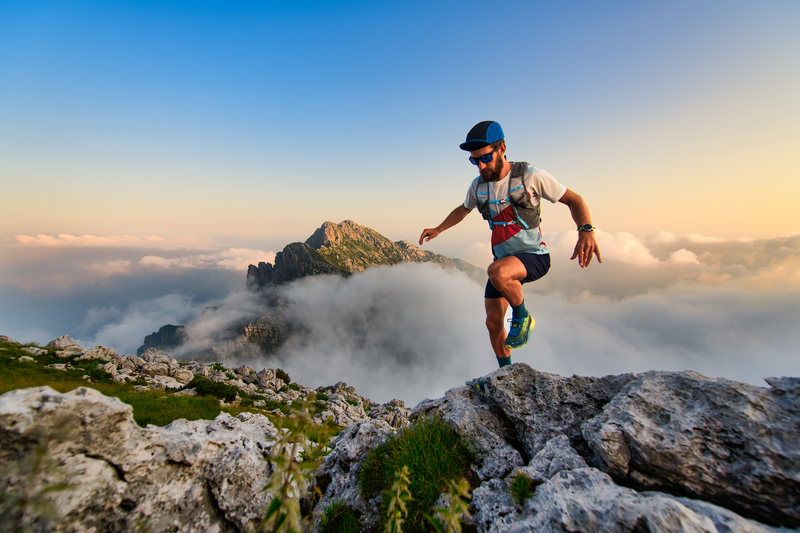
95% of researchers rate our articles as excellent or good
Learn more about the work of our research integrity team to safeguard the quality of each article we publish.
Find out more
ORIGINAL RESEARCH article
Front. Digit. Health
Sec. Health Informatics
Volume 7 - 2025 | doi: 10.3389/fdgth.2025.1497130
This article is part of the Research Topic Large Language Models for Medical Applications View all 12 articles
The final, formatted version of the article will be published soon.
You have multiple emails registered with Frontiers:
Please enter your email address:
If you already have an account, please login
You don't have a Frontiers account ? You can register here
Since clinical letters contain sensitive information, clinical-related datasets cannot be widely applied in model training, medical research, and education. This work aims to generate reliable, diverse, and de-identified synthetic clinical letters. We explored various pre-trained language models (PLMs) for text masking and generation. After that, we worked on Bio ClinicalBERT, a high-performing model, and experimented with different masking strategies. Both qualitative and quantitative methods were used for evaluation. A downstream task, Named Entity Recognition (NER), was further implemented to assess the usability of these synthetic letters. We also applied clinically focused evaluation methods -including BioGPT and GPT-3.5-turbo -to evaluate the clinical performance of the synthetic texts.The results indicate that 1) encoder-only models outperform encoder-decoder models. 2) Among encoder-only models, those trained on general corpora perform comparably to those trained on clinical data when clinical information is preserved. 3) Preserving clinical entities and document structure aligns more closely with our objectives than simply fine-tuning the model. 4) Masking strategies have a noticeable impact on the quality of synthetic clinical letters: masking stopwords has a positive impact, while masking nouns or verbs has a negative effect. 5) BERTScore should be the primary quantitative evaluation metric, with other metrics serving as supplementary references. 6) Contextual information has only a limited effect on the models' understanding, suggesting that synthetic letters can effectively substitute real ones in downstream NER tasks. 7) Although the model occasionally generates hallucinated content, it appears to have little effect on overall clinical performance.Unlike previous research, which primarily focuses on reconstructing the original letters by training language models, this paper provides a foundational framework for generating diverse, de-identified clinical letters. It offers a direction for utilising the model to process real-world clinical letters, thereby helping to expand datasets in the clinical domain. Our codes and trained models are available at https://github.com/HECTA-UoM/Synthetic4Health
Keywords: Pre-trained Language Models (PLMs), Encoder-Only Models, Encoder-decoder models, Named entity recognation, Masking and Generating, Synthetic data creation, Clinical NLP (Natural Language Processing)
Received: 16 Sep 2024; Accepted: 31 Mar 2025.
Copyright: © 2025 Ren, Belkadi, Han, Del-Pinto and Nenadic. This is an open-access article distributed under the terms of the Creative Commons Attribution License (CC BY). The use, distribution or reproduction in other forums is permitted, provided the original author(s) or licensor are credited and that the original publication in this journal is cited, in accordance with accepted academic practice. No use, distribution or reproduction is permitted which does not comply with these terms.
* Correspondence:
Lifeng Han, The University of Manchester, Manchester, United Kingdom
Disclaimer: All claims expressed in this article are solely those of the authors and do not necessarily represent those of their affiliated organizations, or those of the publisher, the editors and the reviewers. Any product that may be evaluated in this article or claim that may be made by its manufacturer is not guaranteed or endorsed by the publisher.
Research integrity at Frontiers
Learn more about the work of our research integrity team to safeguard the quality of each article we publish.