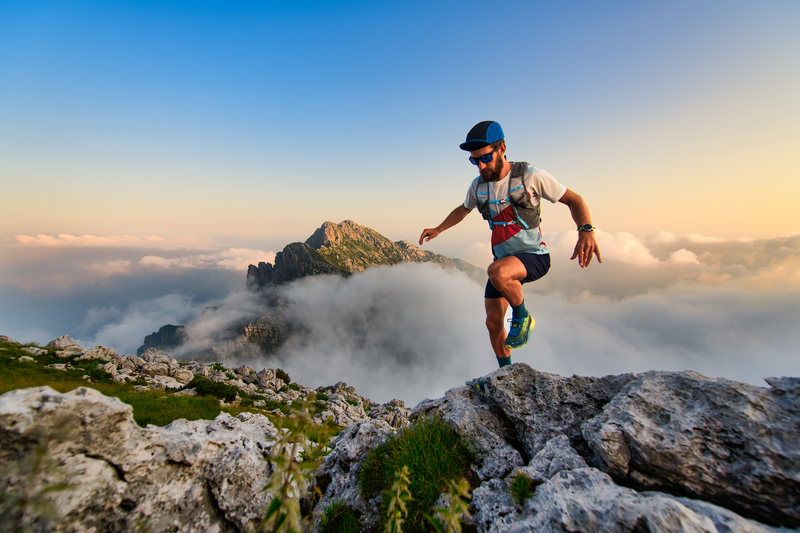
94% of researchers rate our articles as excellent or good
Learn more about the work of our research integrity team to safeguard the quality of each article we publish.
Find out more
ORIGINAL RESEARCH article
Front. Digit. Health
Sec. Health Technology Implementation
Volume 7 - 2025 | doi: 10.3389/fdgth.2025.1535335
This article is part of the Research Topic Advances in Artificial Intelligence-Enhanced Electrocardiography: A Pathway towards Improved Diagnosis and Patient Care. View all 6 articles
The final, formatted version of the article will be published soon.
You have multiple emails registered with Frontiers:
Please enter your email address:
If you already have an account, please login
You don't have a Frontiers account ? You can register here
The electrocardiogram (ECG) is vital for diagnosing heart diseases, offering insights into cardiac health through electrical activity patterns. Portable monitoring solutions have emerged to meet the growing demand for timely evaluations. This paper introduces a portable ECG monitoring system featuring classification capabilities using Convolutional Neural Networks (CNN). An Arduino Nano microcontroller and an AD8232 ECG sensor are used for cost-effective, continuous monitoring. The hardware involves reliable ECG signal acquisition by interfacing the AD8232 sensor with the Arduino Nano. CNNs enhance real time ECG data classification, enabling identification of cardiac abnormalities, including arrhythmias. Extensive training on MIT-BIH datasets illustrates the CNN model's accuracy. It achieves binary and multi-class classification accuracies of 98.35% and 99.3%, respectively, surpassing existing benchmarks. The system's strong performance highlights its potential impact on clinical practice, providing a cost-effective and reliable solution for continuous ECG monitoring. The proposed portable ECG monitoring system with CNN-based classification shows great promise for early arrhythmia diagnosis and continuous cardiac monitoring. Its accurate classification skills, low-cost hardware, and real-time monitoring features make it an effective tool for improving cardiovascular health management.
Keywords: ECG, Portable monitoring, Convolutional Neural Networks, Arrhythmia detection, Arduino nano, AD8232 ECG sensor
Received: 27 Nov 2024; Accepted: 21 Feb 2025.
Copyright: © 2025 Narendra, Panwar, Arya, Raj and Kumar. This is an open-access article distributed under the terms of the Creative Commons Attribution License (CC BY). The use, distribution or reproduction in other forums is permitted, provided the original author(s) or licensor are credited and that the original publication in this journal is cited, in accordance with accepted academic practice. No use, distribution or reproduction is permitted which does not comply with these terms.
* Correspondence:
Modigari Narendra, School of Computer Science and Engineering, Vellore Institute of Technology (VIT), Chennai, India
Disclaimer: All claims expressed in this article are solely those of the authors and do not necessarily represent those of their affiliated organizations, or those of the publisher, the editors and the reviewers. Any product that may be evaluated in this article or claim that may be made by its manufacturer is not guaranteed or endorsed by the publisher.
Research integrity at Frontiers
Learn more about the work of our research integrity team to safeguard the quality of each article we publish.