- 1Fowler School of Engineering, Chapman University, Orange, CA, United States
- 2School of Education, University of California, Riverside, CA, United States
- 3Department of Psychology, College of Sciences, San Diego State University, San Diego, CA, United States
- 4Department of Psychiatry and Neuroscience, University of California, Riverside, CA, United States
Introduction: In spite of rapid advances in evidence-based treatments for attention deficit hyperactivity disorder (ADHD), community access to rigorous gold-standard diagnostic assessments has lagged far behind due to barriers such as the costs and limited availability of comprehensive diagnostic evaluations. Digital assessment of attention and behavior has the potential to lead to scalable approaches that could be used to screen large numbers of children and/or increase access to high-quality, scalable diagnostic evaluations, especially if designed using user-centered participatory and ability-based frameworks. Current research on assessment has begun to take a user-centered approach by actively involving participants to ensure the development of assessments that meet the needs of users (e.g., clinicians, teachers, patients).
Methods: The objective of this mapping review was to identify and categorize digital mental health assessments designed to aid in the initial diagnosis of ADHD as well as ongoing monitoring of symptoms following diagnosis.
Results: Results suggested that the assessment tools currently described in the literature target both cognition and motor behaviors. These assessments were conducted using a variety of technological platforms, including telemedicine, wearables/sensors, the web, virtual reality, serious games, robots, and computer applications/software.
Discussion: Although it is evident that there is growing interest in the design of digital assessment tools, research involving tools with the potential for widespread deployment is still in the early stages of development. As these and other tools are developed and evaluated, it is critical that researchers engage patients and key stakeholders early in the design process.
1 Introduction
Attention Deficit Hyperactivity Disorder (ADHD) is the most widespread psychiatric condition among children, affecting approximately 11.4% of children aged 3–17 years old in the United States (1). The societal costs associated with ADHD were estimated at $19.4 billion among children ($6,799 per child) and $13.8 billion among adolescents ($8,349 per adolescent) in the United States (2).
1.1 Gold standard assessments for a diagnosis of ADHD
A gold-standard diagnostic assessment of ADHD involves a comprehensive evaluation of symptoms related to inattention, hyperactivity, and impulsiveness (3). Inattention includes difficulty with focusing and maintaining attention, poor organizational skills, and forgetfulness. Behaviors often considered reflective of hyperactivity include: (1) movement behaviors (e.g., fidgeting, leaving seats when staying seated is expected, constant motion, restlessness) and (2) communication behaviors (e.g., talking nonstop, blurting out answers, interrupting others). Although gold-standard evaluations typically involve data from multiple sources (children, clinicians, parents, teachers) and multiple methods (standardized rating scales, structured and semi-structured clinical interviews, neuropsychological tests), in most parts of the world, these types of evaluations can often be difficult to obtain, are costly, and are not widely available.
In clinical practice, a diagnosis of ADHD is provided after a series of behavioral observations, combined with neuropsychological assessments and the completion of behavior rating scales by the individuals’ parent, guardian, or another informant. Self-reports of internal feelings and challenges experienced by the patient are also collected. Those reports can be influenced by factors intrinsic to the children themselves or extrinsic roles such as parents, the medical system, or school (4).
Unfortunately, scores derived using self-report, parent-, or teacher- report rating scales can be influenced by several factors (4), including rater bias, differences in behaviors across settings, and the relationship between the rater and the child (5). The limitations of rating scales have led to concerns about the validity of diagnoses, such as the potential for over-diagnosis, while barriers to gold-standard evaluations have raised concerns about under-recognition of ADHD. Failure to recognize and treat ADHD early on may adversely affect academic achievement (6), family and social relationships (7), employment (8), and functioning in other domains (4). Hence, there is a need to both increase the rigor and availability of diagnostic tools as well as the tools that could be used to assess progress in response to a variety of interventions. Given these challenges with assessment of ADHD symptoms, there is growing interest in increasing the rigor of diagnostic procedures as well as the assessment of progress in response to interventions using digital tools.
1.2 Towards a user-centered approach to develop assessment digital tools for ADHD
Currently, standardized assessment tools could go far to bolster the accuracy of diagnosis and the acceptance by families and others of the clinical diagnosis procedure, in which technology offers an opportunity to support human professionals and experts in their diagnostic and assessment work. Rapid technological advances in the last few decades have introduced tremendous opportunities to support professionals and experts in their diagnostic and assessment work. Despite these advances, only a handful of technology-supported assessment tools are used widely in practice. For example, the Continuous Performance Test (9) is one of the few computerized tests of attention that clinicians consistently use in their assessment battery during a neuropsychological evaluation.
On the other hand, research on digital tools has explored three main approaches to support the assessment and diagnosis of individuals with ADHD (10): (1) classify data from brain activity, either EEG or fMRI [e.g., (11–20)], (2) classify data collected from sensors (on the body, in the environment, or inherent to computational tool use) used during everyday activities and then create computational models that can classify unseen data instances [e.g., (21–27)], and (3) design and employ serious games or environments where users can play and interact (the interactions of the users with the game are analyzed to infer if the user has ADHD or related symptoms) [e.g., (28, 29)]. While the first approach considers only the data from brain activity of ADHD individuals without their input; the second and third approaches involve end-users to some degree in certain stages of the development process for a given digital assessment tool.
Recently, there has been a tendency to use user-centered, participatory, and ability-based design (30–35) and similar types of frameworks to include the needs and consideration of the primary end users through the whole process of designing, developing and evaluating digital tools to assess symptoms and behaviors, including ADHD (36–38). In the case of ADHD diagnosis and assessment tools, there are two primary end users that should be considered: the clinicians (psychologist, psychiatrist, among others) who are conducting the assessment, and the individuals (patients) who are performing the activities requested by the clinicians. Therefore, research needs to find ways in which people with ADHD and experts might be empowered through technology and included in research teams to develop assessment tools.
Given the early stages of research in this area, our goal in this research was to conduct a mapping review of digital assessments with the potential to diagnose and measure ADHD symptoms. A mapping review has been defined as a “preliminary assessment of the potential size and scope of available research literature” that “aims to identify the nature and extent of research evidence,” including ongoing research (39). Scoping reviews typically do not include a formal quality assessment and typically provide tables of findings along with some narrative commentary. They are systematic and can provide preliminary evidence that indicates whether a full systematic review (with quality assessment) is warranted at a given time.
2 Methods
Due to the breadth of the topic and our aims, we utilized the mapping review approach described by the Evidence for Policy and Practice Information and Coordinating Centre (EPPI-Centre), Institute of Education, London (40) and summarized by Grant & Booth (39). This method of review aims to map and categorize published scientific journal articles and reports to provide an overview of a particular field that can aid in identifying gaps in the evidence and directions for future research. Mapping reviews typically do not include meta-analysis or formal systematic appraisal but may characterize the strength of the evidence-based on the study design or characteristics (39). Grant & Booth (39) noted that mapping reviews are particularly helpful in providing a systematic map that can help reviewers identify more narrowly focused review questions for future work and potential subsets of studies for future systematic reviews and meta-analyses.
2.1 Data sources and searches
Following the PRISMA process for systematic reviews (41), we searched PubMed, Web of Science, ACM Digital Library, and IEEE Xplore for articles published in English from January 1, 2004, to January 1, 2024. With an interdisciplinary approach, we conducted this search using both the world's largest medical research database (PubMed), a multidisciplinary database (Web of Science), and the two largest databases for computed sciences (ACM Digital Library and IEEE Xplore). The Association for Computing Machinery (ACM) is the largest educational and scientific computing society in the world. The IEEE, an acronym for the Institute of Electrical and Electronics Engineers, has grown beyond electrical engineering and is now the “world's largest technical professional organization dedicated to advancing technology for the benefit of humanity.” (42). Together, the ACM and IEEE digital libraries comprise the vast majority of computing and digital indexing of publications from the organizations’ journals and conferences. We also reviewed the references from the included papers to identify additional relevant studies.
Our search strategy and search items are summarized in Table 1. We limited results to peer-reviewed research papers, excluding abstracts and short papers. Manuscripts were organized and reviewed using Zotero (an open-source reference management software). Keywords from retrieved articles are shown in Table 1.
2.2 Study selection
Study selection criteria are summarized in Table 2. We included research articles focusing on digital assessment for children and adolescents and excluded research focused on digital health interventions only. We included assessments for participants of all ages. We included assessments aimed for use by clinical settings, researchers, and community settings (e.g., schools). Importantly, we included assessments across various stages of development. To only focus on papers with empirical evidence regarding the use, adoption, usefulness, and effectiveness of digital assessment grounded by empirical evidence, we excluded papers focused solely on the theoretical design of technological tools, if they included no prototype or testing in individuals with ADHD.
Two researchers reviewed abstracts and full papers and selected papers that both agreed met inclusion criteria. This process was completed two times to ensure accuracy. Further, inter-rater agreement was calculated on the basis of researchers’ categorization of articles using the previously mentioned inclusion and exclusion criteria (see Table 1). Researchers randomly selected 20% of the papers at the abstract review stage and coded the abstracts according to inclusion criteria. The agreement among researchers’ decision to include the article for these 20% of randomly selected articles was calculated to be greater than 80% (0.8125).
2.3 Evaluation of the stage of development of the assessment
This literature review includes research from diverse fields mainly including a clinical approach and a Computer Science/Human-Computer Interaction (HCI) approach. Therefore, literature on these fields follows different research lifecycles when developing technology in general and for assessment in particular (43). Typically, HCI researchers explore how emerging and commercially available technology can be designed and developed to support digital assessments using a user-centered approach and then conduct pilot feasibility studies to prove that the technology can be used in this context. On the other hand, clinicians validate digital assessments that follow a well-known or evidence-based theory (43) when conducting pilot testing and randomized control trials to validate the assessment. Thus, in this work, we proposed two categories to classify the digital assessment depending on the stage of development: (1) validated or (2) exploratory. The types of assessments that tended to fall in the ‘validated’ category for this sample of articles were computerized assessments commonly used by clinicians (e.g., Continuous Performance Test) that were adapted to develop a digital version. Alternately, the type of assessments that tended to fall in the ‘exploratory’ category were pilot studies, studies with few participants, and studies that were at the early stages of data collection, because these types of studies tend to involve the patients in early stages of the design of the tools as well as clinicians.
3 Results
After applying the PRISMA (41) process for identifying appropriate articles for inclusion, our results are summarized in Figure 1. After duplicates were removed using Zotero, there were 808 records. Among those 808 records screened, 712 articles were excluded using the previously provided exclusion criteria. Then, 96 full-text articles were assessed for eligibility. Of those 96 full-text articles, 39 papers were excluded because they did not target assessment or diagnosis of ADHD, they did not present an evaluation of ADHD users, or they were focused on the use of machine learning to improve questionnaires [i.e., utilizing machine learning to eliminate non-significant variables from psychometric questionnaires for ADHD diagnosis, aiming to reduce the administration time of these assessments (44, 45)]. Ultimately, 57 papers were included in this mapping review (see Figure 1).
3.1 Targeted domains of cognitive and behavioral functioning
The domains of cognitive and behavioral functioning assessed by the tools in the included studies were grouped into four categories: cognition/attention, social/emotional skills, behavior management/self-regulation, or motor behaviors/physical activity (Figure 2-left).
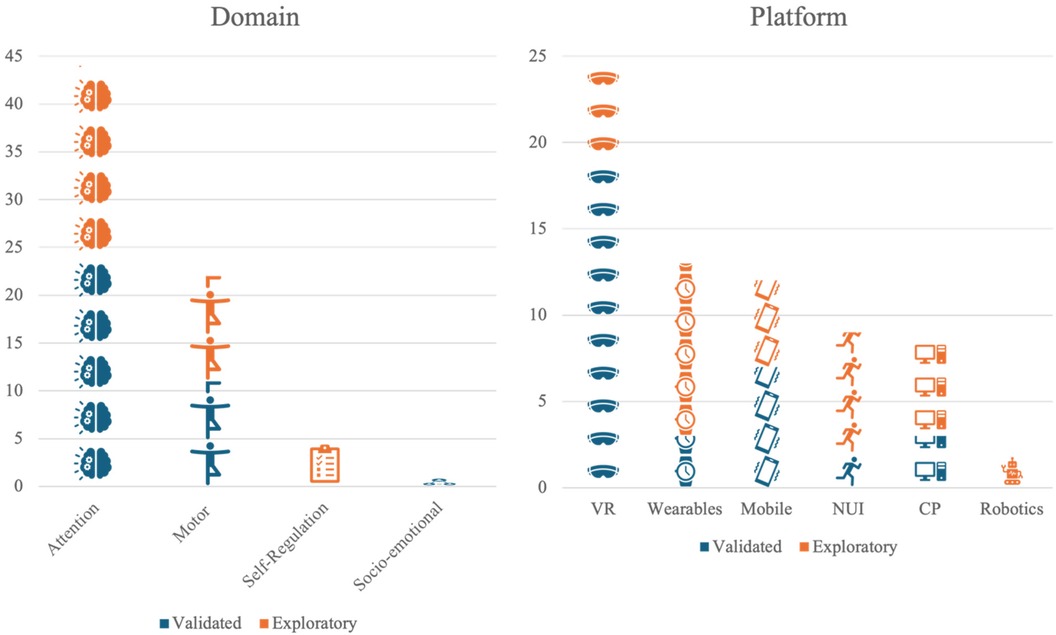
Figure 2. A pictograph showing the distribution of paper by domain (left) and by platforms (right). VR, virtual reality; NUI, natural user interfaces; CP, personal computer and web.
Most papers (86%) described tools designed to assess attention and other aspects of cognition. Two main projects have been widely explored, the Virtual Reality Classroom, then called ClinicalVR (46–50), and AULA (51–53), which demonstrate the potential of VR in assessing attention. Even though VR has become more accessible, less expensive, less heavy, and more tolerable (e.g., it creates less motion sickness), it is still not particularly intuitive for many people. It may be totally out of reach for people with sensory challenges, including children with neurodevelopmental disorders like ADHD, but it provides a controlled environment to conduct assessments.
The second most common domain was motor behaviors or physical activity, with 43% of papers describing tools to assess behaviors in this domain. One approach is to use an indirect sensing device, such as depth cameras (e.g., Kinect), to track the movements. For example, Sempere-Tortosa and colleagues (2020) developed ADHD Movements, a computer software that uses the Microsoft Kinect V.2 device to capture 17 joints and evaluate the movements. A study with 6 subjects in a teaching/learning situation showed that there were significant differences in the movements between the ADHD and control group (54).
On the other hand, Muñoz-Organero and colleagues (2018) tested direct sensing (e.g., wearables) using accelerometers on the dominant wrist and non-dominant ankle of 22 children (11 with ADHD, 6 of whom were also medicated) during school hours. They used deep learning algorithms [e.g., Convolutional Neural Network (CNN)] to recognize the movement differences between nonmedicated ADHD children and their paired controls. There were statistically significant differences in the way children with ADHD and those without moved for the wrist accelerometer, but only between nonmedicated children with ADHD and children without ADHD for the ankle accelerometer.
None of the papers described tools designed to measure social/emotional functioning, and only one paper described a tool designed to measure behavior management or self-regulation (28). Their research group developed a Contextualized and Objective System (COSA) to support ADHD diagnosis by measuring symptoms of inattention, hyperactivity, and impulsivity. Impulsivity is often used as a proxy for measuring self-regulation using performance-based tasks, or Serious Games. The Serious Games developed for COSA were informed by existing auxiliary diagnostic performance-based tasks of inhibition and impulsivity, including CPT, Go/No-Go, and the Matching Familiar Figures Test. The COSA instruments were used to measure inhibition and impulsivity (e.g., stop yourself from eating “eCandy”).
A hybrid approach, meaning the measurement of multiple symptoms, using multiple digital tools (e.g., wearable sensor, intelligent hardware, paired with mobile application), has also been explored to create a system to support the assessment of multiple domains of ADHD (e.g., attention and hyperactivity). An initial pilot study to investigate children's attentional control in a VR classroom was combined with instruments to detect “head turning” and gross motor movements. These instruments included motoric tracking devices on the VR head-mounted display and wearable hand and leg tracking systems (47). Combining the use of VR, Serious Games, and motor behaviors allowed Parsons and colleagues (2007) to predict the body movements a hyperactive child may be engaging in the classroom. Similarly, the WEDA system, tested with 160 children ages 7 to 12, half with ADHD, attempted to discriminate between challenges in inattention from those related to hyperactivity and impulsivity, finding that the tasks cover all symptoms but perform better related to inattention (29). Overall, the summarized works suggest that it is possible to assess several ADHD-related behaviors using a multimodal technology approach. However, it is unclear how we can refine the current assessment tools to collect data augmented with contextual, or real-life, information.
3.2 Technology platforms applied to ADHD assessment
Among the included studies, the technology platforms used in the assessment process were varied: virtual or augmented reality (49%), natural user interfaces (17%), personal computers (17%), mobile devices or tablets (23%), sensor/wearables/EEG (25%), and robotics (3%) (Figure 2-right). Virtual and augmented reality is a rapidly shifting label in the literature, but for the purpose of this review, studies assigned to that category included fully immersive virtual reality as well as mixed-reality and augmented reality approaches. This category included virtual worlds and immersive video games. Natural user interfaces included the use of input devices beyond traditional mice and keyboards, such as pens, gestures, speech, eye tracking, and multi-touch interaction. The personal computers category included applications that require a traditional keyboard, mouse/touchpad, and monitor. Mobile devices and tablets can access such applications, as well, but this category was reserved for so-called “mobile first” applications, focused on an intentional design towards mobility. Sensors and wearables include the use of automated sensing technologies, such as accelerometers, heart rate sensors, microphones, and brain-computer interfaces, both in the environment and on the body. Robotics, a similarly broad and dynamic category, included physical instantiations of digital interactions, such as both humanoid or anthropomorphic robots and general digital devices that carry out physical tasks. This grouping included autonomous robots and those operated remotely by humans.
3.3 Stage of development
Results from the literature review revealed that 27 of the projects that met inclusion criteria were considered “validated assessments”. The projects with validated assessment studies tended to adopt widely accepted assessments, such as the Continuous Performance Test (55), and implemented the assessment within a novel digital environment, such as via virtual reality (see Table 3). For example (51, 58, 75), all administered the CPT within a virtual reality classroom environment. In some cases, these widely used assessments seemed to inspire ideas for the measurement of symptoms of ADHD (e.g., attentional control, inhibition, reaction time) in a gamified virtual environment (e.g., the Nesplora Aquarium test) (52). Other studies in this stage of development administered widely used assessments via telemedicine. For example, Sabb and colleagues (2013) administered the Stroop task (76) via a web-based platform typically used to meet patients virtually.
On the other hand, 26 of the projects were considered “exploratory assessments” as they were either pilot studies, studies with small sample sizes, or the research team was in the early stages of data collection, and the primary goal was to launch the assessment tool rather than collect usable data. The studies aimed at refining exploratory assessments using novel technological platforms or a combination of the following platforms, including (a) personal computers and the internet (24%), (b) mobile devices or tablets (20%), (c) sensors, wearables, or EEG (40%), virtual reality (24%), robotics (8%), and a natural user interface (28%) (see Table 4). These types of technologies were created by researchers to better meet the needs of participants (patients and clinicians), as current commercial devices may not provide the sensors and feedback needed to conduct in-depth assessments of ADHD symptoms in accordance with current American Psychological Association diagnostic criteria [DSM−5; (3)]. However, the approach of exploratory research is first to develop the technology and provide evidence that it is feasible for application, once feasibility is established, the primary objective of researchers is to test the usability of the assessment data collected for either patients or clinicians. For example, Son and colleagues (2021) are in the early stages of developing an ‘objective diagnosis of ADHD by analyzing a quantified representation of the action of potential patients in multiple natural environments’. The research team applies the diagnostic criteria for ADHD listed in the DSM-5 (3) to virtual reality and artificial intelligence applications in order to build an AI model which will classify the potential patient as having either ADHD inattentive type, hyperactive-impulsive type, combined type, or no diagnosis. Future steps for this research may include comparing the decisions of the AI model to those of a clinician in a clinical trial.
Overall, it is ideal to combine both approaches. To better conceptualize this goal, it is useful to position these approaches on a continuum of digital health technological tool development where stages of development from exploratory (early stages often piloted by human-computer interaction researchers) to validated (late stages such as clinical trials led by clinicians) lie. Thus, researchers in the field should strive to recruit multidisciplinary teams that are capable of implementing methodologies that combine both approaches over the course of a tool's developmental lifetime. When made, these proposed changes will accelerate the validation and widespread use of diagnostic digital health technologies for ADHD.
4 Discussion
Recent estimates suggest that nearly 11% of children and adolescents in the United States experience ADHD symptoms (1). Thus, there is a significant need to broaden access to evidence-based treatments to support individuals with ADHD. In this paper, we argue that digital health assessments have the potential for widespread impact on the assessment infrastructure necessary to connect individuals with ADHD to the necessary treatments designed to support them. This scoping review addresses a critical gap in the literature and illustrates the growing international interest in digital health assessment for ADHD. Many of the excluded papers in our search described novel digital health assessment tools that were not sufficiently developed or have yet to be evaluated. This suggests that this field of research will continue to grow rapidly and, therefore, intentional investment in translation from early designs for digital assessment tools to robust products as well as from pilot studies to larger scale clinical trials are necessary next steps to meet the needs of the field.
4.1 Participant engagement and user-centered assessment
Involving the final users in the development of the assessment is a crucial step in trying to create unbiased digital tools. Therefore, different points of view should be held up to the light. Traditionally, clinicians are charged with developing the assessment tools for ADHD, and subsequently, researchers in the technological fields “translated” those tools into digital health assessments. The advantage of this approach is that the tools tend to be more widely “accepted” by other clinicians as they use “validated” assessments to evaluate symptoms and behaviors without input from the patients who are responsible for conducting the activities requested by the clinicians.
On the other hand, studies have reported conducting interviews with one (29) or more (78) clinicians to incorporate their perspectives before building the tools. Additionally, these studies consider or evaluate patient and caregiver satisfaction (99) prior to deploying the actual tool, showing an initial commitment to following a user-centric approach instead of translating current theories into digital interventions [e.g., (53, 62)]. Unfortunately, developing quality assessment tools is time-consuming and starts from co-design sessions before developing low and high-fidelity prototypes. The first evaluation of those prototypes targets the tools’ feasibility and usability before developing the final tools that can be then “validated.” While this approach is highly recommended, the approach neglects to answer important research questions that have yet to be answered, including how to engage ADHD participants in the co-design sessions, how to balance their needs with the clinicians’ needs, how the collected data can be used fairly, and what should need to be done to transform those prototypes into valid assessment.
4.2 Clinical implications
The underdiagnosis of ADHD results in patients not receiving treatment, which poses psychological, financial, academic, and social burden to the patient and their community (101). Further, failure to diagnose ADHD prevents children and their families from getting the assistance necessary to achieve their full potential in academic, workplace, and psychosocial settings (102). A lack of diagnosis can lead to a lack of treatment and restricted access to accommodations that will have a cascade of consequences for an individual's academic achievement (6), family and social relationships (7), employment (8), and other critical components of life success (4).
The clinical implications for the development of diagnostic digital health technologies to diagnose ADHD are vast and varied. To support access to digital assessment tools researchers will need to adopt rigorous approaches to ensure the development of reliable and feasible tools designed to be used by clinicians who seek to evaluate ADHD symptoms and diagnose ADHD. Innovative computational approaches paired with expert human decision-making have the potential to improve the quality of assessments while decreasing their costs. Thus, novel technologies can support clinicians through the collection of data from multiple modes of assessment that support the decision-making of the experts and improve the accuracy of diagnosis.
4.3 Future research directions
Only a handful of studies collected from this scoping review examined products that were designed using user-centered participatory and ability-based design methods. User-centered, participatory and ability-based design frameworks demand two parallel approaches: inclusion of the needs and consideration of the primary end users of these technologies early in the design process and consideration for the ways in which people with ADHD might be empowered through technology and included in research teams. In our own work, we strive to include children and adolescents with ADHD on our design teams, engaging them in creating their own inventions as well as commenting on and critiquing ours (103–108). Although these approaches are time consuming and can be more challenging to implement, the long-term adoption and ultimate success of digital health tools requires the input and perspectives of those who experience the conditions as well as other relevant stakeholders.
In terms of hyperactivity, research has shown that measuring and predicting movement-related behaviors using data gathered from wearables and cameras to assess ADHD is feasible and helps to understand more about the role of hyperactivity in motor performance. However, the assessment should also consider the core features of ADHD related to attention, socioemotional functioning, and self-regulation. Therefore, a multimodal approach should be adopted.
A possible reason for the lack of tools designed to measure social/emotional functioning and self-regulation is the challenge of eliciting the real-life emotions involved in behavioral management and self-regulation, especially with respect to the demands placed on children with neurodevelopmental disorders in schools and at home. Take for example a child who is being bullied by peers at school or a child who has difficulty reading and, therefore, cannot access the academic material and becomes frustrated in a classroom. These are challenges children with ADHD are faced with daily and it is possible Serious Games have not yet been developed to tap into these charged and challenging socioemotional and behavioral contexts.
4.4 Study limitations
There are several limitations to consider when reading this mapping review. First, we limited our review to papers published in English language journals and to PubMed, IEEE, and ACM databases. Although these databases contain the largest collections of research in the field and can be considered comprehensive for scholarly publications in English, limiting the search to articles published in English and to articles available through these databases has inherent limitations. For example, it excludes grey literature, which includes white papers that are not peer-reviewed but that can be common surrounding consumer products. We excluded papers that developed diagnostic assessments for multiple diagnostic groups other than ADHD (e.g., for individuals with Autism who also exhibited symptoms of ADHD, children who demonstrate self-regulation difficulties). We also excluded papers for which the focus was on digital health intervention and treatment, rather than assessment. Finally, the broad range of terms used in this space makes a true comprehensive review incredibly difficult. Keyword selection, terminology usage, and digital libraries in the mHealth space are not consistent within disciplines, across disciplines, nor across countries. Despite these limitations, our work provides a map of the current scientific work in this space that can aide clinical and computing scientists in identifying gaps and potential targets for future work.
5 Conclusion
Recently, there has been rapid growth in collaboration between the fields of computing and clinical sciences. Given the explosion in telehealth and telemedicine since the beginning of the COVID-19 pandemic, this growth underscores the need for empirically and well-developed technological diagnostic tools. This mapping review highlights current work on the development of diagnostic tools used to assess symptoms of ADHD, providing examples of how emerging technologies can enhance diagnostic processes for both researchers and clinicians.
The included studies show that while some diagnostic technologies seem promising, there are still opportunities that should be addressed to widespread clinical use. Specifically, future work should focus on:
1. User-Centered Design: Emphasizing user-centered design strategies to tailor diagnostic tools to the needs and experiences of clinicians, patients, and caregivers, thereby improving acceptability and usability.
2. Interdisciplinary Collaboration: Fostering multidisciplinary collaboration between computer science, HCI researchers, clinicians, and other stakeholders to bridge gaps in knowledge and practice, ensuring that technological advancements are clinically relevant and evidence-based.
3. Integration with Clinical Workflow: Developing strategies to seamlessly integrate diagnostic technologies into existing clinical workflows, ensuring they complement rather than disrupt standard practices.
4. Rigorous Validation: Conducting comprehensive validation studies to ensure the accuracy, reliability, and effectiveness of diagnostic technologies in diverse clinical settings.
Data availability statement
The original contributions presented in the study are included in the article/Supplementary Material, further inquiries can be directed to the corresponding author.
Author contributions
FC: Conceptualization, Data curation, Formal Analysis, Funding acquisition, Investigation, Methodology, Project administration, Resources, Validation, Visualization, Writing – original draft, Writing – review & editing. EM: Conceptualization, Data curation, Formal Analysis, Investigation, Methodology, Validation, Visualization, Writing – original draft, Writing – review & editing. KL: Conceptualization, Data curation, Formal Analysis, Funding acquisition, Investigation, Methodology, Project administration, Resources, Supervision, Validation, Visualization, Writing – original draft, Writing – review & editing.
Funding
The author(s) declare financial support was received for the research, authorship, and/or publication of this article. This work was partially supported by AHRQ under award number R21HS028871 by the National Science Foundation under award 2245495.
Acknowledgments
We thank Gillian Hayes and Sabrina Schuck for their support and feedback.
Conflict of interest
The authors declare that the research was conducted in the absence of any commercial or financial relationships that could be construed as a potential conflict of interest.
Publisher's note
All claims expressed in this article are solely those of the authors and do not necessarily represent those of their affiliated organizations, or those of the publisher, the editors and the reviewers. Any product that may be evaluated in this article, or claim that may be made by its manufacturer, is not guaranteed or endorsed by the publisher.
References
1. Danielson ML, Claussen AH, Bitsko RH, Katz SM, Newsome K, Blumberg SJ, et al. ADHD Prevalence among US children and adolescents in 2022: diagnosis, severity, co-occurring disorders, and treatment. J Clin Child Adolesc Psychol. (2024) 53(3):343–60. doi: 10.1080/15374416.2024.2335625
2. Schein J, Adler LA, Childress A, Cloutier M, Gagnon-Sanschagrin P, Davidson M, et al. Economic burden of attention-deficit/hyperactivity disorder among children and adolescents in the United States: a societal perspective. J Med Econ. (2022) 25(1):193–205. doi: 10.1176/appi.books.9780890425787
3. American Psychiatric Association. Diagnostic and Statistical Manual of Mental Disorders (5th ed., Text Revision). Washington, DC: American Psychiatric Publishing (2023). doi: 10.1176/appi.books.9780890425596.744053
4. Hamed AM, Kauer AJ, Stevens HE. Why the diagnosis of attention deficit hyperactivity disorder matters. Front Psychiatry. (2015) 6(NOV):1–10. doi: 10.3389/fpsyt.2015.00168
5. Lakes KD, Hoyt WT. Applications of generalizability theory to clinical child and adolescent psychology research? J Clin Child Adolesc Psychol. (2009) 38(1):144–65. doi: 10.1080/15374410802575461
6. Powers RL, Marks DJ, Miller CJ, Newcorn JH, Halperin JM. Stimulant treatment in children with attention-deficit/hyperactivity disorder moderates adolescent academic outcome. J Child Adolesc Psychopharmacol. (2008) 18(5):449–59. doi: 10.1089/cap.2008.021
7. Taylor E, Chadwick O, Heptinstall E, Danckaerts M. Hyperactivity and conduct problems as risk factors for adolescent development. J Am Acad Child Adolesc Psychiatry. (1996) 35(9):1213–26. doi: 10.1097/00004583-199609000-00019
8. De Graaf R, Kessler RC, Fayyad J, Ten Have M, Alonso J, Angermeyer M, et al. The prevalence and effects of adult attention-deficit/hyperactivity disorder (ADHD) on the performance of workers: results from the WHO world mental health survey initiative. Occup Environ Med. (2008) 65(12):835–42. doi: 10.1136/oem.2007.038448
9. Conners CK, Epstein JN, Angold A, Klaric J. Continuous performance test performance in a normative epidemiological sample. J Abnorm Child Psychol. (2003) 31:557–64. doi: 10.1023/A:1025457300409
10. Cibrian FL, Lakes KD, Tavakoulnia A, Guzman K, Schuck S, Hayes GR. Supporting self-regulation of children with ADHD using wearables. CHI Conference on Human Factors in Computing Systems (2020). p. 1–13. doi: 10.1145/3313831.3376837
11. Sachnev V. An efficient classification scheme for ADHD problem based on binary coded genetic algorithm and McFIS. in Proceedings - 2015 International Conference on Cognitive Computing and Information Processing, CCIP 2015 (2015). p. 1–6. doi: 10.1109/CCIP.2015.7100690
12. Ghiassian S, Greiner R, Jin P, Brown MRG. Using functional or structural magnetic resonance images and personal characteristic data to identify ADHD and autism. PLoS One. (2016) 11(12):e0166934. doi: 10.1371/journal.pone.0166934
13. Tan L, Guo X, Ren S, Epstein JN, Lu LJ. A computational model for the automatic diagnosis of attention deficit hyperactivity disorder based on functional brain volume. Front Comput Neurosci. (2017) 11. doi: 10.3389/fncom.2017.00075
14. Zou L, Zheng J, Miao C, McKeown MJ, Wang ZJ. 3D CNN based automatic diagnosis of attention deficit hyperactivity disorder using functional and structural MRI. IEEE Access. (2017) 5:23626–36. doi: 10.1109/ACCESS.2017.2762703
15. Eslami T, Saeed F. Similarity based classification of ADHD using singular value decomposition. CF '18: Proceedings of the 15th ACM International Conference on Computing Frontiers (2018). p. 19–25. doi: 10.1145/3203217.3203239
16. Riaz A, Asad M, Alonso E, Slabaugh G. Fusion of fMRI and non-imaging data for ADHD classification. Comput Med Imaging Graph. (2018) 65:115–28. doi: 10.1016/j.compmedimag.2017.10.002
17. Sen B, Borle NC, Greiner R, Brown MRG. A general prediction model for the detection of ADHD and autism using structural and functional MRI. PLoS One. (2018) 13(4):e0194856. doi: 10.1371/journal.pone.0194856
18. Miao B, Zhang LL, Guan JL, Meng QF, Zhang YL. Classification of ADHD individuals and neurotypicals using reliable RELIEF: a resting-state study. IEEE Access. (2019) 7:62163–71. doi: 10.1109/ACCESS.2019.2915988
19. Wang Z, Sun Y, Shen Q, Cao L. Dilated 3d convolutional neural networks for brain mri data classification. IEEE Access. (2019) 7:134388–98. doi: 10.1109/ACCESS.2019.2941912
20. Ariyarathne G, De Silva S, Dayarathna S, Meedeniya D, Jayarathne S. ADHD Identification using convolutional neural network with seed-based approach for fMRI data. ACM Int Conf Proceeding Ser. (2020) 2020:31–5. doi: 10.1145/3384544.3384552
21. Lis S, Baer N, Stein-En-Nosse C, Gallhofer B, Sammer G, Kirsch P. Objective measurement of motor activity during cognitive performance in adults with attention-deficit/hyperactivity disorder. Acta Psychiatr Scand. (2010) 122:285–94. doi: 10.1111/j.1600-0447.2010.01549.x
22. O'Mahony N, Florentino-Liano B, Carballo JJ, Baca-García E, Rodríguez AA. Objective diagnosis of ADHD using IMUs. Med Eng Phys. (2014) 36:922–6. doi: 10.1016/j.medengphy.2014.02.023
23. Kaneko M, Yamashita Y, Iramina K. Quantitative evaluation system of soft neurological signs for children with attention deficit hyperactivity disorder. Sensors (Basel). (2016) 16(1):116. doi: 10.3390/s16010116
24. Mock P, Tibus M, Ehlis AC, Baayen H, Gerjets P. Predicting ADHD risk from touch interaction data. ICMI 2018 - Proceedings of the 2018 International Conference on Multimodal Interaction (2018). p. 446–54. doi: 10.1145/3242969.3242986
25. Muñoz-Organero M, Powell L, Heller B, Harpin V, Parker J. Automatic extraction and detection of characteristic movement patterns in children with ADHD based on a convolutional neural network (CNN) and acceleration images. Sensors (Basel). (2018) 18(11):3924. doi: 10.3390/s18113924
26. Farran EK, Bowler A, Karmiloff-Smith A, D’Souza H, Mayall L, Hill EL. Cross-domain associations between motor ability, independent exploration, and large-scale spatial navigation; attention deficit hyperactivity disorder, williams syndrome, and typical development. Front Hum Neurosci. (2019) 13:225. doi: 10.3389/fnhum.2019.00225
27. Ricci M, Terribili M, Giannini F, Errico V, Pallotti A, Galasso C, et al. Wearable-based electronics to objectively support diagnosis of motor impairments in school-aged children. J Biomech. (2019) 83:243–52. doi: 10.1016/j.jbiomech.2018.12.005
28. Chen OT-C, Chen P, Tsai Y. Attention estimation system via smart glasses. 2017 IEEE Conference on Computational Intelligence in Bioinformatics and Computational Biology (CIBCB) (2017). p. 1–5. doi: 10.1109/CIBCB.2017.8058565
29. Jiang X, Chen Y, Huang W, Zhang T, Gao C, Xing Y, et al.. WeDA: designing and evaluating A scale-driven wearable diagnostic assessment system for children with ADHD. CHI '20: Proceedings of the 2020 CHI Conference on Human Factors in Computing Systems (2020). p. 1–12. doi: 10.1145/3313831.3376374
30. Wobbrock JO, Kane SK, Gajos KZ, Harada S, Froehlich J. Ability-based design: concept, principles and examples. ACM Trans Access Comput. (2011) 3(3):1–27. doi: 10.1145/1952383.1952384
31. Still B, Crane K. Fundamentals of User-Centered Design: A Practical Approach. Boca Ration, FL: CRC press (2017).
32. Bennett CL, Brady E, Branham SM. Interdependence as a frame for assistive technology research and design. In Proceedings of the 20th International acm Sigaccess Conference on Computers and Accessibility (2018). p. 161–73
33. Spiel K, Frauenberger C, Keyes O, Fitzpatrick G. Agency of autistic children in technology research-A critical literature review. ACM Trans Comput Hum Interact. (2019) 26(6):1–40. doi: 10.1145/3344919
34. Wobbrock JO, Gajos KZ, Kane SK, Vanderheiden GC. Ability-based design. Commun ACM. (2018) 61(6):62–71. doi: 10.1145/3148051
35. Wilson C, Brereton M, Ploderer B, Sitbon L. Co-Design beyond words: ‘moments of interaction'with minimally-verbal children on the autism Spectrum. In Proceedings of the 2019 CHI Conference on Human Factors in Computing Systems (2019). p. 1–15
36. Spiel K, Hornecker E, Williams RM, Good J. ADHD And technology research-investigated by neurodivergent readers. In Proceedings of the 2022 CHI Conference on Human Factors in Computing Systems (2022). p. 1–21
37. Cibrian F, Hayes G, Lakes K. Research advances in ADHD and technology. Syn Lect Assist Rehabil Health-Pres Techn. (2020) 9(3):i–156. doi: 10.2200/S01061ED1V01Y202011ARH015
38. Stefanidi E, Schöning J, Rogers Y, Niess J. Children with ADHD and their care ecosystem: designing beyond symptoms. In Proceedings of the 2023 CHI Conference on Human Factors in Computing Systems (2023). p. 1–17
39. Grant MJ, Booth A. A typology of reviews: an analysis of 14 review types and associated methodologies. Health Info Libr J. (2009) 26:91–108. doi: 10.1111/j.1471-1842.2009.00848.x
40. Bates S, Clapton J, Coren E. Systematic maps to support the evidence base in social care. Evid Pol. (2007) 3(4):539–51. doi: 10.1332/174426407782516484
41. PRISMA Group, Moher D, Liberati A, Tetzlaff J, Altman DG. Preferred reporting items for systematic reviews and meta-analyses: the PRISMA statement. Ann Intern Med. (2009) 151(4):264–9. doi: 10.7326/0003-4819-151-4-200908180-00135
42. IEEE. IEEE mission & visión. (2020). Available online at: https://www.ieee.org/about/vision-mission.html
43. Cibrian FL, Monteiro E, Schuck SE, Nelson M, Hayes GR, Lakes KD. Interdisciplinary tensions when developing digital interventions supporting individuals with ADHD. Front Digit Health. (2022) 4:876039. doi: 10.3389/fdgth.2022.876039
44. Duda M, Haber N, Daniels J, Wall DP. Crowdsourced validation of a machine-learning classification system for autism and ADHD. Transl Psychiatry. (2017) 7(5):e1133. doi: 10.1038/tp.2017.86
45. Caselles-Pina L, Quesada-López A, Sújar A, Garzón Hernández EM, Delgado-Gómez D. A systematic review on the application of machine learning models in psychometric questionnaires for the diagnosis of attention deficit hyperactivity disorder. Eur J Neurosci. (2024) 60(3):4115–27. doi: 10.1111/ejn.16288
46. Coleman B, Marion S, Rizzo A, Turnbull J, Nolty A. Virtual reality assessment of classroom-related attention: an ecologically relevant approach to evaluating the effectiveness of working memory training. Front Psychol. (2019) 10:1851. doi: 10.3389/fpsyg.2019.01851
47. Parsons TD, Bowerly T, Buckwalter JG, Rizzo AA. A controlled clinical comparison of attention performance in children with ADHD in a virtual reality classroom compared to standard neuropsychological methods. Child Neuropsychol. (2007) 13(4):363–81. doi: 10.1080/13825580600943473
48. Pollak Y, Weiss PL, Rizzo AA, Weizer M, Shriki L, Shalev RS, et al. The utility of a continuous performance test embedded in virtual reality in measuring ADHD-related deficits. J Dev Behav Pediatr. (2009) 30(1):2–6. doi: 10.1097/DBP.0b013e3181969b22
49. Rizzo AA, Buckwalter JG, Neumann U. Virtual reality and cognitive rehabilitation: a brief review of the future. J Head Trauma Rehabil. (1997) 12(6):1–15. doi: 10.1097/00001199-199712000-00002
50. Yeh S, Tsai C, Fan Y, Liu P, Rizzo A. An innovative ADHD assessment system using virtual reality. 2012 IEEE-EMBS Conference on Biomedical Engineering and Sciences (2012). p. 78–83. doi: 10.1109/IECBES.2012.6498026
51. Areces D, Cueli M, Garcia T, Gonzalez-Castro P, Rodriguez C. Using brain activation (nir-HEG/Q-EEG) and execution measures (CPTs) in a ADHD assessment protocol. J Visualized Exp. (2018) 134:56796. doi: 10.3791/56796
52. Camacho-Conde JA, Climent G. Attentional profile of adolescents with ADHD in virtual-reality dual execution tasks: a pilot study. Appl Neuropsychol Child. (2022) 11(1):81–90. doi: 10.1080/21622965.2020.1760103
53. Díaz-Orueta U, Garcia-López C, Crespo-Eguílaz N, Sánchez-Carpintero R, Climent G, Narbona J. AULA Virtual reality test as an attention measure: convergent validity with conners’ continuous performance test. Child Neuropsychol. (2014) 20(3):328–42. doi: 10.1080/09297049.2013.792332
54. Sempere-Tortosa M, Fernandez-Carrasco F, Mora-Lizan F, Rizo-Maestre C. Objective analysis of movement in subjects with ADHD. Multidisciplinary control tool for students in the classroom. Int J Environ Res Public Health. (2020) 17(15):5620. doi: 10.3390/ijerph17155620
55. Conner ML. Attention Deficit Disorder in Children and Adults: Strategies for Experiential Educators (1994).
56. Adamou M, Jones SL, Fullen T, Galab N, Abbott K, Yasmeen S. Remote assessment in adults with autism or ADHD: a service user satisfaction survey. PLoS One. (2021) 16(3):e0249237. doi: 10.1371/journal.pone.0249237
57. Adams R, Finn P, Moes E, Flannery K, Rizzo AS. Distractibility in attention/deficit/hyperactivity disorder (ADHD): the virtual reality classroom. Child Neuropsychol. (2009) 15(2):120–35. doi: 10.1080/09297040802169077
58. Areces D, Rodríguez C, García T, Cueli M. Is an ADHD observation-scale based on DSM criteria able to predict performance in a virtual reality continuous performance test? Appl Sci. (2020) 10(7):2409. doi: 10.3390/app10072409
59. Eom H, Kim KK, Lee S, Hong Y-J, Heo J, Kim J-J, et al. Development of virtual reality continuous performance test utilizing social cues for children and adolescents with attention-deficit/hyperactivity disorder. Cyberpsychol Behav Soc Netw. (2019) 22(3):198–204. doi: 10.1089/cyber.2018.0377
60. Gutierrez-Maldonado J, Letosa-Porta A, Rus-Calafell M, Penaloza-Salazar C. The assessment of attention deficit hyperactivity disorder in children using continous performance tasks in virtual environments. Anuario de Psicologia. (2009) 40(2):211–22. http://hdl.handle.net/2445/98279
61. Hyun GJ, Park JW, Kim JH, Min KJ, Lee YS, Kim SM, et al. Visuospatial working memory assessment using a digital tablet in adolescents with attention deficit hyperactivity disorder. Comput Methods Programs Biomed. (2018) 157:137–43. doi: 10.1016/j.cmpb.2018.01.022
62. Iriarte Y, Diaz-Orueta U, Cueto E, Irazustabarrena P, Banterla F, Climent G. AULA-advanced virtual reality tool for the assessment of attention: normative study in Spain. J Atten Disord. (2016) 20(6):542–68. doi: 10.1177/1087054712465335
63. Johnson KA, Dáibhis A, Tobin CT, Acheson R, Watchorn A, Mulligan A, et al. Right-sided spatial difficulties in ADHD demonstrated in continuous movement control. Neuropsychologia. (2010) 48(5):1255–64. doi: 10.1016/j.neuropsychologia.2009.12.026
64. Lalonde G, Henry M, Drouin-Germain A, Nolin P, Beauchamp MH. Assessment of executive function in adolescence: a comparison of traditional and virtual reality tools. J Neurosci Methods. (2013) 219(1):76–82. doi: 10.1016/j.jneumeth.2013.07.005
65. Leitner Y, Doniger GM, Barak R, Simon ES, Hausdorff JM. A novel multidomain computerized cognitive assessment for attention-deficit hyperactivity disorder: evidence for widespread and circumscribed cognitive deficits. J Child Neurol. (2007) 22(3):264–76. doi: 10.1177/0883073807299859
66. Loskutova NY, Lutgen CB, Callen EF, Filippi MK, Robertson EA. Evaluating a web-based adult ADHD toolkit for primary care clinicians. J Am Board Fam Med. (2021a) 34(4):741–52. doi: 10.3122/jabfm.2021.04.200606
67. Loskutova NY, Callen E, Pinckney RG, Staton EW, Pace WD. Feasibility, implementation and outcomes of tablet-based two-step screening for adult ADHD in primary care practice. J Atten Disord. (2021b) 25(6):794–802. doi: 10.1177/1087054719841133
68. Mangalmurti A, Kistler WD, Quarrie B, Sharp W, Persky S, Shaw P. Using virtual reality to define the mechanisms linking symptoms with cognitive deficits in attention deficit hyperactivity disorder. Sci Rep. (2020) 10(1):529. doi: 10.1038/s41598-019-56936-4
69. Muhlberger A, Jekel K, Probst T, Schecklmann M, Conzelmann A, Andreatta M, et al. The influence of methylphenidate on hyperactivity and attention deficits in children with ADHD: a virtual classroom test. J Atten Disord. (2020) 24(2, SI):277–89. doi: 10.1177/1087054716647480
70. Mwamba HM, Fourie PR, van den Heever D. PANDAS: paediatric attention-deficit/hyperactivity disorder application software. Appl Sci (Basel). (2019) 9(8):1645. doi: 10.3390/app9081645
71. Neguț A, Jurma AM, David D. Virtual-reality-based attention assessment of ADHD: clinicaVR: classroom-CPT versus a traditional continuous performance test. Child Neuropsychol. (2017) 23(6):692–712. doi: 10.1080/09297049.2016.1186617
72. Nolin P, Stipanicic A, Henry M, Lachapelle Y, Lussier-Desrochers D, Rizzo AS, et al. ClinicaVR: classroom-CPT: a virtual reality tool for assessing attention and inhibition in children and adolescents. Comput Human Behav. (2016) 59:327–33. doi: 10.1016/j.chb.2016.02.023
73. Sabb FW, Hellemann G, Lau D, Vanderlan JR, Cohen HJ, Bilder RM, et al. High-throughput cognitive assessment using BrainTest.org: examining cognitive control in a family cohort. Brain Behav. (2013) 3(5):552–61. doi: 10.1002/brb3.158
74. Zulueta A, Díaz-Orueta U, Crespo-Eguilaz N, Torrano F. Virtual reality-based assessment and rating scales in ADHD diagnosis. Psicología Educativa. (2019) 25(1):13–22. doi: 10.5093/psed2018a18
75. Rodríguez C, Areces D, García T, Cueli M, González-Castro P. Comparison between two continuous performance tests for identifying ADHD: traditional vs. virtual reality. Int J Clin Health Psychol. (2018) 18(3):254–63. doi: 10.1016/j.ijchp.2018.06.003
76. MacLeod CM. Half a century of research on the stroop effect: an integrative review. Psychol Bull. (1991) 109(2):163. doi: 10.1037/0033-2909.109.2.163
77. Aflalo J, Caldani S, Acquaviva E, Moscoso A, Delorme R, Bucci MP. Pilot study to explore poor visual searching capabilities in children with ADHD: a tablet-based computerized test battery study. Nord J Psychiatry. (2023) 77(5):491–7. doi: 10.1080/08039488.2022.2162122
78. Arakawa R, Ahuja K, Mak K, Thompson G, Shaaban S, Lindhiem O, et al. Lemurdx: using unconstrained passive sensing for an objective measurement of hyperactivity in children with no parent input. Proc ACM Interact Mob Wearable Ubiquitous Technol. (2023) 7(2):1–23. doi: 10.1145/3596244
79. Brkić D, Ng-Cordell E, O'Brien S, Martin J, Scerif G, Astle D, et al. Farmapp: a new assessment of cognitive control and memory for children and young people with neurodevelopmental difficulties. Child Neuropsychol. (2022) 28(8):1097–115. doi: 10.1080/09297049.2022.2054968
80. Cazzato D, Castro SM, Agamennoni O, Fernández G, Voos H. A non-invasive tool for attention-deficit disorder analysis based on gaze tracks. Proceedings of the 2nd International Conference on Applications of Intelligent Systems (2019). doi: 10.1145/3309772.3309777
81. Cho YJ, Yum JY, Kim K, Shin B, Eom H, Hong YJ, et al. Evaluating attention deficit hyperactivity disorder symptoms in children and adolescents through tracked head movements in a virtual reality classroom: the effect of social cues with different sensory modalities. Front Hum Neurosci. (2022) 16:943478. doi: 10.3389/fnhum.2022.943478
82. Delgado-Gómez D, Sújar A, Ardoy-Cuadros J, Bejarano-Gómez A, Aguado D, Miguelez-Fernandez C, et al. Objective assessment of attention-deficit hyperactivity disorder (ADHD) using an infinite runner-based computer game: a pilot study. Brain Sci. (2020) 10(10):716. doi: 10.3390/brainsci10100716
83. Gardner M, Metsis V, Becker E, Makedon F. Modeling the effect of attention deficit in game-based motor ability assessment of cerebral palsy patients. Proceedings of the 6th International Conference on Pervasive Technologies Related to Assistive Environments (2013). doi: 10.1145/2504335.2504405
84. Luo J, Huang H, Wang S, Yin S, Chen S, Guan L, et al. A wearable diagnostic assessment system vs. SNAP-IV for the auxiliary diagnosis of ADHD: a diagnostic test. BMC Psychiatry. (2022) 22(1):415. doi: 10.1186/s12888-022-04038-3
85. Kim WP, Kim HJ, Pack SP, Lim JH, Cho CH, Lee HJ. Machine learning-based prediction of attention-deficit/hyperactivity disorder and sleep problems with wearable data in children. JAMA Netw Open. (2023) 6(3):e233502–e233502. doi: 10.1001/jamanetworkopen.2023.3502
86. Krichmar JL, Chou T-S. A tactile robot for developmental disorder therapy. Proceedings of the Technology, Mind, and Society (2018). doi: 10.1145/3183654.3183657
87. Lee W, Lee D, Lee S, Jun K, Kim MS. Deep-learning-based ADHD classification using children’s skeleton data acquired through the ADHD screening game. Sensors. (2022) 23(1):246. doi: 10.3390/s23010246
88. Lee W, Lee S, Lee D, Jun K, Ahn DH, Kim MS. Deep learning-based ADHD and ADHD-RISK classification technology through the recognition of children’s abnormal behaviors during the robot-led ADHD screening game. Sensors. (2022) 23(1):278. doi: 10.3390/s23010278
89. Lindhiem O, Goel M, Shaaban S, Mak KJ, Chikersal P, Feldman J, et al. Objective measurement of hyperactivity using mobile sensing and machine learning: pilot study. JMIR Form Res. (2022) 6(4):e35803. doi: 10.2196/35803
90. Loleska S, Pop-Jordanova N. Web platform for gathering and analyzing data from the neurogame mobile application. Pril (Makedon Akad Nauk Umet Odd Med Nauki). (2023) 44(2):189–201. doi: 10.2478/prilozi-2023-0040
91. Santos FEG, Bastos APZ, Andrade LCV, Revoredo K, Mattos P. Assessment of ADHD through a computer game: an experiment with a sample of students. 2011 Third International Conference on Games and Virtual Worlds for Serious Applications (2011). p. 104–11. doi: 10.1109/VS-GAMES.2011.21
92. Seesjärvi E, Puhakka J, Aronen ET, Lipsanen J, Mannerkoski M, Hering A, et al. Quantifying ADHD symptoms in open-ended everyday life contexts with a new virtual reality task. J Atten Disord. (2022) 26(11):1394–411. doi: 10.1177/10870547211044214
93. Jylkkä J, Ritakallio L, Merzon L, Kangas S, Kliegel M, Zuber S, et al. Assessment of goal-directed behavior and prospective memory in adult ADHD with an online 3D videogame simulating everyday tasks. Sci Rep. (2023) 13(1):9299. doi: 10.1038/s41598-023-36351-6
94. Merzon L, Pettersson K, Aronen ET, Huhdanpää H, Seesjärvi E, Henriksson L, et al. Eye movement behavior in a real-world virtual reality task reveals ADHD in children. Sci Rep. (2022) 12(1):20308. doi: 10.1038/s41598-022-24552-4
95. Son HM, Lee DG, Joung YS, Lee JW, Seok EJ, Chung TM, et al. A novel approach to diagnose ADHD using virtual reality. Int J Web Inf Syst. (2021) 17(5):516–36. doi: 10.1108/IJWIS-03-2021-0021
96. Stokes JD, Rizzo A, Geng JJ, Schweitzer JB. Measuring attentional distraction in children with ADHD using virtual reality technology with eye-tracking. Front Virtual Real. (2022) 3:855895. doi: 10.3389/frvir.2022.855895
97. Ulberstad F, Boström H, Chavanon M-L, Knollmann M, Wiley J, Christiansen H, et al. Objective measurement of attention deficit hyperactivity disorder symptoms outside the clinic using the QbCheck: reliability and validity. Int J Methods Psychiatr Res. (2020) 29(2):e1822. doi: 10.1002/mpr.1822
98. Wehmeier PM, Schacht A, Wolff C, Otto WR, Dittmann RW, Banaschewski T. Neuropsychological outcomes across the day in children with attention-deficit/hyperactivity disorder treated with atomoxetine: results from a placebo-controlled study using a computer-based continuous performance test combined with an infra-red motion-tracking device. J Child Adolesc Psychopharmacol. (2011) 21(5):433–44. doi: 10.1089/cap.2010.0142
99. Chen Y, Zhang Y, Jiang X, Zeng X, Sun R, Yu H. COSA: contextualized and objective system to support ADHD diagnosis. Proceedings - 2018 IEEE International Conference on Bioinformatics and Biomedicine, BIBM 2018 (2019). p. 1195–202. doi: 10.1109/BIBM.2018.8621308
100. Wiguna T, Bahana R, Dirgantoro B, Minayati K, Teh SD, Ismail RI, et al. Developing attention deficits/hyperactivity disorder-virtual reality diagnostic tool with machine learning for children and adolescents. Front Psychiatry. (2022) 13:984481. doi: 10.3389/fpsyt.2022.984481
101. Ginsberg Y, Quintero J, Anand E, Casillas M, Upadhyaya HP. Underdiagnosis of attention-deficit/hyperactivity disorder in adult patients: a review of the literature. Prim Care Companion J Clin Psychiatry. (2014) 16:3. doi: 10.4088/PCC.13r01600
102. Faraone SV, Sergeant J, Gillberg C, Biederman J. The worldwide prevalence of ADHD: is it an American condition? World Psychiatry. (2003) 2(2):104–13.16946911
103. Cibrian FL, Lakes KD, Schuck S, Tavakoulnia A, Guzman K, Hayes G. Balancing caregivers and children interaction to support the development of self-regulation skills using a smartwatch application. UbiComp/ISWC 2019- - Adjunct Proceedings of the 2019 ACM International Joint Conference on Pervasive and Ubiquitous Computing and Proceedings of the 2019 ACM International Symposium on Wearable Computers (2019). p. 459–60. doi: 10.1145/3341162.3345612
104. Doan M, Cibrian F, Jang A, Khare N, Chang S, Li A, et al.. Coolcraig?: a smart watch/phone application supporting co-regulation of children with ADHD. Adjunt CHI Conference on Human Factors in Computing Systems (2020).
105. Tavakoulnia A, Guzman K, Cibrian FL, Lakes KD, Hayes G, Schuck SEB. Designing a wearable technology application for enhancing executive functioning skills in children with ADHD. UbiComp/ISWC 2019- - Adjunct Proceedings of the 2019 ACM International Joint Conference on Pervasive and Ubiquitous Computing and Proceedings of the 2019 ACM International Symposium on Wearable Computers (2019). p. 222–5. doi: 10.1145/3341162.3343819
106. Silva LM, Cibrian FL, Monteiro E, Bhattacharya A, Beltran JA, Bonang C, et al.. Unpacking the lived experiences of smartwatch mediated self and co-regulation with ADHD children. In Proceedings of the 2023 CHI Conference on Human Factors in Computing Systems (2023). p. 1–19
107. Silva LM, Cibrian FL, Bonang C, Bhattacharya A, Min A, Monteiro EM, et al.. Co-Designing situated displays for family co-regulation with ADHD children. In Proceedings of the CHI Conference on Human Factors in Computing Systems (2024). p. 1–19
Keywords: ADHD, assessment, digital health, computer, technology, attention, behavior, hyperactivity
Citation: Cibrian FL, Monteiro EM and Lakes KD (2024) Digital assessments for children and adolescents with ADHD: a scoping review. Front. Digit. Health 6:1440701. doi: 10.3389/fdgth.2024.1440701
Received: 29 May 2024; Accepted: 6 September 2024;
Published: 8 October 2024.
Edited by:
Stephane Meystre, University of Applied Sciences and Arts of Southern Switzerland, SwitzerlandReviewed by:
Habtamu Alganeh Guadie, Bahir Dar University, EthiopiaFrancesca Faraci, University of Applied Sciences and Arts of Southern Switzerland, Switzerland
Copyright: © 2024 Cibrian, Monteiro and Lakes. This is an open-access article distributed under the terms of the Creative Commons Attribution License (CC BY). The use, distribution or reproduction in other forums is permitted, provided the original author(s) and the copyright owner(s) are credited and that the original publication in this journal is cited, in accordance with accepted academic practice. No use, distribution or reproduction is permitted which does not comply with these terms.
*Correspondence: Franceli L. Cibrian, Y2licmlhbkBjaGFwbWFuLmVkdQ==