowing models to be trained on decentralized data sources without compromising patient privacy (131, 132). This technique facilitates large-scale, multi-centre studies and enhances the robustness of predictive models. Moreover, there is an increasing emphasis on the interpretability and explainability of ML models. Techniques such as SHAP (SHapley Additive exPlanations) (133) and LIME (Local Interpretable Model-agnostic Explanations) (134) are being used to help clinicians understand and trust the predictions made by these complex models (135, 136). The use of hybrid models and transfer learning is also on the rise.
Dataset utilized: Pima Indian Diabetes Dataset and EHR are still often cited due to their comprehensive features relevant to diabetes prediction (122, 126, 137). Additionally, EHR are widely used, providing real-world data essential for developing and validating predictive models (122, 126, 137). Other significant datasets include UK Biobank (117, 138, 139) extensive health-related data that supports robust predictive analysis. Although minimal studies found to be using NHANES and Korean National Health and Nutrition Examination Survey (KNHANES) dataset (126, 140, 141). A notable pattern in the use of these datasets is their application in cohort studies, highlighting their value in longitudinal research that tracks health outcomes over time (142–144). Additionally, many studies leverage real-world data and databases, indicating a trend towards using diverse and large-scale data sources to enhance the accuracy and generalizability of predictive models (138, 145).
Predominant predictors used for training ML models: From 2021 to 2024, numerous studies investigated various predictors encompassing domains such as demographic, medical condition, hereditary, anthropometric, and laboratory data. To provide clarity, we categorised these predictors into five groups: demographic, medical condition, lifestyle, hereditary & psychological, anthropometric, and laboratory data. Some studies [e.g., (146, 147)] integrated demographic, lifestyle, and clinical data to predict diabetes. Tables 5–9 provide a comprehensive summary of predictors used in T2DM prediction studies, emphasizing their frequency and significance. Predictors marked with are identified as the most influential by the authors. Figure 6 visualizes the distribution of these key predictors through a pie chart, where each slice represents a significant factor, proportional to its frequency across studies. Each slice’s size reflects how often different studies have identified it as a key determinant of T2DM risk. While the tables present a broad overview of all explored predictors, the pie chart highlights only those deemed most impactful ().
1. Demographic data & lifestyle: In predicting T2DM, demographic and lifestyle predictors play a crucial role. Table 5 shows the different features used by various studies. Figure 6a highlights that age and gender are the most influential predictors in the demographic domain, emphasizing their critical role in the development and progression of T2DM. Ageing correlates with the natural decline in insulin sensitivity and beta-cell function, which are critical determinants of T2DM development. Several studies, including (137, 168, 194), emphasize age as a primary determinant. Similarly, gender disparities in T2DM prevalence and progression, influenced by hormonal changes and lifestyle differences, are evident in studies such as (148, 151). Lifestyle factors, including smoking, alcohol consumption, and physical activity, are modifiable predictors with a direct influence on metabolic health. Regular physical activity is shown to significantly lower T2DM risk, while sedentary lifestyles and high alcohol intake exacerbate insulin resistance and hyperglycemia (117, 199). Smoking introduces oxidative stress and inflammation, further increasing diabetes risk. These insights are pivotal for designing public health interventions targeting lifestyle modifications.
2. Hereditary & psychological: Hereditary factors, including the pedigree function and family history of diabetes, are robust indicators of genetic predisposition, as shown in Table 6, Figure 6b. These predictors capture the familial aggregation of T2DM and are widely cited in studies such as (163, 166). Psychological factors, including stress and psychiatric disorders, are emerging as significant contributors to T2DM risk. Patients with mental illnesses exhibit higher prevalence rates of T2D due to lifestyle disruptions, medication side effects, and stress-induced physiological changes (137). Machine learning models incorporating these predictors provide enhanced accuracy in risk stratification by capturing the intricate interplay between mental health and metabolic function.
3. Medical condition: Medical conditions offer critical insights into the biological and physiological precursors of T2DM, as summarized in Table 7. Figure 6c highlights pregnancies and blood pressure as key predictors in this domain. Pregnancies, particularly those complicated by gestational diabetes, significantly increase the risk of future T2DM, as demonstrated in studies like (122) and (167). Blood pressure, another core component of metabolic syndrome, is strongly associated with insulin resistance and T2DM. This relationship is highlighted in studies by (148, 170). Classic symptoms of diabetes, commonly referred to as the “3 Polys”—polyuria, polydipsia, and polyphagia—are commonly acknowledged as cardinal signs of diabetes. Studies such as (150, 180) emphasise their diagnostic importance. These symptoms, combined with other medical conditions, enhance the sensitivity and specificity of ML models in diagnosing T2DM.
4. Laboratory/clinical: Laboratory and clinical markers offer precise, quantifiable data for T2DM prediction. It can be observed from Table 8, Figure 6d that blood glucose levels, insuline and HbA1c are foundational metrics for diagnosing and monitoring T2DM. Studies such as (168, 194) consistently identify these as the most significant laboratory predictors. Lipid profiles, including TG and HDL-C, provide insights into metabolic health (117, 200). High TG levels and low HDL-C are associated with insulin resistance, as demonstrated by (137, 198). Additionally, renal function markers such as Scr and BUN are critical for assessing diabetes-associated kidney complications, as highlighted in (177). Liver function tests, including ALT, AST, and GGT, are increasingly recognised for their role in T2DM risk prediction. Elevated levels of these enzymes often correlate with non-alcoholic fatty liver disease (NAFLD), a condition closely linked to insulin resistance, as reported by (177, 185, 192).
5. Anthropometric measurements: Anthropometric measurements are non-invasive, cost-effective predictors of T2DM. Table 9 shows different anthropometric features used by various studies. Anthropometric measurements are critical in evaluating obesity and its role in T2D pathogenesis. It can be observed from Figure 6e that among different features, indicators like BMI, waist circumference, and waist-to-hip ratio are widely regarded as reliable predictors (117, 148, 187, 201). Advanced indices such as body adiposity index and body shape index offer refined assessments of body composition and its metabolic implications (201). These predictors are particularly valuable in population-based screening programs, enabling early identification of at-risk individuals. These findings confirm that diabetes is a multifaceted disease influenced by various factors, necessitating a comprehensive and interdisciplinary approach for its understanding and management.
Figure 6. Key predictors of T2DM categorized into demographic, hereditary, medical, laboratory, and anthropometric domains, highlighting their relative significance. (a) Demographic variables. (b) Hereditary & psychological predictors. (c) Medical conditions. (d) Laboratory & Clinical predictors. (e) Anthropometric measurements.
Emerging trends: Researchers are increasingly leveraging ML and AI tools to enhance the accuracy and robustness of predictive models for T2DM. The integration of omics data, including genomics, proteomics, metabolomics, and transcriptomics, is another significant trend (203). By incorporating these comprehensive molecular datasets, researchers aim to uncover the underlying biological mechanisms of T2DM. The use of omics data facilitates the identification of novel biomarkers and enhances the predictive power of models, offering deeper insights into the disease’s aetiology and progression. The use of real-time data from wearable technologies (204, 205) is an emerging trend in diabetes research. Devices such as activity trackers and continuous glucose monitors provide real-time insights into patients’ physical activity, dietary habits, and blood glucose levels. These data are invaluable for developing dynamic prediction models that can adapt to changing health behaviors and conditions, enabling timely interventions. There is a noticeable trend towards integrating data from multiple sources to enhance the robustness of predictive models. For example, combining genetic data from the UK Biobank with lifestyle information from NHANES or EHRs provides a more comprehensive risk assessment (117, 141, 206). The application of ML and AI techniques to these datasets is increasing, with researchers leveraging these advanced analytical tools to develop more accurate and personalized prediction models.
In conclusion, the frequent use of comprehensive datasets such as the UK Biobank, EHRs, and national health surveys underscores their critical role in advancing T2DM prediction research. The integration of multiple data sources, the application of ML, and the focus on personalized and preventive medicine are key trends shaping the future of this field. These efforts aim to improve the accuracy of predictions and the effectiveness of interventions, ultimately contributing to better health outcomes for individuals at risk of T2DM.
Building on the insights from this comprehensive analysis, several future directions are proposed to further advance the field of T2DM prediction research:
1. Digital Twins (DT) and Real-Time monitoring for personalized diabetes care: The integration of DTs and real-time monitoring offers a transformative approach to T2DM prediction and management. DTs create virtual replicas of patients using real-time data from wearable devices, CGMs, and EHRs to simulate personalized treatment strategies, predict complications, and optimize disease management (208, 209). Meanwhile, advancements in wearable technology (e.g., smartwatches, biosensors, insulin pumps) enable continuous health monitoring, allowing AI models to detect glucose fluctuations, automate insulin adjustments, and provide lifestyle recommendations (209–211). Future research should focus on enhancing DT models with multi-omics data for greater predictive accuracy (212), ensuring interoperability between real-time monitoring systems and healthcare platforms, and developing ML algorithms capable of processing high-frequency health data while maintaining stability and accuracy (213, 214). Additionally, large-scale clinical trials are necessary to validate the effectiveness of these technologies in real-world diabetes management (131, 215, 216).
2. Strengthening interdisciplinary collaboration: Collaboration between data scientists, healthcare professionals, and policymakers is essential to develop technically robust and clinically relevant models for T2DM prediction and management. Effective interdisciplinary partnerships can bridge the gap between ML advancements and real-world clinical application, ensuring that models are not only accurate but also interpretable and actionable for healthcare providers (217, 218). Large-scale, multi-center studies are needed to diversify datasets, enhance model generalizability, and improve applicability across different demographic and geographic populations. Additionally, policymakers must prioritize the development and enforcement of standardized regulations, ethical guidelines, and governance frameworks to address the challenges posed by AI in healthcare (219).
3. Development of explainable AI models: Our analysis shows that traditional ML models such as SVM, RF, and deep neural networks are widely used for T2DM prediction, but their black-box nature limits transparency and interpretability in clinical settings. Explainable AI (XAI) addresses this issue by offering techniques to interpret model predictions, identify key decision factors, and assess reliability, thereby enhancing trust among healthcare professionals (220, 221). Future research should focus on developing clinically interpretable AI models using techniques like SHAP (Shapley Additive Explanations), LIME (Local Interpretable Model-Agnostic Explanations), and attention mechanisms to provide meaningful insights into model behavior (133, 134). By integrating transparency and interpretability into AI models, XAI can enhance clinical decision-making and facilitate the wider acceptance of AI-driven T2DM management strategies.
4. Integration of multi-omics data: The incorporation of multi-omics data, including genomics, proteomics, metabolomics, and microbiomics, provides deeper insights into the biological mechanisms underlying T2DM (203, 222). By integrating these diverse datasets, predictive models can uncover novel biomarkers, disease pathways, and therapeutic targets, leading to improved risk stratification and precision medicine approaches (223). Multi-omics data facilitate the identification of gene-environment interactions, which play a crucial role in diabetes onset and progression. For instance, integrating various omics data has elucidated mechanisms through which T2DM-associated genetic variations impact disease risk (224). Future research should focus on developing advanced machine learning frameworks capable of efficiently integrating multi-omics data to enhance predictive accuracy while maintaining interpretability and scalability. Recent studies have highlighted the potential of DL based approaches for multi-omics data integration in cancer, suggesting similar methodologies could be beneficial in diabetes research (225).
5. Cross-population validation in predictive modeling: Generalizability is a major challenge in T2DM predictive modeling, as most models are developed using data from specific ethnic, genetic, and geographic cohorts, restricting their broader applicability. To ensure fairness, equity, and clinical relevance, models must be validated across diverse populations. Variations in genetics, environmental exposures, socioeconomic factors, and healthcare access play a crucial role in diabetes risk, highlighting the necessity of rigorous external validation across heterogeneous datasets. Without it, predictive models may perpetuate healthcare disparities and limit their real-world effectiveness. A study developed questionnaire-based prediction models for T2DM prevalence and incidence, training them on a white population and validating them across multiple ethnicities, demonstrating the importance of such cross-population validation (226). Future research should prioritize multi-center studies that incorporate genetically and environmentally diverse populations to improve model robustness and fairness (227, 228). Additionally, the integration of transfer learning and domain adaptation techniques could help models learn generalizable patterns and improve performance across heterogeneous datasets (229, 230). Ensuring rigorous external validation is crucial for equitable AI-driven diabetes care and broader clinical adoption.
6. Policymaker guidelines and support: To facilitate the integration of ML models into public health initiatives, policymakers must play a central role in resource allocation, regulatory oversight, and ethical governance (217, 219). Supporting pilot programs that test and refine AI-driven diabetes prediction models in real-world clinical and community settings will be crucial to ensuring their clinical utility and scalability (231). Policymakers should establish standardized guidelines for AI adoption in healthcare, focusing on data privacy, security, fairness, and algorithmic bias mitigation to promote safe and equitable AI applications (232, 233). Additionally, investment in publicly accessible datasets and federated learning frameworks can enhance data diversity and model generalizability while preserving patient confidentiality (234). Regulatory frameworks must ensure that AI-driven diabetes prediction models adhere to global health standards (e.g., GDPR, HIPAA, FDA/EMA guidelines) and are transparent, explainable, and ethically deployed (22). Encouraging interdisciplinary collaboration among data scientists, clinicians, regulatory bodies, and patient advocacy groups will be key to developing trustworthy AI-driven healthcare solutions that benefit all populations.
7. Addressing patient privacy and security concerns in data sharing: Patient data stored on cloud services is vulnerable to breaches, causing privacy concerns that limit data sharing and hinder research (235, 236). Privacy-preserving solutions, such as blockchain and federated learning, should be implemented to protect patient data and encourage widespread adoption (234, 237, 238). Future research should focus on frameworks integrating these technologies to alleviate privacy concerns, encourage data sharing, and improve the diversity and robustness of datasets, enhancing diabetes prediction and management.
8. Exploring the role of generative AI: Generative AI, including Large Language Models (LLMs) and Generative Adversarial Networks (GANs), has emerged as a transformative technology with significant potential in healthcare. In T2DM research, generative AI can be leveraged to synthesize realistic patient data, augmenting limited datasets and improving model generalizability. For instance, GANs can generate synthetic EHRs that preserve patient privacy while enhancing the diversity and size of training datasets (239). LLMs like GPT-4 can assist in clinical decision-making by providing personalized recommendations based on patient history and real-time data (240). Future research should explore the integration of generative AI with predictive models to improve their robustness, scalability, and applicability across diverse populations. Additionally, multi-modal AI approaches, integrating text, images, and structured health records, could enhance prediction accuracy and provide a more holistic understanding of diabetes progression (241). However, ethical considerations, such as ensuring data authenticity and mitigating bias in generated data, must be addressed to fully realize the potential of generative AI in T2DM management (242, 243).
9. Enhancing pointwise reliability: Beyond overall model accuracy, an equally crucial aspect is pointwise reliability, which refers to assessing the trustworthiness of each individual prediction before it is used in clinical decision-making. Ensuring pointwise reliability is essential for integrating ML models into real-world healthcare settings, where incorrect predictions can have significant consequences (232, 244). To enhance pointwise reliability, uncertainty quantification techniques should be employed. Bayesian neural networks, for example, estimate uncertainty by treating model parameters as probability distributions rather than fixed values (245), while conformal prediction provides mathematically rigorous confidence intervals for individual predictions (246). Additionally, ML models often produce probability estimates that may not align with real-world likelihoods. Therefore, calibration techniques, such as Platt scaling (247), isotonic regression (248), and temperature scaling (249), should be explored to adjust model outputs and improve their interpretability. Another approach to enhancing reliability is the computation of trust scores, which measure how similar a given patient’s data is to the training distribution, helping clinicians gauge confidence in each prediction (250). Future research should focus on enhancing AI-driven healthcare solutions by integrating out-of-distribution (OOD) detection with a human-in-the-loop approach and developing clinical decision support systems (CDSS) that incorporate confidence scores and reliability indicators. This will enable the identification of novel or unexpected data, ensure expert intervention for uncertain predictions, and provide clinically actionable insights with measurable confidence.
This study provides a comprehensive bibliometric and literature analysis of ML and AI applications in T2DM prediction over a 33-year period (1991–2024). By analyzing publication trends, thematic clusters, research methodologies, and emerging technologies, we highlight the transformative impact of AI-driven predictive modeling in diabetes research. Our findings indicate a significant shift in research focus, from traditional statistical models in the 1990s to sophisticated ensemble learning and deep learning techniques in recent years. The exponential growth in publications, particularly post-2010, underscores the increasing interest and technological advancements in this domain. However, despite these advancements, several challenges persist. The reliance on a limited number of datasets, lack of model generalizability across diverse populations, and insufficient integration of psychosocial and lifestyle factors hinder the full potential of AI in clinical applications. Moreover, while ML models have shown promising accuracy in T2DM prediction, their adoption in real-world clinical settings remains limited. The increasing use of explainability tools, such as SHAP and LIME, represents a step forward in bridging the gap between AI-driven predictions and clinical decision-making. However, ensuring model interpretability, ethical considerations, and patient-centric outcomes will be crucial for widespread adoption. Future research should prioritize interdisciplinary collaborations, integrating insights from epidemiology, genetics, lifestyle sciences, and computational intelligence. Additionally, efforts should be directed towards developing clinically actionable AI models that enhance early detection, personalized interventions, and ultimately, improved patient outcomes. Addressing these gaps will pave the way for a more effective and equitable application of AI in diabetes prevention and management. By systematically mapping the evolution of ML in T2DM prediction, this study serves as a foundational resource for researchers, clinicians, and policymakers. As AI continues to advance, a collaborative, data-driven, and patient-centered approach will be essential in mitigating the global burden of diabetes and improving healthcare outcomes.
MK: Conceptualization, Data curation, Formal analysis, Investigation, Methodology, Software, Validation, Visualization, Writing – original draft, Writing – review & editing. YX: Conceptualization, Funding acquisition, Project administration, Resources, Supervision, Writing – review & editing. NA: Formal analysis, Investigation, Methodology, Validation, Writing – review & editing. GB: Conceptualization, Funding acquisition, Methodology, Project administration, Resources, Supervision, Validation, Writing – review & editing. BP: Conceptualization, Formal analysis, Methodology, Project administration, Validation, Writing – review & editing. DR: Funding acquisition, Resources, Writing – review & editing.
The authors declare that no financial support was received for the research and/or publication of this article.
We would like to extend our gratitude to all individuals and organizations that supported this research. We especially acknowledge the contributions of our institutional and academic colleagues for their invaluable insights and feedback during the research process.
The authors declare that the research was conducted in the absence of any commercial or financial relationships that could be construed as a potential conflict of interest. The authors declared that they were an editorial board member of Frontiers, at the time of submission. This had no impact on the peer review process and the final decision.
The author(s) declare that no Generative AI was used in the creation of this manuscript.
All claims expressed in this article are solely those of the authors and do not necessarily represent those of their affiliated organizations, or those of the publisher, the editors and the reviewers. Any product that may be evaluated in this article, or claim that may be made by its manufacturer, is not guaranteed or endorsed by the publisher.
1. ^More details on the methodology can be found in (45).
1. Zhu T, Li K, Herrero P, Georgiou P. Deep learning for diabetes: a systematic review. IEEE J Biomed Health Inform. (2020) 25:2744–57. doi: 10.1109/JBHI.2020.3040225
2. Shamanna P, Joshi S, Shah L, Dharmalingam M, Saboo B, Mohammed J, et al. Type 2 diabetes reversal with digital twin technology-enabled precision nutrition and staging of reversal: a retrospective cohort study. Clin Diabetes Endocrinol. (2021) 7:21. doi: 10.1186/s40842-021-00134-7
3. IDF. Data from: Diabetes facts and figures: IDF diabetes atlas tenth edition 2021 (2021). Available at: https://idf.org/aboutdiabetes/what-is-diabetes/facts-figures.html (Accessed November 23, 2022).
4. Kavakiotis I, Tsave O, Salifoglou A, Maglaveras N, Vlahavas I, Chouvarda I. Machine learning and data mining methods in diabetes research. Comput Struct Biotechnol J. (2017) 15:104–16. doi: 10.1016/j.csbj.2016.12.005
5. Anderson JP, Parikh JR, Shenfeld DK, Ivanov V, Marks C, Church BW, et al. Reverse engineering and evaluation of prediction models for progression to type 2 diabetes: an application of machine learning using electronic health records. J Diabetes Sci Technol. (2016) 10:6–18. doi: 10.1177/1932296815620200
6. Hu P, Li X, Lu N, Dong K, Bai X, Liang T, et al. Prediction of new-onset diabetes after pancreatectomy with subspace clustering based multi-view feature selection. IEEE J Biomed Health Inform. (2023) 27:1588–99. doi: 10.1109/JBHI.2022.3233402
7. Lekha S, Suchetha M. Recent advancements and future prospects on e-nose sensors technology and machine learning approaches for non-invasive diabetes diagnosis: a review. IEEE Rev Biomed Eng. (2020) 14:127–38. doi: 10.1109/RBME.2020.2993591
8. Sharma T, Shah M. A comprehensive review of machine learning techniques on diabetes detection. Vis Comput Ind Biomed Art. (2021) 4:1–16. doi: 10.1186/s42492-021-00097-7
9. Lu HY, Ding X, Hirst JE, Yang Y, Yang J, Mackillop L, et al. Digital health and machine learning technologies for blood glucose monitoring and management of gestational diabetes. IEEE Rev Biomed Eng. (2023) 17:98–117. doi: 10.1109/RBME.2023.3242261
10. Siddiqui SA, Zhang Y, Lloret J, Song H, Obradovic Z. Pain-free blood glucose monitoring using wearable sensors: Recent advancements and future prospects. IEEE Rev Biomed Eng. (2018) 11:21–35. doi: 10.1109/RBME.2018.2822301
11. Jaiswal V, Negi A, Pal T. A review on current advances in machine learning based diabetes prediction. Prim Care Diabetes. (2021) 15:435–43. doi: 10.1016/j.pcd.2021.02.005
12. Theis J, Galanter WL, Boyd AD, Darabi H. Improving the in-hospital mortality prediction of diabetes icu patients using a process mining/deep learning architecture. IEEE J Biomed Health Inform. (2021) 26:388–99. doi: 10.1109/JBHI.2021.3092969
13. Khedkar V, Patel S. Diabetes prediction using machine learning: a bibliometric analysis. Libr Philos Pract. (2021) 2021:4751.
14. Krishnamoorthy G, Ramakrishnan J, Devi S. Bibliometric analysis of literature on diabetes (1995–2004). (2009).
15. Wang Y, Wang C, Zheng L. Bibliometric analysis of systematic review and meta-analysis on diabetic foot ulcer. Heliyon. (2024) 10:e27534. doi: 10.1016/j.heliyon.2024.e27534
16. García-Jaramillo M, Luque C, León-Vargas F. Machine learning and deep learning techniques applied to diabetes research: a bibliometric analysis. J Diabetes Sci Technol. (2024) 18:287–301. doi: 10.1177/19322968231215350
17. Qaiser S, Ali R. Text mining: use of tf-idf to examine the relevance of words to documents. Int J Comput Appl. (2018) 181:25–9. doi: 10.5120/ijca2018917395
18. Aria M, Cuccurullo C. Data from: Bibliometrix: an r-tool for comprehensive science mapping analysis. Website 2024. (2017).
19. Van Eck N, Waltman L. Software survey: vosviewer, a computer program for bibliometric mapping. Scientometrics. (2010) 84:523–38. doi: 10.1007/s11192-009-0146-3
20. King MR. The future of ai in medicine: a perspective from a chatbot. Ann Biomed Eng. (2023) 51:291–5. doi: 10.1007/s10439-022-03121-w
21. Byrne DW, Domenico HJ, Moore RP. Artificial intelligence for improved patient outcomes—the pragmatic randomized controlled trial is the secret sauce. Korean J Radiol. (2024) 25:123. doi: 10.3348/kjr.2023.1016
22. Topol E. High-performance medicine: the convergence of human and artificial intelligence. Nat Med. (2019) 25:44–56. doi: 10.1038/s41591-018-0300-7
23. Vollmer S, Mateen BA, Bohner G, Király FJ, Ghani R, Jonsson P, et al. Machine learning and artificial intelligence research for patient benefit: 20 critical questions on transparency, replicability, ethics, and effectiveness. BMJ. (2020) 368:m1312. doi: 10.1136/bmj.l6927
24. Saxena R, Sharma SK, Gupta M, Sampada G. [Retracted] a comprehensive review of various diabetic prediction models: a literature survey. J Healthc Eng. (2022) 2022:8100697. doi: 10.1155/2022/8100697
25. Holzinger A, Langs G, Denk H, Zatloukal K, Müller H. Causability and explainability of artificial intelligence in medicine. Wiley Interdiscip Rev Data Min Knowl Discov. (2019) 9:e1312. doi: 10.1002/widm.1312
26. Mohsen F, Al-Absi HR, Yousri NA, El Hajj N, Shah Z. A scoping review of artificial intelligence-based methods for diabetes risk prediction. NPJ Digit Med. (2023) 6:197. doi: 10.1038/s41746-023-00933-5
27. Hasan R, Dattana V, Mahmood S, Hussain S. Towards transparent diabetes prediction: combining automl and explainable AI for improved clinical insights. Information. (2024) 16:7. doi: 10.3390/info16010007
28. Khokhar PB, Pentangelo V, Palomba F, Gravino C. Towards transparent and accurate diabetes prediction using machine learning and explainable artificial intelligence. arXiv [Preprint]. arXiv:2501.18071 (2025).
29. Fortunato S, Bergstrom CT, Börner K, Evans JA, Helbing D, Milojević S, et al. Science of science. Science. (2018) 359:eaao0185. doi: 10.1126/science.aao0185
30. Munafò MR, Nosek BA, Bishop DV, Button KS, Chambers CD, Percie du Sert N, et al. A manifesto for reproducible science. Nat Hum Behav. (2017) 1:1–9.
31. Aldousari E, Kithinji D. Artificial intelligence and health information: a bibliometric analysis of three decades of research. Health Inform J. (2024) 30:14604582241283969. doi: 10.1177/14604582241283969
32. Li J, Hale A. Identification of, and knowledge communication among core safety science journals. Saf Sci. (2015) 74:70–8. doi: 10.1016/j.ssci.2014.12.003
33. Chawla NV, Bowyer KW, Hall LO, Kegelmeyer WP. Smote: synthetic minority over-sampling technique. J Artif Intell Res. (2002) 16:321–57. doi: 10.1613/jair.953
34. Pedregosa F, Varoquaux G, Gramfort A, Michel V, Thirion B, Grisel O, et al. Scikit-learn: machine learning in python. J Mach Learn Res. (2011) 12:2825–30. doi: 10.5555/1953048.2078195
35. Group DPPR. Reduction in the incidence of type 2 diabetes with lifestyle intervention or metformin. New Engl J Med. (2002) 346:393–403. doi: 10.1056/NEJMoa012512
36. Lindstrom J, Tuomilehto J. The diabetes risk score: a practical tool to predict type 2 diabetes risk. Diabetes Care. (2003) 26:725–31. doi: 10.2337/diacare.26.3.725
37. Wild S, Roglic G, Green A, Sicree R, King H. Global prevalence of diabetes: estimates for the year 2000 and projections for 2030. Diabetes Care. (2004) 27:1047–53. doi: 10.2337/diacare.27.5.1047
38. Dinh A, Miertschin S, Young A, Mohanty SD. A data-driven approach to predicting diabetes and cardiovascular disease with machine learning. BMC Med Inform Decis Mak. (2019) 19:1–15. doi: 10.1186/s12911-019-0918-5
39. Lai H, Huang H, Keshavjee K, Guergachi A, Gao X. Predictive models for diabetes mellitus using machine learning techniques. BMC Endocr Disord. (2019) 19:1–9. doi: 10.1186/s12902-019-0436-6
40. Dagliati A, Marini S, Sacchi L, Cogni G, Teliti M, Tibollo V, et al. Machine learning methods to predict diabetes complications. J Diabetes Sci Technol. (2018) 12:295–302. doi: 10.1177/1932296817706375
41. Zou Q, Qu K, Luo Y, Yin D, Ju Y, Tang H. Predicting diabetes mellitus with machine learning techniques. Front Genet. (2018) 9:515. doi: 10.3389/fgene.2018.00515
42. Kopitar L, Kocbek P, Cilar L, Sheikh A, Stiglic G. Early detection of type 2 diabetes mellitus using machine learning-based prediction models. Sci Rep. (2020) 10:11981. doi: 10.1038/s41598-020-68771-z
43. Kandhasamy JP, Balamurali S. Performance analysis of classifier models to predict diabetes mellitus. Procedia Comput Sci. (2015) 47:45–51. doi: 10.1016/j.procs.2015.03.182
44. Sneha N, Gangil T. Analysis of diabetes mellitus for early prediction using optimal features selection. J Big Data. (2019) 6:1–19. doi: 10.1186/s40537-019-0175-6
45. Keupp MM, Palmié M, Gassmann O. The strategic management of innovation: a systematic review and paths for future research. Int J Manage Rev. (2012) 14:367–90. doi: 10.1111/j.1468-2370.2011.00321.x
46. Bellazzi R, Larizza C, Magni P, Montani S, Stefanelli M. Intelligent analysis of clinical time series: an application in the diabetes mellitus domain. Artif Intell Med. (2000) 20:37–57. doi: 10.1016/S0933-3657(00)00052-X
47. Lehmann E, Deutsch T. Computer assisted diabetes care: a 6-year retrospective. Comput Methods Programs Biomed. (1996) 50:209–30. doi: 10.1016/0169-2607(96)01751-8
48. Ramoni M, Riva A, Stefanelli M, Patel V. An ignorant belief network to forecast glucose concentration from clinical databases. Artif Intell Med. (1995) 7:541–59. doi: 10.1016/0933-3657(95)00026-1
49. Tresp V, Briegel T, Moody J. Neural-network models for the blood glucose metabolism of a diabetic. IEEE Trans Neural Netw. (1999) 10:1204–13. doi: 10.1109/72.788659
50. Shanker MS. Using neural networks to predict the onset of diabetes mellitus. J Chem Inf Comput Sci. (1996) 36:35–41. doi: 10.1021/ci950063e
52. Balakrishnan S, Narayanaswamy R. Feature selection using fcbf in type ii diabetes databases. Int J Comput Internet Manage. (2009) 17:50–8.
53. Bhat VH, Rao PG, Shenoy PD, Venugopal K, Patnaik LM. An efficient prediction model for diabetic database using soft computing techniques. In: International Workshop on Rough Sets, Fuzzy Sets, Data Mining, and Granular-Soft Computing. Springer (2009). p. 328–35.
54. Breault JL. Data mining diabetic databases: are rough sets a useful addition. Comput Sci Stat. (2001) 34:54.
55. Ilango BS, Ramaraj N. A hybrid prediction model with f-score feature selection for type II diabetes databases. In: Proceedings of the 1st Amrita ACM-W Celebration on Women in Computing in India. (2010). p. 1–4.
56. Kahramanli H, Allahverdi N. Design of a hybrid system for the diabetes and heart diseases. Expert Syst Appl. (2008) 35:82–9. doi: 10.1016/j.eswa.2007.06.004
57. Kayaer K, Yildirim T. Medical diagnosis on Pima Indian diabetes using general regression neural networks. In: Proceedings of the International Conference on Artificial Neural Networks and Neural Information Processing (ICANN/ICONIP). (2003). Vol. 181. p. 184.
58. Liang W. Approaches to Diabetes Data Mining. Ottawa: National Library of Canada, Bibliotheque Nationale Du Canada (2004).
59. Patil BM, Joshi RC, Toshniwal D. Hybrid prediction model for type-2 diabetic patients. Expert Syst Appl. (2010) 37:8102–8. doi: 10.1016/j.eswa.2010.05.078
60. Patil BM, Joshi RC, Toshniwal D. Impact of k-means on the performance of classifiers for labeled data. In: International Conference on Contemporary Computing. Springer (2010). p. 423–34.
61. Pham HNA, Triantaphyllou E. Prediction of diabetes by employing a new data mining approach which balances fitting and generalization. In: Computer and Information Science. Springer (2008). p. 11–26.
62. Wu J, Diao Y-B, Li M-L, Fang Y-P, Ma D-C. A semi-supervised learning based method: Laplacian support vector machine used in diabetes disease diagnosis. Interdiscip Sci Comput Life Sci. (2009) 1:151–5. doi: 10.1007/s12539-009-0016-2
64. Bellazzi R, Abu-Hanna A. Data mining technologies for blood glucose and diabetes management. J Diabetes Sci Technol. (2009) 3:603–12. doi: 10.1177/193229680900300326
65. Jaafar SFB, Ali DM. Diabetes mellitus forecast using artificial neural network (ANN). In: 2005 Asian Conference on Sensors and the International Conference on New Techniques in Pharmaceutical and Biomedical Research. IEEE (2005). p. 135–9.
66. Patil B, Joshi R, Toshniwal D. Association rule for classification of type-2 diabetic patients. In: 2010 Second International Conference on Machine Learning and Computing. IEEE (2010). p. 330–4.
67. Yu W, Liu T, Valdez R, Gwinn M, Khoury MJ. Application of support vector machine modeling for prediction of common diseases: the case of diabetes and pre-diabetes. BMC Med Inform Decis Mak. (2010) 10:1–7. doi: 10.1186/1472-6947-10-16
68. Ban H-J, Heo JY, Oh K-S, Park K-J. Identification of type 2 diabetes-associated combination of snps using support vector machine. BMC Genet. (2010) 11:1–11. doi: 10.1186/1471-2156-11-26
69. Patil BM, Joshi RC, Toshniwal D. Hybrid prediction model for type-2 diabetic patients. Expert Syst Appl. (2010) 37:8102–8. doi: 10.1016/j.eswa.2010.05.078
70. Barakat N, Bradley AP, Barakat MNH. Intelligible support vector machines for diagnosis of diabetes mellitus. IEEE Trans Inf Technol Biomed. (2010) 14:1114–20. doi: 10.1109/TITB.2009.2039485
71. Sharifi A, Vosolipour A, Sh MA, Teshnehlab M. Hierarchical takagi-sugeno type fuzzy system for diabetes mellitus forecasting. In: 2008 International Conference on Machine Learning and Cybernetics. IEEE (2008). Vol. 3. p. 1265–70.
72. Li J, Huang Q, Dong M, Qiu W, Jiang L, Luo X, et al. Construction of risk prediction model of type 2 diabetes mellitus based on logistic regression. In: BIO Web of Conferences. EDP Sciences (2017). Vol. 8. p. 02002.
73. Mani S, Chen Y, Elasy T, Clayton W, Denny J. Type 2 diabetes risk forecasting from EMR data using machine learning. In: AMIA Annual Symposium Proceedings. American Medical Informatics Association (2012). Vol. 2012. p. 606.
74. Razavian N, Blecker S, Schmidt AM, Smith-McLallen A, Nigam S, Sontag D. Population-level prediction of type 2 diabetes from claims data and analysis of risk factors. Big Data. (2015) 3:277–87. doi: 10.1089/big.2015.0020
75. Tigga NP, Garg S. Prediction of type 2 diabetes using machine learning classification methods. Procedia Comput Sci. (2020) 167:706–16. doi: 10.1016/j.procs.2020.03.336
76. Zhang L, Wang Y, Niu M, Wang C, Wang Z. Machine learning for characterizing risk of type 2 diabetes mellitus in a rural chinese population: the henan rural cohort study. Sci Rep. (2020) 10:1–10. doi: 10.1038/s41598-020-61123-x
77. Bernardini M, Morettini M, Romeo L, Frontoni E, Burattini L. Early temporal prediction of type 2 diabetes risk condition from a general practitioner electronic health record: a multiple instance boosting approach. Artif Intell Med. (2020) 105:101847. doi: 10.1016/j.artmed.2020.101847
78. Farran B, AlWotayan R, Alkandari H, Al-Abdulrazzaq D, Channanath A, Thanaraj TA. Use of non-invasive parameters and machine-learning algorithms for predicting future risk of type 2 diabetes: a retrospective cohort study of health data from kuwait. Front Endocrinol. (2019) 10:624. doi: 10.3389/fendo.2019.00624
79. Muhammad L, Algehyne EA, Usman SS. Predictive supervised machine learning models for diabetes mellitus. SN Comput Sci. (2020) 1:240. doi: 10.1007/s42979-020-00250-8
80. Zheng T, Xie W, Xu L, He X, Zhang Y, You M, et al. A machine learning-based framework to identify type 2 diabetes through electronic health records. Int J Med Inform. (2017) 97:120–7. doi: 10.1016/j.ijmedinf.2016.09.014
81. Mujumdar A, Vaidehi V. Diabetes prediction using machine learning algorithms. Procedia Comput Sci. (2019) 165:292–9. doi: 10.1016/j.procs.2020.01.047
82. Tripathi G, Kumar R. Early prediction of diabetes mellitus using machine learning. In: 2020 8th International Conference on Reliability, Infocom Technologies and Optimization (Trends and Future Directions) (ICRITO). IEEE (2020). p. 1009–14.
83. Chen W, Chen S, Zhang H, Wu T. A hybrid prediction model for type 2 diabetes using k-means and decision tree. In: 2017 8th IEEE International Conference on Software Engineering and Service Science (ICSESS). IEEE (2017). p. 386–90.
84. Kannadasan K, Edla DR, Kuppili V. Type 2 diabetes data classification using stacked autoencoders in deep neural networks. Clin Epidemiol Glob Health. (2019) 7:530–5. doi: 10.1016/j.cegh.2018.12.004
85. Nadesh RK, Arivuselvan K. Type 2: diabetes mellitus prediction using deep neural networks classifier. Int J Cogn Comput Eng. (2020) 1:55–61. doi: 10.1016/j.ijcce.2020.10.002
86. Wu H, Yang S, Huang Z, He J, Wang X. Type 2 diabetes mellitus prediction model based on data mining. Inform Med Unlocked. (2018) 10:100–7. doi: 10.1016/j.imu.2017.12.006
87. Xu Z, Wang Z. A risk prediction model for type 2 diabetes based on weighted feature selection of random forest and xgboost ensemble classifier. In: 2019 Eleventh International Conference on Advanced Computational Intelligence (ICACI). IEEE (2019). p. 278–83.
88. Bernardini M, Romeo L, Misericordia P, Frontoni E. Discovering the type 2 diabetes in electronic health records using the sparse balanced support vector machine. IEEE J Biomed Health Inform. (2019) 24:235–46. doi: 10.1109/JBHI.2019.2899218
89. Casanova R, Saldana S, Simpson SL, Lacy ME, Subauste AR, Blackshear C, et al. Prediction of incident diabetes in the jackson heart study using high-dimensional machine learning. PLoS One. (2016) 11:e0163942. doi: 10.1371/journal.pone.0163942
90. Lee BJ, Kim JY. Identification of type 2 diabetes risk factors using phenotypes consisting of anthropometry and triglycerides based on machine learning. IEEE J Biomed Health Inform. (2015) 20:39–46. doi: 10.1109/JBHI.2015.2396520
91. Liu Y, Ye S, Xiao X, Sun C, Wang G, Wang G, et al. Machine learning for tuning, selection, and ensemble of multiple risk scores for predicting type 2 diabetes. Risk Manag Healthc Policy. (2019) 12:189–98. doi: 10.2147/RMHP.S225762
92. Hu H, Nakagawa T, Yamamoto S, Honda T, Okazaki H, Uehara A, et al. Development and validation of risk models to predict the 7-year risk of type 2 diabetes: the Japan epidemiology collaboration on occupational health study. J Diabetes Invest. (2018) 9:1052–9. doi: 10.1111/jdi.12809
93. Ozery-Flato M, Parush N, El-Hay T, Visockienė Ž, Ryliškytė L, Badarienė J, et al. Predictive models for type 2 diabetes onset in middle-aged subjects with the metabolic syndrome. Diabetol Metab Syndr. (2013) 5:1–9. doi: 10.1186/1758-5996-5-36
94. Lee J-W, Lim N-K, Park H-Y. The product of fasting plasma glucose and triglycerides improves risk prediction of type 2 diabetes in middle-aged Koreans. BMC Endocr Disord. (2018) 18:1–10. doi: 10.1186/s12902-018-0259-x
95. Nai-Arun N, Moungmai R. Comparison of classifiers for the risk of diabetes prediction. Procedia Comput Sci. (2015) 69:132–42. doi: 10.1016/j.procs.2015.10.014
96. Bagherzadeh-Khiabani F, Ramezankhani A, Azizi F, Hadaegh F, Steyerberg EW, Khalili D. A tutorial on variable selection for clinical prediction models: feature selection methods in data mining could improve the results. J Clin Epidemiol. (2016) 71:76–85. doi: 10.1016/j.jclinepi.2015.10.002
97. Farran B, Channanath AM, Behbehani K, Thanaraj TA. Predictive models to assess risk of type 2 diabetes, hypertension and comorbidity: machine-learning algorithms and validation using national health data from Kuwait—a cohort study. BMJ Open. (2013) 3:e002457. doi: 10.1136/bmjopen-2012-002457
98. Meng X-H, Huang Y-X, Rao D-P, Zhang Q, Liu Q. Comparison of three data mining models for predicting diabetes or prediabetes by risk factors. Kaohsiung J Med Sci. (2013) 29:93–9. doi: 10.1016/j.kjms.2012.08.016
99. Perveen S, Shahbaz M, Guergachi A, Keshavjee K. Performance analysis of data mining classification techniques to predict diabetes. Procedia Comput Sci. (2016) 82:115–21. doi: 10.1016/j.procs.2016.04.016
100. Kumar PM, Lokesh S, Varatharajan R, Babu GC, Parthasarathy P. Cloud and iot based disease prediction and diagnosis system for healthcare using fuzzy neural classifier. Future Gener Comput Syst. (2018) 86:527–34. doi: 10.1016/j.future.2018.04.036
101. Nilashi M, Ahmadi H, Shahmoradi L. An analytical method for diseases prediction using machine learning techniques. Comput Chem Eng. (2017) 106:212–23. doi: 10.1016/j.compchemeng.2017.06.011
102. Hasan MK, Alam MA, Das D, Hossain E, Hasan M. Diabetes prediction using ensembling of different machine learning classifiers. IEEE Access. (2020) 8:76516–31. doi: 10.1109/ACCESS.2020.2989857
103. Alghamdi M, Al-Mallah M, Keteyian S, Brawner C, Ehrman J, Sakr S. Predicting diabetes mellitus using smote and ensemble machine learning approach: the henry ford exercise testing (fit) project. PLoS One. (2017) 12:e0179805. doi: 10.1371/journal.pone.0179805
104. Arellano-Campos O, Gómez-Velasco DV, Bello-Chavolla OY, Cruz-Bautista I, Melgarejo-Hernandez MA, Muñoz-Hernandez L, et al. Development and validation of a predictive model for incident type 2 diabetes in middle-aged mexican adults: the metabolic syndrome cohort. BMC Endocr Disord. (2019) 19:1–10. doi: 10.1186/s12902-019-0361-8
105. Songthung P, Sripanidkulchai K. Improving type 2 diabetes mellitus risk prediction using classification. In: 2016 13th International Joint Conference on Computer Science and Software Engineering (JCSSE). IEEE (2016). p. 1–6.
106. Ashtagi R, Dhumale P, Mane D, Naveen H, Bidwe RV, Zope B. Iot-based hybrid ensemble machine learning model for efficient diabetes mellitus prediction. Int J Intell Syst Appl Eng. (2023) 11:714–26. doi: 10.1155/2022/2389636
107. Hennebelle A, Materwala H, Ismail L. Healthedge: a machine learning-based smart healthcare framework for prediction of type 2 diabetes in an integrated iot, edge, and cloud computing system. Procedia Comput Sci. (2023) 220:331–8. doi: 10.1016/j.procs.2023.03.043
108. Patel MS, Polsky D, Small DS, Park S-H, Evans CN, Harrington T, et al. Predicting changes in glycemic control among adults with prediabetes from activity patterns collected by wearable devices. NPJ Digit Med. (2021) 4:1–7. doi: 10.1038/s41746-021-00541-1
109. Yun J-S, Kim J, Jung S-H, Cha S-A, Ko S-H, Ahn Y-B, et al. A deep learning model for screening type 2 diabetes from retinal photographs. Nutr Metab Cardiovasc Dis. (2022) 32:1218–26. doi: 10.1016/j.numecd.2022.01.010
110. Ahmad HF, Mukhtar H, Alaqail H, Seliaman M, Alhumam A. Investigating health-related features and their impact on the prediction of diabetes using machine learning. Appl Sci. (2021) 11:1173. doi: 10.3390/app11031173
111. Al-Tawil M, Mahafzah BA, Al Tawil A, Aljarah I. Bio-inspired machine learning approach to type 2 diabetes detection. Symmetry. (2023) 15:764. doi: 10.3390/sym15030764
112. Gollapalli M, Alansari A, Alkhorasani H, Alsubaii M, Sakloua R, Alzahrani R, et al. A novel stacking ensemble for detecting three types of diabetes mellitus using a Saudi Arabian dataset: pre-diabetes, T1DM, and T2DM. Comput Biol Med. (2022) 147:105757. doi: 10.1016/j.compbiomed.2022.105757
113. Lu H, Uddin S, Hajati F, Moni MA, Khushi M. A patient network-based machine learning model for disease prediction: the case of type 2 diabetes mellitus. Appl Intell. (2022) 52:2411–22. doi: 10.1007/s10489-021-02533-w
114. Suryadevara CK. Diabetes risk assessment using machine learning: a comparative study of classification algorithms. IEJRD-Int Multidiscip J. (2023) 8:10.
115. Ginting JB, Suci T, Ginting CN, Girsang E. Early detection system of risk factors for diabetes mellitus type 2 utilization of machine learning-random forest. J Fam Community Med. (2023) 30:171–9. doi: 10.4103/jfcm.jfcm_33_23
116. Li J, Ding J, Zhi D, Gu K, Wang H. Identification of type 2 diabetes based on a ten-gene biomarker prediction model constructed using a support vector machine algorithm. Biomed Res Int. (2022) 2022:1230761. doi: 10.1155/2022/1230761
117. Lugner M, Rawshani A, Helleryd E, Eliasson B. Identifying top ten predictors of type 2 diabetes through machine learning analysis of UK biobank data. Sci Rep. (2024) 14:2102. doi: 10.1038/s41598-024-52023-5
118. Nagassou M, Mwangi RW, Nyarige E. A hybrid ensemble learning approach utilizing light gradient boosting machine and category boosting model for lifestyle-based prediction of type-II diabetes mellitus. J Data Anal Inform Process. (2023) 11:480–511. doi: 10.4236/jdaip.2023.114025
119. Ooka T, Johno H, Nakamoto K, Yoda Y, Yokomichi H, Yamagata Z. Random forest approach for determining risk prediction and predictive factors of type 2 diabetes: large-scale health check-up data in Japan. BMJ Nutr Prev Health. (2021) 4:140. doi: 10.1136/bmjnph-2020-000200
120. Ramadhan NG, Romadhony A. Preprocessing handling to enhance detection of type 2 diabetes mellitus based on random forest. Int J Adv Comput Sci Appl. (2021) 12:223–8. doi: 10.14569/IJACSA.2021.0120726
121. Ramotra K, Mansotra V. Hybrid type-2 diabetes prediction model using smote, k-means clustering, pca, and logistic regression. Asian Pac J Health Sci. (2021) 8:137–40. doi: 10.21276/apjhs.2021.8.3.23
122. Shrestha B, Alsadoon A, Prasad P, Al-Naymat G, Al-Dala’in T, Rashid TA, et al. Enhancing the prediction of type 2 diabetes mellitus using sparse balanced svm. Multimed Tools Appl. (2022) 81:38945–69. doi: 10.1007/s11042-022-13087-5
123. Shrestha M, Alsadoon OH, Alsadoon A, Al-Dala’in T, Rashid TA, Prasad P, et al. A novel solution of deep learning for enhanced support vector machine for predicting the onset of type 2 diabetes. Multimed Tools Appl. (2023) 82:6221–41. doi: 10.1007/s11042-022-13582-9
124. Suriya S, Muthu J. Type 2 diabetes prediction using k-nearest neighbor algorithm. J Trends Comput Sci Smart Technol. (2023) 5:190–205. doi: 10.36548/jtcsst.2023.2.007
125. Waberi AD, Mwangi RW, Rimiru RM. Advancing type II diabetes predictions with a hybrid lstm-xgboost approach. J Data Anal Inform Process. (2024) 12:163–88. doi: 10.4236/jdaip.2024.122010
126. Deberneh HM, Kim I. Prediction of type 2 diabetes based on machine learning algorithm. Int J Environ Res Public Health. (2021) 18:3317. doi: 10.3390/ijerph18063317
127. Srinivasu PN, Shafi J, Krishna TB, Sujatha CN, Praveen SP, Ijaz MF. Using recurrent neural networks for predicting type-2 diabetes from genomic and tabular data. Diagnostics. (2022) 12:3067. doi: 10.3390/diagnostics12123067
128. Alex SA, Nayahi JJV, Shine H, Gopirekha V. Deep convolutional neural network for diabetes mellitus prediction. Neural Comput Appl. (2022) 34:1319–27. doi: 10.1007/s00521-021-06431-7
129. Chowdary PBK, Kumar RU. An effective approach for detecting diabetes using deep learning techniques based on convolutional lstm networks. Int J Adv Comput Sci Appl. (2021) 12:519–25. doi: 10.14569/IJACSA.2021.0120466
130. Kumari GLA, Padmaja P, Suma JG. A novel method for prediction of diabetes mellitus using deep convolutional neural network and long short-term memory. Indones J Electr Eng Comput Sci. (2022) 26:404–13. doi: 10.11591/ijeecs.v26.i1.pp404-413
131. Sarani Rad F, Hendawi R, Yang X, Li J. Personalized diabetes management with digital twins: a patient-centric knowledge graph approach. J Pers Med. (2024) 14:359. doi: 10.3390/jpm14040359
132. Stiglic G, Wang F, Sheikh A, Cilar L. Development and validation of the type 2 diabetes mellitus 10-year risk score prediction models from survey data. Prim Care Diabetes. (2021) 15:699–705. doi: 10.1016/j.pcd.2021.04.008
133. Lundberg SM, Lee S-I. A unified approach to interpreting model predictions. In: Advances in Neural Information Processing Systems (NeurIPS). (2017). p. 4765–74.
134. Ribeiro MT, Singh S, Guestrin C. “Why should I trust you?”: explaining the predictions of any classifier. In: Proceedings of the 22nd ACM SIGKDD International Conference on Knowledge Discovery and Data Mining. (2016). p. 1135–44.
135. Prendin F, Pavan J, Cappon G, Del Favero S, Sparacino G, Facchinetti A. The importance of interpreting machine learning models for blood glucose prediction in diabetes: an analysis using shap. Sci Rep. (2023) 13:16865. doi: 10.1038/s41598-023-44155-x
136. Vishwarupe V, Joshi PM, Mathias N, Maheshwari S, Mhaisalkar S, Pawar V. Explainable AI and interpretable machine learning: a case study in perspective. Procedia Comput Sci. (2022) 204:869–76. doi: 10.1016/j.procs.2022.08.105
137. Bernstorff M, Hansen L, Enevoldsen K, Damgaard J, Haestrup F, Perfalk E, et al. Development and validation of a machine learning model for prediction of type 2 diabetes in patients with mental illness. Acta Psychiatr Scand. (2024) 151:145–58. doi: 10.1111/acps.13687
138. Lam B, Catt M, Cassidy S, Bacardit J, Darke P, Butterfield S, et al. Using wearable activity trackers to predict type 2 diabetes: machine learning–based cross-sectional study of the UK biobank accelerometer cohort. JMIR Diabetes. (2021) 6:e23364. doi: 10.2196/23364
139. Widen E, Raben TG, Lello L, Hsu SD. Machine learning prediction of biomarkers from snps and of disease risk from biomarkers in the UK biobank. Genes. (2021) 12:991. doi: 10.3390/genes12070991
140. Islam MM, Rahman MJ, Menhazul Abedin M, Ahammed B, Ali M, Ahmed NF, et al. Identification of the risk factors of type 2 diabetes and its prediction using machine learning techniques. Health Syst. (2023) 12:243–54. doi: 10.1080/20476965.2022.2141141
141. Vangeepuram N, Liu B, Chiu P-H, Wang L, Pandey G. Predicting youth diabetes risk using nhanes data and machine learning. Sci Rep. (2021) 11:11212. doi: 10.1038/s41598-021-90406-0
142. Liu Q, Zhou Q, He Y, Zou J, Guo Y, Yan Y. Predicting the 2-year risk of progression from prediabetes to diabetes using machine learning among chinese elderly adults. J Pers Med. (2022) 12:1055. doi: 10.3390/jpm12071055
143. Mansoori A, Sahranavard T, Hosseini ZS, Soflaei SS, Emrani N, Nazar E, et al. Prediction of type 2 diabetes mellitus using hematological factors based on machine learning approaches: a cohort study analysis. Sci Rep. (2023) 13:663. doi: 10.1038/s41598-022-27340-2
144. Ravaut M, Harish V, Sadeghi H, Leung KK, Volkovs M, Kornas K, et al. Development and validation of a machine learning model using administrative health data to predict onset of type 2 diabetes. JAMA Netw Open. (2021) 4:e2111315. doi: 10.1001/jamanetworkopen.2021.11315
145. Ganie SM, Malik MB, Arif T. Performance analysis and prediction of type 2 diabetes mellitus based on lifestyle data using machine learning approaches. J Diabetes Metab Disord. (2022) 21:339–52. doi: 10.1007/s40200-022-00981-w
146. Liu Q, Zhang M, He Y, Zhang L, Zou J, Yan Y, et al. Predicting the risk of incident type 2 diabetes mellitus in chinese elderly using machine learning techniques. J Pers Med. (2022) 12:905. doi: 10.3390/jpm12060905
147. Yang H, Luo Y, Ren X, Wu M, He X, Peng B, et al. Risk prediction of diabetes: big data mining with fusion of multifarious physical examination indicators. Inform Fusion. (2021) 75:140–9. doi: 10.1016/j.inffus.2021.02.015
148. Patil RN, Rawandale S, Rawandale N, Rawandale U, Patil S. An efficient stacking based nsga-II approach for predicting type 2 diabetes. Int J Electr Comput Eng. (2023) 13:1015–23. doi: 10.11591/ijece.v13i1.pp1015-1023
149. Abnoosian K, Farnoosh R, Behzadi MH. Prediction of diabetes disease using an ensemble of machine learning multi-classifier models. BMC Bioinf. (2023) 24:337. doi: 10.1186/s12859-023-05465-z
150. Dritsas E, Trigka M. Data-driven machine-learning methods for diabetes risk prediction. Sensors. (2022) 22:5304. doi: 10.3390/s22145304
151. Ismail L, Materwala H, Tayefi M, Ngo P, Karduck AP. Type 2 diabetes with artificial intelligence machine learning: methods and evaluation. Arch Comput Methods Eng. (2022) 29:313–33. doi: 10.1007/s11831-021-09582-x
152. Chaves L, Marques G. Data mining techniques for early diagnosis of diabetes: a comparative study. Appl Sci. (2021) 11:2218. doi: 10.3390/app11052218
153. Cicek IB, Yologlu S, Sahin I. A comparison of multivariate statistical methods to detect risk factors for type 2 diabetes mellitus. (2023).
154. Joshi RD, Dhakal CK. Predicting type 2 diabetes using logistic regression and machine learning approaches. Int J Environ Res Public Health. (2021) 18:7346. doi: 10.3390/ijerph18147346
155. Roobini M, Lakshmi M. Autonomous prediction of type 2 diabetes with high impact of glucose level. Comput Electr Eng. (2022) 101:108082. doi: 10.1016/j.compeleceng.2022.108082
156. Yasar A. Data classification of early-stage diabetes risk prediction datasets and analysis of algorithm performance using feature extraction methods and machine learning techniques. Int J Intell Syst Appl Eng. (2021) 9:273–81. doi: 10.18201/ijisae.2021473767
157. Khanam JJ, Foo SY. A comparison of machine learning algorithms for diabetes prediction. ICT Express. (2021) 7:432–9. doi: 10.1016/j.icte.2021.02.004
158. Singh A, Dhillon A, Kumar N, Hossain MS, Muhammad G, Kumar M. ediapredict: an ensemble-based framework for diabetes prediction. ACM Trans Multimed Comput Commun Appl. (2021) 17:1–26. doi: 10.1145/341515
159. Islam R, Sultana A, Tuhin MN, Saikat MSH, Islam MR. Clinical decision support system for diabetic patients by predicting type 2 diabetes using machine learning algorithms. J Healthc Eng. (2023) 2023:6992441. doi: 10.1155/2023/6992441
160. Sivaranjani S, Ananya S, Aravinth J, Karthika R. Diabetes prediction using machine learning algorithms with feature selection and dimensionality reduction. In: 2021 7th International Conference on Advanced Computing and Communication Systems (ICACCS). IEEE (2021). Vol. 1. p. 141–6.
161. Ahmed N, Ahammed R, Islam MM, Uddin MA, Akhter A, Talukder MA-A, et al. Machine learning based diabetes prediction and development of smart web application. Int J Cogn Comput Eng. (2021) 2:229–41. doi: 10.1016/j.ijcce.2021.12.001
162. Azad C, Bhushan B, Sharma R, Shankar A, Singh KK, Khamparia A. Prediction model using smote, genetic algorithm and decision tree (PMSGD) for classification of diabetes mellitus. Multimedia Syst. (2022) 28:1289–307. doi: 10.1007/s00530-021-00817-2
163. Mounika V, Neeli DS, Sree GS, Mourya P, Babu MA. Prediction of type-2 diabetes using machine learning algorithms. In: 2021 International Conference on Artificial Intelligence and Smart Systems (ICAIS). IEEE (2021). p. 127–31.
164. Oh R, Lee HK, Pak YK, Oh M-S. An interactive online app for predicting diabetes via machine learning from environment-polluting chemical exposure data. Int J Environ Res Public Health. (2022) 19:5800. doi: 10.3390/ijerph19105800
165. Samet S, Laouar MR, Bendib I. Use of machine learning techniques to predict diabetes at an early stage. In: 2021 International Conference on Networking and Advanced Systems (ICNAS). IEEE (2021). p. 1–6.
166. Nagaraj P, Deepalakshmi P. Diabetes prediction using enhanced svm and deep neural network learning techniques: an algorithmic approach for early screening of diabetes. Int J Healthc Inform Syst Inform. (2021) 16:1–20. doi: 10.4018/IJHISI.20211001.oa25
167. Rajagopal A, Jha S, Alagarsamy R, Quek SG, Selvachandran G. A novel hybrid machine learning framework for the prediction of diabetes with context-customized regularization and prediction procedures. Math Comput Simul. (2022) 198:388–406. doi: 10.1016/j.matcom.2022.03.003
168. Chowdary PBK, Kumar RU. Diabetes classification using an expert neuro-fuzzy feature extraction model. Int J Adv Comput Sci Appl. (2021) 12:368–74. doi: 10.14569/IJACSA.2021.0120842
169. Reshmi S, Biswas SK, Boruah AN, Thounaojam DM, Purkayastha B. Diabetes prediction using machine learning analytics. In: 2022 International Conference on Machine Learning, Big Data, Cloud and Parallel Computing (COM-IT-CON). IEEE (2022). Vol. 1. p. 108–12.
170. Liza FR, Samsuzzaman M, Azim R, Mahmud MZ, Bepery C, Masud MA, et al. An ensemble approach of supervised learning algorithms and artificial neural network for early prediction of diabetes. In: 2021 3rd International Conference on Sustainable Technologies for Industry 4.0 (STI). IEEE (2021). p. 1–6.
171. Marzouk R, Alluhaidan AS. An analytical predictive models and secure web-based personalized diabetes monitoring system. IEEE Access. (2022) 10:105657–73. doi: 10.1109/ACCESS.2022.3211264
172. Panda M, Mishra DP, Patro SM, Salkuti SR. Prediction of diabetes disease using machine learning algorithms. IAES Int J Artif Intell. (2022) 11:284. doi: 10.11591/ijai.v11.i1.pp284-290
173. Ganie SM, Malik MB. An ensemble machine learning approach for predicting type-ii diabetes mellitus based on lifestyle indicators. Healthc Anal. (2022) 2:100092. doi: 10.1016/j.health.2022.100092
174. Olisah CC, Smith L, Smith M. Diabetes mellitus prediction and diagnosis from a data preprocessing and machine learning perspective. Comput Methods Programs Biomed. (2022) 220:106773. doi: 10.1016/j.cmpb.2022.106773
175. Sadeghi S, Khalili D, Ramezankhani A, Mansournia MA, Parsaeian M. Diabetes mellitus risk prediction in the presence of class imbalance using flexible machine learning methods. BMC Med Inform Decis Mak. (2022) 22:36. doi: 10.1186/s12911-022-01775-z
176. Madan P, Singh V, Chaudhari V, Albagory Y, Dumka A, Singh R, et al. An optimization-based diabetes prediction model using CNN and bi-directional LSTM in real-time environment. Appl Sci. (2022) 12:3989. doi: 10.3390/app12083989
177. Kodama S, Fujihara K, Horikawa C, Kitazawa M, Iwanaga M, Kato K, et al. Predictive ability of current machine learning algorithms for type 2 diabetes mellitus: a meta-analysis. J Diabetes Invest. (2022) 13:900–8. doi: 10.1111/jdi.13736
178. Huang L-Y, Chen F-Y, Jhou M-J, Kuo C-H, Wu C-Z, Lu C-H, et al. Comparing multiple linear regression and machine learning in predicting diabetic urine albumin–creatinine ratio in a 4-year follow-up study. J Clin Med. (2022) 11:3661. doi: 10.3390/jcm11133661
179. Xu X, Huang X, Ma J, Luo X. Prediction of diabetes with its symptoms based on machine learning. In: 2021 IEEE International Conference on Computer Science, Artificial Intelligence and Electronic Engineering (CSAIEE). IEEE (2021). p. 147–56.
180. Liu J, Fan L, Jia Q, Wen L, Shi C. Early diabetes prediction based on stacking ensemble learning model. In: 2021 33rd Chinese Control and Decision Conference (CCDC). IEEE (2021). p. 2687–92.
181. Mravik M, Vetriselvi T, Venkatachalam K, Sarac M, Bacanin N, Adamovic S. Diabetes prediction algorithm using recursive ridge regression L2. Comput Mater Continua. (2022) 71:457–71. doi: 10.32604/cmc.2022.020687
182. Qin Y, Wu J, Xiao W, Wang K, Huang A, Liu B, et al. Machine learning models for data-driven prediction of diabetes by lifestyle type. Int J Environ Res Public Health. (2022) 19:15027. doi: 10.3390/ijerph192215027
183. Rajkamal R, Karthi A, Gao X-Z. Diabetes prediction using derived features and ensembling of boosting classifiers. Comput Mater Continua. (2022) 73:2013–33. doi: 10.32604/cmc.2022.027142
184. Wu Y, Hu H, Cai J, Chen R, Zuo X, Cheng H, et al. Machine learning for predicting the 3-year risk of incident diabetes in Chinese adults. Front Public Health. (2021) 9:626331. doi: 10.3389/fpubh.2021.626331
185. Li J, Xu Z, Xu T, Lin S. Predicting diabetes in patients with metabolic syndrome using machine-learning model based on multiple years’ data. Diabetes Metab Syndr Obes Targets Ther. (2022) 15:2951–61. doi: 10.2147/DMSO.S381146
186. Tak A, Punjabi P, Yadav A, Sankhla M, Mathur S, Dave HS, et al. Prediction of type 2 diabetes mellitus using soft computing. Mod Med. (2022) 29:135–43. doi: 10.31689/rmm.2021.29.2.135
187. Chen B, Yan M, Zhong H, He B. Prediction model of diabetes based on machine learning. In: 2021 5th Asian Conference on Artificial Intelligence Technology (ACAIT). IEEE (2021). p. 128–36.
188. Jaiswal S, Gupta P, Prasad LN, Kulkarni R. An empirical model for the classification of diabetes and diabetes_types using ensemble approaches. J Artif Intell Technol. (2023) 3:181–6. doi: 10.37965/jait.2023.0220
189. Aguilera-Venegas G, López-Molina A, Rojo-Martínez G, Galán-García JL. Comparing and tuning machine learning algorithms to predict type 2 diabetes mellitus. J Comput Appl Math. (2023) 427:115115. doi: 10.1016/j.cam.2023.115115
190. Agliata A, Giordano D, Bardozzo F, Bottiglieri S, Facchiano A, Tagliaferri R. Machine learning as a support for the diagnosis of type 2 diabetes. Int J Mol Sci. (2023) 24:6775. doi: 10.3390/ijms24076775
191. Reza MS, Hafsha U, Amin R, Yasmin R, Ruhi S. Improving svm performance for type ii diabetes prediction with an improved non-linear kernel: insights from the pima dataset. Comput Methods Programs Biomed Update. (2023) 4:100118. doi: 10.1016/j.cmpbup.2023.100118
192. Talebi Moghaddam M, Jahani Y, Arefzadeh Z, Dehghan A, Khaleghi M, Sharafi M, et al. Predicting diabetes in adults: identifying important features in unbalanced data over a 5-year cohort study using machine learning algorithm. BMC Med Res Methodol. (2024) 24:220. doi: 10.1186/s12874-024-02341-z
193. Talari PBN, Kaur G, Alshahrani H, Al Reshan MS, Sulaiman A, Shaikh A. Hybrid feature selection and classification technique for early prediction and severity of diabetes type 2. PLoS One. (2024) 19:e0292100. doi: 10.1371/journal.pone.0292100
194. Saha P, Marouf Y, Pozzebon H, Guergachi A, Keshavjee K, Noaeen M, et al. Predicting time to diabetes diagnosis using random survival forests. MedRxiv [Preprint]. (2024).
195. Salih MS, Ibrahim RK, Zeebaree SR, Asaad D, Zebari LM, Abdulkareem NM. Diabetic prediction based on machine learning using pima Indian dataset. Commun Appl Nonlinear Anal. (2024) 31:138–56. doi: 10.52783/cana.v31.1008
196. Chang V, Bailey J, Xu QA, Sun Z. Pima indians diabetes mellitus classification based on machine learning (ML) algorithms. Neural Comput Appl. (2023) 35:16157–73. doi: 10.1007/s00521-022-07049-z
197. Ahmed A, Khan J, Arsalan M, Ahmed K, Shahat A, Alhalmi A, et al. Machine learning algorithm-based prediction of diabetes among female population using pima dataset. Healthcare. (2024) 13:37. doi: 10.3390/healthcare13010037
198. Velu SR, Ravi V, Tabianan K. Machine learning implementation to predict type-2 diabetes mellitus based on lifestyle behaviour pattern using hba1c status. Health Technol. (2023) 13:437–47. doi: 10.1007/s12553-023-00751-5
199. Alsadi B, Musleh S, Al-Absi HR, Refaee M, Qureshi R, El Hajj N, et al. An ensemble-based machine learning model for predicting type 2 diabetes and its effect on bone health. BMC Med Inform Decis Mak. (2024) 24:144. doi: 10.1186/s12911-024-02540-0
200. Xie R, Herder C, Sha S, Peng L, Brenner H, Schöttker B. Novel type 2 diabetes prediction score based on traditional risk factors and circulating metabolites: model derivation and validation in two large cohort studies. eClinicalMedicine. (2024) 79:102971. doi: 10.1016/j.eclinm.2024.102971
201. Saberi-Karimian M, Mansoori A, Bajgiran MM, Hosseini ZS, Kiyoumarsioskouei A, Rad ES, et al. Data mining approaches for type 2 diabetes mellitus prediction using anthropometric measurements. J Clin Lab Anal. (2023) 37:e24798. doi: 10.1002/jcla.24798
202. Ritchie SC, Taylor HJ, Liang Y, Manikpurage HD, Pennells L, Foguet C, et al. Integrated clinical risk prediction of type 2 diabetes with a multifactorial polygenic risk score. medRxiv [Preprint]. 2024.08.22.24312440 (2024). doi: 10.1101/2024.08.22.24312440
203. Rönn T, Perfilyev A, Oskolkov N, Ling C. Predicting type 2 diabetes via machine learning integration of multiple omics from human pancreatic islets. Sci Rep. (2024) 14:14637. doi: 10.1038/s41598-024-64846-3
204. Baig MM, GholamHosseini H, Gutierrez J, Ullah E, Lindén M. Early detection of prediabetes and t2dm using wearable sensors and internet-of-things-based monitoring applications. Appl Clin Inform. (2021) 12:1–9. doi: 10.1055/s-0040-1719043
205. Stolfi P, Valentini I, Palumbo MC, Tieri P, Grignolio A, Castiglione F. Potential predictors of type-2 diabetes risk: machine learning, synthetic data and wearable health devices. BMC Bioinf. (2020) 21:1–19. doi: 10.1186/s12859-020-03763-4
206. Hunag Y, Farid F, Suleiman B. Predicting the relationship between meal frequency and type 2 diabetes: empirical study using machine and deep learning. In: Current and Future Trends in Health and Medical Informatics. Springer (2023). p. 235–57.
207. Björnsson B, Borrebaeck C, Elander N, Gasslander T, Gawel DR, Gustafsson M, et al. Digital twins to personalize medicine. Genome Med. (2019) 12(1):4. doi: 10.1186/s13073-019-0701-3
208. Sun L. Digital twins in healthcare: from data-driven to ai-enabled frameworks. Nat. Mach Intell. (2023) 5:548–62.
209. Katsoulakis E, Wang Q, Wu H, Shahriyari L, Fletcher R, Liu J, et al. Digital twins for health: a scoping review. NPJ Digit Med. (2024) 7:77. doi: 10.1038/s41746-024-01073-0
210. Mansour M, Darweesh MS, Soltan A. Wearable devices for glucose monitoring: a review of state-of-the-art technologies and emerging trends. Alex Eng J. (2024) 89:224–43. doi: 10.1016/j.aej.2024.01.021
211. Rahimi SA, Baradaran A, Khameneifar F, Gore G, Issa AM. Decide-twin: a framework for AI-enabled digital twins in clinical decision-making. IEEE J Biomed Health Inform. (2024):1–10. doi: 10.1109/JBHI.2024.3521717
212. Ooka T. The era of preemptive medicine: developing medical digital twins through omics, IoT, and AI integration. JMA J. (2025) 8:1–10. doi: 10.31662/jmaj.2024-0213
213. Benson M. Digital twins for predictive, preventive personalized, and participatory treatment of immune-mediated diseases. Arterioscler Thromb Vasc Biol. (2023) 43:410–6. doi: 10.1161/ATVBAHA.122.318331
214. De Domenico M, Allegri L, Caldarelli G, Di Camillo B, Rocha LM. Challenges and opportunities for digital twins in precision medicine from a complex systems perspective. npj Digit Med. (2025) 8:37. doi: 10.1038/s41746-024-01402-3
215. Kemkar S, Tao M, Ghosh A, Stamatakos G, Graf N, Poorey K, et al. Towards verifiable cancer digital twins: tissue level modeling protocol for precision medicine. Front Physiol. (2024) 15:1473125. doi: 10.3389/fphys.2024.1473125
216. Zhang Y, Qin G, Aguilar B, Rappaport N, Yurkovich JT, Pflieger L, et al. A framework towards digital twins for type 2 diabetes. Front Digit Health. (2024) 6:1336050. doi: 10.3389/fdgth.2024.1336050
217. He J, Baxter SL, Xu J, Xu J, Zhou X, Zhang K. The practical implementation of artificial intelligence technologies in medicine. Nat Med. (2019) 25:30–6. doi: 10.1038/s41591-018-0307-0
218. Yin J, Ngiam KY, Teo HH. Role of artificial intelligence applications in real-life clinical practice: systematic review. J Med Internet Res. (2021) 23:e25759. doi: 10.2196/25759
219. Rigby MJ. Ethical dimensions of using artificial intelligence in health care. AMA J Ethics. (2019) 21:E121–4. doi: 10.1001/amajethics.2019.121
220. Barredo Arrieta A, Díaz-Rodríguez N, Del Ser J, Bennetot A, Tabik S, Barbado A, et al. Explainable artificial intelligence (XAI): concepts, taxonomies, opportunities, and challenges toward responsible AI. Inform Fusion. (2020) 58:82–115. doi: 10.1016/j.inffus.2019.12.012
221. Xu F, Uszkoreit H, Du Y, Fan W, Zhao D, Zhu J. Explainable AI: a brief survey on history, research areas, approaches and challenges. In: Natural Language Processing and Chinese Computing: 8th cCF International Conference, NLPCC 2019, Dunhuang, China, October 9–14, 2019, Proceedings, Part II 8. Springer (2019). p. 563–74.
222. Carrasco-Zanini J, Pietzner M, Wheeler E, Kerrison ND, Langenberg C, Wareham NJ. Multi-omic prediction of incident type 2 diabetes. Diabetologia. (2024) 67:102–12. doi: 10.1007/s00125-023-06027-x
223. Hasin Y, Seldin M, Lusis A. Multi-omics approaches to disease. Genome Biol. (2017) 18:83. doi: 10.1186/s13059-017-1215-1
224. Mandla R, Lorenz K, Yin X, Bocher O, Huerta-Chagoya A, Arruda AL, et al. Multi-omics characterization of type 2 diabetes associated genetic variation. medRxiv [Preprint]. (2024).
225. Cai Z, Poulos RC, Liu J, Zhong Q. Machine learning for multi-omics data integration in cancer. Iscience. (2022) 25:103798. doi: 10.1016/j.isci.2022.103798
226. Kokkorakis M, Folkertsma P, van Dam S, Sirotin N, Taheri S, Chagoury O, et al. Effective questionnaire-based prediction models for type 2 diabetes across several ethnicities: a model development and validation study. eClinicalMedicine. (2023) 64:102235. doi: 10.1016/j.eclinm.2023.102235
227. Ge T, Irvin MR, Patki A, Srinivasasainagendra V, Lin Y-F, Tiwari HK, et al. Development and validation of a trans-ancestry polygenic risk score for type 2 diabetes in diverse populations. Genome Med. (2022) 14:70. doi: 10.1186/s13073-022-01074-2
228. Mahajan A, Spracklen CN, Zhang W, Ng MC, Petty LE, Kitajima H, et al. Multi-ancestry genetic study of type 2 diabetes highlights the power of diverse populations for discovery and translation. Nat Genet. (2022) 54:560–72. doi: 10.1038/s41588-022-01058-3
229. Chato L, Regentova E. Survey of transfer learning approaches in the machine learning of digital health sensing data. J Pers Med. (2023) 13:1703. doi: 10.3390/jpm13121703
230. Deng Y, Lu L, Aponte L, Angelidi AM, Novak V, Karniadakis GE, et al. Deep transfer learning and data augmentation improve glucose levels prediction in type 2 diabetes patients. NPJ Digit Med. (2021) 4:109. doi: 10.1038/s41746-021-00480-x
231. Khoury M, et al. Artificial intelligence and public health: the challenges and opportunities ahead. Am J Public Health. (2021) 111:972–80. doi: 10.1016/B978-0-443-22270-2.00023-X
232. Ghassemi M, Naumann T, Schulam P, Beam AL, Chen IY, Ranganath R. A review of challenges and opportunities in machine learning for health. Nat Med. (2021) 27:34–8.33442008
233. Molyneux S, Sukhtankar P, Thitiri J, Njeru R, Muraya K, Sanga G, et al. Ethics of AI in healthcare: a policy framework for responsible innovation. BMJ Glob Health. (2021) 6:e004937. doi: 10.1136/bmjgh-2021-004937
234. Wen J, Zhang Z, Lan Y, Cui Z, Cai J, Zhang W. A survey on federated learning: challenges and applications. Int J Mach Learn Cybern. (2023) 14:513–35. doi: 10.1007/s13042-022-01647-y
235. Anjum N, Alibakhshikenari M, Rashid J, Jabeen F, Asif A, Mohamed EM, et al. Iot-based covid-19 diagnosing and monitoring systems: a survey. IEEE Access. (2022) 10:87168–81. doi: 10.1109/ACCESS.2022.3197164
236. Chenthara S, Ahmed K, Wang H, Whittaker F. Security and privacy-preserving challenges of e-health solutions in cloud computing. IEEE Access. (2019) 7:74361–82. doi: 10.1109/ACCESS.2019.2919982
237. Hu F, Qiu S, Yang X, Wu C, Nunes MB, Chen H. Privacy-preserving healthcare and medical data collaboration service system based on blockchain and federated learning. Comput Mater Continua. (2024) 80:2897–915. doi: 10.32604/cmc.2024.052570
238. Tripathi G, Ahad MA, Casalino G. A comprehensive review of blockchain technology: underlying principles and historical background with future challenges. Decis Anal J. (2023) 9:100344. doi: 10.1016/j.dajour.2023.100344
239. Baowaly MK, Lin C-C, Liu C-L, Chen K-T. Synthesizing electronic health records using improved generative adversarial networks. J Am Med Inform Assoc. (2019) 26:228–41. doi: 10.1093/jamia/ocy142
240. Singhal K, Azizi S, Tu T, Mahdavi SS, Wei J, Chung HW, et al. Large language models encode clinical knowledge. Nature. (2023) 620:172–80. doi: 10.1038/s41586-023-06291-2
241. Ding J-E, Thao PNM, Peng W-C, Wang J-Z, Chug C-C, Hsieh M-C, et al. Large language multimodal models for new-onset type 2 diabetes prediction using five-year cohort electronic health records. Sci Rep. (2024) 14:20774. doi: 10.1038/s41598-024-71020-2
242. Geukes Foppen RJ, Gioia V, Gupta S, Johnson CL, Giantsidis J, Papademetris M. Data from: Methodology for safe and secure AI in diabetes management (2024).
243. Singhal A, Neveditsin N, Tanveer H, Mago V. Toward fairness, accountability, transparency, and ethics in ai for social media and health care: scoping review. JMIR Med Inform. (2024) 12:e50048. doi: 10.2196/50048
244. Zheng S, Li Y, Chen S, Xu J, Yang Y. Assessing reliability and challenges of uncertainty quantification in ai-driven medical imaging. Nat Mach Intell. (2020) 2:551–60. doi: 10.1038/s42256-020-0224-z
245. Gal Y, Ghahramani Z. Dropout as a bayesian approximation: representing model uncertainty in deep learning. In: Proceedings of ICML 2016. (2016). p. 1050–9.
246. Shafer G, Vovk V. A tutorial on conformal prediction. J Mach Learn Res. (2008) 9:371–421. doi: 10.5555/1390681.1390693
247. Platt J. Probabilistic outputs for support vector machines and comparisons to regularized likelihood methods. In: Advances in Large Margin Classifiers. (1999). Vol. 10. p. 61–74.
248. Zadrozny B, Elkan C. Transforming classifier scores into accurate multiclass probability estimates. In: Proceedings of the Eighth ACM SIGKDD International Conference on Knowledge Discovery and Data Mining. (2002). p. 694–9.
249. Guo C, Pleiss G, Sun Y, Weinberger KQ. On calibration of modern neural networks. In: Proceedings of ICML 2017. (2017). p. 1321–30.
250. Jiang H, Kim B, Guan M, Gupta M. To trust or not to trust a classifier. Adv Neural Inf Process Syst. (2018) 31:5541–52. doi: 10.48550/arXiv.1805.11783
After establishing a foundational set of keywords, further refinement was achieved by applying the TF-IDF algorithm to the preliminary keyword screening dataset. TF-IDF assigns weights to terms based on how frequently they appear in a document and how rare they are across the entire corpus. It is calculated by dividing the number of times a term () appears in a document by the total number of terms in the document ().
Mathematically, and is calculated as follows:
As shown in Equation (A1) Term Frequency (TF) measures the occurrence of a term within a document, whereas Inverse Document Frequency (IDF) adjusts for how common or rare the term is across the entire dataset [Equation (A2)].
Where, is the term, is the corpus of documents and is the total number of documents in the corpus. Finally, the TF-IDF score for a term in a document is calculated by multiplying its TF by its Inverse IDF, as defined in Equation (A3).
The idea is that if a term appears multiple times in a document, it is likely to be more important. Whereas, IDF measures how unique or rare a term is across all documents in the corpus. Terms that occur frequently in many documents are considered less important compared to those that occur in only a few documents. IDF is calculated by taking the logarithm of the ratio of the total number of documents to the number of documents containing the term and adding 1 to avoid division by zero errors. In Table A1, the top thirty terms are presented, ranked by their TF-IDF scores, which highlight those with the greatest impact on specialized discussions within the field.
Keywords: type 2 diabetes mellitus (T2DM), machine learning (ML), artificial intelligence (AI), bibliometric analysis, predictive models
Citation: Kiran M, Xie Y, Anjum N, Ball G, Pierscionek B and Russell D (2025) Machine learning and artificial intelligence in type 2 diabetes prediction: a comprehensive 33-year bibliometric and literature analysis. Front. Digit. Health 7:1557467. doi: 10.3389/fdgth.2025.1557467
Received: 8 January 2025; Accepted: 10 March 2025;
Published: 27 March 2025.
Edited by:
Adnan Haider, Dongguk University Seoul, Republic of KoreaReviewed by:
Simão Paredes, Polytechnical Institute of Coimbra, PortugalCopyright: © 2025 Kiran, Xie, Anjum, Ball, Pierscionek and Russell. This is an open-access article distributed under the terms of the Creative Commons Attribution License (CC BY). The use, distribution or reproduction in other forums is permitted, provided the original author(s) and the copyright owner(s) are credited and that the original publication in this journal is cited, in accordance with accepted academic practice. No use, distribution or reproduction is permitted which does not comply with these terms.
*Correspondence: Mahreen Kiran, bWVocmVlbi5raXJhbjg5QGdtYWlsLmNvbQ==
Disclaimer: All claims expressed in this article are solely those of the authors and do not necessarily represent those of their affiliated organizations, or those of the publisher, the editors and the reviewers. Any product that may be evaluated in this article or claim that may be made by its manufacturer is not guaranteed or endorsed by the publisher.
Research integrity at Frontiers
Learn more about the work of our research integrity team to safeguard the quality of each article we publish.