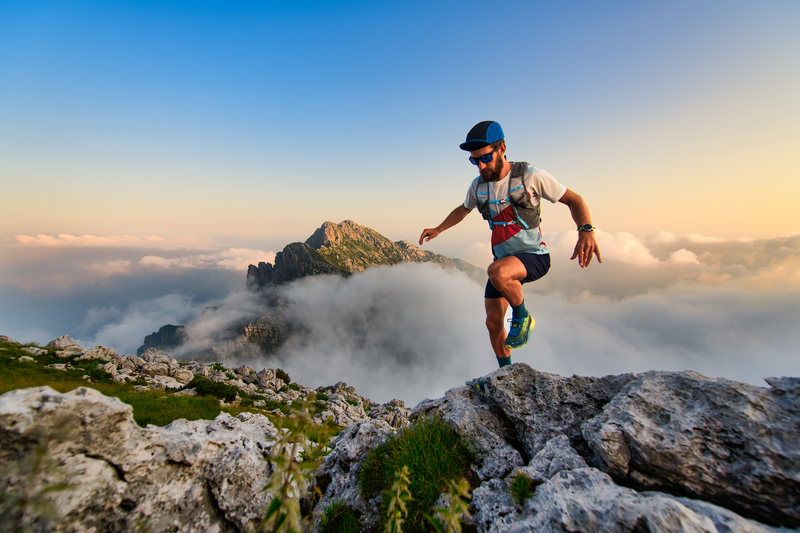
95% of researchers rate our articles as excellent or good
Learn more about the work of our research integrity team to safeguard the quality of each article we publish.
Find out more
ORIGINAL RESEARCH article
Front. Digit. Health
Sec. Health Informatics
Volume 7 - 2025 | doi: 10.3389/fdgth.2025.1523953
This article is part of the Research Topic Healthcare Text Analytics: Unlocking the Evidence from Free Text, Volume V View all articles
The final, formatted version of the article will be published soon.
You have multiple emails registered with Frontiers:
Please enter your email address:
If you already have an account, please login
You don't have a Frontiers account ? You can register here
A readily available outcome measure that reflects the success of a patient's treatment is needed to demonstrate the value of orthopaedic interventions. Patient-reported outcome measures (PROMs) are survey-based instruments that collect joint-specific and general health perceptions on symptoms, functioning, and health-related quality of life. PROMs are considered the gold standard outcome measure in orthopaedic medicine, but their use is limited in real-world practice due to challenges with technology integration, the pace of clinic workflows, and patient compliance. Clinical notes generated during each encounter patients have with their physician contain rich information on current disease symptoms, rehabilitation progress, and unexpected complications. Artificial intelligence (AI) methods can be used to identify phrases of treatment success or failure captured in clinical notes and discern an indicator of treatment success for orthopaedic patients. This was a cross-sectional analysis of clinical notes from a sample of patients with an acute shoulder injury. The study included adult patients presenting to a Level-1 Trauma Center and regional health system for an acute Proximal Humerus Fracture (PHF) between January 1, 2019 and December 31, 2021. We used the progress note from the office visit for PHF-related care (ICD10: S42.2XXX) or shoulder pain (ICD10: M45.2XXX) closest to 1-year after the injury date.Clinical notes were reviewed by an orthopaedic resident and labeled as treatment success or failure. A structured comparative analysis of classifiers including both machine and deep learning algorithms was performed. The final sample included 868 clinical notes from patients treated by 123 physicians across 35 departments within one regional health system. The study sample was stratified into 465 notes labeled as treatment success and 403 labeled as treatment failure. The Bio-ClinicalBERT model had the highest performance of 87% accuracy (AUC = 0.87 +/-0.04) in correctly distinguishing between treatment success and failure notes. Our results suggest that text classifiers applied to clinical notes are capable of differentiating patients with successful treatment outcomes with high levels of accuracy. This finding is encouraging, signaling that routinely collected clinical note content may serve as a data source to develop an outcome measure for orthopaedic patients.
Keywords: Proximal humerus fractures, artificial intelligence, Treatment outcomes, Clinical text, Natural Language Processing, Electronic health record system
Received: 04 Dec 2024; Accepted: 26 Mar 2025.
Copyright: © 2025 Floyd, Alameldin, Smith, Judkins, Krohn, Reynolds, Jeray and Obeid. This is an open-access article distributed under the terms of the Creative Commons Attribution License (CC BY). The use, distribution or reproduction in other forums is permitted, provided the original author(s) or licensor are credited and that the original publication in this journal is cited, in accordance with accepted academic practice. No use, distribution or reproduction is permitted which does not comply with these terms.
* Correspondence:
Sarah B. Floyd, Clemson University, Department of Public Health Sciences, Clemson, United States
Disclaimer: All claims expressed in this article are solely those of the authors and do not necessarily represent those of their affiliated organizations, or those of the publisher, the editors and the reviewers. Any product that may be evaluated in this article or claim that may be made by its manufacturer is not guaranteed or endorsed by the publisher.
Research integrity at Frontiers
Learn more about the work of our research integrity team to safeguard the quality of each article we publish.