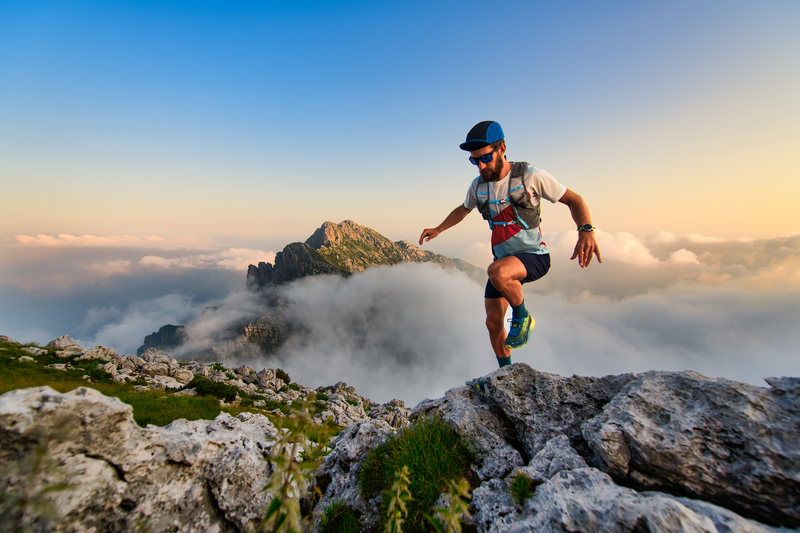
94% of researchers rate our articles as excellent or good
Learn more about the work of our research integrity team to safeguard the quality of each article we publish.
Find out more
ORIGINAL RESEARCH article
Front. Digit. Health
Sec. Health Informatics
Volume 7 - 2025 | doi: 10.3389/fdgth.2025.1528882
The final, formatted version of the article will be published soon.
You have multiple emails registered with Frontiers:
Please enter your email address:
If you already have an account, please login
You don't have a Frontiers account ? You can register here
Identifying and understanding the co-occurrence of multiple long-term conditions (MLTCs) in individuals with intellectual disability (ID) is crucial for effective healthcare management.Individuals with ID often experience earlier onset and higher prevalence of MLTCs compared to the general population, however, the specific patterns of co-occurrence and temporal progression of these conditions remain largely unexplored. This study presents an innovative unsupervised approach for examining and characterising clusters of MLTC in individuals with ID, based on their shared disease trajectories. Using a dataset of electronic health records (EHRs) from 13069 individuals with ID, encompassing primary and secondary care data in Wales from 2000 to 2021, this study analysed the time sequences of disease diagnoses. Significant pairwise disease associations were identified, and their temporal directionality assessed. Subsequently, an unsupervised clustering algorithm - spectral clustering - was applied to the shared disease trajectories, grouping them based on common temporal patterns. The study population comprised 52.3% males and 47.7% females, with a mean of 4.5 ± 3 long-term conditions (LTCs) per patient. Distinct MLTC clusters were identified in both males and females, stratified by age groups (< 45 and ≥ 45 years). For males under 45, a single cluster dominated by neurological conditions (32.4%), while three clusters were identified for older males, with the largest characterised by circulatory (51.8%). In females under 45, one cluster was found with digestive system conditions (24.6%) being most prevalent. For females ≥ 45 years, two clusters were identified: the first cluster was predominantly defined by circulatory (34.1%), while the second cluster by digestive (25.9%) and musculoskeletal (21.9%) system conditions. Mental illness, epilepsy, and reflux disorders were prevalent across all groups. This study reveals complex multimorbidity patterns in individuals with ID, highlighting age and sex differences. The identified clusters provide new insights into disease progression and co-occurrence in this population. These findings can inform the development of targeted interventions and risk stratification strategies, potentially improving personalised healthcare for individuals with ID and MLTCs with the aim of improving health outcome for this vulnerable group of patients i.e. reducing frequency and length of hospital admissions and premature mortality.
Keywords: Disease trajectories, Chronic Disease, co-morbidity, clustering, Intellectual Disability
Received: 15 Nov 2024; Accepted: 12 Mar 2025.
Copyright: © 2025 Kousovista, Cosma, Abakasanga, Akbari, Zaccardi, Jun, Kiani and Gangadharan. This is an open-access article distributed under the terms of the Creative Commons Attribution License (CC BY). The use, distribution or reproduction in other forums is permitted, provided the original author(s) or licensor are credited and that the original publication in this journal is cited, in accordance with accepted academic practice. No use, distribution or reproduction is permitted which does not comply with these terms.
* Correspondence:
Georgina Cosma, Department of Computer Science, School of Science, Loughborough University, Loughborough, Leicestershire, United Kingdom
Disclaimer: All claims expressed in this article are solely those of the authors and do not necessarily represent those of their affiliated organizations, or those of the publisher, the editors and the reviewers. Any product that may be evaluated in this article or claim that may be made by its manufacturer is not guaranteed or endorsed by the publisher.
Research integrity at Frontiers
Learn more about the work of our research integrity team to safeguard the quality of each article we publish.