- 1Department of Health Informatics, College of Medicine and Health Science, Samara University, Samara, Ethiopia
- 2Department of Epidemiology and Biostatistics, School of Public Health College of Medicine and Health Science, Haramaya University, Harar, Ethiopia
- 3Department of Nursing, College of Medicine and Health Sciences, Samara University, Samara, Ethiopia
- 4Department of Public Health, College of Medicine and Health Science, University of Gondar, Gondar, Ethiopia
- 5Department of Demography & Social Statistics, Federal University, Birnin-Kebbi, Kebbi State, Nigeria
Background: Fertility preferences refer to the number of children an individual would like to have, regardless of any obstacles that may stand in the way of fulfilling their aspirations. Despite the creation and application of numerous interventions, the overall fertility rate in West African nations, particularly Nigeria, is still high at 5.3% according to 2018 Nigeria Demographic and Health Survey data. Hence, this study aimed to predict the fertility preferences of reproductive age women in Nigeria using state-of-the-art machine learning techniques.
Methods: Secondary data analysis from the recent 2018 Nigeria Demographic and Health Survey dataset was employed using feature selection to identify predictors to build machine learning models. Data was thoroughly assessed for missingness and weighted to draw valid inferences. Six machine learning algorithms, namely, Logistic Regression, Support Vector Machine, K-Nearest Neighbors, Decision Tree, Random Forest, and eXtreme Gradient Boosting, were employed on a total sample size of 37,581 in Python 3.9 version. Model performance was assessed using accuracy, precision, recall, F1-score, and area under the receiver operating characteristic curve (AUROC). Permutation and Gini techniques were used to identify the feature's importance.
Results: Random Forest achieved the highest performance with an accuracy of 92%, precision of 94%, recall of 91%, F1-score of 92%, and AUROC of 92%. Factors influencing fertility preferences were number of children, age group, and ideal family size. Region, contraception intention, ethnicity, and spousal occupation had a moderate influence. The woman's occupation, education, and marital status had a lower impact.
Conclusion: This study highlights the potential of machine learning for analyzing complex demographic data, revealing hidden factors associated with fertility preferences among Nigerian women. In conclusion, these findings can inform more effective family planning interventions, promoting sustainable development across Nigeria.
Background
Fertility preferences, or desire, refer to the number of children that an individual would like to have, regardless of any obstacles that may stand in the way of fulfilling their aspirations (1). These preferences are dynamic rather than constant, often changing due to life circumstances, policy interventions, or broader societal trends (2). Studies on the stability of fertility preferences have critiqued the measurement of fertility preferences, demonstrating that fertility preferences do not remain constant throughout life (3). Over the past 50 years, one of the most significant developments in the demography of low- and middle-income countries (LMICs) has been the rising prevalence of modern contraception (4). Changes in the use of contraceptives have been intensely debated in both scientific and policy settings because they directly address the policy question of expanding family planning services (5). During the COVID-19 pandemic, the fertility preferences of women started to change, leading to a decrease in national fertility rates (6). This shift has been attributed to the financial and emotional burdens of parenting, the influence of their partners' desires, and the stability of their relationships (7).
In sub-Saharan Africa (SSA), most nations have a fertility rate of more than five children per woman (8). While the total number of births per woman in SSA in 2022 was 4.5, estimates for the Arab world, South Asia, Latin America and the Caribbean, and the European Union were 3.1, 2.2, 1.8, and 1.5, respectively (9). High fertility rates contribute to increased maternal and child health burdens, strain public health infrastructure, and slow progress toward achieving global development goals, such as those outlined in the Sustainable Development Goals (SDGs) (10–12).
Despite the development and implementation of multiple treatments, the general fertility rate in West African countries remains high. Efforts to reduce fertility rates in Nigeria include the National Population Policy, which advocates voluntary fertility regulation to achieve economic and social development goals (13). As a result, according to the Nigeria Demographic and Health Survey (NDHS), the fertility rate declined from 6.0 in 1990 to 5.3 in 2018 (14). Regardless of such initiatives, many factors, including limited access to contraception, low levels of female education, and deeply ingrained cultural norms, continue to sustain high fertility preferences. Understanding these preferences and the factors that influence them is critical for designing effective interventions. Previous research has predominantly relied on traditional statistical methods, such as logistic regression, to analyze predictors of fertility preferences (2, 13, 15, 16). While these methods are valuable for hypothesis-driven analyses, they are often constrained by their inability to model complex, non-linear relationships between predictors and outcomes. For example, demographic and health variables such as age, marital status, education, and contraceptive use may interact in ways that are not easily captured by linear models (10, 17, 18). In addition, traditional methods often require strong assumptions about the data distribution, which may not hold in real-world demographic datasets (19, 20). Machine learning (ML) has emerged as a transformative tool in demographic research, offering advanced techniques to identify hidden patterns within large datasets (21, 22). This study addresses this gap by employing ML algorithms to predict fertility preferences using nationally representative data from the 2018 NDHS. Furthermore, this study contributes to the growing body of literature on ML in demographic research by providing a novel framework for predicting fertility preferences.
Methods
Study setting and design
The NDHS employed a population-based cross-sectional study design to collect the data in two stages using a stratified sampling technique to select the study participants. This study used a predictive modeling approach to predict the fertility preference of reproductive age women based on data obtained from the NDHS.
Study population
All women aged 15–49 years from the 2018 NDHS were the study population.
Data source and sample size
This study utilizes data from the 2018 NDHS, obtained with authorization through an online request system on their official website (https://dhsprogram.com/). The study focuses on the individual record (IR) file, extracting both dependent and independent variables relevant to fertility preferences. An actual sample size of 37,581 was considered for this analysis of women who expressed their desire to either “have another” child or “no more” children.
Study variables
This study defines the outcome variable as “fertility preference” among Nigerian women. This variable is encoded in binary, with “0” indicating a desire for “no more children” and “1” indicating a desire to “have another child.” The original NDHS data categorized this variable into five options. However, the analysis excluded the remaining categories—“undecided,” “sterilized,” and “declared infecund”—as they do not give unambiguous information on current fertility preference.
The explanatory variables in the study include demographic, socioeconomic, and health factors. They are age, region, woman's education level, ethnicity, wealth index, number of children ever born, birth intentions in the next 5 years, contraceptive use intention, marital status, age at first sexual intercourse, ideal number of children, husband's occupation, and woman’s occupation.
Data management and analysis
Data pre-processing
The raw NDHS data underwent extensive pre-processing to ensure its suitability for machine learning analysis. ML algorithms perform best with clean, complete, and balanced datasets where the number of observations in each category is comparable (23).
The initial analysis revealed a class imbalance in the target variable (fertility preference). Specifically, the class representing women who desired “no more children” (coded as 0) was significantly under-represented compared to those wanting “another child” (coded as 1). To address this, the synthetic minority oversampling technique (SMOTE) was employed. SMOTE creates synthetic data points for the minority class (“no more children”), effectively balancing the class distribution and improving the model's ability to learn from both preferences.
Missing data less than 10% were handled by multiple imputations by multivariate imputation by chained equations (MICE) after checking the type of missing type [missing completely at random (MCAR), missing at random (MAR), or missing not at random (MNAR)]. Continuous variables were transformed into categorical bins, and variables with low-frequency categories were recategorized. These pre-processing steps ensured minimal missingness, near-complete data for all included variables, and improved compatibility with the chosen machine learning algorithms, ultimately leading to a robust and reliable analysis of fertility preferences among Nigerian women.
To identify the most informative variables influencing fertility preferences among Nigerian women, this study employed a multi-step feature selection process. The process began by leveraging existing knowledge and exploratory data analysis (EDA) techniques such as descriptive statistics and visualization to assess data distribution and relationships between variables and outliers. Bivariate logistic regression was then used to analyze the association between each feature and the outcome variable. This method provided a more comprehensive understanding of how each feature impacts the probability of a woman wanting “no more children” compared to wanting “another child.” Following this initial screening, recursive feature elimination (RFE) was implemented, which is a machine learning technique that iteratively removes the least informative features. In addition, a correlation heatmap was then used to identify and eliminate highly correlated features within the continuous feature set to avoid multicollinearity and potential model instability. By incorporating these rigorous steps alongside domain knowledge, this methodology ensured the selection of the most relevant and informative features for building the final prediction model for fertility preferences.
Following the feature selection process, we employed two key techniques to evaluate the relative importance of the chosen features within the final model: permutation importance and Gini importance. Permutation importance is a reliable method that stands out for its model-agnostic nature. It works by randomly shuffling the values of each feature and observing the resulting decrease in model performance (24). Features that lead to a substantial decline in performance when shuffled are deemed more critical for the model's predictions. This method offers a broad understanding of feature importance across different models. In contrast, Gini importance is particularly valuable for understanding feature significance within decision tree models, such as the random forest model used in this study. It quantifies the frequency with which a feature is used to partition the data at each decision node within the individual trees of the random forest. Features that consistently appear at these decision points are considered more influential in the model's final prediction of fertility preference (25).
Model development and evaluation
This study implemented six machine learning algorithms in Python 3.9 to develop a model for predicting fertility preference. The selection of six ML algorithms, which include Logistic Regression (LR), Support Vector Machine (SVM), K-Nearest Neighbors (KNN), Decision Tree (DT), Random Forest (RF), and eXtreme Gradient Boosting (XGBoost), was intentional to capture diverse modeling paradigms (26, 27). Logistic Regression serves as a baseline linear model, while SVM is well-suited for capturing non-linear decision boundaries. KNN, an instance-based method, leverages distance metrics to classify observations, while Decision Tree offers high interpretability. Ensemble methods such as Random Forest and XGBoost, known for their robustness and ability to handle structured datasets, are included to enhance predictive accuracy (26, 28). To ensure the model generalizes well to unseen data, which is a crucial step in the machine learning process, the pre-processed data was split into training (80%) and testing (20%) sets. The training data were used to fit the model, where the target variable (fertility preference) was what the model was predicting, and the selected features (factors influencing fertility preference) acted as predictors. The model's performance was then evaluated on the unseen testing data using various metrics [accuracy, precision, recall, F1-score, and area under the receiver operating characteristic curve (AUROC)] and confusion matrix results. In addition, stratified k-fold cross-validation was employed to further assess the model's generalizability by training and evaluating it on multiple random splits of the data. This evaluation of unseen data helps prevent overfitting, where the model performs well on the training data but fails to generalize to new data. To identify the best model for predicting fertility preference, a comprehensive evaluation using various performance metrics such as accuracy, precision, sensitivity (recall), and F1-score was conducted. These metrics assess the performance of each model in accurately classifying cases based on their fertility preference. To achieve this, a confusion matrix was used to identify true positives (TPs), false positives (FPs), true negatives (TNs), and false negatives (FNs) and all performance metrics were calculated based on these values.
Generalizability assessment using cross-validation
In addition to the metrics above, stratified k-fold cross-validation was employed to assess the generalizability of the models. This technique involves splitting the data into multiple folds while ensuring each fold preserves the original data's class distribution (desire for another child). Training and evaluating the model on these stratified folds provides a more robust estimate of its performance on unseen data, especially for imbalanced datasets. This evaluation of unseen data helps prevent overfitting, where the model performs well on the training data but fails to generalize to new data (Figure 1).
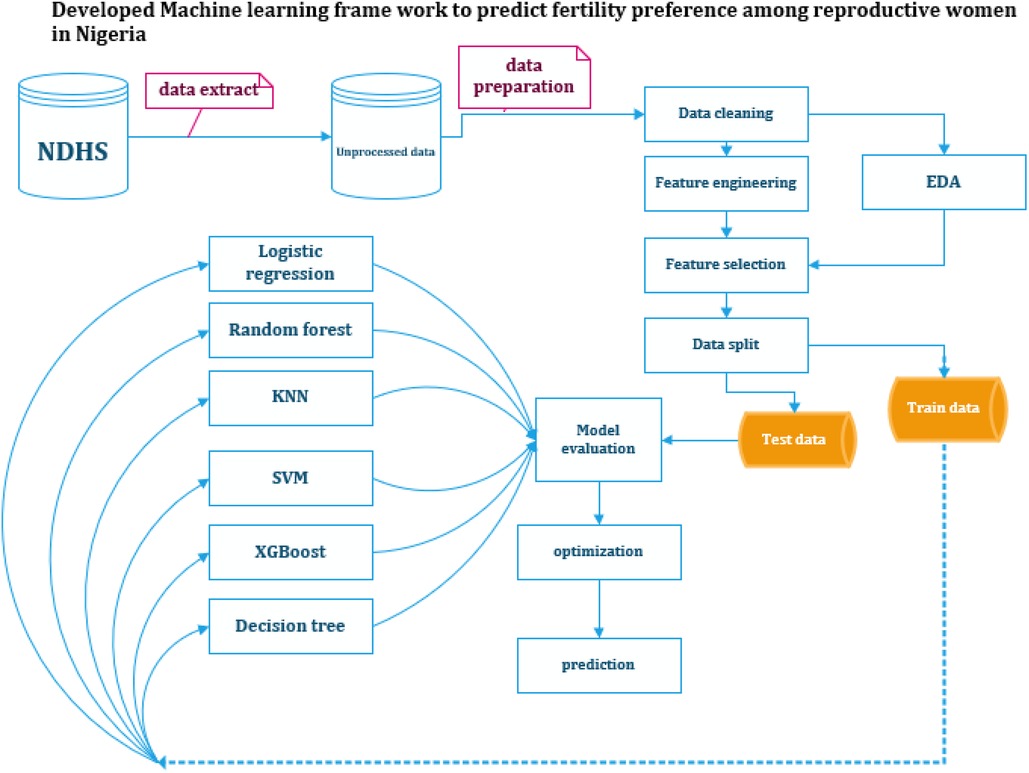
Figure 1. Summary of the applied framework for predicting fertility preference among reproductive age women in Nigeria. NDHS, Nigeria Demographic and Health Survey; EDA, exploratory data analysis; SVM, Support Vector Machine; KNN, K-Nearest Neighbors; XGBoost, eXtreme Gradient Boosting. Created using Microsoft Visio.
Statistical analysis
A comprehensive statistical analysis using STATA 15 was conducted as a crucial preliminary step to understand fertility preference and enhance the subsequent machine learning models. This analysis served three key purposes. First, exploring the distribution of fertility preference across key variables with descriptive statistics and cross-tabulations provided insights into factors influencing fertility preference. Second, the statistical analysis informed the selection of features for the machine learning models. Finally, logistic regression analysis was employed to explore further the relationship between key variables and the outcome variable (fertility preference). This analysis, particularly focusing on adjusted odds ratios (aORs), identified statistically significant associations between several variables and the desire for another child. By integrating these statistical methods, the analysis not only enriched the understanding of fertility dynamics but also laid the groundwork for robust and reliable predictive machine learning models.
Results
Sociodemographic characteristics of respondents
Table 1 presents the sociodemographic characteristics of the 37,581 women aged 15–49 years included in the study. Women aged 15–29 years constituted 57.91% of the respondents. The participants were distributed across the six geopolitical zones of Nigeria, with the North West having the highest proportion (23.79%). Most respondents (59.19%) resided in rural areas. Regarding education, nearly two-thirds (64.1%) had at least a primary education. The wealth index distribution showed a relatively even spread across the five categories. Contraceptive use was prevalent among 13.63% of the respondents, with a significant proportion (34.83%) intending to use contraceptives in the future. The majority (66.21%) had exposure to media. The reproductive history of the respondents varied, with a substantial proportion (42.03%) having one or fewer children.
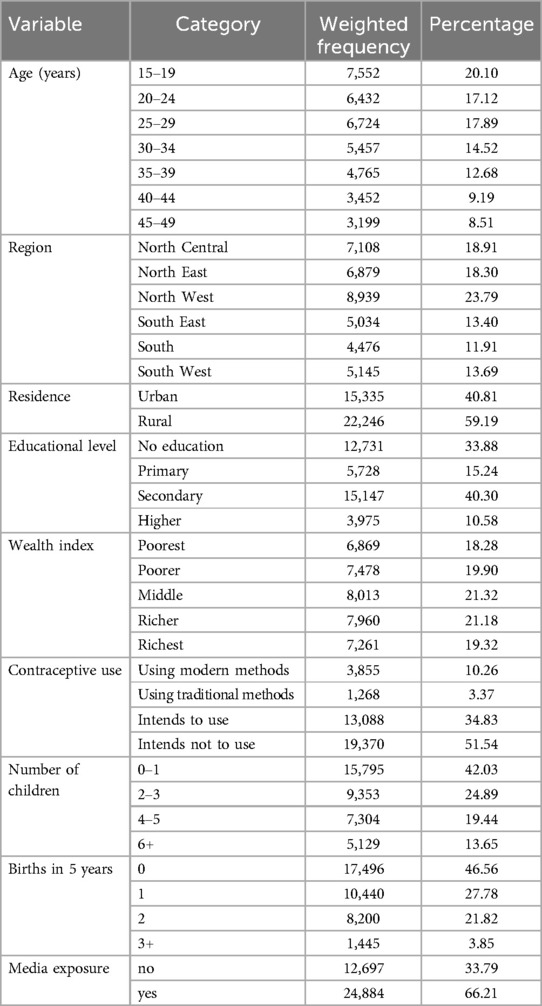
Table 1. Sociodemographic characteristics of reproductive age women in Nigeria in 2018 (N = 37,581).
Inferential statistics
Table 2 illustrates the inferential statistics analysis with logistic regression. This analysis reveals the strong relationships between sociodemographic factors and fertility desires.
Age shows a clear negative association, with women aged 30–34 years having a 64% lower desire for more children (aOR = 0.64, 95% CI: 0.43–0.94, p = 0.024) compared to the youngest group (15–19 years). This trend strengthens further, with the 45-to-49-year-old group exhibiting a 96% lower desire for more children (aOR = 0.04, 95% CI: 0.03–0.06, p < 0.001). Significant regional disparities existed, with the North West and North East regions exhibiting a distinctly higher desire for additional children compared to the national average. Women in the North West were three times more likely to desire more children (aOR = 3.01, 95% CI: 2.59–3.49, p < 0.001), while the North East also showed a similar increase (aOR = 2.87). In contrast, those in the South West region were the least likely to desire more children (aOR = 0.59).
Educational attainment also played a significant role, with individuals who were educated having statistically lower odds of wanting more children compared to those with no education (reference category). The odds ratios for primary, secondary, and higher education were 0.80 (95% CI 0.71–0.91, p = 0.001), 0.81 (95% CI 0.71–0.93, p = 0.003), and 0.78 (95% CI 0.64–0.95, p = 0.013), respectively, indicating a 20%–22% decrease in the desire for more children with each increase in the level of education. Wealth followed a similar trend, with wealthier individuals (richest and middle income) being less likely to desire more children (aOR = 0.70, 95% CI: 0.59–0.84, p < 0.001; aOR = 0.77, 95% CI: 0.67–0.90, p = 0.001) compared to the poorest group (reference category, RC).
Contraceptive use was another significant factor influencing fertility preferences, with those intending not to use contraception more likely to want more children (aOR = 2.19, 95% CI: 1.93–2.47, p < 0.001) compared to those intending to use contraceptives (aOR = 1.87, 95% CI: 1.65–2.13, p < 0.001). This points to a strong association between family planning intention and fertility desire.
As expected, the number of children a woman already had significantly reduced the desire for more children. Women with 3–5 children were 90% less likely to want more children than those with 0–1 child (RC), with an aOR of 0.10 (95% CI: 0.80–0.13, p < 0.001). Women with 6 or more children had an odds ratio of 0.01 (95% CI: 0.01–0.01, p < 0.001), indicating a 99% decrease in their desire for more children compared to those with 0–1 children.
Interestingly, recent childbirth experiences also influenced fertility preferences. Women who had two births in the last 5 years were more likely to want another child (aOR = 2.37, 95% CI: 2.09–2.70, p < 0.001) compared to those with no births (RC). Similarly, women with three or more births in the last 5 years had demonstrated a greater desire for additional children (aOR = 2.00, 95% CI: 1.62–2.46, p < 0.001).
Machine learning analysis
This study evaluated six machine learning models (LR, SVM, KNN, DT, RF, and XGBoost) to assess their ability to predict women's fertility preferences in Nigeria. The performance of each model was assessed using various metrics (accuracy, precision, recall, F1-score, and AUROC) and the confusion matrix results (Table 3). The machine learning evaluation analysis revealed exceptional performance from both Random Forest and XGBoost. These models achieved a high accuracy of 0.92 and well-balanced metrics across the board, solidifying their effectiveness in predicting fertility preferences. While both models excelled, the ROC curves revealed a slight advantage for Random Forest which has of area under the curve (AUC)-ROC of 0.98 compared to XGBoost's 0.97 (Figure 2). In addition, Random Forest exhibited a superior precision score (0.94) compared to XGBoost (0.92), indicating a stronger ability to accurately identify true positives (women who want more children) while minimizing false positives.
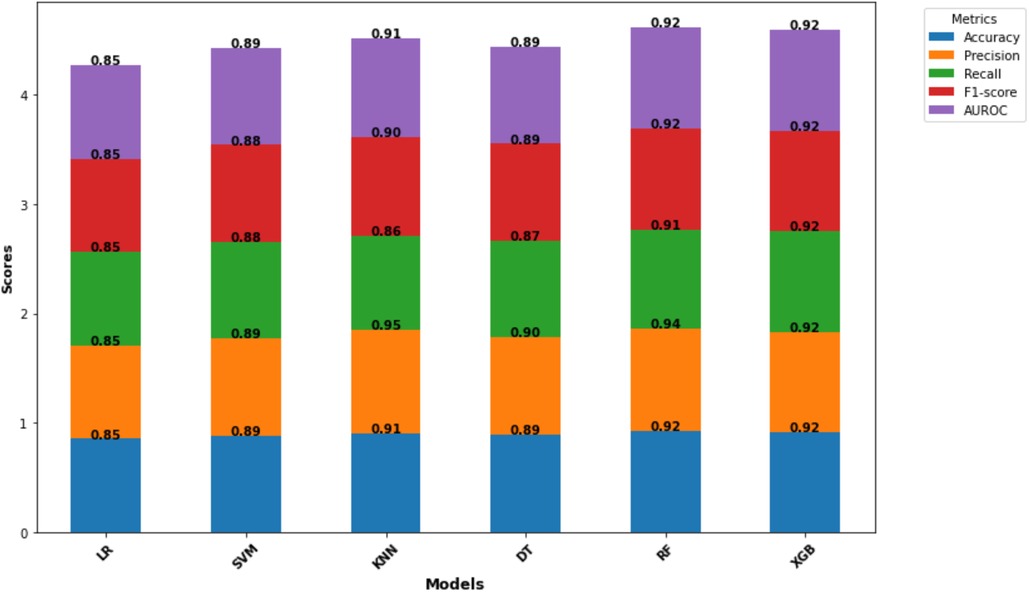
Figure 2. Model comparison and evaluation metrics. Copyright (c) 2024 Project Jupyter Contributors. All rights reserved. Licensed under BSD-3-Clause.
Given the research focus on minimizing false positives (mistakenly classifying women who do not desire more children as wanting more), Random Forest emerges as the preferred model. This prioritizes a cautious approach, reducing the risk of misclassification. While XGBoost demonstrated exceptional consistency with near-perfect recall and F1-score metrics, Random Forest's combined strengths in AUROC and precision, and its alignment with the research goal make it the optimal choice for this study.
The performance of the six ML models employed for the study was further analyzed using ROC curves. The ROC curve is a fundamental tool in machine learning classification tasks, providing a visual representation of the model's ability to discriminate between positive and negative cases across various classification thresholds (24). It plots the true positive rate (TPR) of correctly identifying women desiring more children against the false positive rate (FPR) of incorrectly classifying women who do not. By analyzing the ROC curve and the AUROC, a valuable insight is gained into how effectively the model balances these competing factors, i.e., accurately identifying true positives while minimizing false positives. The ROC curve analysis reveals impressive performance across all six models in predicting the outcome, with AUROC values ranging from 0.90 to 0.98 (Figure 3). This signifies a strong overall ability to distinguish between women who want more children and those who do not. Among the models, Random Forest stands out with the highest AUROC score of 0.98, followed closely by XGBoost at 0.97 and KNN at 0.96.
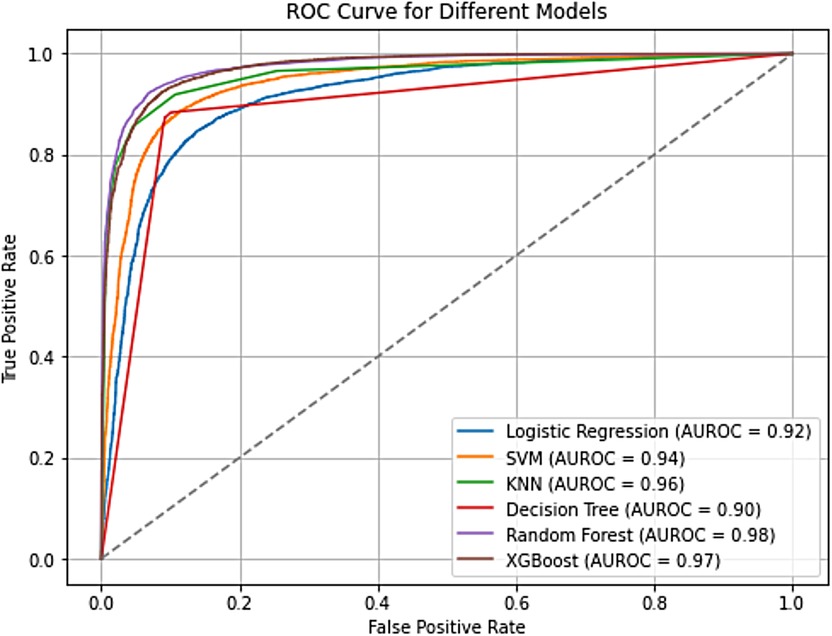
Figure 3. ROC curve for the predictive models. Copyright (c) 2024 Project Jupyter Contributors. All rights reserved. Licensed under BSD-3-Clause.
Features importance analysis
This analysis utilizes two feature importance techniques to shed light on these factors within the Random Forest model.
Permutation feature importance
Permutation feature analysis from the Random Forest model reveals the factors most influential in predicting fertility desire among women in Nigeria (Figure 4). The chart ranks features based on their “permutation importance,” with the X-axis representing the feature and the Y-axis showing its relative significance. The number of children a woman already has (“no_child”) emerged as the most critical factor, reaffirming its pivotal role in shaping fertility preferences. This was followed closely by a woman's age group (“age_group”) and her ideal number of children (“ideal_no”), which also holds substantial influence over the model's predictions. In addition, features such as the number of births in 5 years (“birth_5”), regional variations (“region”), and age at first sexual intercourse (“age_first_sex”) demonstrate moderate importance. The remaining features, including contraceptive intention, ethnicity, spousal occupation, wealth index, woman's occupation, education level, and marital status, contribute to the model's prediction but to a lesser extent.
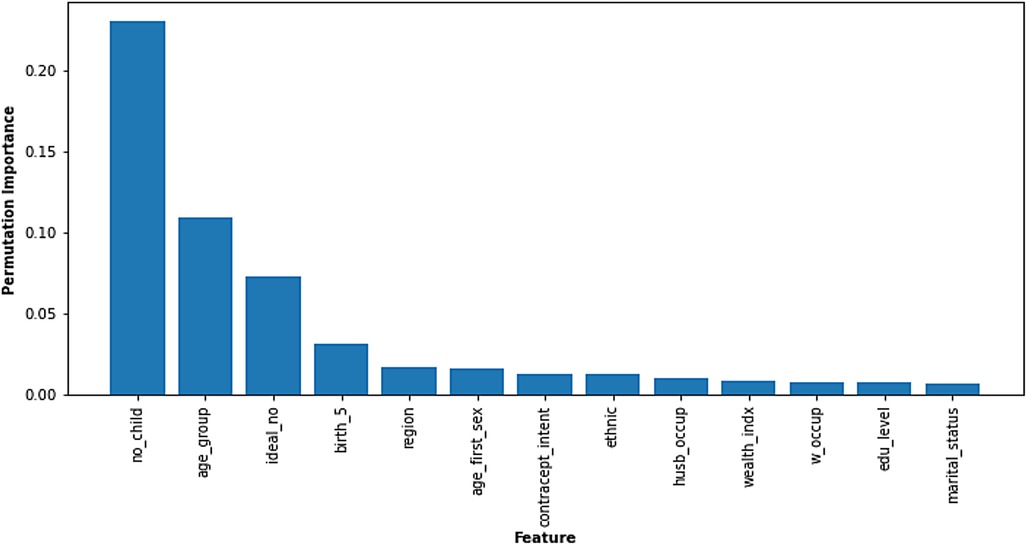
Figure 4. Permutation feature importance. Copyright (c) 2024 Project Jupyter Contributors. All rights reserved. Licensed under BSD-3-Clause.
Gini impurity feature importance
Gini impurity, a measure of node impurity within decision trees, serves as another metric for feature importance in the Random Forest model. The Gini impurity features importance chart (Figure 5) reflects this, depicting a similar pattern to the permutation importance analysis. Features ranked higher on the X-axis with greater Gini importance (Y-axis) contribute more significantly to the model's predictions. Notably, the number of children a woman already has (“no_child”) emerged as the most influential factor, reaffirming its paramount importance. This was followed closely by a woman's age group (“age_group”) and her ideal family size (“ideal_no”), which also exhibited substantial influence on the model's predictions. In addition, regional variations (“region”), number of births in the last 5 years (“birth_5”), and age at first sexual intercourse (“age_first_sex”) demonstrate moderate importance. While these features prominently shape the model's predictions, others such as contraceptive intention, ethnicity, spousal occupation, wealth index, woman's occupation, education level, and marital status are also considered but with a lesser impact.
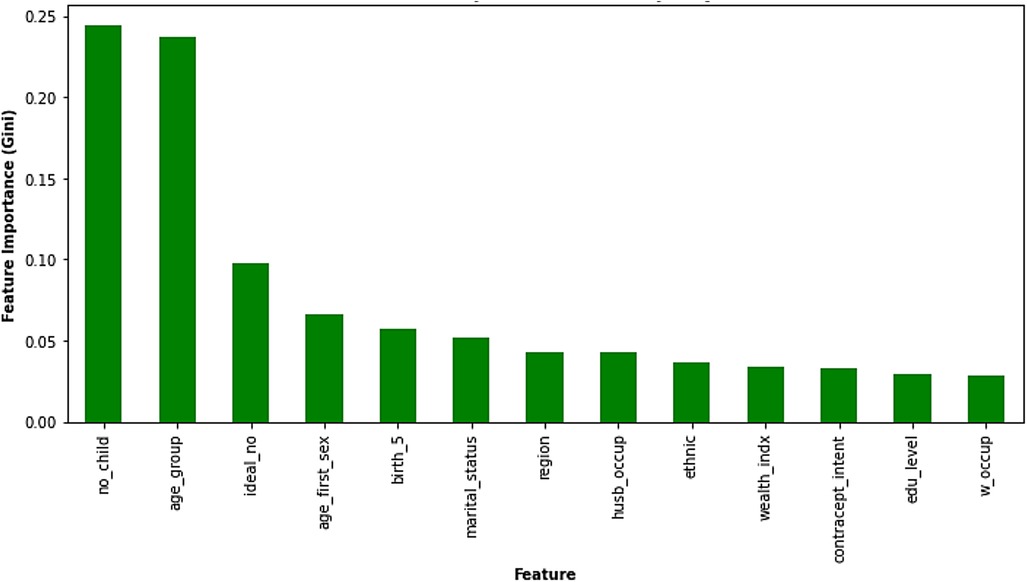
Figure 5. Gini impurity feature importance. Copyright (c) 2024 Project Jupyter Contributors. All rights reserved. Licensed under BSD-3-Clause.
Discussion
This study aimed to predict and identify the predictors of fertility preference among women in Nigeria. A sample of 37,581 women was included from the 2018 NDHS data. This study covers a less-explored area of demographic research methodology by employing machine learning algorithms to predict the fertility preferences of women in Nigeria. Before employing the ML algorithms, a preliminary statistical analysis of the predictors was performed using traditional logistic regression, which served as a starting point. The six machine learning algorithms employed and evaluated for the study include LR, SVM, KNN, DT, RF, and XGBoost. All the models performed well, with accuracy scores ranging from 0.85 for LR to 0.92 for both RF and XGBoost. However, since the best model needed to be chosen, a closer examination revealed trade-offs between each model's strengths and weaknesses. Model selection was guided by the research objectives and, in this study, minimizing false positives, i.e., incorrectly classifying women as preferring to have more children, was paramount due to the implication of misclassification in healthcare and policy interventions (29–32).
Logistic Regression, with a consistency score of 0.85 across all the metrics, provided a foundation. Its critical limitation was its inability to minimize FN (887) which indicates that it might have missed many women who would truly prefer to have more children. This could lead to a misallocation of resources (33, 34). SVM, however, demonstrated a commendable overall performance with accuracy ranging from 0.88 to 0.89, but the model struggled to minimize true positives with FP (629). KNN stood out for its exceptional precision of 0.95 which indicates its strong ability to minimize false positives compared to LR and SVM. This is critical for policy interventions (35). However, it has a lower recall rate of 0.86 which suggests that it might have missed more true positives, exemplifying the trade-offs between precision and recall (29, 32). DT exhibited a balanced performance with scores of around 0.89 for most of the metrics (Figure 2). Notably, it was able to minimize FN (565), offering a good advantage for interpretability (36). However, it is sensitive to data quality and has a tendency of overfitting which requires careful consideration (37). Random Forest and XGBoost emerged as the top performers with the highest accuracy of 0.92 and precision of 0.94. The confusion matrix demonstrated a balanced performance for RF with high TP and fewer misclassifications. It also exhibited a high recall of 0.91 which further emphasized its effectiveness. XGBoost, in comparison, showed a slightly higher recall of 0.92, but RF surpassed it in precision with 0.94 (Figure 2). Similarly, a recent study conducted in Abadan and Khorramshahr (Khuzestan Province, Iran) compared seven ML models to predict the tendency of childbearing and RF was chosen as the best model (38).
While this study primarily focused on the prediction of fertility preferences among Nigerian women using ML algorithms, a comparison with recent state-of-the-art methodologies highlights the robustness of the employed approach. For instance, in a study utilizing ML algorithms SVM, RF, and multi-layer perceptron (MLP) for breast cancer classification, similarly, high accuracy and interpretability were achieved, with MLP attaining an AUC of 99.71% (39). Further comparisons with other research studies have demonstrated the utility of ML techniques in disease prediction and healthcare, emphasizing the value of feature-level insights for improving model interpretability and applicability (40, 41).
The analysis of feature importance with the RF model revealed that the number of living children, woman's age, ideal number of children, number of births in the last 5 years, and regional variations were key predicting factors influencing fertility preferences among Nigerian women. In this study, the number of living children appeared to be the highest predicting factor, which is consistent with previous studies showing that women with a large number of children are more likely to want to limit further childbearing (42, 43). A possible explanation could be that women with a larger number of living children may be satisfied with their current family size or have met their reproductive goals. This finding is also in line with a study from sub-Saharan Africa (44). We observed from this study that a woman's age had a significant contribution to predicting fertility preference. This finding is supported by studies conducted in Ethiopia (45), Iran (46), and Ghana (47) where women aged 15–24 years were more likely to desire more children than other age groups. This could be because younger women have not yet achieved their reproductive goals and are more inclined to want more children later in life (48).
Our study also showed that the ideal number of children is another predicting factor, which aligns with studies conducted in 53 (49) and 78 LMICs from five geographical regions (East and South Africa, Middle and West Africa, Latin America, South and Southeast Asia, and West Asia and North Africa) (50), respectively. Similarly, a study conducted in Ghana (51) indicated that the importance of the ideal number of children resonates with studies that highlight that women who express an ideal number of children tend to strive to achieve their preference (52). Furthermore, other significant factors were educational attainment, marital status, and economic status, which are all known to influence reproductive choices and opportunities, but these had a smaller effect on our findings (51–53). Discrepancies in research scope and setting, the sample population, and the time these studies were conducted are all plausible explanations for the differences in study findings.
Conclusion
This study explored the application of machine learning algorithms to predict fertility preferences among Nigerian women, offering a novel approach with potential application in family planning interventions. By evaluating and comparing ML models, this study demonstrated the potential of advanced analytical methods in capturing complex interactions between variables that traditional statistical models may ignore. Random Forest emerged as the best model due to its high precision and AUROC values, offering a dependable tool for minimizing false positives in fertility predictions. The analysis of feature importance highlighted key factors such as a woman's current number of children, age group, and ideal family size, which are consistent with existing demographic studies. These findings not only improve our understanding of fertility preferences in Nigeria, but also demonstrate the broader applicability of machine learning techniques in demographic studies, notably for family planning and resource allocation.
Limitation and strength
This study leverages Demographic and Health Survey (DHS) data which is a valuable resource due to its extensive population coverage. However, DHS data, including the 2018 Nigerian data used for this study, are susceptible to self-reporting biases. Social desirability bias, for example, can influence responses on sensitive topics such as fertility preferences. In addition, we only accounted for the fertility preference of women, but fertility preference could be affected by the preference of a partner. Furthermore, the complexity of DHS data presented challenges during feature engineering, raising the possibility that relevant variables influencing fertility preferences might have been overlooked.
Despite these limitations, the study offers valuable insights. Cross-validation techniques effectively addressed concerns about overfitting, strengthening the confidence we can have in the generalizability of the models. These findings can inform future research and policy interventions aimed at addressing fertility concerns. Furthermore, this study contributes to the existing literature on fertility preference and machine learning.
Data availability statement
The original contributions presented in the study are included in the article/Supplementary Material, further inquiries can be directed to the corresponding author.
Ethics statement
The studies involving humans were approved by the Demographic and Health Survey via email. The studies were conducted in accordance with the local legislation and institutional requirements. Written informed consent for participation was not required from the participants or the participants' legal guardians/next of kin in accordance with the national legislation and institutional requirements.
Author contributions
ZT: Conceptualization, Data curation, Formal Analysis, Investigation, Methodology, Software, Supervision, Visualization, Writing – original draft, Writing – review & editing. TN: Conceptualization, Data curation, Supervision, Validation, Writing – original draft, Writing – review & editing. KM: Conceptualization, Data curation, Supervision, Validation, Writing – original draft, Writing – review & editing. FG: Conceptualization, Data curation, Supervision, Validation, Writing – original draft, Writing – review & editing. IW: Conceptualization, Data curation, Supervision, Validation, Writing – original draft, Writing – review & editing. JS: Conceptualization, Data curation, Formal Analysis, Investigation, Methodology, Software, Writing – original draft, Writing – review & editing.
Funding
The author(s) declare that no financial support was received for the research, authorship, and/or publication of this article.
Acknowledgments
We are grateful to the Demographic and Health Survey Program for their tremendous work in making the survey data accessible to the public for study.
Conflict of interest
The authors declare that the research was conducted in the absence of any commercial or financial relationships that could be construed as a potential conflict of interest.
Publisher's note
All claims expressed in this article are solely those of the authors and do not necessarily represent those of their affiliated organizations, or those of the publisher, the editors and the reviewers. Any product that may be evaluated in this article, or claim that may be made by its manufacturer, is not guaranteed or endorsed by the publisher.
Abbreviations
AUC, area under the curve; EDA, exploratory data analysis; FN, false negative; FP, false positive; LMIC, low- and middle-income countries; MICE, multivariate imputation by chained equations; ML, machine learning; NDHS, Nigeria Demographic and Health Survey; RFE, recursive feature elimination; ROC, receiver operating characteristic; TP, true positive; TN, true negative.
References
1. Thomson E. Family size preferences. In: James DW, editor. International Encyclopedia of the Social & Behavioral Sciences, Vol. 8. Oxford: Elsevier (2015). p. 805–8.
2. Akinyemi JO, Dipeolu OI, Adebayo AM, Gbadebo BM, Ajuwon GA, Olowolafe TA, et al. Social consequences of COVID-19 on fertility preference consistency and contraceptive use among Nigerian women: insights from population-based data. Contracept Reprod Med. (2022) 7(1):14. doi: 10.1186/s40834-022-00181-0
3. van Tintelen AMG, Stulp G. Explaining uncertainty in women’s fertility preferences. Heliyon. (2024) 10(6):e27610. doi: 10.1016/j.heliyon.2024.e27610
4. United Nation population division. Family planning indicators. (2022). Available online at: https://www.un.org/development/desa/pd/data/family-planning-indicators (Cited June, 2024).
5. Ibitoye M, Casterline JB, Zhang C. Fertility preferences and contraceptive change in low- and middle-income countries. Stud Fam Plann. (2022) 53(2):361–76. doi: 10.1111/sifp.12202
6. Naya CH, Saxbe DE, Dunton GF. Early effects of the COVID-19 pandemic on fertility preferences in the United States: an exploratory study. Fertil Steril. (2021) 116(4):1128–38. doi: 10.1016/j.fertnstert.2021.05.092
7. Iacovou M, Tavares LP. Yearning, learning, and conceding: reasons men and women change their childbearing intentions. Popul Dev Rev. (2011) 37(1):89–123. doi: 10.1111/j.1728-4457.2011.00391.x
8. Atake E-H, Gnakou Ali P. Women’s empowerment and fertility preferences in high fertility countries in Sub-Saharan Africa. BMC Women’s Health. (2019) 19(1):54. doi: 10.1186/s12905-019-0747-9
10. Götmark F, Andersson M. Human fertility in relation to education, economy, religion, contraception, and family planning programs. BMC Public Health. (2020) 20(1):265. doi: 10.1186/s12889-020-8331-7
11. Kruk ME, Gage AD, Arsenault C, Jordan K, Leslie HH, Roder-DeWan S, et al. High-quality health systems in the Sustainable Development Goals era: time for a revolution. Lancet Glob Health. (2018) 6(11):e1196–252. doi: 10.1016/S2214-109X(18)30386-3
12. Sobotka T, Matysiak A, Brzozowska Z. Policy Responses to low Fertility: How Effective are They, Vol. 98. New York, NY: United Nations Population Fund (2019).
13. Odusina EK, Ayotunde T, Kunnuji M, Ononokpono DN, Bishwajit G, Yaya S. Fertility preferences among couples in Nigeria: a cross sectional study. Reprod Health. (2020) 17(1):92. doi: 10.1186/s12978-020-00940-9
15. Tesfa D, Tiruneh SA, Gebremariam AD, Azanaw MM, Engidaw MT, Kefale B, et al. The pooled estimate of the total fertility rate in sub-Saharan Africa using recent (2010–2018) Demographic and Health Survey data. Front Public Health. (2022) 10:1053302. doi: 10.3389/fpubh.2022.1053302
16. Akeju K, Owoeye T, Ayeni R, Jegede L, et al. Variations in desired fertility preferences among young and older women in Nigeria: evidence from demographic health survey 2018. Open Public Health J. (2021) 14(1):84–93. doi: 10.2174/1874944502114010084
17. Prata N, Bell S, Weidert K, Nieto-Andrade B, Carvalho A, Neves I. Varying family planning strategies across age categories: differences in factors associated with current modern contraceptive use among youth and adult women in Luanda, Angola. Open Access J Contracept. (2016) 7:1–9. doi: 10.2147/OAJC.S93794
18. Kraft JM, Serbanescu F, Schmitz MM, Mwanshemele Y, Ruiz C AG, Maro G, et al. Factors associated with contraceptive use in sub-Saharan Africa. J Womens Health (Larchmt). (2022) 31(3):447–57. doi: 10.1089/jwh.2020.8984
19. Christen P, Schnell R. Thirty-three myths and misconceptions about population data: from data capture and processing to linkage. Int J Popul Data Sci. (2023) 8(1):2115. doi: 10.23889/ijpds.v8i1.2115
20. Liu F, Panagiotakos D. Real-world data: a brief review of the methods, applications, challenges and opportunities. BMC Med Res Methodol. (2022) 22(1):287. doi: 10.1186/s12874-022-01768-6
21. Mobarak MH, Mimona MA, Islam MA, Hossain N, Zohura FT, Imtiaz I, et al. Scope of machine learning in materials research—a review. Appl Surf Sci Adv. (2023) 18:100523. doi: 10.1016/j.apsadv.2023.100523
22. Bozkurt S, Cahan EM, Seneviratne MG, Sun R, Lossio-Ventura JA, Ioannidis JPA, et al. Reporting of demographic data and representativeness in machine learning models using electronic health records. J Am Med Inform Assoc. (2020) 27(12):1878–84. doi: 10.1093/jamia/ocaa164
23. Frye M, Mohren J, Schmitt RH. Benchmarking of data preprocessing methods for machine learning-applications in production. Procedia CIRP. (2021) 104:50–5. doi: 10.1016/j.procir.2021.11.009
24. Pedregosa F, Varoquaux G, Gramfort A, Michel V, Thirion B, Grisel O, et al. Scikit-learn: machine learning in python. J Mach Learn Res. (2011) 12:2825–30.
25. Lundberg SM, Lee S-I. A unified approach to interpreting model predictions. Adv Neural Inf Process Syst. (2017) 30:4765–74.
26. Mahesh B. Machine learning algorithms-a review. Int J Sci Res. (2020) 9(1):381–6. doi: 10.21275/ART20203995
27. Prathap KR, Bhavani R. Study comparing classification algorithms for loan approval predictability (logistic regression, XG boost, random forest, decision tree). J Surv Fish Sci. (2023) 10(1S):2438–47.
28. Bennett M, Hayes K, Kleczyk EJ, Mehta R. Similarities and differences between machine learning and traditional advanced statistical modeling in healthcare analytics. arXiv preprint arXiv:2201.02469 (2022).
29. Antoniou T, Mamdani M. Evaluation of machine learning solutions in medicine. CMAJ. (2021) 193(36):E1425–9. doi: 10.1503/cmaj.210036
30. López Seguí F, Cuxart OE, Villar OMI, Guillamet GH, Gil NP, Bonet JM, et al. A cost-benefit analysis of the COVID-19 asymptomatic mass testing strategy in the north metropolitan area of Barcelona. Int J Environ Res Public Health. (2021) 18(13):7028. doi: 10.3390/ijerph18137028
31. Adeshina YO, Deeds EJ, Karanicolas J. Machine learning classification can reduce false positives in structure-based virtual screening. Proc Natl Acad Sci U S A. (2020) 117(31):18477–88. doi: 10.1073/pnas.2000585117
32. Hossain S, Hasan MK, Faruk MO, Aktar N, Hossain R, Hossain K. Machine learning approach for predicting cardiovascular disease in Bangladesh: evidence from a cross-sectional study in 2023. BMC Cardiovasc Disord. (2024) 24(1):214. doi: 10.1186/s12872-024-03883-2
33. Nusinovici S, Tham YC, Chak Yan MY, Wei Ting DS, Li J, Sabanayagam C, et al. Logistic regression was as good as machine learning for predicting major chronic diseases. J Clin Epidemiol. (2020) 122:56–69. doi: 10.1016/j.jclinepi.2020.03.002
34. Weigard A, Spencer RJ. Benefits and challenges of using logistic regression to assess neuropsychological performance validity: evidence from a simulation study. Clin Neuropsychol. (2023) 37(1):34–59. doi: 10.1080/13854046.2021.2023650
35. Abbas F, Cai Z, Shoaib M, Iqbal J, Ismail M, Ullah A, et al. Machine learning models for water quality prediction: a comprehensive analysis and uncertainty assessment in Mirpurkhas, Sindh, Pakistan. Water (Basel). (2024) 16:941. doi: 10.3390/w16070941
36. Naser MZ. An engineer’s guide to eXplainable artificial intelligence and interpretable machine learning: navigating causality, forced goodness, and the false perception of inference. Autom Constr. (2021) 129:103821. doi: 10.1016/j.autcon.2021.103821
37. Bramer M. Avoiding overfitting of decision trees. In: Bramer M, editor. Principles of Data Mining. London: Springer London (2016). p. 121–36.
38. Moulaei K, Mahboubi M, Ghorbani Kalkhajeh S, Kazemi-Arpanahi H. Prediction of childbearing tendency in women on the verge of marriage using machine learning techniques. Sci Rep. (2024) 14(1):20811. doi: 10.1038/s41598-024-71854-w
39. Paudel P, Saud R, Karna SK, Bhandari M. Determining the major contributing features to predict breast cancer imposing ML algorithms with LIME and SHAP. 2023 International Conference on Electrical, Computer and Energy Technologies (ICECET) (2023). IEEE.
40. Sharma V, Samant SS. A multi-level decision-making framework for heart-related disease prediction and recommendation. Syst Res Inf Technol. (2023) 01(4):7–20. doi: 10.20535/SRIT.2308-8893.2023.4.01
41. Rajpoot NK, Singh P, Pant B, Tripathi V. The future of healthcare: a machine learning revolution. 2023 International Conference on Artificial Intelligence for Innovations in Healthcare Industries (ICAIIHI) (2023). IEEE.
42. Babalola S, Figueroa M-E, Krenn S. Association of mass media communication with contraceptive use in sub-Saharan Africa: a meta-analysis of demographic and health surveys. J Health Commun. (2017) 22(11):885–95. doi: 10.1080/10810730.2017.1373874
43. Dibaba Y. Factors influencing women’s intention to limit child bearing in Oromia, Ethiopia. Ethiop J Health Dev. (2009) 23(1):28–33. doi: 10.4314/ejhd.v23i1.44834
44. Ahinkorah BO, Seidu A-A, Armah-Ansah EK, Budu E, Ameyaw EK, Agbaglo E, et al. Drivers of desire for more children among childbearing women in sub-Saharan Africa: implications for fertility control. BMC Pregnancy Childbirth. (2020) 20(1):778. doi: 10.1186/s12884-020-03470-1
45. Muluneh MW, Moyehodie YA. Determinants of desire for more children among women in Ethiopia. BMC Women’s Health. (2021) 21(1):408. doi: 10.1186/s12905-021-01563-3
46. Kariman N, Simbar M, Ahmadi F, Vedadhir AA. Socioeconomic and emotional predictors of decision making for timing motherhood among Iranian women in 2013. Iran Red Crescent Med J. (2014) 16(2):e13629. doi: 10.5812/ircmj.13629
47. Kodzi IA, Johnson DR, Casterline JB. Examining the predictive value of fertility preferences among Ghanaian women. Demogr Res. (2010) 22:965–84. doi: 10.4054/DemRes.2010.22.30
48. Teshale AB, Worku MG, Tesema GA. Fertility decision and its associated factors in sub-Saharan Africa: a multilevel multinomial logistic regression analysis. BMC Women’s Health. (2022) 22(1):337. doi: 10.1186/s12905-022-01920-w
49. Haque R, Alam K, Rahman SM, Keramat SA, Al-Hanawi MK. Women’s empowerment and fertility decision-making in 53 low and middle resource countries: a pooled analysis of demographic and health surveys. BMJ Open. (2021) 11(6):e045952. doi: 10.1136/bmjopen-2020-045952
50. Casterline JB, El-Zeini LO. Multiple perspectives on recent trends in unwanted fertility in low- and middle-income countries. Demography. (2022) 59(1):371–88. doi: 10.1215/00703370-9644472
51. Ahinkorah BO, Seidu A-A, Armah-Ansah EK, Ameyaw EK, Budu E, Yaya S. Socio-economic and demographic factors associated with fertility preferences among women of reproductive age in Ghana: evidence from the 2014 demographic and health survey. Reprod Health. (2021) 18(1):2. doi: 10.1186/s12978-020-01057-9
52. Endriyas M, Gebru A, Assefa A. Ideal family size decision and its associated factors among women of reproductive age: community survey in southern Ethiopia. PeerJ. (2023) 11:e15103. doi: 10.7717/peerj.15103
Keywords: fertility preference, Demographic and Health Survey, Nigeria, machine learning (ML), maternity
Citation: Tadese ZB, Nimani TD, Mare KU, Gubena F, Wali IG and Sani J (2025) Exploring machine learning algorithms for predicting fertility preferences among reproductive age women in Nigeria. Front. Digit. Health 6:1495382. doi: 10.3389/fdgth.2024.1495382
Received: 12 September 2024; Accepted: 23 December 2024;
Published: 16 January 2025.
Edited by:
Xiaofeng Li, Heilongjiang International University, ChinaReviewed by:
Mohan Bhandari, Samridhhi College, NepalVikas Tripthi, Graphic Era University, India
Judgi T, Sathyabama Institute of Science and Technology, India
Copyright: © 2025 Tadese, Nimani, Mare, Gubena, Wali and Sani. This is an open-access article distributed under the terms of the Creative Commons Attribution License (CC BY). The use, distribution or reproduction in other forums is permitted, provided the original author(s) and the copyright owner(s) are credited and that the original publication in this journal is cited, in accordance with accepted academic practice. No use, distribution or reproduction is permitted which does not comply with these terms.
*Correspondence: Zinabu Bekele Tadese, emluYWJ1YmVrZWxlMTJAZ21haWwuY29t