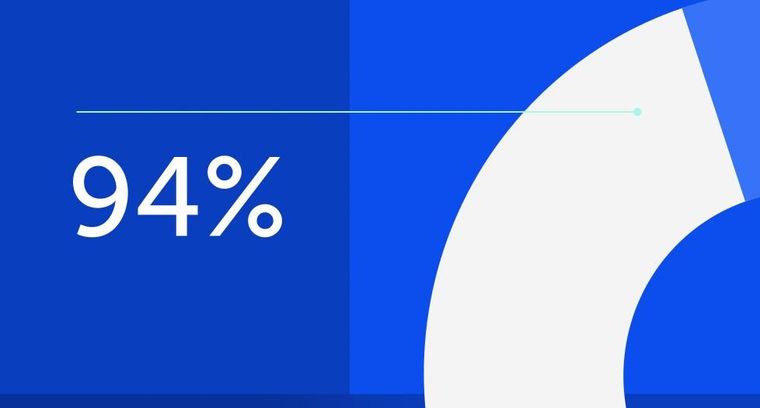
94% of researchers rate our articles as excellent or good
Learn more about the work of our research integrity team to safeguard the quality of each article we publish.
Find out more
ORIGINAL RESEARCH article
Front. Digit. Health
Sec. Health Informatics
Volume 7 - 2025 | doi: 10.3389/fdgth.2025.1544797
This article is part of the Research TopicExpanding Insights Into Structure, Function, and Disorder of Genome by the Power of Artificial Intelligence in BioinformaticsView all 3 articles
The final, formatted version of the article will be published soon.
Select one of your emails
You have multiple emails registered with Frontiers:
Notify me on publication
Please enter your email address:
If you already have an account, please login
You don't have a Frontiers account ? You can register here
Ophthalmology consultations play a vital role in the diagnosis, treatment, and prevention of eye diseases. Nevertheless, the surging demand for consultations has outstripped the supply of ophthalmologists. In the era of digital health, electronic diagnosis and online treatment have emerged as potential solutions. By harnessing large pre-trained language models, we can design effective dialogues for specific ophthalmology scenarios, thereby facilitating consultations. However, conventional fine-tuning strategies for question-answering tasks have proven impractical due to the escalating model size, the frequent oversight of the patient-doctor role function during consultations, and the lack of exploration into the underlying mechanisms of ophthalmic diseases. In this paper, we propose EyeDoctor, an ophthalmic medical questioning large language model which augments its accuracy via doctor-patient role perception guidance and an enhanced knowledge base incorporating external disease information and electronic medical records using Retrieval-Augmented Generation (RAG). Specifically, the knowledge base incorporates curated genetic and disease-related information from PubMed to provide a deeper understanding of ophthalmic disease mechanisms and improve responses to medical queries. Experimental results show EyeDoctor achieves higher questionanswering precision in ophthalmology consultations. Notably, EyeDoctor demonstrated a 7.25% improvement in Rouge-1 scores and a 10.16% improvement in F1 scores on multi-round datasets compared to second best model ChatGPT, highlighting the importance of doctor-patient role differentiation, external knowledge integration, and a deeper focus on disease mechanism exploration. Moreover, EyeDoctor demonstrates promising clinical utility by enhancing the relevance and accuracy of ophthalmic consultation responses. It assists physicians in preliminary triage and symptom interpretation, helps reduce consultation time, and improves consistency in diagnostic support. These capabilities contribute to better alignment with real-world clinical practices and improved patient experience, particularly in resource-constrained settings. EyeDoc also serves as a free available web based service and source code is available at https://github.com/sperfu/EyeDoc.
Keywords: Ophthalmology Consultations, Large Language Model, Natural Language Processing, Role-specific Encoding, Doctor-Patient Style Differentiation
Received: 19 Dec 2024; Accepted: 10 Apr 2025.
Copyright: © 2025 Fu, Fan, Du, Feng, Li and Song. This is an open-access article distributed under the terms of the Creative Commons Attribution License (CC BY). The use, distribution or reproduction in other forums is permitted, provided the original author(s) or licensor are credited and that the original publication in this journal is cited, in accordance with accepted academic practice. No use, distribution or reproduction is permitted which does not comply with these terms.
* Correspondence:
Laiyi Fu, Xi'an Jiaotong University, Xi'an, China
Huping Song, Xi’an Fourth Hospital, xi'an, China
Disclaimer: All claims expressed in this article are solely those of the authors and do not necessarily represent those of their affiliated organizations, or those of the publisher, the editors and the reviewers. Any product that may be evaluated in this article or claim that may be made by its manufacturer is not guaranteed or endorsed by the publisher.
Supplementary Material
Research integrity at Frontiers
Learn more about the work of our research integrity team to safeguard the quality of each article we publish.