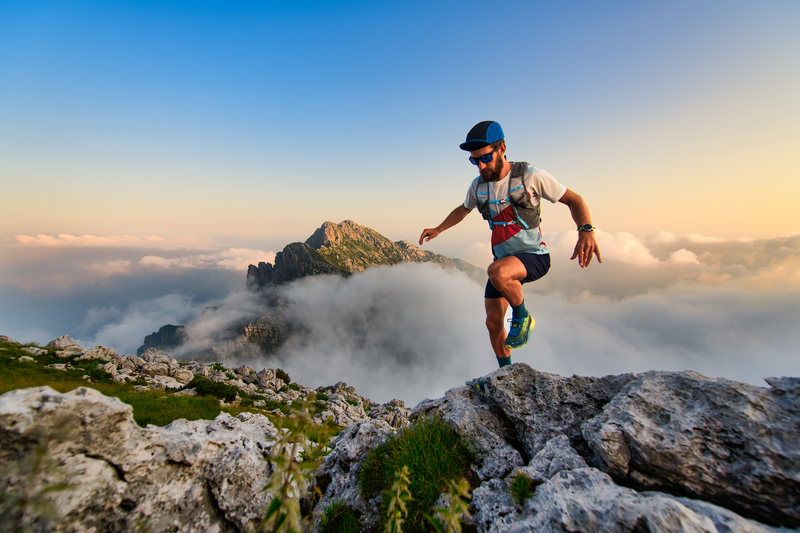
94% of researchers rate our articles as excellent or good
Learn more about the work of our research integrity team to safeguard the quality of each article we publish.
Find out more
PERSPECTIVE article
Front. Digit. Health
Sec. Health Communications and Behavior Change
Volume 7 - 2025 | doi: 10.3389/fdgth.2025.1544185
This article is part of the Research Topic AI for Health Behavior Change View all articles
The final, formatted version of the article will be published soon.
You have multiple emails registered with Frontiers:
Please enter your email address:
If you already have an account, please login
You don't have a Frontiers account ? You can register here
As intelligent systems become more integrated into people's daily life, systems designed to facilitate lifestyle and behavior change for health and well-being have also become more common. Previous work has identified challenges in the development and deployment of such AI-based support for diabetes lifestyle management and shown that it is necessary to shift the design process of AI-based support systems towards a human-centered approach that can be addressed by hybrid intelligence (HI). However, this shift also means adopting a user-centric design process, which brings its own challenges in terms of stakeholder involvement, evaluation processes and ethical concerns. In this perspective paper, we aim to more comprehensively identify challenges and future research directions in the development of HI systems for behavior change from four different viewpoints: 1) challenges on an individual level, such as understanding the individual enduser's context 2) challenges on an evaluation level, such as evaluation pipelines and identifying success criteria and 3) challenges in addressing ethical implications. We show that developing HI systems for behavior change is an interdisciplinary process that requires further collaboration and consideration from various fields.
Keywords: human computer interaction, Hybrid intelligence, behavior change, healthcare, Support system
Received: 12 Dec 2024; Accepted: 05 Mar 2025.
Copyright: © 2025 Hao, Uusitalo, Figueroa, Smit, Strange, Chang, Ribeiro, Nana, Tielman and de Boer. This is an open-access article distributed under the terms of the Creative Commons Attribution License (CC BY). The use, distribution or reproduction in other forums is permitted, provided the original author(s) or licensor are credited and that the original publication in this journal is cited, in accordance with accepted academic practice. No use, distribution or reproduction is permitted which does not comply with these terms.
* Correspondence:
Chenxu Hao, Delft University of Technology, Delft, Netherlands
Disclaimer: All claims expressed in this article are solely those of the authors and do not necessarily represent those of their affiliated organizations, or those of the publisher, the editors and the reviewers. Any product that may be evaluated in this article or claim that may be made by its manufacturer is not guaranteed or endorsed by the publisher.
Research integrity at Frontiers
Learn more about the work of our research integrity team to safeguard the quality of each article we publish.