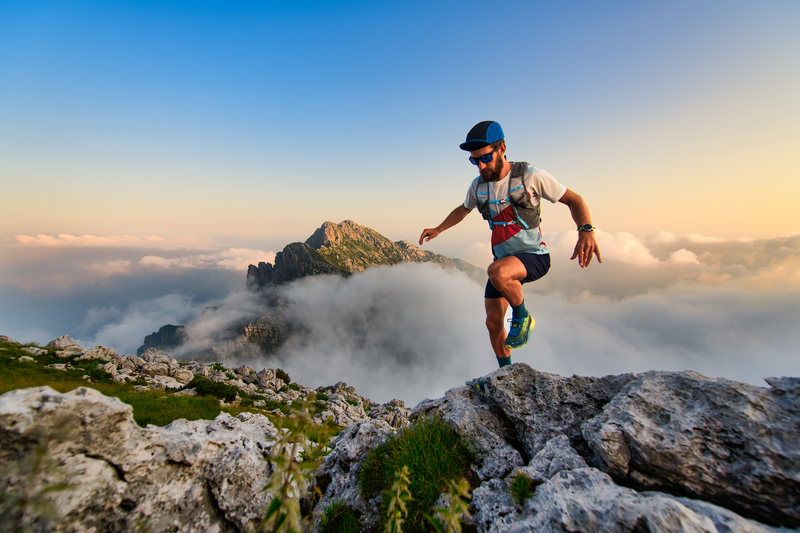
94% of researchers rate our articles as excellent or good
Learn more about the work of our research integrity team to safeguard the quality of each article we publish.
Find out more
ORIGINAL RESEARCH article
Front. Digit. Health , 28 January 2025
Sec. Connected Health
Volume 6 - 2024 | https://doi.org/10.3389/fdgth.2024.1448351
This article is part of the Research Topic Advancing Vocal Biomarkers and Voice AI in Healthcare: Multidisciplinary Focus on Responsible and Effective Development and Use View all articles
Introduction: Artificial intelligence (AI) models trained on audio data may have the potential to rapidly perform clinical tasks, enhancing medical decision-making and potentially improving outcomes through early detection. Existing technologies depend on limited datasets collected with expensive recording equipment in high-income countries, which challenges deployment in resource-constrained, high-volume settings where audio data may have a profound impact on health equity.
Methods: This report introduces a novel protocol for audio data collection and a corresponding application that captures health information through guided questions.
Results: To demonstrate the potential of Voice EHR as a biomarker of health, initial experiments on data quality and multiple case studies are presented in this report. Large language models (LLMs) were used to compare transcribed Voice EHR data with data (from the same patients) collected through conventional techniques like multiple choice questions. Information contained in the Voice EHR samples was consistently rated as equally or more relevant to a health evaluation.
Discussion: The HEAR application facilitates the collection of an audio electronic health record (“Voice EHR”) that may contain complex biomarkers of health from conventional voice/respiratory features, speech patterns, and spoken language with semantic meaning and longitudinal context–potentially compensating for the typical limitations of unimodal clinical datasets.
The COVID-19 pandemic underscored the limitations of healthcare systems and highlighted the need for data innovations to support both care providers and patients. The high volume of patients seeking medical care for COVID-19 and other viral infections has caused extraordinary challenges, including long waitlists, limited time for each patient, increased testing costs, exposure risks for healthcare workers, and documentation burdens (1). Adding to the problem, the world is facing nursing and physician shortages that are expected to rise dramatically over the next 10 years (2–4). This contributes to the increasing rates of provider burnout and a loss of trust in the healthcare system, both of which have been particularly severe since the onset of the COVID-19 pandemic (5–7). To address these problems, artificial intelligence (AI) has been proposed as a mechanism to rapidly perform key clinical tasks such as diagnostics, triage, and patient monitoring, improving the efficiency of the healthcare system. This has become particularly true with the advent of GPT and other multimodal large language models (LLMs), which have advanced capabilities in question answering, image interpretation, programming, and other complex tasks (8, 9). As a result, technology companies have begun to develop foundation AI models for the healthcare space. These are often designed for preprocessing and diagnostic tasks with privileged data (e.g., images) or as Chatbot tools for question-answering (10, 11). While future LLMs may add value to the healthcare system, serious data challenges remain for the widespread, equitable deployment of AI models in healthcare. Below, several primary obstacles are outlined:
In many cases, clinical AI models require correlated data—different sources of information from the same patient within the same approximate period of time. Datasets also require extensive curation, which is often expensive, inconvenient, and frequently overlooked as a challenge in the development of health AI. Multimodal data must be linked from across disjointed sources/centers, which often have incompatible systems and different regulatory structures.
Currently, many AI technologies are dependent on the availability, quality, and breadth of data in electronic health records (EHR). Yet, robust EHR data is often unavailable or inaccurate in many settings, particularly in resource-constrained areas such as low and middle- income countries (LMICs) or rural areas in high-income countries (12). These disparities are due to many factors, which include biased allocation of healthcare services, gaps in insurance coverage, and other barriers (e.g., transportation) due to a lack of providers or facilities (13). As a result, training data for AI models is often biased against underserved populations (14).
The data collected in current clinical workflows is incompatible with most AI systems, causing development challenges and hesitancy from healthcare workers, who make decisions based on patient reporting, their own observations, and various tests—not narrow unimodal datasets collected in research settings. Figure 1 (below) highlights the disconnect between the conditions for data collection in funded research projects and those for data collection or inference in real-world settings, which, in some ways, are more similar to the uncontrolled nature of data mined from online sources.
Figure 1. Comparison of audio data collected from research studies with hospital audio data and data from public online sources (i.e., YouTube).
This work makes the following contributions to the structure and collection of healthcare data:
1. Development of an online application (Healthcare via Electronic and Acoustic Records, “HEAR”) to facilitate the collection of semi-structured multimodal data (text-audio pairs) for developing AI models. The application is designed to be intuitive for patients and technically lightweight for deployment in low-connectivity areas. This system simultaneously captures patient-reported health information (via recorded speech) and unique variations in sound data (changes in voice/speech) without the potential inconsistency of methods such as ambient listening. In a single setting, the HEAR application facilitates rapid collection of health data for training AI models, including retrospective context and factors related to circumstances/lifestyle. The user is not required to type text into a lengthy form, which may cause respondent fatigue and result in data with a high degree of missingness (15, 16).
2. Presentation of demographic statistics, experimental results, and case studies from an initial Voice EHR dataset. Large language models were used to compare the value of information contained in the Voice EHR with data collected via manual inputs. These preliminary results demonstrate the potential viability of low-cost voice EHR data collected across multiple settings, including hospitals.
Audio data has previously shown potential as a diagnostic tool. The idea that patients with certain conditions might present with unique changes in their voice before showing more progressive signs of disease largely originated with Parkinson's disease. Multiple studies have shown that Parkinson's disease is associated with characteristic and progressive changes in phonation over the disease course, including biomarkers such as decreased word stress, softened consonants, abnormal silences, and monotone speech (17–20). Similarly, many studies have since identified specific voice changes in patients with asthma, COPD, interstitial lung disease, rheumatoid arthritis, chronic pain, diabetes, and laryngeal cancer (21–27). The formation of multi-site data generation projects like the Bridge2AI Voice Consortium shows the increasing interest in leveraging voice as a data modality for healthcare applications (28).
During the COVID-19 pandemic, the demand for low-cost digital healthcare solutions surged, providing an ideal setting to advance audio AI technology. As a result, multiple machine learning methods were trained on voice data to predict COVID-19 positivity or variant status (29–42). However, many of these models were not deployed due to limitations of the training datasets (described below), and there was no significant evidence that voice/audio AI methods improved COVID-19 screening during the pandemic (43, 44).
1. Dataset Size/Diversity: Many voice AI studies are reliant on small datasets collected from a narrow range of English-speaking patients using high-cost technology like recording booths, preventing deployment in hospitals or at-home settings (See Figure 1 for comparison of “research data” and data from real-world environments).
2. Data Quality: Multiple past studies were built around crowdsourced datasets, which face significant issues with data quality– reliable annotations (specific indications of disease or health state) are difficult to achieve when collecting limited data from a wide range of possible environments (45, 46). Many datasets, which contain scripted voice samples, may have limited utility due to the lack of context that is needed to account for sources of noise. Moreover, very few datasets were curated through partnerships with healthcare workers in clinical settings, and, as such, do not confirm diagnosis of COVID-19 or other illnesses.
3. Data Breadth: Past audio AI studies, particularly those involving COVID-19 screening/diagnostics, often excluded patients with confirmed cases of other respiratory illnesses—in some cases, only healthy samples were included in the control cohorts (39–42). Typically, users of any diagnostic tool would choose to test themselves because of newly emerging symptoms. This may then confuse an AI model that was trained only to separate between one specific disease state and fully healthy controls or chronic conditions. Moreover, although illnesses like COVID-19 can cause laryngitis and inflammation of the vocal cords causing voice changes, many other factors, such as smoking habits, can also cause laryngitis (47).
This study introduces “Voice EHR”—patients share their past medical history and progression of present illness (if applicable) through audio recordings, creating a patient-driven temporal record of clinical information to compliment and contextualize acoustic data collected simultaneously.
The development of AI models to accurately detect audio biomarkers of disease is dependent on the acquisition of robust training datasets from diverse settings. The proposed “Voice EHR” methods were designed to enable semantic representations of clinical information containing approximate temporal context (e.g., changes from baseline health) with correlated samples of acoustic data: voice/breathing sounds and speech patterns.
This study was approved by the Institutional Review Board of the U.S. National Institutes of Health (NIH). Informed consent was obtained from all participants prior to data collection, using a consent form on the application. Data is stored on NIH-secured cloud servers maintained by Amazon Web Services (AWS) (48). No personally identifying information is stored at this time.
The data collection process was deployed through two primary channels: (1) public use of the application, which is available online at https://www.hearai.org, and (2) partnerships with healthcare professionals working at collaborating point-of care settings. The HEAR app is low-cost, low-bandwidth, fast/easy to use, and does not rely on any specific expensive technologies (e.g., recording booths), facilitating partnerships with healthcare workers in diverse environments. Collaboration with healthcare professionals will help improve the reliability of voice EHR data by providing validated annotations through recruitment of patients with confirmed diagnoses. Providers may engage with patients and ask follow-up questions during the collection process if necessary to enhance data robustness or if internally useful within the clinical workflow (this can be removed before analysis of the sound data). The application can be used by both providers and patients.
The HEAR application was designed to efficiently collect multimodal audio data for health—voice EHR—via a combination of short survey questions and recorded voice/speech/breathing tasks. The HEAR app contains three main sections (Figure 2—left). After obtaining informed consent, data collection begins with multiple-choice questions focused on basic health information (pages 1–5). This section is necessary during the data collection process to ensure a balanced training dataset for initial model development and validation. The recorded Voice EHR data is collected based on written instructions (pages 7–12). The final section is ideally completed with the assistance of a researcher or care provider to document findings, next steps, diagnosis, and other components of the appointment (pages 14–17). Control participants do not complete pages 4, 8, or 14–17. For this study, a control is defined as a participant who does not have an acute condition.
Figure 2. (Left) Overview of the voice EHR data collection app, including initial survey, patient audio, and information from HCWs. (Right) Screenshot from the app (second audio prompt).
This section of the report describes methods for collecting multimodal audio data containing information on voice/breathing sounds and speech patterns as well as semantic meaning from spoken language. Each prompt is designed based on real-world clinical workflows, which may enable the collection of training data that is more aligned with existing healthcare systems. Table 1 contains the voice prompts and a short descriptor of each. After collection, all audio recordings containing spoken language about health were transcribed with Whisper, a large foundation model for speech-to-text tasks (49).
AI models developed from voice EHR data may be trained to perform clinical tasks using only multimodal audio data. However, in the experimental stages, respondents were asked to complete an initial set of questions to contextualize the collected audio data. This was done to ensure class balance, account for possible sources of bias, and run comparative experiments. These data include race, sex, symptoms (including duration and progression), education, insurance, and health history. Zip codes were also collected for epidemiological studies.
The health baseline prompt was designed to provide background data on the participant, ensuring that disease can be modeled as a function of change from a fixed point. Purely cross-sectional datasets are unrealistic, potentially misinforming clinicians in real-world scenarios. No patient would be seen, let alone treated, before the care team reviewed the medical records or collected past medical history.
The second prompt was designed to capture a key interaction between a patient and their provider: “What brings you in today?”. During this interaction, temporal descriptions of illnesses and corresponding patient-initiated interventions (e.g., “taking Tylenol”) are collected, mirroring basic clinical assessments. The aim of this prompt is to ensure clinical information with temporal context is available to complement the sound data. The application asks patients to use basic terminology to describe, in chronological order, the progression of their illness with any associated signs, symptoms, complications, and corresponding interventions. Collecting this information through an audio recording is less burdensome than a typed/written form, potentially serving as a viable substitute for conventional time-series EHR data that is often sparse or unavailable, especially regarding over-the-counter or alternative therapies.
Past Voice AI studies have shown the obstructive impact of variables such as chronic laryngeal conditions or lifestyle factors such as smoking (35). As such, the HEAR application prompts the patient to report any recent changes in voice, speech, or breathing noticed by themselves or others. As with Prompt 1, this prompt aims to replace baseline information in conventional form (i.e., voice samples from prior to illness), which may be unobtainable for many patients. This data may reduce the confounding effect of altered voice sounds or speech patterns that are not related to the current complaint.
Prompt 4 facilitates the collection of conventional acoustic data that is often used in voice AI studies. The first task (Prompt 4, part 1), in which the patient phonates an elongated vowel for as long as possible, may help assess the impact of different variables on how air flows over the vocal cords and indicate the current overall function of the respiratory system. This prompt is a simple method of collecting acoustic features, can be easily translated into other languages, and has been previously used in projects involving crowdsourced data (35). Prompt 4, part 2—the “rainbow passage”—is a validated passage designed to maximize the diversity of acoustic features contained in a single data sample, ensuring that biomarkers are not missed due to limited/narrow inputs (50). These data are collected not only to ensure that pure sound samples are available alongside transcribed speech, but also to provide a mechanism for interoperability and comparison with unimodal data from past studies.
Participants are asked to breathe through the nose normally for 30s (prompt 5, part 1) and take 3 deep breaths with the mouth open (prompt 5, part 2). This data facilitates downstream tasks such as the calculation of respiratory rate (widely used in continuous vital sign monitoring) and the capture of dangerous airway conditions such as stridor or distinctive alterations from supraglottic edema (51, 52).
To further ensure that Voice EHR data contains patient-centered data about medical history and the present illness, Prompt 6 asks if the respondent has any other information that may be important to share (i.e., any contributing information that might not have been covered by past prompts), including challenges faced in engaging with the healthcare system. The addition of this information may lead to improvements in model performance when compared to past health datasets, which have been biased against underserved minority groups or individuals with unique clinical needs not considered in the design of structured EHR systems and standardized surveys.
If available, a healthcare worker will be asked to provide a brief recorded description of the appointment, diagnosis, and treatment plan. This recording may approximate types of clinical data that are often not collected/stored in low-resource settings, including diagnostic tests and other lab results.
This study resulted in the development of an application for the collection of multimodal audio data. “Preliminary efforts resulted in a multi-site dataset of 130 English-speaking patients.”
The total combined length of the recordings was 5.3 hours. Data was excluded from the study if a participant recorded fewer than 2 audio samples, provided audio samples which could not be converted into a readable transcription, or reported no health-related information. These criteria were assessed via manual evaluation by the research team. Data points were also removed if the participant did not complete the demographic/clinical information sections (Pages 1–5 of the application), which were necessary for comparison purposes.
Figure 3 presents demographic statistics for the patients in the initial dataset, including race, age, gender identity, and location of recording (hospital/clinic, home, other). In contrast to other crowdsourced/multi-site voice data generation projects, over half of the samples came from hospital settings (28, 45).
Figure 4 shows the prevalence of health conditions in the dataset, indicating a high occurrence of chronic conditions like hypertension, sleep disorders, depression/anxiety, thyroid disorders, and pain conditions.
Pre-trained large language models (LLMs) were used for conducting additional experiments to compare the information contained in Voice EHR audio recordings with the data initially provided by the patient through manual methods (e.g., multiple choice, short answer). GPT-4o was chosen for this study because this line of models has achieved state-of-the-art performances on various complex tasks, including medical diagnostics (53). Moreover, the use of a pre-trained foundation model eliminated the requirement of additional training/fine-tuning, thereby mitigating concerns about overfitting due to the small size of the dataset featured in this study. These experiments were performed using Voice EHR from participants with an acute complaint who, at minimum, completed the prompts related to health history and current complaint (Prompts 1–2, Section 3.3), resulting in a subset of 41 data points.
In the experiments, GPT-4o was instructed to compare the audio transcripts with the manually input information and rate the two data sources based on a simple rubric designed to reflect general utility of the data in a potential healthcare assessment (Figure 5, Left) (54). The manually input information included the following variables (Table 1): co-morbidities/health challenges (select all that apply, short answer), current symptoms (select all that apply, short answer), progression of symptoms (multiple choice), and duration of symptoms (multiple choice). Results of this experiment showed that, despite averaging less than 90 seconds in length, Voice EHR data on health history and current complaint was consistently more informative (Figure 5, Right).
Figure 5. Left: prompt used for instructing GPT-4o to compare voice EHR audio transcripts with variables collected through conventional mechanisms on the HEAR application. Right: distribution of LLM-generated ratings for the 41 patients included in this experiment.
Figure 5 (Right) shows that, in 83% of cases, the semi-structured audio data from the HEAR application was equally or more informative than manually input data (rating > 2). In 59% of cases, the Voice EHR audio transcript was significantly more valuable (rating = 5). The mean LLM-generated rating was 4.10, with a median of 5 and a standard deviation of 1.36.
To further demonstrate the potential value of information contained in Voice EHR data, examples of basic health information and audio transcripts for patients with illnesses and control participants are presented in Tables 2–6. This is a limited sample of the dataset, for illustrative purposes.
The background health information provided by both patients and controls (Table 3) exemplified valuable data which was not captured in the initial demographic data (Table 2). For example, Patient A discussed acid reflux and recent use of histamines, both of which may be connected to voice changes or other respiratory biomarkers (55, 56). Control A described multiple potential sources of chronic voice and/or speech changes, which may confuse an AI model attempting to diagnose an acute condition. These included asthma, anxiety, fatigue, brain fog, and dysautonomia. Control B described various thyroid conditions which have been associated with changes in the voice, and Control C explained a history of multiple sclerosis (also known to impact the voice/speech) (57–59).
Verbal illness descriptions provided not only longitudinal symptom progression but also extensive use of qualifiers (“moderate”, “very”) that quantify severity or other relationships between signs/symptoms. Additionally, the data contained several instances of patient-initiated interventions within the illness window that could potentially account for fluctuations in audio biomarkers.
Initial viability of voice EHR is further supported by data from subjects reporting changes in their audio profile (Table 5). Patients A and B described voice changes due to illness, which can be linked to conventional sound data, thereby ensuring that these changes are considered separately from irrelevant voice/speech anomalies due to lifestyle, recording quality, or other factors. Control A reported voice and speech changes due to dysautonomia, including voice cracks and difficulty speaking coherently. Control B mentioned two separate voice changes due to Atrial fibrillation and hyperthyroidism. Finally, Control C described voice changes due to multiple sclerosis (MS). Each of these voice/speech irregularities could be falsely predicted as an infection or other new, emerging condition. This type of information is not captured in existing datasets.
The final Voice EHR prompt was used to capture information regarding other aspects of the patient's life or health which they felt were important and may have impacted their current illness. For example, Patient B mentioned a time just before the illness when cleaning products evoked similar symptoms. Control C talked about residual post-operative pain.
Results of this study show that semi-structured Voice EHR data may have equal or additional clinical value compared to manually input data (e.g., multiple choice, short answer). This was true in over 80% of cases, even without considering features like the correlated conventional acoustic data (i.e., vowel phonation, rainbow passage) or the patient-reported data on other circumstances that may have impacted overall health. The creation of a “voice EHR” system introduces numerous potential benefits to the clinical AI space, particularly in settings (i.e., low- and middle- income countries) without a developed health records system to consistently provide detailed longitudinal data for digital health technologies.
The use of voice EHR as training data for AI models may overcome multiple barriers to the safe deployment of such tools for low-resource settings. EHR-driven AI technologies developed in high-income settings may not provide optimal support to medical decision making in resource-constrained settings where the data may be incomplete, incorrect, or “low tech.” While gold-standard annotations like lab results are not collected in all cases, prompts which were co-designed by healthcare workers and data collection partnerships with clinics will help ensure the viability of voice EHR.
The HEAR application facilitates the rapid collection of “Voice EHR” data in a user-friendly way, without (1) requiring time-consuming and error-prone text data entry on the part of the individual, and (2) enforcing a rigid, pre-defined data schema found in traditional EHR, which may limit the incorporation of information that the patient considers to be important. Furthermore, the process of creating a “voice EHR” may be useful to healthcare workers. In the future, transcribed audio may serve as an accompaniment to clinical notes, reducing the redundancy often associated with data collection and potentially enhancing clinical workflows.
With the introduction of text-sound correlates, Voice EHR may additionally compensate for sources of confusion that are often found in clinical data through “biomarker reinforcement.” Even if participants provide incoherent/incomplete data in terms of semantic meaning, the HEAR application still captures voice and breathing data which may independently contribute to the robustness of the data. For example, lapses in patient memory, incomplete notes from healthcare workers, or information reported in colloquial terminology may compromise the value of language data, but acoustic features from the voice may be unaffected in these scenarios. The converse may also be true, in which transcripts of patient-reported health information still provide usable data despite background noise or recording errors (e.g., the device was held too far from the mouth).
For cases in which both modalities are viable, the use of voice/sound data in combination with transcribed health information may capture a more comprehensive composite of diseases with diverse phenotypes, particularly at the time of presentation. For certain diseases, sound data may contribute biomarkers that would not currently be captured in clinician notes. Ultimately, multimodal audio data expands upon the basic health information that is often used for developing digital health systems, potentially allowing AI models to better consider chronic conditions, voice changes, speech patterns, word choices indicating mood/sentiment, potential exposures, behavioral influences, and specific disease progression. Compared to similar methods like ambient listening, semi-structured Voice EHR may also reduce the variability of multimodal audio data, potentially enabling machine learning modelling from a smaller sample size. This methodology may also reduce AI biases against clinics/healthcare environments that do not engage in conventional workflows or styles of patient interaction (reducing the value of ambient listening in these settings).
Implementation of the voice EHR data collection process has presented multiple challenges that must be overcome for adaptation at scale. Prompts for semi-structured data collection, particularly in uncontrolled settings, must be optimized to ensure that patients are easily able to complete the tasks correctly. In the initial voice EHR dataset, there were numerous incomplete samples containing only the initial text survey (no recorded audio). There were also cases in which participants miscategorized themselves as controls—potentially due to unclear criteria—resulting in missing data. Clearer instructions with example videos will be included in future versions of the application. The dataset must also be expanded to ensure access to (1) diverse participants from different demographic subpopulations and (2) data from a broader range of illnesses. The current dataset was mainly collected at a hospital or in the home. However, the highest volume of data for some types of disease (e.g., respiratory infections) might be found in primary/urgent care settings, which may explain the imbalance between chronic and acute conditions. Moreover, this data contains only English speakers, and further study is needed to understand how different languages, levels of literacy, accents, or other linguistic nuances may affect the data transcription process. Finally, in low-bandwidth areas, the simultaneous capture of voice and other modalities like vital signs was time-consuming, posing questions about the scalability of data collection.
Future work will mainly involve dataset expansion to additional sites/settings, including tropical disease hospitals in Vietnam and primary/urgent care centers in the United States, enhancing the overall diversity of the data. Moreover, a privacy-aware, patient-controlled option to create a time-series voice EHR may be introduced to collect personalized control data from participants and run longitudinal studies on how changes in voice/speech/language may prognose future health challenges. Future work will also involve the development of AI models which use Voice EHR data to perform specific clinical tasks, such as diagnosis of respiratory conditions or the prediction of hospital admission based on health status in the emergency room.
This report demonstrates that multimodal audio data can serve as a safe, private, and equitable foundation for new AI models in healthcare. Voice EHR may offer a proxy for detailed time-series data only found in high-resource areas, while simultaneously providing voice, speech, and respiratory data to compliment patient-reported information. Ultimately, AI models trained on voice EHR may be used in the clinic and home, supporting patients in hospital “deserts” where healthcare is not readily accessible. While challenges remain, this work highlights the rich information potentially contained in voice EHR.
The datasets presented in this article are not readily available because a data use agreement must be put in place to protect patient privacy. Requests to access the datasets should be directed toYW5pYmFsanRAbmloLmdvdg==.
The studies involving humans were approved by NIH Institutional Review Board (IRB). The studies were conducted in accordance with the local legislation and institutional requirements. The ethics committee/institutional review board waived the requirement of written informed consent for participation from the participants or the participants' legal guardians/next of kin because online consent was collected via the digital health application presented in the study.
JA: Conceptualization, Data curation, Methodology, Software, Supervision, Writing – original draft, Writing – review & editing. HH: Conceptualization, Methodology, Writing – original draft, Writing – review & editing. ML: Methodology, Software, Writing – original draft, Writing – review & editing. LH: Project administration, Resources, Writing – original draft, Writing – review & editing. VD: Data curation, Methodology, Writing – review & editing. DE: Data curation, Methodology, Writing – review & editing. YL: Methodology, Writing – review & editing. HN: Methodology, Writing – review & editing. PH: Methodology, Writing – review & editing. MK: Data curation, Writing – review & editing. SO: Data curation, Writing – review & editing. CJ: Data curation, Writing – review & editing. LS: Data curation, Writing – review & editing. CE: Data curation, Writing – review & editing. BK: Data curation, Writing – review & editing. LA: Data curation, Writing – review & editing. IL: Data curation, Writing – review & editing. IE: Methodology, Writing – review & editing. LE: Methodology, Writing – review & editing. SJ: Methodology, Writing – review & editing. RN: Methodology, Writing – review & editing. CG: Project administration, Resources, Writing – review & editing. JP: Methodology, Project administration, Writing – review & editing. JB: Writing – review & editing. MS: Writing – review & editing. ER: Methodology, Supervision, Writing – original draft, Writing – review & editing. DC: Methodology, Supervision, Writing – original draft, Writing – review & editing. CT: Methodology, Supervision, Writing – original draft, Writing – review & editing. YB: Methodology, Supervision, Writing – original draft, Writing – review & editing. BW: Methodology, Supervision, Writing – original draft, Writing – review & editing.
The author(s) declare financial support was received for the research, authorship, and/or publication of this article.
This work was supported by the NIH Center for Interventional Oncology and the Intramural Research Program of the National Institutes of Health, National Cancer Institute, and the National Institute of Biomedical Imaging and Bioengineering, via intramural NIH Grants Z1A CL040015 and 1ZIDBC011242. Work was also supported by the NIH Intramural Targeted Anti-COVID-19 (ITAC) Program, funded by the National Institute of Allergy and Infectious Diseases. The participation of HH was made possible through the NIH Medical Research Scholars Program, a public-private partnership supported jointly by the NIH and contributions to the Foundation for the NIH from the Doris Duke Charitable Foundation, Genentech, the American Association for Dental Research, the Colgate-Palmolive Company, and other private donors. DAC was supported by the Pandemic Sciences Institute at the University of Oxford; the National Institute for Health Research (NIHR) Oxford Biomedical Research Centre (BRC); an NIHR Research Professorship; a Royal Academy of Engineering Research Chair; the Wellcome Trust funded VITAL project (grant 204904/Z/16/Z); the EPSRC (grant EP/W031744/1); and the InnoHK Hong Kong Centre for Cerebro-cardiovascular Engineering (COCHE).
The authors declare no competing non-financial interests but the following competing financial interests. NIH may own intellectual property in the field. NIH and BJW receive royalties for licensed patents from Philips, unrelated to this work. BW is Principal Investigator on the following CRADA's=Cooperative Research & Development Agreements, between NIH and industry: Philips, Philips Research, Celsion Corp, BTG Biocompatibles/Boston Scientific, Siemens, NVIDIA, XACT Robotics. Promaxo (in progress). The following industry partners also support research in CIO lab via equipment, personnel, devices and/or drugs: 3T Technologies (devices), Exact Imaging (data), AngioDynamics (equipment), AstraZeneca (pharmaceuticals, NCI CRADA), ArciTrax (devices and equipment), Imactis (Equipment), Johnson & Johnson (equipment), Medtronic (equipment), Theromics (Supplies), Profound Medical (equipment and supplies), QT Imaging (equipment and supplies). The content of this manuscript does not necessarily reflect the views, policies, or opinions of the Uniformed Services University of the Health Sciences, the National Institutes of Health, the US Department of Health and Human Services, the US Department of Defense, the U.K. National Health Service, the U.K. National Institute for Health Research, the U.K. Department of Health, InnoHK – ITC, or the University of Oxford. The mention of commercial products, their source, or their use in connection with material reported herein is not to be construed as an actual or implied endorsement of such products by the U.S. government. This work was prepared by a military or civilian employee of the US Government as part of the individual's official duties and therefore is in the public domain and does not possess copyright protection (public domain information may be freely distributed and copied; however, as a courtesy it is requested that the Uniformed Services University and the author be given an appropriate acknowledgement).
The remaining authors declare that the research was conducted in the absence of any commercial or financial relationships that could be construed as a potential conflict of interest.
All claims expressed in this article are solely those of the authors and do not necessarily represent those of their affiliated organizations, or those of the publisher, the editors and the reviewers. Any product that may be evaluated in this article, or claim that may be made by its manufacturer, is not guaranteed or endorsed by the publisher.
1. Smyrnakis E, Symintiridou D, Andreou M, Dandoulakis M, Theodoropoulos E, Kokkali S, et al. Primary care professionals’ experiences during the first wave of the COVID-19 pandemic in Greece: a qualitative study. BMC Fam Pract. (2021) 22(1):174. doi: 10.1186/s12875-021-01522-9
2. Available online at: https://www.goodrx.com/healthcare-access/research/healthcare-deserts-80-percent-of-country-lacks-adequate-healthcare-access (Accessed March 13, 2024).
3. Zhang X, Lin D, Pforsich H, Lin VW. Physician workforce in the United States of America: forecasting nationwide shortages. Hum Resour Health. (2020) 18(1):8. doi: 10.1186/s12960-020-0448-3
4. Hoyler M, Finlayson SR, McClain CD, Meara JG, Hagander L. Shortage of doctors, shortage of data: a review of the global surgery, obstetrics, and anesthesia workforce literature. World J Surg. (2014) 38:269–80. doi: 10.1007/s00268-013-2324-y
5. Shin P, Desai V, Hobbs J, Conte AH, Qiu C. Time out: the impact of physician burnout on patient care quality and safety in perioperative medicine. Perm J. (2023) 27(2):160. doi: 10.7812/TPP/23.015
6. Ortega MV, Hidrue MK, Lehrhoff SR, Ellis DB, Sisodia RC, Curry WT, et al. Patterns in physician burnout in a stable-linked cohort. JAMA Netw Open. (2023) 6(10):e2336745. doi: 10.1001/jamanetworkopen.2023.36745
7. Pasquini M. Mistrustful dependency: mistrust as risk management in an Italian emergency department. Med Anthropol. (2023) 42(6):579–92. doi: 10.1080/01459740.2023.2240942
9. Touvron H, Martin L, Stone K, Albert P, Almahairi A, Babaei Y, et al. Llama 2: open foundation and fine-tuned chat models. arXiv preprint arXiv:2307.09288 (2023).
10. Li C, Wong C, Zhang S, Usuyama N, Liu H, Yang J, et al. Llava-med: training a large language-and-vision assistant for biomedicine in one day. Adv Neural Inf Process Syst. (2024) 36.39484069
11. Available online at: https://sites.research.google/med-palm/ (Accessed February 21, 2024).
12. Celi LA, Cellini J, Charpignon ML, Dee EC, Dernoncourt F, Eber R, et al. Sources of bias in artificial intelligence that perpetuate healthcare disparities—a global review. PLoS Digit Health. (2022) 1(3):e0000022. doi: 10.1371/journal.pdig.0000022
13. Yang R, Nair SV, Ke Y, D'Agostino D, Liu M, Ning Y, et al. Disparities in clinical studies of AI enabled applications from a global perspective. NPJ Digit Med. (2024) 7(1):209. doi: 10.1038/s41746-024-01212-7
14. Jayatilleke K. Challenges in implementing surveillance tools of high-income countries (HICs) in low middle income countries (LMICs). Curr Treat Options Infect Dis. (2020) 12:191–201. doi: 10.1007/s40506-020-00229-2
15. Le A, Han BH, Palamar JJ. When national drug surveys “take too long”: an examination of who is at risk for survey fatigue. Drug Alcohol Depend. (2021) 225:108769. doi: 10.1016/j.drugalcdep.2021.108769
16. Jeong D, Aggarwal S, Robinson J, Kumar N, Spearot A, Park DS. Exhaustive or exhausting? Evidence on respondent fatigue in long surveys. J Dev Econ. (2023) 161:102992. doi: 10.1016/j.jdeveco.2022.102992
17. Tracy JM, Özkanca Y, Atkins DC, Ghomi RH. Investigating voice as a biomarker: deep phenotyping methods for early detection of Parkinson’s disease. J Biomed Inform. (2020) 104:103362. doi: 10.1016/j.jbi.2019.103362
18. Suppa A, Costantini G, Asci F, Di Leo P, Al-Wardat MS, Di Lazzaro G, et al. Voice in Parkinson’s disease: a machine learning study. Front Neurol. (2022) 13:831428. doi: 10.3389/fneur.2022.831428
19. Tougui I, Jilbab A, Mhamdi JE. Machine learning smart system for Parkinson disease classification using the voice as a biomarker. Healthc Inform Res. (2022) 28(3):210–21. doi: 10.4258/hir.2022.28.3.210
20. Fagherazzi G, Fischer A, Ismael M, Despotovic V. Voice for health: the use of vocal biomarkers from research to clinical practice. Digit Biomark. (2021) 5(1):78–88. doi: 10.1159/000515346
21. Chintalapudi N, Dhulipalla VR, Battineni G, Rucco C, Amenta F. Voice biomarkers for Parkinson’s disease prediction using machine learning models with improved feature reduction techniques. J Data Sci Intell Syst. (2023) 1(2):92–8. doi: 10.47852/bonviewJDSIS3202831
22. Asim Iqbal M, Devarajan K, Ahmed SM. An optimal asthma disease detection technique for voice signal using hybrid machine learning technique. Concurr Comput Pract Exp. (2022) 34(11):e6856. doi: 10.1002/cpe.6856
23. Idrisoglu A, Dallora AL, Cheddad A, Anderberg P, Jakobsson A, Sanmartin Berglund J. COPDVD: automated classification of chronic obstructive pulmonary disease on a new developed and evaluated voice dataset. Artif Intell Med. (2024) 156:4713043. doi: 10.1016/j.artmed.2024.102953
24. Raju N, Augustine DP, Chandra J. A novel artificial intelligence system for the prediction of interstitial lung diseases. SN Comput Sci. (2024) 5(1):143. doi: 10.1007/s42979-023-02524-3
25. Borna S, Haider CR, Maita KC, Torres RA, Avila FR, Garcia JP, et al. A review of voice-based pain detection in adults using artificial intelligence. Bioengineering. (2023) 10(4):500. doi: 10.3390/bioengineering10040500
26. Saghiri MA, Vakhnovetsky A, Vakhnovetsky J. Scoping review of the relationship between diabetes and voice quality. Diabetes Res Clin Pract. (2022) 185:109782. doi: 10.1016/j.diabres.2022.109782
27. Bensoussan Y, Vanstrum EB, Johns MM III, Rameau A. Artificial intelligence and laryngeal cancer: from screening to prognosis: a state of the art review. Otolaryngol Head Neck Surg. (2023) 168(3):319–29. doi: 10.1177/01945998221110839
28. Bensoussan Y, Elemento O, Rameau A. Voice as an AI biomarker of health—introducing audiomics. JAMA Otolaryngol Head Neck Surg. (2024) 150:283–4. doi: 10.1001/jamaoto.2023.4807
29. Ritwik KVS, Kalluri SB, Vijayasenan D. COVID-19 patient detection from telephone quality speech data. Preprint at arXiv (2020).
30. Usman M, Gunjan VK, Wajid M, Zubair M, Siddiquee KNEA. Speech as a biomarker for COVID-19 detection using machine learning. Comput Intell Neurosci. (2022) 2022. doi: 10.1155/2022/6093613
31. Verde L, De Pietro G, Ghoneim A, Alrashoud M, Al-Mutib KN, Sannino G. Exploring the use of artificial intelligence techniques to detect the presence of coronavirus COVID-19 through speech and voice analysis. IEEE Access. (2021) 9:65750–7. doi: 10.1109/ACCESS.2021.3075571
32. Verde L, de Pietro G, Sannino G. Artificial intelligence techniques for the non-invasive detection of COVID-19 through the analysis of voice signals. Arab J Sci Eng. (2021) 48:11143–53. doi: 10.1007/s13369-021-06041-4
33. Bhattacharya D, Dutta D, Sharma NK, Chetupalli SR, Mote P, Ganapathy S, et al. Analyzing the impact of SARS-CoV-2 variants on respiratory sound signals. arXiv preprint arXiv:2206.12309 (2022).
34. Alkhodari M, Khandoker AH. Detection of COVID-19 in smartphone-based breathing recordings: a pre-screening deep learning tool. PLoS One. (2022) 17(1):1–25. doi: 10.1371/journal.pone.0262448
35. Han J, Xia T, Spathis D, Bondareva E, Brown C, Chauhan J, et al. Sounds of COVID-19: exploring realistic performance of audio-based digital testing. NPJ Digit Med. (2022) 5(1):16. doi: 10.1038/s41746-021-00553-x
36. Suppakitjanusant P, Sungkanuparph S, Wongsinin T, Virapongsiri S, Kasemkosin N, Chailurkit L, et al. Identifying individuals with recent COVID-19 through voice classification using deep learning. Sci Rep. (2021) 11(1):19149. doi: 10.1038/s41598-021-98742-x
37. Anibal JT, Landa AJ, Hang NT, Song MJ, Peltekian AK, Shin A, et al. Omicron detection with large language models and YouTube audio data. Medrxiv preprint medrxiv: 2022.09.13.22279673 (2024).
38. Deshpande G, Batliner A, Schuller BW. AI-Based human audio processing for COVID-19: a comprehensive overview. Pattern Recognit. (2022) 122:108289. doi: 10.1016/j.patcog.2021.108289
39. Subirana B, Hueto F, Rajasekaran P, Laguarta J, Puig S, Malvehy J, et al. Hi sigma, do I have the coronavirus? Call for a new artificial intelligence approach to support health care professionals dealing with the covid-19 pandemic. arXiv preprint arXiv:2004.06510 (2020).
40. Brown C, Chauhan J, Grammenos A, Han J, Hasthanasombat A, Spathis D, et al. Exploring automatic diagnosis of COVID-19 from crowdsourced respiratory sound data. Proceedings of the 26th ACM SIGKDD International Conference on Knowledge Discovery & Data Mining (2020).
41. Despotovic V, Ismael M, Cornil M, Call RM, Fagherazzi G. Detection of COVID-19 from voice, cough and breathing patterns: dataset and preliminary results. Comput Biol Med. (2021) 138:104944. doi: 10.1016/j.compbiomed.2021.104944
42. Coppock H, Gaskell A, Tzirakis P, Baird A, Jones L, Schuller B. End-to-end convolutional neural network enables COVID-19 detection from breath and cough audio: a pilot study. BMJ Innov. (2021) 7(2). doi: 10.1136/bmjinnov-2021-000668
43. Coppock H, Nicholson G, Kiskin I, Koutra V, Baker K, Budd J, et al. Audio-based AI classifiers show no evidence of improved COVID-19 screening over simple symptoms checkers. Nat Mach Intell. (2024) 6(2):229–42. doi: 10.1038/s42256-023-00773-8
44. Heaven WD. Hundreds of AI tools have been built to catch covid. None of them helped. MIT Technology Review. (2021).
45. Bhattacharya D, Sharma NK, Dutta D, Chetupalli SR, Mote P, Ganapathy S, et al. Coswara: a respiratory sounds and symptoms dataset for remote screening of SARS-CoV-2 infection. Sci Data. (2023) 10(1):397. doi: 10.1038/s41597-023-02266-0
46. Triantafyllopoulos A, Semertzidou A, Song M, Pokorny FB, Schuller BW. COVYT: introducing the coronavirus YouTube and TikTok speech dataset featuring the same speakers with and without infection. arXiv preprint arXiv:2206.11045 (2022).
47. Awan SN. The effect of smoking on the dysphonia severity index in females. Folia Phoniatr Logop. (2011) 63(2):65–71. doi: 10.1159/000316142
48. Available online at: https://datascience.nih.gov/strides (Accessed March 10, 2024).
49. Radford A, Kim JW, Xu T, Brockman G, McLeavey C, Sutskever I. Robust speech recognition via large-scale weak supervision. International Conference on Machine Learning. PMLR (2023).
51. Nam Y, Reyes BA, Chon KH. Estimation of respiratory rates using the built-in microphone of a smartphone or headset. IEEE J Biomed Health Inform. (2015) 20(6):1493–501. doi: 10.1109/JBHI.2015.2480838
52. Anibal J, Doctor R, Boyer M, Newberry K, DeSantiago I, Awan S, et al. Transformers for rapid detection of airway stenosis and stridor. medRxiv (2024): 2024-10.
53. Goh E, Gallo R, Hom J, Strong E, Weng Y, Kerman H, et al. Large language model influence on diagnostic reasoning: a randomized clinical trial. JAMA Netw Open. (2024) 7(10):e2440969. doi: 10.1001/jamanetworkopen.2024.40969
54. Available online at: https://platform.openai.com/docs/models (Accessed August 14, 2024).
55. Abaza MM, Levy S, Hawkshaw MJ, Sataloff RT. Effects of medications on the voice. Otolaryngol Clin North Am. (2007) 40(5):1081–90. doi: 10.1016/j.otc.2007.05.010
56. Vashani K, Murugesh M, Hattiangadi G, Gore G, Keer V, Ramesh VS, et al. Effectiveness of voice therapy in reflux-related voice disorders. Dis Esophagus. (2010) 23(1):27–32. doi: 10.1111/j.1442-2050.2009.00992.x
57. Junuzović-Žunić L, Ibrahimagić A, Altumbabić S. Voice characteristics in patients with thyroid disorders. Eurasian J Med. (2019) 51(2):101. doi: 10.5152/eurasianjmed.2018.18331
58. Stogowska E, Kamiński KA, Ziółko B, Kowalska I. Voice changes in reproductive disorders, thyroid disorders and diabetes: a review. Endocr Connect. (2022) 11(3). doi: 10.1530/EC-21-0505
Keywords: AI for health, natural language processing, large language models (LLM), multimodal data, voice biomarkers
Citation: Anibal J, Huth H, Li M, Hazen L, Daoud V, Ebedes D, Lam YM, Nguyen H, Hong PV, Kleinman M, Ost S, Jackson C, Sprabery L, Elangovan C, Krishnaiah B, Akst L, Lina I, Elyazar I, Ekawati L, Jansen S, Nduwayezu R, Garcia C, Plum J, Brenner J, Song M, Ricotta E, Clifton D, Thwaites C.L, Bensoussan Y and Wood B (2025) Voice EHR: introducing multimodal audio data for health. Front. Digit. Health 6:1448351. doi: 10.3389/fdgth.2024.1448351
Received: 13 June 2024; Accepted: 26 December 2024;
Published: 28 January 2025.
Edited by:
Kun Qian, Beijing Institute of Technology, ChinaReviewed by:
Thomas Quatieri, Massachusetts Institute of Technology, United StatesCopyright: © 2025 Anibal, Huth, Li, Hazen, Daoud, Ebedes, Lam, Nguyen, Hong, Kleinman, Ost, Jackson, Sprabery, Elangovan, Krishnaiah, Akst, Lina, Elyazar, Ekawati, Jansen, Nduwayezu, Garcia, Plum, Brenner, Song, Ricotta, Clifton, Thwaites, Bensoussan and Wood. This is an open-access article distributed under the terms of the Creative Commons Attribution License (CC BY). The use, distribution or reproduction in other forums is permitted, provided the original author(s) and the copyright owner(s) are credited and that the original publication in this journal is cited, in accordance with accepted academic practice. No use, distribution or reproduction is permitted which does not comply with these terms.
*Correspondence: James Anibal, YW5pYmFsLmphbWVzQG5paC5nb3Y=
†Present Address: Richard Nduwayezu, Department of Primary Health Care, College of Medicine and Health Sciences, University of Rwanda, Kigali, Rwanda
‡These authors have contributed equally to this work
Disclaimer: All claims expressed in this article are solely those of the authors and do not necessarily represent those of their affiliated organizations, or those of the publisher, the editors and the reviewers. Any product that may be evaluated in this article or claim that may be made by its manufacturer is not guaranteed or endorsed by the publisher.
Research integrity at Frontiers
Learn more about the work of our research integrity team to safeguard the quality of each article we publish.