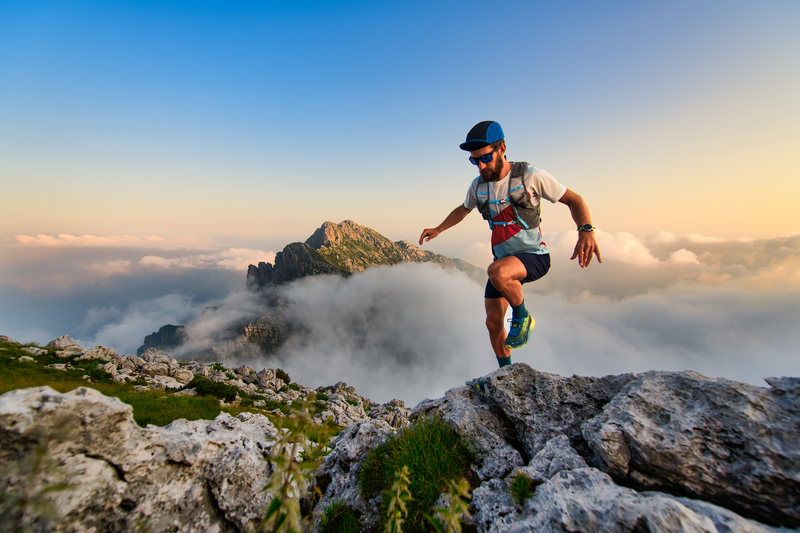
95% of researchers rate our articles as excellent or good
Learn more about the work of our research integrity team to safeguard the quality of each article we publish.
Find out more
MINI REVIEW article
Front. Digit. Health
Sec. Health Informatics
Volume 7 - 2025 | doi: 10.3389/fdgth.2025.1510166
This article is part of the Research Topic Revolutionizing sleep instability: advanced diagnosis and management of sleep disorders View all articles
The final, formatted version of the article will be published soon.
You have multiple emails registered with Frontiers:
Please enter your email address:
If you already have an account, please login
You don't have a Frontiers account ? You can register here
Sleep apnea is one of the most common sleep disorders, which, if left untreated, may have severe health consequences in the long term. Many sleep apnea patients remain non-diagnosed due to lacking access to medical tests. In recent years, portable and wearable sensors that measure blood oxygen saturation (SpO 2 ) are becoming common and affordable for daily use, and they open the door for affordable and accessible sleep apnea screening in the context of everyday life. To learn about the advancement in SpO 2 -based sleep apnea screening, we conducted a survey of published studies. We searched databases including Springer, Science Direct, Web of Science, ACM Digital Library, and IEEE Xplore using the keywords "sleep apnea" AND ("SpO2" OR "blood oxygen saturation") AND ("machine learning" OR "deep learning"). After screening 835 results, we included 31 publications for a full-text review. Analysis shows that SpO 2 -based sleep apnea screening studies consist of three main categories: 1) individual apnea events detection, 2) apnea-hypopnea index prediction, and 3) apnea severity classification. We found two significant research gaps: a lack of sufficient and diverse publicly available datasets, and the absence of standardized protocols for data collection, signal preprocessing, and model bench marking. Future research should focus on addressing these gaps to enhance the effectiveness and reliability of AI-driven sleep apnea screening methods using SpO 2 signals.
Keywords: Sleep Apnea, SpO2, Oximeter, Mobile health (mHealth), Digital Health, machine learning, deep learning, apnea-hypopnea index
Received: 12 Oct 2024; Accepted: 24 Mar 2025.
Copyright: © 2025 Hoang and Liang. This is an open-access article distributed under the terms of the Creative Commons Attribution License (CC BY). The use, distribution or reproduction in other forums is permitted, provided the original author(s) or licensor are credited and that the original publication in this journal is cited, in accordance with accepted academic practice. No use, distribution or reproduction is permitted which does not comply with these terms.
* Correspondence:
Nhung Huyen Hoang, Kyoto University of Advanced Science (KUAS), Kyoto, Japan
Disclaimer: All claims expressed in this article are solely those of the authors and do not necessarily represent those of their affiliated organizations, or those of the publisher, the editors and the reviewers. Any product that may be evaluated in this article or claim that may be made by its manufacturer is not guaranteed or endorsed by the publisher.
Research integrity at Frontiers
Learn more about the work of our research integrity team to safeguard the quality of each article we publish.