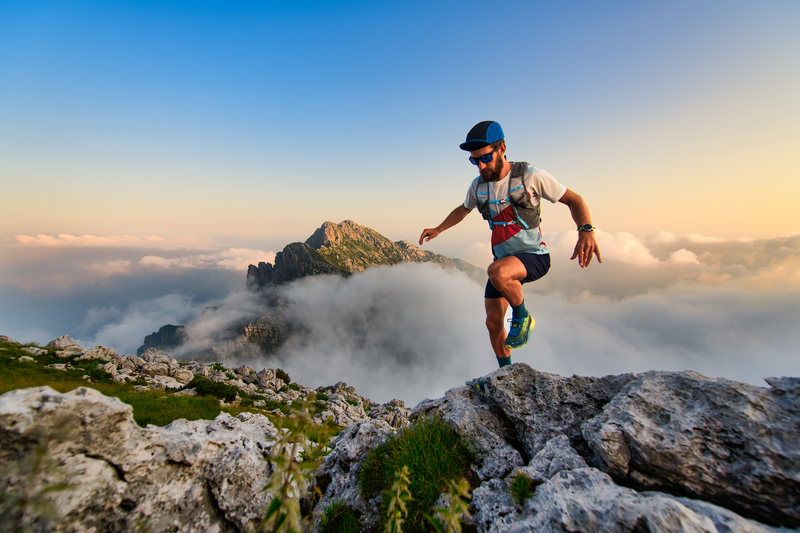
94% of researchers rate our articles as excellent or good
Learn more about the work of our research integrity team to safeguard the quality of each article we publish.
Find out more
EDITORIAL article
Front. Digit. Health , 12 December 2023
Sec. Health Informatics
Volume 5 - 2023 | https://doi.org/10.3389/fdgth.2023.1340517
This article is part of the Research Topic Human-Centred Computer Audition: Sound, Music, and Healthcare View all 11 articles
Editorial on the Research Topic
Human-centred computer audition: sound, music, and healthcare
At the time of writing this editorial, OpenAI has announced its newest model called chatGPT-4 Turbo.1 When dreaming for the blue print that we can better the life via this revolution of AI technologies by foundation models, it is a time for almost every person to think how to live with the powerful artificial intelligence (AI) models in the future.
A future that may also challenge our societies and current living in many ways (1) including or even particularly in healthcare (2). Thinking especially of audio, a similar rise of increasingly capable and powerful foundation models appears at highly accelerated pace and with increasingly emergent behaviour. One of the latest at the time of writing is Uniaudio—showing an overly impressive range of zero-shot abilities (3).
For a long time in the field of health, machines have been taught to “see” and/or to “read” rather than to “listen.” This is one of the reasons why more progress was achieved in the field of computer vision (CV) and natural language processing (NLP) rather than computer audition (CA) in this domain. Nevertheless, the promising contributions of audio cannot be ignored for its excellent performance in healthcare (4).
Motivated by the concept of human-centred AI (HAI), we organised the research topic on “Human-Centred Computer Audition: Sound, Music, and Healthcare,” which lasted from April 2021 to January 2023. Finally, 10 articles were accepted and published after a rigorous peer-review process. There are 57 authors involved in this research topic.
In the remainder of this editorial, we will briefly introduce the published research articles in this research topic collection. Then, insights and perspectives will be given towards the future work.
The published contributions have covered the planned scope, e.g., computational analysis of sound scenes and events, digital music, computer audition for healthcare, computational paralinguistics, and explainable AI in computer audition. In the following, grouped by categories, we provide a brief description of the collected articles.
Whether audio could serve as a novel digital phenotype for detection of COVID-19 has been increasingly studied in the past three years (5, 6). Coppock et al. summarise the contributions in the organised INTERSPEECH 2021 Computational Paralinguistics Challenges: COVID-19 Cough, (CCS) and COVID-19 Speech, (CSS) (7). They indicated that, a classifier trained by the infected individuals’ respiratory sounds can achieve moderate detection rates of COVID-19. However, whether the audio biomarkers in respiratory sounds of infected individuals are unique for COVID-19 or not is still a question to be answered. Chang et al. introduced a “CovNet” which uses a transfer learning framework to improve the models’ generalisation. Experimental results show their models’ efficiency by considering a parameter transferring strategy and an embedding incorporation strategy. Akman et al. propose an end-to-end deep neural network model (called “CIdeR”) for exploring the methodological adaptation to new datasets with different modalities. From the experiments, their proposed model can serve across multiple audio types. However, they found that it is difficult to train a common COVID-19 classifier due to the limitations of a joint usage of datasets.
Audio tagging of domestic activities can provide important information on health and wellbeing. Yang et al. present an explainable tensor network for monitoring domestic activities via audio signals. They indicated that, the combination of the tensor network can reduce the redundancy of the network.
Music therapy appears promising for its non-drug characteristic, specifically for treatment of mental disorders (8). However, the influences of music on the brain are still an open question to be answered. Wei et al. contribute a review on neurocognition for timbre perception. They conclude that, timbre perception is promising in psychological application. Further. Liu et al. studied timbre fusion of Chinese and Western instruments. This bears interest, given that in a recent study, timbre features are found to be strongly associated with the human affective states (9). Next, Miyamoto et al. introduce a meta-learning strategy in a music generation system. More fundamentally, Corona-González et al. presented a study on personalised theta and beta binaural beats for brain entrainment. The conclusion made is that the neural resynchronisation was met with both personalised theta and beta binaural beats whereas there seemed to be no different mental conditions achieved.
A disyllabic corpus that could be used to examine the performance of pitch recognition of cochlear implant users was introduced. Wang et al. found that, higher scores of tone recognition tend to be achieved by listeners with longer cochlear implant listening experience.
Speech emotion recognition is a widely-studied field in affective computing. The combination of task-specific speech enhancement and data augmentation as a strategy has been used for improving the overall multimodal emotion recognition in noisy conditions. This contribution of Kshirsagar et al. can benefit the speech-based affective information retrieval task in real-world applications.
When reading over the collection of this research topic, one finds promising potential of computer audition that can benefit manifold health-related aspects of our life. However, one needs to fully consider the current limitations and keep an eye on the future progress of computer audition.
First, data scarcity is still a serious challenge (10) that constrains the fast development of audio based large models. The hardware limitations and further factors impede the collection of high-quality audio data at large scale which could provide sufficient training for current state-of-the-art large models in this domain. Besides, the annotation of audio data (specifically for medical applications) is often difficult. Therefore, advanced strategies such as meta-learning (11), and self-supervised learning should be taken into account prior to the event of generalist (medical) AI (12).
Second, fundamental studies on features, models, and strategies are of interest but limited. Among this collection, we can see some contributions focus on extracting novel audio features to improve the performance of models. We hope to see more works in the future towards the interpretation of the models (13).
Third, the mechanism of the brain’s perception of audio is worth exploring in considerably more depth. It will not only be beneficial for building brain-inspired deep learning models, but also for our understanding more deeply music/audio therapy.
Last but not the least, how to leverage the power of the coming large models to discover more possibilities of computer audition is an open question to be answered.
KQ: Writing – original draft, Writing – review & editing; GF: Writing – review & editing; SL: Writing – review & editing; ZL: Writing – review & editing; BS: Writing – original draft, Writing – review & editing.
The author(s) declare financial support was received for the research, authorship, and/or publication of this article.
This work was partially supported by the Ministry of Science and Technology of the People’s Republic of China with the STI2030-Major Projects 2021ZD0201900, the National Natural Science Foundation of China (No. 62272044), and the Teli Young Fellow Program from the Beijing Institute of Technology, China.
The authors declare that the research was conducted in the absence of any commercial or financial relationships that could be construed as a potential conflict of interest.
1https://openai.com/blog/new-models-and-developer-products-announced-at-devday
All claims expressed in this article are solely those of the authors and do not necessarily represent those of their affiliated organizations, or those of the publisher, the editors and the reviewers. Any product that may be evaluated in this article, or claim that may be made by its manufacturer, is not guaranteed or endorsed by the publisher.
1. Peters MA, Jackson L, Papastephanou M, Jandrić P, Lazaroiu G, Evers CW, et al. AI, the future of humanity: ChatGPT-4, philosophy, education—critical responses. Educ Philos Theory. (2023) 1–35.
2. Wornow M, Xu Y, Thapa R, Patel B, Steinberg E, Fleming S, et al. The shaky foundations of large language models and foundation models for electronic health records. npj Digit Med. (2023) 6:135. doi: 10.1038/s41746-023-00879-8
3. Yang D, Tian J, Tan X, Huang R, Liu S, Chang X, et al. UniAudio: an audio foundation model toward universal audio generation [Preprint] (2023). Available at: https://doi.org/10.48550/arXiv.2310.00704
4. Qian K, Li X, Li H, Li S, Li W, Ning Z, et al. Computer audition for healthcare: opportunities and challenges. Front Digit Health. (2020) 2:1–4. doi: 10.3389/fdgth.2020.00005
5. Coppock H, Jones L, Kiskin I, Schuller BW. COVID-19 detection from audio: seven grains of salt. Lancet Digit Health. (2021) 3:e537–8. doi: 10.1016/S2589-7500(21)00141-2
6. Deshpande G, Batliner A, Schuller BW. AI-based human audio processing for COVID-19: a comprehensive overview. Pattern Recognit. (2022) 122:1–10. doi: 10.1016/j.patcog.2021.108289
7. Schuller B, Batliner A, Bergler C, Mascolo C, Han J, Lefter I. COVID-19 cough, COVID-19 speech, escalation & primates. Proc. INTERSPEECH; Brno, Czechia (2021). p. 431–5.
8. Qian K, Schuller BW, Guan X, Hu B. Intelligent music intervention for mental disorders: insights and perspectives. IEEE Trans Comput Soc Syst. (2023) 10:2–9. doi: 10.1109/TCSS.2023.3235079
9. Luo G, Sun S, Qian K, Hu B, Schuller BW, Yamamoto Y, et al. How does music affect your brain? A pilot study on EEG, music features for automatic analysis. Proc. EMBC; Sydney, Australia. IEEE (2023). p. 1–4.
10. Alzubaidi L, Bai J, Al-Sabaawi A, Santamaría J, Albahri A, Al-dabbagh BSN, et al. A survey on deep learning tools dealing with data scarcity: definitions, challenges, solutions, tips, and applications. J Big Data. (2023) 10:46. doi: 10.1186/s40537-023-00727-2
11. Vettoruzzo A, Bouguelia MR, Vanschoren J, Rögnvaldsson T, Santosh K. Advances challenges in meta-learning: a technical review [Preprint] (2023). Available at: https://doi.org/10.48550/arXiv.2307.04722
12. Moor M, Banerjee O, Abad ZSH, Krumholz HM, Leskovec J, Topol EJ, et al. Foundation models for generalist medical artificial intelligence. Nature. (2023) 616:259–65. doi: 10.1038/s41586-023-05881-4
13. Frommholz A, Seipel F, Lapuschkin S, Samek W, Vielhaben J. XAI-based comparison of input representations for audio event classification [Preprint] (2023). https://doi.org/10.48550/arXiv.2304.14019
Keywords: computer audition, machine learning, deep learning, artificial intelligence, brain sciences
Citation: Qian K, Fazekas G, Li S, Li Z and Schuller BW (2023) Editorial: Human-centred computer audition: sound, music, and healthcare. Front. Digit. Health 5:1340517. doi: 10.3389/fdgth.2023.1340517
Received: 18 November 2023; Accepted: 21 November 2023;
Published: 12 December 2023.
Edited and Reviewed by: Uwe Aickelin, The University of Melbourne, Australia
© 2023 Qian, Fazekas, Li, Li and Schuller. This is an open-access article distributed under the terms of the Creative Commons Attribution License (CC BY). The use, distribution or reproduction in other forums is permitted, provided the original author(s) and the copyright owner(s) are credited and that the original publication in this journal is cited, in accordance with accepted academic practice. No use, distribution or reproduction is permitted which does not comply with these terms.
*Correspondence: Kun Qian cWlhbkBiaXQuZWR1LmNu
Disclaimer: All claims expressed in this article are solely those of the authors and do not necessarily represent those of their affiliated organizations, or those of the publisher, the editors and the reviewers. Any product that may be evaluated in this article or claim that may be made by its manufacturer is not guaranteed or endorsed by the publisher.
Research integrity at Frontiers
Learn more about the work of our research integrity team to safeguard the quality of each article we publish.